Prediction and analysis of osteoarthritis hub genes with bioinformatics
Highlight box
Key findings
• Analysis of cartilage samples for osteoarthritis based on known biological information identified differential genes, providing potential biomarkers and therapeutic targets for osteoarthritis.
What is known and what is new?
• Osteoarthritis still has no definitive biomarkers for a definitive diagnosis, and there are no definitive drugs available for its treatment.
• Comparative access to biomarkers of osteoarthritis using biological information from known transcriptomes may be an effective solution to this problem.
What is the implication, and what should change now?
• The discovery of biomarkers for osteoarthritis can help drug development and clinicians to detect osteoarthritis at an early stage for early diagnosis and treatment.
Introduction
Osteoarthritis (OA) is the most common form of arthritis worldwide, affecting approximately 40% of people over 70 years old (1). According to the World Health Organization, the number of people with OA will double by 2025 and again by 2050. OA can cause joint pain, stiffness, and loss of function. It is characterized by cartilage erosion, osteophyte formation, subchondral bone formation, and synovial inflammation due to biomechanical and biochemical joint changes (2). Since the first description of hip OA in 1793 (3), a large amount of in-depth research has been done on its pathophysiology (4-6). To date, the “gold standard” for clinical diagnosis of OA is still imageology. However, there is a lag in imaging examinations, which often require 1–3 years to obtain reliable information on progression (7).
As high throughput sequencing technology has advanced and costs have fallen, bioinformatics analysis is now widely used to identify disease-specific biomarkers (7-10) for screening, diagnosis, prediction, and identification of drug targets of tumor genes. In recent years, researchers have reported the use of bioinformatics and microarray technology to screen the genes and signal transduction pathways of OA, providing theoretical support for the pathogenesis of OA and new therapeutic targets (11,12). Although the pathogenesis of OA is not fully known, we can refer to its main risk factors (13). Cartilage tissue in OA may be the first to undergo pathophysiological changes, including cartilage matrix proteolysis, cartilage cell erosion, and fibrosis, while the release of collagen fragments and proteoglycans into synovial fluid leads to synovial tissue inflammation (14). Data from current bioinformatics studies on OA are mostly from synovial tissue (15,16) and peripheral blood (11), and some scholars believe that although inflammatory response as a major mechanism may be involved in the pathogenesis of OA (17), local or systemic reactions are affected by other factors.
Many driving factors (18-20) have been found to induce OA development, including mechanical injury, aging, cartilage degeneration, and synovitis, among others. A major challenge for researchers is applying bioinformatics to find the switch that drives OA initiation in a wide variety of sample types so that it can be used to improve the early diagnosis and prediction of OA. Therefore, we speculated that looking for “the Big Bang” might be the key to better understanding OA. Further, bioinformatics is usually calculated by the cytoHubba plug-in of Cytoscape software, which contains many kinds of calculation methods. Most previous research has involved a single method (15,21), and it is unclear whether there are differences in the hub genes obtained by the various calculation methods.
To address the above problems, we employed biological information analysis of OA cartilage tissue rather than synovium and blood samples. We then performed cluster analysis of differential genes, and grouped and compared functional paths. In addition, we selected multiple computational models for the screening of hub genes to avoid the bias of using a single model. Finally, protein-protein interaction (PPI) analysis of the acquired hub genes was conducted to provide a more intuitive understanding of their interrelationships. Figure 1 shows a workflow of the steps undertaken in this study. We present the following article in accordance with the STREGA reporting checklist (available at https://atm.amegroups.com/article/view/10.21037/atm-22-6450/rc).
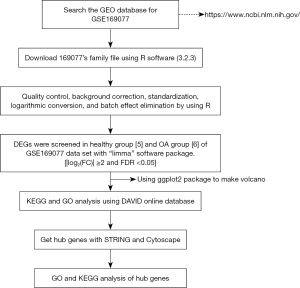
Methods
Data sources
The GSE169077 dataset was downloaded from the National Biotechnology Information Center’s Gene Expression Omnibus (GEO) public database (https://www.ncbi.nlm.nih.gov/geo/) (22). The chip platform used was the GPL96 (HG-U133A) Affymetrix Human Genome U133A Array. The study used data sets from 11 knee replacement surgery patients. The patient data in this work were obtained from a public database, which included informed consent and ethical approval. The study was conducted in accordance with the Declaration of Helsinki (as revised in 2013).
Screening of differentially expressed genes (DEGs)
R version 3.2.3 (The R Project for Statistical Computing, Vienna, Austria) was used to analyze and read the chip and download the matrix file. The “affy” (23) and “limma” packages (24) were employed for quality control, background correction, standardization, logarithmic conversion, and batch effect elimination. Samples without clinical data were filtered, and the rest of the data were analyzed. The “limma” software package was used to screen DEGs among 5 normal controls and 6 OA samples from the GSE169077 dataset. An absolute value of the log2 difference multiple [fold change (FC)] ≥2 and false discovery rate (FDR) <0.05 were set as the thresholds for screening DEGs (25). The “ggplot2” package was utilized to draw the volcano map for the obtained DEG data (26).
Statistical analysis
After gene expression quantification is completed, statistical analysis of their expression data is required to screen the samples for genes with significantly different expression levels in different states. First, to correct for the sequencing depth, we normalized the original readcount; then the statistical model was used to calculate the probability of hypothesis testing (P value), and finally multiple hypothesis testing was performed to correct for the FDR.
Enrichment analysis of the DEGs
The online database for annotation, visualization, and integrated discovery (DAVID, https://david.ncifcrf.gov) (27) was used to perform gene ontology (GO) (28) and Kyoto Encyclopedia of Gene and Genomes (KEGG) pathway enrichment analysis (29). GO functional enrichment data were annotated and classified according to the biological pathway (BP), cellular composition (CC), and molecular function (MF). KEGG enrichment analysis was performed to identify the possible biological pathways. Using the Expression Analysis Systematic Explorer (EASE) score statistical method (30), the screening conditions of the GO and KEGG data were set at a P value <0.05. The upregulated and downregulated DEGs were explored with GO and KEGG to analyze the effects of the two kinds of DEGs on OA. The “ggplot2” package was used to draw the visualization chart based on results of the analyses.
DEG network construction and hub gene screening
The search tool for the retrieval of interacting genes/proteins (STRING version 11.5, https://string-db.org/), an online analysis tool (31), was utilized to present and evaluate the protein interaction (protein-protein interaction, PPI) network. The 27 DEGs selected in this study were imported into the STRING website, and the potential relationships among these DEGs were further explored using STRING analysis tools. The filter condition was set as follows: network type selected; “full-STRING network”; confidence ≥ medium confidence (0.4). The calculation results from STRING were imported into Cytoscape version 3.8.2 (Cytoscape Consortium, San Diego, CA, USA) (32). The hub genes (33) in key positions in the PPI network were screened with a variety of algorithms through the cytoHubba plug-in of Cytoscape (34). The obtained hub genes were reintroduced into the STRING website to determine the PPI interaction (35).
Results
Results of the downloaded data
The analyzed data included 11 samples, including 6 OA cartilage tissues and 5 normal cartilage tissues. The fresh OA cartilage tissue was washed with aseptic phosphate-buffered saline and immediately frozen in liquid nitrogen. The samples were preserved at −140 ℃ until the extraction of RNA. Healthy cartilage tissue was acquired from the National Center for Disease Research and Exchange (National Disease Research Interchange, NDRI). Eigenvalues of the main components in each sample were analyzed through covariance matrix analysis (36), and 3-dimensional principal component analysis diagrams were drawn (Figure 2).
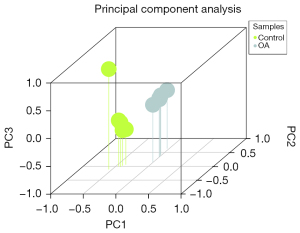
DEG screening results
A total of 31 DEGs from the OA and control groups were identified. After weight removal, 27 DEGs were retained (Table S1), including 18 and 9 upregulated and downregulated genes, respectively (Table 1). A volcanic map (Figure 3) was used to analyze and observe the |log2(FC)| distribution of the DEGs showing changes of more than 2 times. The upregulated and downregulated genes were marked in red and blue, respectively. The genes with |log2(FC)| values less than 2 or P<0.05 were categorized as indifferent genes and marked in gray. The DEGs in each sample were depicted by a heat map, which presented upregulated and downregulated genes as red and green, respectively (Figure 4).
Table 1
Gene ID | Description | Log2(FC) |
---|---|---|
DDIT4 | DNA damage inducible transcript 4 | 4.248 |
TSC22D3 | TSC22 domain family member 3 | 3.665 |
TMOD1 | Tropomodulin 1 | 3.632 |
HILPDA | Hypoxia inducible lipid droplet associated | 3.578 |
CYP4B1 | Cytochrome P450 family 4 subfamily B member 1 | 3.572 |
PCK1 | Phosphoenolpyruvate carboxykinase 1 | 3.511 |
PDK4 | Pyruvate dehydrogenase kinase 4 | 3.281 |
PDE2A | Phosphodiesterase 2A | 3.193 |
ADM | Adrenomedullin | 3.135 |
GAB2 | GRB2 associated binding protein 2 | 2.831 |
C10orf10 | Chromosome 10 open reading frame 10 | 2.828 |
STC2 | Stanniocalcin 2 | 2.811 |
ACADL | Acyl-CoA dehydrogenase, long chain | 2.762 |
TXNIP | Thioredoxin interacting protein | 2.736 |
GLRX | Glutaredoxin | 2.689 |
GLUL | Glutamate-ammonia ligase | 2.666 |
SCNN1A | Sodium channel epithelial 1 alpha subunit | 2.654 |
GADD46B | Growth arrest and DNA damage inducible beta | 2.626 |
MXRA5 | Matrix remodeling associated 5 | −2.937 |
EPHB2 | EPH receptor B2 | −2.977 |
SERPINF1 | Serpin family F member 1 | −2.991 |
POSTN | Periostin | −3.115 |
BMP1 | Bone morphogenetic protein 1 | −3.206 |
COL1A2 | Collagen type I alpha 2 chain | −3.518 |
MMP13 | Matrix metallopeptidase 13 | −3.578 |
COL1A1 | Collagen type I alpha 2 chain | −3.622 |
HLA-DRA | Major histocompatibility complex, class II, DR alpha | −3.83 |
FC, fold change.
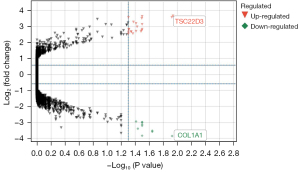
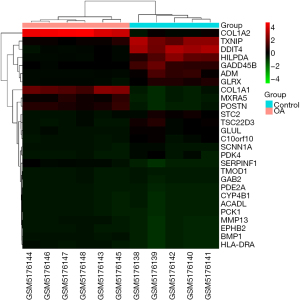
Results of GO and KEGG analysis of the DEGs
The results of GO and KEGG signal pathway enrichment analysis of the DEGs using DAVID are shown in Figure 5. In the BP category, DEGs were mainly correlated with oxygen, nutrition, and corticosteroid levels. In the CC category, DEGs were mainly enriched in fibrous collagen trimers, banded collagen fibrils, and extracellular matrices containing collagen. In the MF category DEGs were mainly involved in extracellular matrix structural components for tensile strength and platelet-derived growth factor binding. GO enrichment showed that the upregulated DEGs were mainly correlated with hypoxic response and mitochondrial matrix and magnesium ion binding. In comparison, the downregulated DEGs were mainly correlated with the extracellular matrix structure. KEGG analysis demonstrated that upregulated genes were mainly related to the peroxisome proliferator-activated receptor (PPAR) signal pathway, whereas downregulated genes were associated with protein digestion and absorption, extracellular matrix receptor interaction, and other signal pathways (Figure 6).
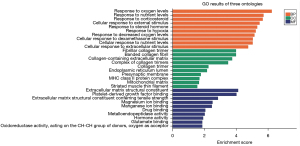
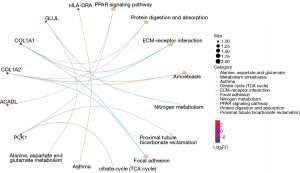
Results of the PPI network analysis of the DEGs
STRING analysis demonstrated that the PPI network consisted of 27 nodes and 22 edges. The average degree of the nodes among the networks was 1.63. The P value was 6.33e-15, and the degrees for COL1A2, COL1A1, BMP1, MXRA5, and SERPINF1 ranked the top 5. In order to better explore the pathogenesis of OA, 27 clustered DEGs were analyzed by k-means (Figure 7). These DEGs were visualized by hiding the non-interacting genes. Clusters were distinguished by 3 colors: the green group (14 genes) was primarily involved in the glucocorticoid receptor pathway, the blue group (7 genes) was involved in the relaxin signaling pathway, and the red group (6 genes) was associated with the estrogen receptor pathway (Table 2).
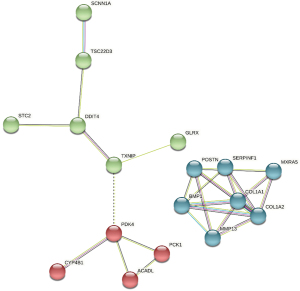
Table 2
Clusters | Genes | P value | Pathway |
---|---|---|---|
Green | ADM, C10orf10, DDIT4, EPHB2, GAB2, GADD45B, GLRX, GLUL, HILPDA, PDE2A, SCNN1A, STC2, TSC22D3, TXNIP | 3.06×10−4 | Glucocorticoid receptor pathway |
Blue | BMP1, COL1A1, COL1A2, MMP13, MXRA5, POSTN, SERPINF1 | <10−16 | Relaxin signaling pathway |
Red | ACADL, CYP4B1, HLA-DRA, PCK1, PDK4, TMOD1 | 2.59×10−5 | Estrogen receptor pathway |
DEGs, differentially expressed genes.
The results of the PPI network were imported into Cytoscape, and the 27 DEGs were sequenced by the cytoHubba plug-in according to various calculation methods. Ten candidate hub genes were selected, and 5 hub genes, namely, COL1A1, COL1A2, POSTN, BMP1, and MMP13, were selected after combining 6 calculation methods (MCC, DMNC, degree, closeness, clustering coefficient, and EPC) (Table 3). The 5 genes were analyzed by PPI, with a total of 5 nodes and 10 interaction networks on the left side. We performed heatmap analysis on the 5 hub genes and found significant differences between the OA and normal groups (Figure 8). The average degree of the nodes among the networks was 4. The P value was 3.83e-13, which was annotated by GO and KEGG in different colors (Figure 9). In the cluster grouping, the genes contained were the hub genes in the blue group (Table 2).
Table 3
Rank | MCC | DMNC | Degree | Closeness | Clustering coefficient | EPC |
---|---|---|---|---|---|---|
1 | COL1A1* | BMP1 | COL1A1* | COL1A1* | MMP13 | COL1A2* |
2 | COL1A2* | MMP13 | COL1A2* | COL1A2* | MXRA5 | COL1A1* |
3 | POSTN* | MXRA5 | POSTN* | POSTN* | SERPINF1 | POSTN* |
4 | BMP1* | SERPINF1 | BMP1* | BMP1* | PCK1 | BMP1* |
5 | MMP13* | POSTN | MMP13* | MMP13* | ACADL | MMP13* |
6 | MXRA5 | COL1A1 | MXRA5 | MXRA5 | PDK4 | MXRA5 |
7 | SERPINF1 | COL1A2 | SERPINF1 | SERPINF1 | BMP1 | SERPINF1 |
8 | PCK1 | PCK1 | PCK1 | PCK1 | POSTN | PCK1 |
9 | ACADL | ACADL | ACADL | ACADL | COL1A2 | ACADL |
10 | PDK4 | PDK4 | PDK4 | PDK4 | COL1A1 | PDK4 |
*, the gene ID was screened for hub genes in this study. MCC, Maximal Clique Centrality; DMNC, Density of Maximum Neighborhood Component; EPC, Edge Percolated Component.
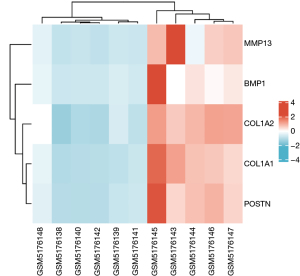
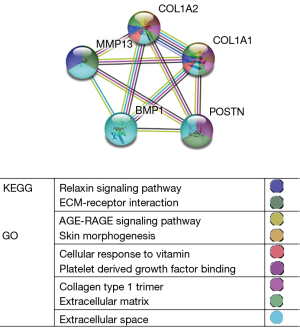
Discussion
In 2015, the Osteoarthritis Research Society International (OARSI) proposed a new definition of arthritis (37) to refer to cellular stress and extracellular matrix degeneration caused by microscopic and macroscopic damage. These involve preinflammatory pathways of innate immunity, which is primarily a molecular disorder, followed by anatomical and/or physiological disorders, including cartilage degeneration, bone remodeling, and osteophyte formation. Therefore, it is particularly important to understand the physiological and pathological changes of OA at molecular level.
In this study, we focused on the cartilage of OA, selected the GSE169077 dataset in the GEO database, and obtained 27 DEGs using R software. We used STRING to cluster the 27 DEGs, of which the blue cluster group contained all of the hub genes involved in the relaxin signaling pathway. Relaxin is a peptide hormone discovered by Frederick Hisaw in 1926 in the study of changes in the pelvic canal during pregnancy. It is mainly produced in the ovarian corpus luteum of pregnant mammals and the male prostate. As the research progressed, researchers found relaxin in the heart, liver, lungs, and kidneys was closely related to various functions and diseases, such as regulation of the uterus during pregnancy, lowering blood pressure and vascular resistance, and anti-pulmonary fibrosis and liver fibrosis. Clifton’s study of thumb arthritis found that matrix metalloproteinases (MMPs) could act as a potential target for relaxin, which in turn affects joint stability (38). In addition, Ko et al. showed that relaxin antagonizes synovial fibroblasts in OA, thereby preventing the flexion contracture of OA (39). The role of the relaxin signaling pathway in the development of OA cartilage should be further explored.
The upregulated genes involved in BP were mainly concerned with hypoxic response, while the genes involved in CC were correlated with mitochondrial matrix enrichment. The identified DEGs were not concentrated in critical pathways, but GO identified 3 genes involved in the extracellular matrix, while KEGG identified genes involved in protein digestion and absorption. Downregulated DEGs were analyzed by cytoHubba and utilized as the hub genes in this study (Figures 5,6).
Among the 5 hub genes, COL1A1 and COL1A2 are major collagen producers and important regulatory genes for bone, skin, and other connective tissues. Fang et al. suggested that they may be important biomarkers for hydroxymethylation and could be used as target genes for accurate diagnosis and treatment of OA (40). Another study proposed that increased secretion of type I collagen encoded by homologous COL1A1 and COL1A2 trimers could lead to subchondral bone stenosis and collagen fiber disorganization in OA (41).
MMPs are zinc-dependent extracellular proteases, the main function of which is to degrade extracellular interstitial components (42). Recent studies have found that MMP13 prevents articular cartilage damage through binding to metalloproteinase tissue inhibitor (43), which may be a potential treatment target for OA. In addition, Wang et al. found that an MMP13 inhibitor could reduce the severity of meniscus and ligament injury in an induced OA mice model (44).
Periostin (POSTN) is a protein-coding gene functioning as a transforming growth factor β-induced extracellular matrix protein. It is also known as osteoblast-specific factor 2 and is expressed in articular joint components such as cartilage, subchondral bone, meniscus, and ligaments (45). POSTN can be crosslinked with other extracellular matrix proteins, such as type I collagen and fibronectin. The study has found that mice with POSTN deletion exhibit collagen crosslinking defects and a reduced resistance to mechanical stress (46). In the study by Tajika et al., POSTN did not upregulate collagen-related genes such as COL1 and COL2. It is speculated that POSTN might indirectly affect collagen-related genes, for example, COL1 and COL2, through regulating the expression of MMP13 (47).
In this study, bone morphogenetic protein 1 (BMP1) was a pivotal gene, which plays a variety of roles in regulating extracellular matrix formation. BMP1 preprocesses functional structural proteins in the extracellular matrix of active enzymes, binds with collagen I and II, and regulates the size and shape of heteromorphic fibers. Studies have suggested direct interaction between Fas1 domains, and that BMP1 is indirectly related to the EMI domain of POSTN (48,49). Targeted deletion of the POSTN gene in mice led to reduced collagen crosslinking (46,50). Kii et al. reported that the POSTN-BMP 1-LOX axis was the basis of the mechanochemical properties of the collagen matrix (51) (Figure 10). In recent studies, POSTN was found to determine the incidence of OA in women and predicted the occurrence of posttraumatic OA (52). Our interesting finding is that POSTN, BMP1, COL1A1 and COL1A2 are all closely related to collagen synthesis. This suggests that our drug development for osteoarthritis should focus on intervening in the intermediate steps that promote collagen synthesis (Figure 10).
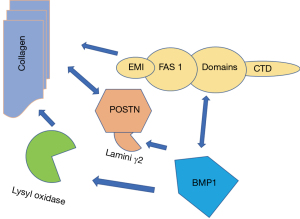
Pain is a common phenotype in osteoarthritis, and extracellular matrix (ECG) receptor interaction is significantly different in the KEGG pathway. The proteoglycan and hyaluronic acid content of the ECM has been found to be elevated in the inflammatory progression of many diseases and, due to its unique reticular fibrous structure, is thought to be a “landing zone” for inflammatory cells. “This “net” influences their adhesion, retention, migration and activation. The process of transfer and localisation of leukocytes directly determines the duration of the inflammatory response. Nanus et al. (53) studies of synovial tissue from multiple painful knee lesions found that the most differentially expressed RNA was in the inflammatory signalling, which is consistent with our findings in this study.
Our study had some limitations. Firstly, we used only 1 dataset, and there may have been bias in the search for markers for OA. Secondly, the effect of OA on cartilage metabolism is only 1 factor, and systemic inflammatory response and diabetes, among others, can affect cartilage metabolism and integrity and possibly have an effect on results. Finally, we did not carry out experiments to prove the research results, which will be considered in future work.
Conclusions
This study applied bioinformatics to analyze the RNA expression profiles of healthy and OA groups. A total of 27 DEGs were identified, and their biological functions mainly contributed to the structural components and tensile strength of the extracellular matrix. KEGG analysis demonstrated that these DEGs were mainly related to protein digestion and absorption, extracellular matrix receptor interaction, and the PPAR signaling pathway, as well as other signaling pathways. COL1A1, COL1A2, POSTN, BMP1, and MMP13 were identified as possible diagnostic and therapeutic biomarker targets for treating OA.
Acknowledgments
Funding: None.
Footnote
Reporting Checklist: The authors have completed the STREGA reporting checklist. Available at https://atm.amegroups.com/article/view/10.21037/atm-22-6450/rc
Conflicts of Interest: All authors have completed the ICMJE uniform disclosure form (available at https://atm.amegroups.com/article/view/10.21037/atm-22-6450/coif). The authors have no conflicts of interest to declare.
Ethical Statement: The authors are accountable for all aspects of the work in ensuring that questions related to the accuracy or integrity of any part of the work are appropriately investigated and resolved. The study was conducted in accordance with the Declaration of Helsinki (as revised in 2013).
Open Access Statement: This is an Open Access article distributed in accordance with the Creative Commons Attribution-NonCommercial-NoDerivs 4.0 International License (CC BY-NC-ND 4.0), which permits the non-commercial replication and distribution of the article with the strict proviso that no changes or edits are made and the original work is properly cited (including links to both the formal publication through the relevant DOI and the license). See: https://creativecommons.org/licenses/by-nc-nd/4.0/.
References
- Dieppe PA, Lohmander LS. Pathogenesis and management of pain in osteoarthritis. Lancet 2005;365:965-73. [Crossref] [PubMed]
- Lohmander LS. What can we do about osteoarthritis? Arthritis Res 2000;2:95-100. [Crossref] [PubMed]
- Sandifort E. Museum Anatomicum Academiae Lugduno-Batavae. Leiden: University of Leiden, 1793.
- Chenevier-Gobeaux C, Simonneau C, Lemarechal H, et al. Effect of hypoxia/reoxygenation on the cytokine-induced production of nitric oxide and superoxide anion in cultured osteoarthritic synoviocytes. Osteoarthritis Cartilage 2013;21:874-81. [Crossref] [PubMed]
- Gharbi M, Deberg M, Henrotin Y. Application for proteomic techniques in studying osteoarthritis: a review. Front Physiol 2011;2:90. [Crossref] [PubMed]
- Ramasamy TS, Yee YM, Khan IM. Chondrocyte Aging: The Molecular Determinants and Therapeutic Opportunities. Front Cell Dev Biol 2021;9:625497. [Crossref] [PubMed]
- Garnero P, Delmas PD. Biomarkers in osteoarthritis. Curr Opin Rheumatol 2003;15:641-6. [Crossref] [PubMed]
- Cao J, Yang X, Chen S, et al. The predictive efficacy of tumor mutation burden in immunotherapy across multiple cancer types: A meta-analysis and bioinformatics analysis. Transl Oncol 2022;20:101375. [Crossref] [PubMed]
- Cheng X, Wang X, Nie K, et al. Systematic Pan-Cancer Analysis Identifies TREM2 as an Immunological and Prognostic Biomarker. Front Immunol 2021;12:646523. [Crossref] [PubMed]
- Hu T, Zhao X, Zhao Y, et al. Identification and Verification of Necroptosis-Related Gene Signature and Associated Regulatory Axis in Breast Cancer. Front Genet 2022;13:842218. [Crossref] [PubMed]
- Feng Z, Lian KJ. Identification of genes and pathways associated with osteoarthritis by bioinformatics analyses. Eur Rev Med Pharmacol Sci 2015;19:736-44. [PubMed]
- Poole AR. Biochemical/immunochemical biomarkers of osteoarthritis: utility for prediction of incident or progressive osteoarthritis. Rheum Dis Clin North Am 2003;29:803-18. [Crossref] [PubMed]
- Barter MJ, Bui C, Young DA. Epigenetic mechanisms in cartilage and osteoarthritis: DNA methylation, histone modifications and microRNAs. Osteoarthritis Cartilage 2012;20:339-49. [Crossref] [PubMed]
- Yang Y, Zhang Y, Min D, et al. Screening of key pathogenic genes in advanced knee osteoarthritis based on bioinformatics analysis. Ann Transl Med 2022;10:978. [Crossref] [PubMed]
- Li Z, Zhong L, Du Z, et al. Network Analyses of Differentially Expressed Genes in Osteoarthritis to Identify Hub Genes. Biomed Res Int 2019;2019:8340573. [Crossref] [PubMed]
- Zhu Z, Zhong L, Li R, et al. Study of Osteoarthritis-Related Hub Genes Based on Bioinformatics Analysis. Biomed Res Int 2020;2020:2379280. [Crossref] [PubMed]
- Robinson WH, Lepus CM, Wang Q, et al. Low-grade inflammation as a key mediator of the pathogenesis of osteoarthritis. Nat Rev Rheumatol 2016;12:580-92. [Crossref] [PubMed]
- Dell'Isola A, Allan R, Smith SL, et al. Identification of clinical phenotypes in knee osteoarthritis: a systematic review of the literature. BMC Musculoskelet Disord 2016;17:425. [Crossref] [PubMed]
- Mobasheri A, Saarakkala S, Finnilä M, et al. Recent advances in understanding the phenotypes of osteoarthritis. F1000Res 2019;8:F1000 Faculty Rev-2091.
- Mobasheri A, van Spil WE, Budd E, et al. Molecular taxonomy of osteoarthritis for patient stratification, disease management and drug development: biochemical markers associated with emerging clinical phenotypes and molecular endotypes. Curr Opin Rheumatol 2019;31:80-9. [Crossref] [PubMed]
- Sun F, Zhou JL, Peng PJ, et al. Identification of Disease-Specific Hub Biomarkers and Immune Infiltration in Osteoarthritis and Rheumatoid Arthritis Synovial Tissues by Bioinformatics Analysis. Dis Markers 2021;2021:9911184. [Crossref] [PubMed]
- Edgar R, Domrachev M, Lash AE. Gene Expression Omnibus: NCBI gene expression and hybridization array data repository. Nucleic Acids Res 2002;30:207-10. [Crossref] [PubMed]
- Gautier L, Cope L, Bolstad BM, et al. affy--analysis of Affymetrix GeneChip data at the probe level. Bioinformatics 2004;20:307-15. [Crossref] [PubMed]
- Ritchie ME, Phipson B, Wu D, et al. limma powers differential expression analyses for RNA-sequencing and microarray studies. Nucleic Acids Res 2015;43:e47. [Crossref] [PubMed]
- Bruns TD, Palmer JD, Shumard DS, et al. Mitochondrial DNAs of Suillus: three fold size change in molecules that share a common gene order. Curr Genet 1988;13:49-56. [Crossref] [PubMed]
- Ito K, Murphy D. Application of ggplot2 to Pharmacometric Graphics. CPT Pharmacometrics Syst Pharmacol 2013;2:e79. [Crossref] [PubMed]
- Huang da W. Sherman BT, Lempicki RA. Systematic and integrative analysis of large gene lists using DAVID bioinformatics resources. Nat Protoc 2009;4:44-57. [Crossref] [PubMed]
- Ashburner M, Ball CA, Blake JA, et al. Gene ontology: tool for the unification of biology. The Gene Ontology Consortium. Nat Genet 2000;25:25-9. [Crossref] [PubMed]
- Ogata H, Goto S, Sato K, et al. KEGG: Kyoto Encyclopedia of Genes and Genomes. Nucleic Acids Res 1999;27:29-34.
- Fang IE. By computer Flesch's: reading ease score and a syllable counter. Behav Sci 1968;13:249-51. [Crossref] [PubMed]
- Szklarczyk D, Morris JH, Cook H, et al. The STRING database in 2017: quality-controlled protein-protein association networks, made broadly accessible. Nucleic Acids Res 2017;45:D362-8. [Crossref] [PubMed]
- Muetze T, Goenawan IH, Wiencko HL, et al. Contextual Hub Analysis Tool (CHAT): A Cytoscape app for identifying contextually relevant hubs in biological networks. F1000Res 2016;5:1745. [Crossref] [PubMed]
- Sadewasser DA, Kozloff LM. Identification of bacteriophage T4D gene 29 product, a baseplate hub component, as a folylpolyglutamate synthetase. Biochem Biophys Res Commun 1983;116:1119-24. [Crossref] [PubMed]
- Chin CH, Chen SH, Wu HH, et al. cytoHubba: identifying hub objects and sub-networks from complex interactome. BMC Syst Biol 2014;8:S11. [Crossref] [PubMed]
- You R, Liu S, Tan J. Screening and identification of osteoarthritis related differential genes and construction of a risk prognosis model based on bioinformatics analysis. Ann Transl Med 2022;10:444. [Crossref] [PubMed]
- Tarai M, Kumar K, Divya O, et al. Eigenvalue-eigenvector decomposition (EED) analysis of dissimilarity and covariance matrix obtained from total synchronous fluorescence spectral (TSFS) data sets of herbal preparations: Optimizing the classification approach. Spectrochim Acta A Mol Biomol Spectrosc 2017;184:128-33. [Crossref] [PubMed]
- Kraus VB, Blanco FJ, Englund M, et al. Call for standardized definitions of osteoarthritis and risk stratification for clinical trials and clinical use. Osteoarthritis Cartilage 2015;23:1233-41. [Crossref] [PubMed]
- Clifton KB, Rodner C, Wolf JM. Detection of relaxin receptor in the dorsoradial ligament, synovium, and articular cartilage of the trapeziometacarpal joint. J Orthop Res 2014;32:1061-7. [Crossref] [PubMed]
- Ko JH, Kang YM, Yang JH, et al. Regulation of MMP and TIMP expression in synovial fibroblasts from knee osteoarthritis with flexion contracture using adenovirus-mediated relaxin gene therapy. Knee 2019;26:317-29. [Crossref] [PubMed]
- Fang Y, Wang P, Xia L, et al. Aberrantly hydroxymethylated differentially expressed genes and the associated protein pathways in osteoarthritis. PeerJ 2019;7:e6425. [Crossref] [PubMed]
- Steinberg J, Ritchie GRS, Roumeliotis TI, et al. Integrative epigenomics, transcriptomics and proteomics of patient chondrocytes reveal genes and pathways involved in osteoarthritis. Sci Rep 2017;7:8935. [Crossref] [PubMed]
- Egeblad M, Werb Z. New functions for the matrix metalloproteinases in cancer progression. Nat Rev Cancer 2002;2:161-74. [Crossref] [PubMed]
- Swingler TE, Wheeler G, Carmont V, et al. The expression and function of microRNAs in chondrogenesis and osteoarthritis. Arthritis Rheum 2012;64:1909-19. [Crossref] [PubMed]
- Wang M, Sampson ER, Jin H, et al. MMP13 is a critical target gene during the progression of osteoarthritis. Arthritis Res Ther 2013;15:R5. [Crossref] [PubMed]
- Chijimatsu R, Kunugiza Y, Taniyama Y, et al. Expression and pathological effects of periostin in human osteoarthritis cartilage. BMC Musculoskelet Disord 2015;16:215. [Crossref] [PubMed]
- Norris RA, Damon B, Mironov V, et al. Periostin regulates collagen fibrillogenesis and the biomechanical properties of connective tissues. J Cell Biochem 2007;101:695-711. [Crossref] [PubMed]
- Tajika Y, Moue T, Ishikawa S, et al. Influence of Periostin on Synoviocytes in Knee Osteoarthritis. In Vivo 2017;31:69-77. [Crossref] [PubMed]
- Garnero P. The contribution of collagen crosslinks to bone strength. Bonekey Rep 2012;1:182. [Crossref] [PubMed]
- Maruhashi T, Kii I, Saito M, et al. Interaction between periostin and BMP-1 promotes proteolytic activation of lysyl oxidase. J Biol Chem 2010;285:13294-303. [Crossref] [PubMed]
- Shimazaki M, Nakamura K, Kii I, et al. Periostin is essential for cardiac healing after acute myocardial infarction. J Exp Med 2008;205:295-303. [Crossref] [PubMed]
- Kii I, Ito H. Periostin and its interacting proteins in the construction of extracellular architectures. Cell Mol Life Sci 2017;74:4269-77. [Crossref] [PubMed]
- Brophy RH, Cai L, Duan X, et al. Proteomic analysis of synovial fluid identifies periostin as a biomarker for anterior cruciate ligament injury. Osteoarthritis Cartilage 2019;27:1778-89. [Crossref] [PubMed]
- Nanus DE, Badoume A, Wijesinghe SN, et al. Synovial tissue from sites of joint pain in knee osteoarthritis patients exhibits a differential phenotype with distinct fibroblast subsets. EBioMedicine 2021;72:103618. [Crossref] [PubMed]
(English Language Editor: A. Muijlwijk)