Development and validation of an inflammatory response-related prognostic model and immune infiltration analysis in glioblastoma
Highlight box
Key findings
• In this study, we constructed a prognostic risk model consisting of 4 inflammatory response-related genes (PTPRN, OSMR, MYD88, and EFEMP2) to predict the prognosis of glioblastoma (GBM) patients.
What is known and what is new?
• Recent studies have revealed the important role of inflammation in tumorigenesis and progression. However, the prognostic role of inflammatory response-related genes in GBM remains to be further elucidated.
• In this study, we constructed a prognostic risk model consisting of 4 inflammatory response-related genes to predict the prognosis of GBM patients. The model had a stable predictive power in predicting OS, immune infiltration, potential mechanism, immunotherapy response and drug sensitivity. Interference of model gene OSMR inhibited proliferation and migration and promoted apoptosis of GBM cells.
What is the implication, and what should change now?
• The prognostic model provided a potential direction for the development of personalized prognostic prediction and immunotherapy for GBM patients.
Introduction
Glioblastoma (GBM) remains the most prevalent and aggressive primary brain cancer in adults (1,2). Despite standard treatment, including maximal surgical resection and postoperative radiochemotherapy with temozolomide, there is limited efficacy for GBM patients (1). With standard treatment, the median overall survival (OS) of GBM patients is only 15 months, with less than 5% surviving 5 years after initial diagnosis (3-5). Considering the heterogeneity and different prognosis in each patient, individualized treatment strategy is particularly important. Therefore, it is imperative to identify reliable risk model for the prognostic prediction and personalized treatment plan of GBM.
Inflammation is one of the important features of tumors (6,7). Persistent uncontrollable inflammation plays an important role in tumor initiation, promotion, malignant transformation, invasion, and metastasis (8,9). Recently, mounting evidence has indicated that tumor-related inflammation advances tumor cell survival and proliferation, angiogenesis, and immunosuppression by suppressing effector immune cells and accumulating myeloid suppressor cells (6,10). Increased inflammation and immune infiltration in the tumor microenvironment have been reported to associate with shorter OS in patients with high-grade gliomas (11). Inflammatory response-related models have been reported for prognostic prediction in hepatocellular carcinoma, lung adenocarcinoma, breast cancer and clear cell renal cell carcinoma (12-15). These findings prompted the role of inflammatory response in cancer prognosis with a promising therapeutic target for cancers. However, the impact and prognostic prediction of inflammatory response-related genes in GBM is still unclear. Therefore, we sought to develop a model associated with inflammatory genes to scientifically predict the prognosis of GBM patients.
Many prognostic models have previously been reported in GBM. For example, a study reported a prognostic model constructed by 11 cell senescence-associated genes in GBM (16). In addition, it was also found that the model constructed by m6A-Related lncRNA could predict the prognosis of GBM patients (17). However, the large number of model genes increased the difficulty of clinical translation, and the lack of experimental verification made the results less reliable.
In this study, we downloaded transcriptomic gene expression profiles and corresponding clinical data of GBM patients from public databases. Then, we constructed a 4-gene prognostic model using the differentially expressed inflammatory response-related genes in The Cancer Genome Atlas (TCGA) cohort and validated the reliability of the model using the Chinese Glioma Genome Atlas (CGGA) cohort. A nomogram based on risk score and clinical characteristics was plotted to further accurately predict the prognosis for each patient. Next, we explored the possible mechanisms using functional enrichment analysis. In addition, we analyzed the relationship of prognostic gene expression with immune infiltration type. Furthermore, the correlation between prognostic gene expression and drug sensitivity was investigated. Finally, we performed cell function experiments to investigate the potential role of oncostatin M receptor (OSMR) in GBM. We present the following article in accordance with the TRIPOD reporting checklist (available at https://atm.amegroups.com/article/view/10.21037/atm-22-6271/rc).
Methods
Data collection
Transcriptional gene expression of 174 GBM patients and clinical information data (age, gender, survival state, overall survival) of 599 GBM patients were downloaded from TCGA (https://portal.gdc.cancer.gov/) database (Table 1). In addition, we downloaded gene expression data and clinical information (age, gender, survival state, overall survival, PRS type, radiotherapy, chemotherapy, IDH mutation, 1p19q codeletion, and MGMTp methylation) of 249 GBM samples as an external validation from the CGGA (http://www.cgga.org.cn) database (Table 1). Inflammatory response-related genes were extracted from Gene Set Enrichment Analysis (GSEA; http://www.gsea-msigdb.org/gsea/index.jsp) website. The flowchart of the research design was shown in Figure 1. The study was conducted in accordance with the Declaration of Helsinki (as revised in 2013).
Table 1
Characteristics | TCGA | CGGA |
---|---|---|
Total | 599 | 249 |
Age | ||
<65 years | 393 | 216 |
≥65 years | 203 | 33 |
Not reported | 3 | 0 |
Gender | ||
Female | 230 | 102 |
Male | 366 | 147 |
Not reported | 3 | 0 |
Survival status | ||
Alive | 144 | 49 |
Dead | 455 | 166 |
Not reported | 0 | 34 |
PRS | ||
Primary | NA | 140 |
Recurrent | NA | 109 |
Secondary | NA | 0 |
Not reported | NA | 0 |
IDH mutation status | ||
Wildtype | NA | 190 |
Mutant | NA | 49 |
Not reported | NA | 10 |
1p19q codeletion status | ||
Codel | NA | 13 |
Non-codel | NA | 205 |
Not reported | NA | 31 |
MGMTp methylation status | ||
Methylated | NA | 106 |
Un-methylated | NA | 93 |
Not reported | NA | 50 |
GBM, glioblastoma; TCGA, The Cancer Genome Atlas; CGGA, Chinese Glioma Genome Atlas; PRS, primary-recurrent-secondary; NA, not applicable; IDH, isocitrate dehydrogenase.
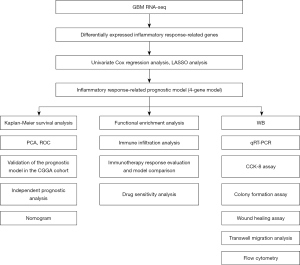
Construction and validation of an inflammatory genes-related prognostic model
The “Bioconductor Limma” R package was used to analyze differentially expressed inflammatory response-related genes in TCGA cohort. Genes with fold change >0.5 and false discovery rate (FDR) <0.05 were considered differentially expressed inflammatory genes. Univariate Cox regression analysis was used to further screen for prognosis-related inflammatory genes (P<0.05). Then, these prognosis-related inflammatory genes were applied to conduct the least absolute shrinkage and selection operator (LASSO) regression analysis. The LASSO algorithm was used to avoid overfitting the prognostic model and screen out the optimal prognostic genes for constructing the prognostic model (18). The risk score was calculated as the sum of the expression of each gene, multiplied by its corresponding regression coefficient. Taking the median risk score as a cutoff, GBM patients were divided into high-risk group and low-risk group according to the risk value. Principal component analysis (PCA) was performed with the “ggplot2” R package to explore the distribution of genes in different groups. OS was analyzed in the high-risk and low-risk groups using the “survival” and “survminer” R packages. The time-dependent receiver operating characteristic (ROC) curve analysis was performed using the “survival”, “survminer”, and “timeROC” R packages. Univariate Cox regression analysis was used to estimate the predictive value of the risk score and clinical characteristics such as age, gender, PRS type, radiotherapy, chemotherapy, IDH mutation, 1p19q codeletion, and MGMTp methylation. A nomogram was plotted to further accurately predict the prognosis for each patient through “regplot” and “rms” R packages.
Functional enrichment analysis
To illuminate the relationship between the risk scores and biological functions, we used GSEA and the GSEA 4.1.0 software to perform Kyoto Encyclopedia of Genes and Genomes (KEGG) pathway enrichment analysis. The pathway was considered statistically significant when P<0.05.
Analysis of tumor immune microenvironment and immune infiltration
Single sample gene set enrichment analysis (ssGSEA) of the “GSVA” R package was applied to calculate the immune cell infiltration score and immune-related function score. Spearman’s correlation was used to analyze the association of risk score with immune cell score, stromal cell score, Estimation of STromal and Immune cells in MAlignant Tumor tissues using Expression data (ESTIMATE) score, and tumor purity.
Immunotherapy efficacy assessment and model comparison
To investigate whether the above inflammatory response-related prognostic model can be used to assess the efficacy of immunotherapy in patients, we analyzed immunotherapy biomarkers based on TCGA database. TCGA sample expression profiles were uploaded to the tumor immune dysfunction and exclusion (TIDE) website (http://tide.dfci.harvard.edu/) for TIDE, microsatellite instability (MSI), and dysfunction scores of each sample. Furthermore, the ROC curves were used to compare the prognostic predictive efficacy of the risk values with TIDE and tumor inflammation signature (TIS) scores (19).
Drug sensitivity analysis
Transcriptomic data and Food and Drug Administration (FDA)-approved drug sensitivity-related data were downloaded from the CellMiner website (https://discover.nci.nih.gov/cellminer/). Pearson’s correlation analysis was used to investigate the relationship between drug sensitivity and prognostic gene expression.
Cell culture and siRNA processing
Human brain vascular pericytes (HBVP), U251 and U118 cells were obtained from the American Type Culture Collection (ATCC; Manassas, VA, USA) and cultured in Dulbecco’s modified Eagle medium (DMEM) medium containing 10% fetal bovine serum (FBS; Gibco, Waltham, MA, USA) in a 37 ℃, 5% CO2 incubator. OSMR small interfering RNA (siRNA) was obtained from OBIO (Shanghai, China) and transfected into cells using polybrene (OBIO, China) according to the manufacturer's instructions.
Western blot (WB) analysis
Proteins from U251 and U118 cells were extracted using radioimmunoprecipitation assay (RIPA) lysis buffer (Servicebio, Wuhan, China). Protein samples were then separated by 10% sodium dodecyl sulfate-polyacrylamide gel electrophoresis (SDS-PAGE) and transferred to polyvinylidene fluoride (PVDF) membranes. After being blocked with 5% skim milk for 1 hour at room temperature, the membranes were incubated with the following primary antibodies: OSMR (Abclonal, Wuhan, China), GAPDH (Proteintech, Rosemont, IL, USA) overnight at 4 ℃, followed by the secondary antibody at 25 ℃ for 1 hour. Finally, the blots were detected and imaged using the ECL Plus Western Blotting Detection System (GE Healthcare, Amersham, UK).
Quantitative real time polymerase chain reaction (qRT-PCR)
Total cellular RNA was extracted with TRIzol reagent (TaKaRa, Shiga, Japan). Then HiScript II QRT SuperMix (Vazyme, Nanjing, China) was used to reverse transcribe total RNA to complementary DNA (cDNA) and qRT-PCR was performed with ChamQ Universal SYBR qPCR Master Mix (Vazyme, China). The primers were listed as following: OSMR-forward: AATGTCAGTGAAGGCATGAAAGG; OSMR-reverse: GAAGGTTGTTTAGACCACCCC; GAPDH-forward: GACCACAGTCCATGCCATCA; GAPDH-reverse: GTCAAAGGTGGAGGAGTGGG.
Cell proliferation assay
After transfection with OSMR siRNA for 48 hours, U251 and U118 cells were cultured in 96-well plates. Cell Counting Kit-8 (CCK-8) reagent [MedChemExpress (MCE), Monmouth Junction, NJ, USA] was added to each well according to the manufacturer’s instructions, and the proliferation capacity was detected at 1, 2, 3, and 4 days after culturing the cells using a microplate reader (BioTek, Winooski, VT, USA) to detect OD450 values.
Colony formation assay
After transfection with OSMR siRNA for 48 hours, U251 cells were cultured in 6-well plate and incubated at 37 ℃ for 10 days. Then, the cell clones were washed with PBS and fixed in 4% paraformaldehyde for 20 minutes and dyed with 0.1% crystal violet for 15 minutes. Finally, cell colonies were counted.
Wound healing assay
Cells were cultured in 6-well plates with serum-free DMEM and then scraped with a 200 µL pipette tip. Images of cell migration were taken at 0, 12, and 24 hours after injury.
Transwell migration assay
Cells were seeded in the upper transwell chamber with 200 µL of serum-free medium, and 600 µL of medium containing 20% FBS was placed in the lower chamber. After 24 hours of incubation, cells in the lower chamber were fixed with methanol for 20 minutes, washed 3 times with phosphate-buffered saline (PBS), and then stained with 1% crystal violet for 15 minutes, and counted under a light microscope in 3 random areas.
Apoptosis and cell cycle assay
For the cell apoptosis assay, cells were collected and washed 3 times with PBS. Then, cells were resuspended in binding buffer and stained with propidium iodide (PI) and Annexin V-FITC [Becton, Dickinson, and Co. (BD) Biosciences, Franklin Lakes, NJ, USA] for 15 minutes in the dark. Apoptosis was detected and analyzed by flow cytometry and FlowJo 10.6.2 (BD Biosciences, USA). For cell cycle assay, cells were collected and fixed in 70% ethanol overnight at 4 ℃ and stained with a cell cycle staining kit (Multi Sciences, Hangzhou, China). Flow cytometry and Modfit LT software (Verity Software House, Topsham, ME, USA) were used to detect and analyze the cell cycle.
Statistical analysis
The gene differences between tumor tissue and normal tissue were analyzed using Wilcoxon test. Kaplan-Meier analysis was utilized to compare OS between the high-risk and low-risk groups. R software (version 4.0.4; The R Foundation for Statistical Computing, Vienna, Austria) was used to conduct the statistical analyses. The difference analysis of the experimental data were conducted using unpaired t-test for two groups or one-way ANOVA test for multiple groups. P<0.05 indicated statistical significance.
Results
Identification of differentially expressed prognosis-related inflammation genes in TCGA cohort
We first downloaded inflammatory response-related genes from the GSEA website and transcriptional gene expression data from TCGA database, and found that 6,850 inflammatory response-related genes were differentially expressed between GBM and normal tissues, including 2,461 down-regulated and 4,389 up-regulated genes (Figure 2, available online: https://cdn.amegroups.cn/static/public/atm-22-6271-1.xlsx). We then performed univariate Cox analysis using the clinical information and gene expression data of GBM samples in TCGA database. A total of 802 inflammatory genes associated with prognosis were obtained, including 157 low-risk genes and 645 high-risk genes (Figure S1).
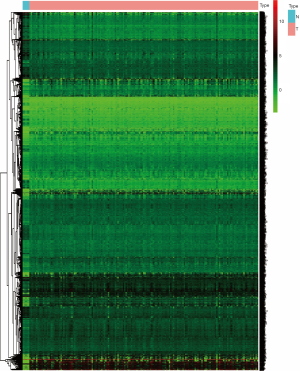
Construction of an inflammatory response genes-related prognostic model in TCGA cohort
Next, we analyzed the 802 differentially expressed prognosis-related inflammatory genes and identified 4 genes (PTPRN, OSMR, MYD88, EFEMP2) to construct a prognostic risk model through LASSO Cox regression analysis (Figure 3A,3B). The risk score was calculated as the following formula: Risk score = 0.155045 × PTPRN + 0.002887 × OSMR + 0.037493 × MYD88 + 0.093558 × EFEMP2 (Table 2). Based on the median cutoff value, GBM patients were divided into high-risk and low-risk groups (Figure 3C). In TCGA cohort, PCA analysis showed that patients in the 2 subgroups were distributed in 2 directions, indicating that the model could effectively distinguish the high-risk and low-risk groups (Figure 3D). In addition, survival status scatter plots indicated that high-risk patients had worse outcomes (Figure 3E). Consistently, Kaplan-Meier curves showed that high-risk patients had significantly shorter OS than low-risk patients (P<0.0001) (Figure 3F). The time-dependent ROC curves showed an area under the curve (AUC) of 0.782, 0.765, and 0.784 for 1-, 2-, and 3-year survival, respectively (Figure 3G).
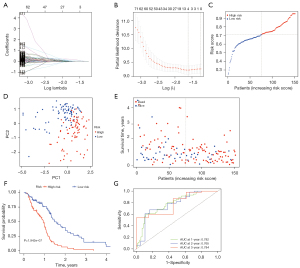
Table 2
Gene | Coef | HR | HR.95L | HR.95H | P value |
---|---|---|---|---|---|
PTPRN | 0.155044751136123 | 1.7523 | 1.336219 | 2.297944 | 5.00E-05 |
OSMR | 0.00288662972796754 | 1.433946 | 1.192988 | 1.723572 | 0.000123 |
MYD88 | 0.0374931050514576 | 1.797359 | 1.291102 | 2.502125 | 0.000513 |
EFEMP2 | 0.0935581536160227 | 1.476297 | 1.188053 | 1.834475 | 0.00044 |
LASSO, least absolute shrinkage and selection operator; HR, hazard ratio.
Validation of the prognostic model in the CGGA cohort
To test the stability of the 4-gene prognostic model, we downloaded the gene expression data and clinical information from CGGA as external validation. Patients in the CGGA cohort were also classified into high- or low-risk groups according to the median cut-off value of TCGA cohort (Figure 4A). Similar to the results of TCGA cohort, PCA analysis showed a discrete distribution of patients in the different risk groups (Figure 4B). Similarly, patients in the high-risk group were more likely to have a less favorable outcome (Figure 4C) and a shorter OS (P<0.001) (Figure 4D) compared to those in the low-risk group. In addition, the AUC of the prognostic model was 0.589 at 1 year, 0.684 at 2 years, and 0.785 at 3 years (Figure 4E). Univariate Cox regression analysis showed the risk score (P<0.01), PRS type (P<0.001), and IDH mutation (P<0.05) could predict the prognosis of GBM patients well (Figure 4F). A nomogram was plotted to further accurately predict the prognosis for each patient according to the total points of the risk and clinical characteristics such as radiotherapy, MGMTp methylation, chemotherapy, age, IDH mutation, 1p19q codeletion, gender, and PRS type. For example, the total points of the patient in the nomogram were 407, corresponding to 47.7%, 9.58%, and 1.13% for 1-, 2-, and 3-year OS, respectively (Figure 4G).
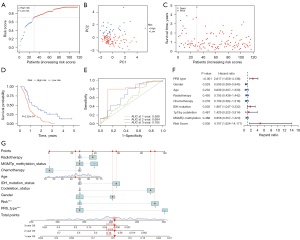
Pathway enrichment analysis
To further explore the potential mechanism of the inflammatory genes affecting the prognosis of GBM patients, we performed KEGG pathway enrichment analysis in high- and low-risk groups. We found that 31 KEGG pathways such as glycan biosynthesis, adhesion molecules cams, hematopoietic cell lineage, cytokine-cytokine receptor interaction, and leukocyte transendothelial migration were significantly enriched in the high-risk group, whereas 39 pathways such as cell cycle, basal transcription factors, RNA degradation, spliceosome, and RNA polymerase were enriched in the low-risk group (Figure 5A). Additionally, tumor-related signaling pathways such as the JAK-STAT pathway was significantly enriched in the high-risk group (Figure 5B), whereas p53 and transforming growth factor beta (TGF-β) signaling pathways were enriched in the low-risk group (Figure 5C,5D). Unexpectedly, we found that 5 immune-related pathways were enriched in the high-risk group, including natural killer (NK) cell-mediated cytotoxicity, chemokine signaling pathway, Fc gamma R-mediated phagocytosis, antigen processing and presentation, and intestinal immune network for IgA production, but failed to find statistically significant pathways in the low-risk group (Figure 5E-5I).
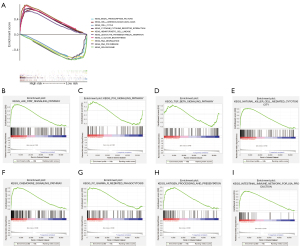
Correlation analysis of immune subtypes and immune response
Since we discovered that immune-related pathways were enriched in the high-risk group in the KEGG pathway enrichment analysis, we further questioned the relationship between risk score and immune infiltration. Thus, we used ssGSEA to quantify immune cell subpopulations, immune-related functions, and pathways. We found that the infiltration of activated dendritic cell (aDCs), B cells, DCs, plasmacytoid DCs (pDCs), macrophages, neutrophils, T-helper cells, Tfh, Th1 cells, Th2 cells, tumor infiltrating lymphocytes (TIL), and Treg were significantly higher in the high-risk group compared with the low-risk group (P<0.05) (Figure 6A). In addition, the results of immune function and pathway analysis showed that the scores of antigen-presenting cells (APC) co-inhibition, APC co-stimulation, chemokine receptors (CCR), check-point, cytolytic activity, human leukocyte antigen (HLA), inflammation-promoting, parainflammation, T-cell co-inhibition, T-cell co-stimulation, and type II interferon (IFN) response were significantly higher in the high-risk group than in the low-risk group (P<0.05) (Figure 6B). Next, we explored the impact of tumor microenvironment on the prognosis of GBM patients and found that risk score was positively correlated with stromal score (P<0.0001), immune score (P<0.0001), and ESTIMATE score (P<0.0001), yet was negatively correlated with tumor purity (P<0.0001) (Figure 6C-6F), suggesting that the higher the stromal and immune cell content and lower tumor purity in GBM patients, the worse the prognosis of patients.
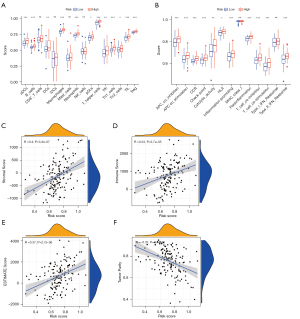
Immunotherapy efficacy assessment and model comparison
To further investigate whether our inflammatory response-related prognostic model can be used to assess the efficacy of immunotherapy in patients, we analyzed immunotherapy biomarkers based on TCGA database. The results demonstrated that the high-risk group had a higher TIDE score (P<0.001) (Figure 7A), a lower MSI score (P<0.05) (Figure 7B), and a higher dysfunction score (P<0.001) (Figure 7C), indicating that the GBM high-risk group had greater immune escape potential and poorer immunotherapy efficacy. In addition, as seen from the ROC curve, our inflammatory response-related prognostic model had the largest AUC value, indicating a higher prognostic predictive efficacy than that of TIDE and TIS scores (Figure 7D).
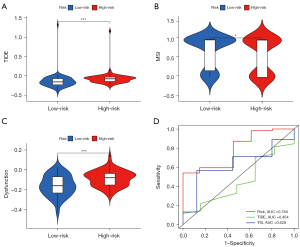
Drug sensitivity analysis
To enhance the clinical value of our model, we further investigated the availability of the prognostic model for clinical drug selection. We found that OSMR expression was positively correlated with the sensitivity of simvastatin (P<0.001) and erlotinib (P<0.01) (Figure 8A,8B) and negatively correlated with the sensitivity of tamoxifen (P<0.001), nilotinib (P<0.001), crizotinib (P<0.001), palbociclib (P<0.001), LDK−378 (P<0.001), oxaliplatin (P<0.001), cyclophosphamide (P<0.01), and raloxifene (P<0.01) (Figure 8C-8J). In addition, MYD88 expression was positively correlated with nelarabine sensitivity (P<0.001) (Figure 8K), whereas PTPRN expression was negatively correlated with nilotinib sensitivity (P<0.001) (Figure 8L). In addition, higher expression of EFEMP2 was associated with increased resistance to homoharringtonine (P<0.001), actinomycin D (P<0.01), vinblastine (P<0.01), and TYROTHRICIN (P<0.01) (Figure 8M-8P).
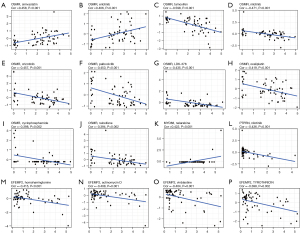
Interference of OSMR expression inhibits GBM cell proliferation and migration
To further increase the credibility of the prognostic model in GBM, we conducted an in vitro experiment. Since the expression of OSMR had the most significant effect on the prognosis of GBM patients among the 4 model genes (Figure S2A-S2D), we selected OSMR for subsequent functional validation. We examined the messenger RNA (mRNA) expression of OSMR in HBVP and different GBM cell lines, and found that OSMR expression was highest in U251 and U118 cells (P<0.0001) (Figure 9A), so we used U251 and U118 cells for the follow-up experiments. OSMR expression in U251 and U118 cells was successfully interfered with siRNA and confirmed by WB and qRT-PCR (P<0.05) (Figure 9B,9C). CCK-8 assays showed that OSMR interference significantly inhibited the proliferation of U251 and U118 cells at day 3 (P<0.0001, P<0.01) and day 4 (P<0.0001, P<0.001) (Figure 9D). Colony formation assay showed that OSMR interference significantly inhibited the colony formation of U251 cells (P<0.01) (Figure S2E,S2F). Subsequently, wound healing and transwell migration assays were applied to explore the effect of OSMR interference on the migratory ability of GBM cells, and the results showed that interference of OSMR significantly inhibited the migration of U251 and U118 cells (P<0.05) (Figure 9E-9H). In addition, the flow cytometry result showed that interference of OSMR greatly enhanced apoptosis of U251 cells (P<0.001) and U118 cells (P<0.01) (Figure 9I,9J). In the cell cycle assay, we found an increase in G1 phase cells and a significant decrease in G2/M phase in U251 cells after OSMR interference (P<0.05), suggesting that interference of OSMR resulted in G1 phase block in U251 cells (Figure S2G,S2H). In conclusion, these results suggested that interference of OSMR expression inhibited proliferation, migration and promoted apoptosis of GBM cells, further confirming the credibility of model in predicting GBM prognosis.
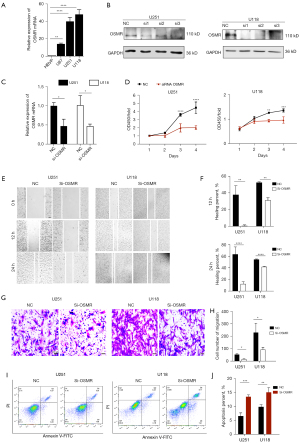
Discussion
GBM is a highly malignant tumor with a high mortality rate (20,21). Despite the application of standard treatment, namely surgical resection, postoperative radiotherapy, and temozolomide chemotherapy, the OS of patients remains unsatisfactory (22,23). Therefore, there is an urgent need to explore effective diagnostic markers and develop molecular targeted therapies. The rapid development of high-throughput sequencing technologies enables researchers to explore the mechanism of GBM progression by analyzing large-scale gene expression data and clinical information of GBM patients (24).
Recent studies have shown that inflammation accelerates GBM progression and causes resistance to treatment. A network analysis showed that survival of GBM patients was associated with inflammatory response (25). Another study revealed that TP53 gain-of-function (GOF) mutations promoted inflammation in GBM, thereby worsening the outcome of GBM patients (26). In addition, studies also reported that increased serum levels of neutrophils and inflammatory proteins were associated with poor prognosis in GBM patients (27,28). Thus, the inflammatory microenvironment is closely related to the progression and prognosis of GBM patients.
However, the prognostic value of inflammation-associated genes in GBM remains to be explored. Therefore, in this study, we systematically analyzed the expression of inflammatory response-related genes in GBM tissues and their association with OS. A prognostic model integrating 4 inflammatory response-related genes (PTPRN, OSMR, MYD88, and EFEMP2) was then constructed through TCGA database and validated in the CGGA cohort. The GBM patients were divided into high- and low-risk groups based on their median risk score. High-risk patients were more likely to have shorter OS. PTPRN, a type I transmembrane protein, plays a key role in the cell functions-related signaling cascades, which may cause cancer, autoimmune diseases, and metabolic syndrome (29-31). Overexpression of PTPRN is significantly associated with poor OS in GBM patients (32). In addition, a recent study showed that PTPRN overexpression induced proliferation and migration of glioma cells, whereas PTPRN knockdown inhibited tumor growth in mouse model of glioma (33). Similarly, by analyzing the relationship between inflammatory response and GBM prognosis, we found that PTPRN might promote GBM progression by affecting the inflammatory response and tumor microenvironment. OSMR is a cytokine receptor gene of the interleukin-6 (IL-6) family that plays a key role in driving glioma cells to the mesenchymal phenotype (34). OSMR expression is significantly higher in GBM than low-grade glioma, and OSMR can be used as a biomarker to predict the response of GBM patients to standard radiotherapy and chemotherapy (35). In our study, we observed that interference of OSMR expression inhibited the proliferation and migratory capacity of GBM cells in vitro, further confirming the important role of OSMR in predicting GBM prognosis. MYD88 is a key adaptor protein in the IL-1 receptor and toll-like receptor signaling pathways. MYD88 expression is significantly associated with OS and grade in glioma patients (36). This is consistent with the findings of our study that MYD88 was significantly upregulated in GBM tissues and correlated with poor OS in GBM patients. EFEMP2, a member of the fibronectin family, has been reported to promote tumor proliferation in lung cancer, colon cancer, and glioma (37,38). In our study, we demonstrated that upregulation of EFEMP2 was associated with shorter OS in GBM. Furthermore, Huang et al. found that EFEMP2 indicated assembly of M0 macrophages in the tumor microenvironment and a more malignant phenotype of glioma (39).
With a better understanding of the association between tumor microenvironment and tumor, the role of inflammation in the tumor microenvironment cannot be ignored. To gain more insight into the correlation between risk scores and immune components, we investigated immune infiltrating cells in different groups. The results showed that immune infiltrating cells were enriched in the high-risk group, such as aDCs, B cells, DCs, pDCs, macrophages, neutrophils, T-helper cells, Tfh, Th1 cells, Th2 cells, TIL, and Treg, indicating that immune regulation was disturbed in the high-risk group, leading to more aggressive tumors and poorer prognosis in GBM patients. In addition, the results of correlation analysis suggested that the higher the stromal and immune cell content and lower tumor purity in GBM patients, the worse the prognosis of patients, which is consistent with the previous study on aggressive gliomas (40).
Although immunotherapy is a promising strategy, GBM has a wide range of immunosuppressive mechanisms, and the GBM microenvironment plays an important role in tumor immune escape, which leads to immunosuppression and affects immunotherapy efficacy (41-44). Predicting the efficacy of immunotherapy is critical to determine the prognosis of GBM patients. By analyzing immunotherapy-related markers, we found that our inflammatory response-related prognostic model can successfully predict the efficacy of immunotherapy in GBM, and the high-risk group has greater immune escape potential and is less likely to benefit from immunotherapy, which can provide clinical guidance on the choice of immunotherapy.
Next, we attempted to further analyze the potential mechanism of inflammatory response-related genes in GBM. GSEA revealed that tumor-related signaling pathways such as the JAK-STAT pathway were significantly enriched in the high-risk group, whereas p53 and TGF-β signaling pathways were enriched in the low-risk group. Studies have demonstrated that inhibition of phosphorylation of JAK or STAT is associated with a decrease in anti-apoptotic proteins, leading to apoptosis in GBM cells (45-47). P53 is a tumor suppressor protein that activates cell cycle arrest or induces apoptosis, preventing further division and growth of damaged cells (48,49). Dysregulated p53 pathway was found in 84% of GBM patients and 94% of GBM cell lines (50,51), which is related to the proliferation, migration, invasion, and evasion of apoptosis of GBM cells (52). The TGF-β signaling pathway plays a very important role in tumorigenesis and progression (53,54). On the one hand, TGF-β inhibits tumorigenesis and early development by suppressing cell cycle progression, inducing apoptosis, and inhibiting the expression of growth factors, cytokines and chemokines, and on the other hand, TGF-β is considered pro-oncogenic by affecting multiple components of the immune system and regulating the tumor microenvironment (55). Therefore, according to the above studies and our results, inflammatory response-related genes may affect the prognosis of GBM patients through the JAK-STAT, P53 and TGF-β signaling pathways. In addition, immune-related signaling pathways, such as NK cell-mediated cytotoxicity, chemokine signaling pathway, Fc gamma R-mediated phagocytosis, antigen processing and presentation, and intestinal immune network for IgA production were significantly enriched in the high-risk group, further validating the close relationship between inflammatory response and tumor progression.
Furthermore, by analyzing the NCI-60 cell lines data, we found that the expression of the prognostic genes was associated with the sensitivity to FDA-approved drugs in our study. For example, OSMR expression was positively correlated with resistance to tamoxifen, nilotinib, crizotinib, palbociclib, LDK-378, oxaliplatin, cyclophosphamide, and raloxifene. Tamoxifen has been reported to have potent antitumor activity in various types of tumors, including glioma (56,57). Thus, patients with high expression of OSMR may be predictive of resistance to tamoxifen treatment. In addition, increased expression of MYD88 was associated with increased sensitivity to nelarabine. Therefore, these data may provide a new perspective for the future precise treatment of GBM patients.
Conclusions
In conclusion, our study constructed a novel prognostic model consisting of 4 inflammatory response-related genes. The prognostic model we constructed was shown to have stable predictive power in predicting OS, immune infiltration, potential mechanism, immunotherapy response, and drug sensitivity, providing a potential direction for the development of personalized therapy for GBM patients.
Acknowledgments
The authors express their appreciation to TCGA and CGGA databases for the availability of the data. Thanks to the technical support by the Huazhong University of Science & Technology Analytical & Testing Center.
Funding: This study was funded by the National Natural Sciences Foundation of China (Grant Nos. 82003312, 82173311).
Footnote
Reporting Checklist: The authors have completed the TRIPOD reporting checklist. Available at https://atm.amegroups.com/article/view/10.21037/atm-22-6271/rc
Data Sharing Statement: Available at https://atm.amegroups.com/article/view/10.21037/atm-22-6271/dss
Conflicts of Interest: All authors have completed the ICMJE uniform disclosure form (available at https://atm.amegroups.com/article/view/10.21037/atm-22-6271/coif). All authors report that this study was funded by the National Natural Sciences Foundation of China (Grant Nos. 82003312, 82173311). The authors have no other conflicts of interest to declare.
Ethical Statement: The authors are accountable for all aspects of the work in ensuring that questions related to the accuracy or integrity of any part of the work are appropriately investigated and resolved. The study was conducted in accordance with the Declaration of Helsinki (as revised in 2013).
Open Access Statement: This is an Open Access article distributed in accordance with the Creative Commons Attribution-NonCommercial-NoDerivs 4.0 International License (CC BY-NC-ND 4.0), which permits the non-commercial replication and distribution of the article with the strict proviso that no changes or edits are made and the original work is properly cited (including links to both the formal publication through the relevant DOI and the license). See: https://creativecommons.org/licenses/by-nc-nd/4.0/.
References
- Wen PY, Kesari S. Malignant gliomas in adults. N Engl J Med 2008;359:492-507. [Crossref] [PubMed]
- Thakkar JP, Dolecek TA, Horbinski C, et al. Epidemiologic and molecular prognostic review of glioblastoma. Cancer Epidemiol Biomarkers Prev 2014;23:1985-96. [Crossref] [PubMed]
- Wick W, Osswald M, Wick A, et al. Treatment of glioblastoma in adults. Ther Adv Neurol Disord 2018;11:1756286418790452. [Crossref] [PubMed]
- Ostrom QT, Bauchet L, Davis FG, et al. The epidemiology of glioma in adults: a "state of the science" review. Neuro Oncol 2014;16:896-913. [Crossref] [PubMed]
- Stupp R, Hegi ME, Mason WP, et al. Effects of radiotherapy with concomitant and adjuvant temozolomide versus radiotherapy alone on survival in glioblastoma in a randomised phase III study: 5-year analysis of the EORTC-NCIC trial. Lancet Oncol 2009;10:459-66. [Crossref] [PubMed]
- Mantovani A, Allavena P, Sica A, et al. Cancer-related inflammation. Nature 2008;454:436-44. [Crossref] [PubMed]
- Balkwill F, Mantovani A. Inflammation and cancer: back to Virchow? Lancet 2001;357:539-45. [Crossref] [PubMed]
- Singh R, Mishra MK, Aggarwal H. Inflammation, Immunity, and Cancer. Mediators Inflamm 2017;2017:6027305. [Crossref] [PubMed]
- Galdiero MR, Marone G, Mantovani A. Cancer Inflammation and Cytokines. Cold Spring Harb Perspect Biol 2018;10:a028662. [Crossref] [PubMed]
- Grivennikov SI, Greten FR, Karin M. Immunity, inflammation, and cancer. Cell 2010;140:883-99. [Crossref] [PubMed]
- Marinari E, Allard M, Gustave R, et al. Inflammation and lymphocyte infiltration are associated with shorter survival in patients with high-grade glioma. Oncoimmunology 2020;9:1779990. [Crossref] [PubMed]
- Lu G, Du R, Feng B, et al. A Novel Gene Signature Associated with Inflammatory Responses and Immune Status Assists in Prognosis and Intervention for Patients with HCC. J Inflamm Res 2022;15:6729-43. [Crossref] [PubMed]
- Shi Y, Zhao Y, Wang Y. An Inflammatory Response-Related Gene Signature Can Predict the Prognosis and Impact the Immune Status of Lung Adenocarcinoma. Cancers (Basel) 2022;14:5744. [Crossref] [PubMed]
- Zhao R, Xie C, Gong Y, et al. A Novel Inflammatory Response-Related Gene Signature Predicts Immune Status and Prognosis of Breast Cancer. J Oncol 2022;2022:5468858. [Crossref] [PubMed]
- Zhang Y, Shi C, Chen Y, et al. Systematic Analysis of Immune Infiltration and Predicting Prognosis in Clear Cell Renal Cell Carcinoma Based on the Inflammation Signature. Genes (Basel) 2022;13:1897. [Crossref] [PubMed]
- Tan C, Wei Y, Ding X, et al. Cell senescence-associated genes predict the malignant characteristics of glioblastoma. Cancer Cell Int 2022;22:411. [Crossref] [PubMed]
- Xie P, Yan H, Gao Y, et al. Construction of m6A-Related lncRNA Prognostic Signature Model and Immunomodulatory Effect in Glioblastoma Multiforme. Front Oncol 2022;12:920926. [Crossref] [PubMed]
- Jiang J, Liu D, Xu G, et al. TRIM68, PIKFYVE, and DYNLL2: The Possible Novel Autophagy- and Immunity-Associated Gene Biomarkers for Osteosarcoma Prognosis. Front Oncol 2021;11:643104. [Crossref] [PubMed]
- Danaher P, Warren S, Lu R, et al. Pan-cancer adaptive immune resistance as defined by the Tumor Inflammation Signature (TIS): results from The Cancer Genome Atlas (TCGA). J Immunother Cancer 2018;6:63. [Crossref] [PubMed]
- Nguyen HM, Guz-Montgomery K, Lowe DB, et al. Pathogenetic Features and Current Management of Glioblastoma. Cancers (Basel) 2021;13:856. [Crossref] [PubMed]
- Janjua TI, Rewatkar P, Ahmed-Cox A, et al. Frontiers in the treatment of glioblastoma: Past, present and emerging. Adv Drug Deliv Rev 2021;171:108-38. [Crossref] [PubMed]
- Ostrom QT, Gittleman H, Liao P, et al. CBTRUS statistical report: primary brain and central nervous system tumors diagnosed in the United States in 2007-2011. Neuro Oncol 2014;16:iv1-63. [Crossref] [PubMed]
- Touat M, Idbaih A, Sanson M, et al. Glioblastoma targeted therapy: updated approaches from recent biological insights. Ann Oncol 2017;28:1457-72. [Crossref] [PubMed]
- Di W, Fan W, Wu F, et al. Clinical characterization and immunosuppressive regulation of CD161 (KLRB1) in glioma through 916 samples. Cancer Sci 2022;113:756-69. [Crossref] [PubMed]
- Arimappamagan A, Somasundaram K, Thennarasu K, et al. A fourteen gene GBM prognostic signature identifies association of immune response pathway and mesenchymal subtype with high risk group. PLoS One 2013;8:e62042. [Crossref] [PubMed]
- Ham SW, Jeon HY, Jin X, et al. TP53 gain-of-function mutation promotes inflammation in glioblastoma. Cell Death Differ 2019;26:409-25. [Crossref] [PubMed]
- Mostafa H, Pala A, Högel J, et al. Immune phenotypes predict survival in patients with glioblastoma multiforme. J Hematol Oncol 2016;9:77. [Crossref] [PubMed]
- Wang PF, Song HW, Cai HQ, et al. Preoperative inflammation markers and IDH mutation status predict glioblastoma patient survival. Oncotarget 2017;8:50117-23. [Crossref] [PubMed]
- Pulido R, Stoker AW, Hendriks WJ. PTPs emerge as PIPs: protein tyrosine phosphatases with lipid-phosphatase activities in human disease. Hum Mol Genet 2013;22:R66-76. [Crossref] [PubMed]
- Jiang ZX, Zhang ZY. Targeting PTPs with small molecule inhibitors in cancer treatment. Cancer Metastasis Rev 2008;27:263-72. [Crossref] [PubMed]
- Solouki S, August A, Huang W. Non-receptor tyrosine kinase signaling in autoimmunity and therapeutic implications. Pharmacol Ther 2019;201:39-50. [Crossref] [PubMed]
- Shergalis A, Bankhead A 3rd, Luesakul U, et al. Current Challenges and Opportunities in Treating Glioblastoma. Pharmacol Rev 2018;70:412-45. [Crossref] [PubMed]
- Wang D, Tang F, Liu X, et al. Expression and Tumor-Promoting Effect of Tyrosine Phosphatase Receptor Type N (PTPRN) in Human Glioma. Front Oncol 2021;11:676287. [Crossref] [PubMed]
- Heinrich PC, Behrmann I, Müller-Newen G, et al. Interleukin-6-type cytokine signalling through the gp130/Jak/STAT pathway. Biochem J 1998;334:297-314. [Crossref] [PubMed]
- Guo Q, Guan GF, Cao JY, et al. Overexpression of oncostatin M receptor regulates local immune response in glioblastoma. J Cell Physiol 2019; Epub ahead of print. [Crossref] [PubMed]
- Guo Q, Xiao X, Zhang J. MYD88 Is a Potential Prognostic Gene and Immune Signature of Tumor Microenvironment for Gliomas. Front Oncol 2021;11:654388. [Crossref] [PubMed]
- Gallagher WM, Greene LM, Ryan MP, et al. Human fibulin-4: analysis of its biosynthetic processing and mRNA expression in normal and tumour tissues. FEBS Lett 2001;489:59-66. [Crossref] [PubMed]
- Wang L, Chen Q, Chen Z, et al. EFEMP2 is upregulated in gliomas and promotes glioma cell proliferation and invasion. Int J Clin Exp Pathol 2015;8:10385-93. [PubMed]
- Huang L, Wang Z, Chang Y, et al. indicates assembly of M0 macrophage and more malignant phenotypes of glioma. Aging 2020;12:8397-412. [Crossref] [PubMed]
- Zhang C, Cheng W, Ren X, et al. Tumor Purity as an Underlying Key Factor in Glioma. Clin Cancer Res 2017;23:6279-91. [Crossref] [PubMed]
- Yang Y. Cancer immunotherapy: harnessing the immune system to battle cancer. J Clin Invest 2015;125:3335-7. [Crossref] [PubMed]
- Tomaszewski W, Sanchez-Perez L, Gajewski TF, et al. Brain Tumor Microenvironment and Host State: Implications for Immunotherapy. Clin Cancer Res 2019;25:4202-10. [Crossref] [PubMed]
- Yu MW, Quail DF. Immunotherapy for Glioblastoma: Current Progress and Challenges. Front Immunol 2021;12:676301. [Crossref] [PubMed]
- Lim M, Xia Y, Bettegowda C, et al. Current state of immunotherapy for glioblastoma. Nat Rev Clin Oncol 2018;15:422-42. [Crossref] [PubMed]
- Weissenberger J, Priester M, Bernreuther C, et al. Dietary curcumin attenuates glioma growth in a syngeneic mouse model by inhibition of the JAK1,2/STAT3 signaling pathway. Clin Cancer Res 2010;16:5781-95. [Crossref] [PubMed]
- Rahaman SO, Harbor PC, Chernova O, et al. Inhibition of constitutively active Stat3 suppresses proliferation and induces apoptosis in glioblastoma multiforme cells. Oncogene 2002;21:8404-13. [Crossref] [PubMed]
- Zhang L, Alizadeh D, Van Handel M, et al. Stat3 inhibition activates tumor macrophages and abrogates glioma growth in mice. Glia 2009;57:1458-67. [Crossref] [PubMed]
- Chen J. The Cell-Cycle Arrest and Apoptotic Functions of p53 in Tumor Initiation and Progression. Cold Spring Harb Perspect Med 2016;6:a026104. [Crossref] [PubMed]
- Mello SS, Attardi LD. Deciphering p53 signaling in tumor suppression. Curr Opin Cell Biol 2018;51:65-72. [Crossref] [PubMed]
- Zawlik I, Kita D, Vaccarella S, et al. Common polymorphisms in the MDM2 and TP53 genes and the relationship between TP53 mutations and patient outcomes in glioblastomas. Brain Pathol 2009;19:188-94. [Crossref] [PubMed]
- Ishii N, Maier D, Merlo A, et al. Frequent co-alterations of TP53, p16/CDKN2A, p14ARF, PTEN tumor suppressor genes in human glioma cell lines. Brain Pathol 1999;9:469-79. [Crossref] [PubMed]
- Zhang Y, Dube C, Gibert M Jr, et al. The p53 Pathway in Glioblastoma. Cancers (Basel) 2018;10:297. [Crossref] [PubMed]
- Massagué J. TGFbeta in Cancer. Cell 2008;134:215-30. [Crossref] [PubMed]
- Bierie B, Moses HL. Tumour microenvironment: TGFbeta: the molecular Jekyll and Hyde of cancer. Nat Rev Cancer 2006;6:506-20. [Crossref] [PubMed]
- Yang L, Pang Y, Moses HL. TGF-beta and immune cells: an important regulatory axis in the tumor microenvironment and progression. Trends Immunol 2010;31:220-7. [Crossref] [PubMed]
- Graham CD, Kaza N, Klocke BJ, et al. Tamoxifen Induces Cytotoxic Autophagy in Glioblastoma. J Neuropathol Exp Neurol 2016;75:946-54. [Crossref] [PubMed]
- Tseng SH, Wang CH, Lin SM, et al. Activation of c-Jun N-terminal kinase 1 and caspase 3 in the tamoxifen-induced apoptosis of rat glioma cells. J Cancer Res Clin Oncol 2004;130:285-93. [Crossref] [PubMed]
(English Language Editor: J. Jones)