Identification of novel key genes associated with uterine corpus endometrial carcinoma progression and prognosis
Highlight box
Key findings
• Based on the integrated bioinformatic analyses, our data indicated that GINS4 and ESR1 might serve as potential prognostic markers and targets for UCEC therapy.
What is known and what is new?
• Surgery, chemotherapy, hormonal therapy, radiotherapy targeted therapy, and immunotherapy (such as the programmed cell death protein 1 inhibitor) are the available treatment choices for patients with UCEC.
• This study identified GINS4 and ESR1 as key genes associated with UCEC progression and prognosis, thus offering an opportunity for therapeutic optimization.
What is the implication, and what should change now?
• We should note that the main limitation of our study is that the prognostic prediction was carried out in TCGA-UCEC cohort and GSE17025 cohort in which clinical information is not sufficient. The 2-gene signature needs to be validated in multiple and larger UCEC cohorts in future studies, and the related molecular mechanisms should be further clarified.
Introduction
Uterine corpus endometrial carcinoma (UCEC), an epithelial neoplasm arising from the innermost layer of the uterus, is the most frequent cancer type and the second leading cause of gynecological cancer death affecting the health of women in developed countries (1,2). According to the 2020 Global Cancer Incidence, Mortality and Prevalence (GLOBOCAN) global cancer statistics, an estimated 417,367 cases were newly diagnosed with UCEC and almost 97,370 deaths occurred in patients with UCEC in 2020 (3). The incidence and mortality of UCEC are estimated to be rapidly increasing worldwide. The incidence is higher in developed countries than in developing countries, while cancer mortality is relatively high in low-income countries (4,5). Although the majority of UCEC occurs in postmenopausal women, about 5% of premenopausal patients are younger than 40 years and may have fertility needs (6).
At present, surgery, chemotherapy, hormonal therapy, radiotherapy targeted therapy, and immunotherapy (such as the programmed cell death protein 1 inhibitor) are the available treatment choices for patients with UCEC. The clinical stage, histopathology, and grade are all important factors that affect treatment options and prognosis of patients (1). Patients diagnosed with early-stage UCEC usually have favorable prognosis, with an overall 5-year survival rate of about 90%; however, the prognosis for advanced or recurrent patients is not satisfactory (7-9), and patients have a 10% to 15% chance of recurrence within 3 years (10,11). Because of the unidentified genetic heterogeneity, patients with UCEC exhibit different outcomes (12). Identifying the gene expression diversity could contribute to optimizing clinical treatments, and it is particularly important to identify high-risk patients. There is still a dearth of effective prognosis predictors for UCEC, and thus discovering more accurate and reliable prognosis biomarkers is urgently needed. The aim of this study was thus to identify novel and potential prognostic biomarkers in UCEC using bioinformatic analysis techniques and public database resources.
With the application of high-throughput RNA sequencing and microarray technologies, huge amounts of data have been generated over the past decades, which facilitate the discovery of potential biomarkers of different cancer types via bioinformatic analysis. Comparing the gene expression levels in samples between different groups, especially between cancer and normal groups, is a now common and widely used method for exploring differentially expressed genes (DEGs). However, determining the relationships between gene transcripts with pairwise correlations has not been extensively undertaken, and the chance of type I and type II errors occurring grows with a higher number of comparisons (13). One useful method to improve this situation is to divide genes with similar expression patterns into clusters or modules. Weighted gene co-expression network analysis (WGCNA) is a useful method for constructing a gene coexpression network and overviewing the gene expression profile, which can avoid the multiple comparison problems (14-16). WGCNA has been proven to be an exceedingly effective approach for identifying potential biomarkers or therapeutic targets (17-22). Different from the protein-protein interaction (PPI) network which reflect relationships between proteins, or the competing endogenous RNA (ceRNA) regulatory network which evaluate the associations between RNAs, WGCNA begins with the generation of scale-free gene coexpression networks that describe the pairwise expression similarities among genes (23-25). Then, modules are detected using unsupervised clustering with hierarchical clustering as a default method choice. Highly interconnected genes are clustered into the same module. Many bioinformatic analyses aim to explore hub genes associated with clinical phenotypes. Instead of testing the association between an individual gene to a sample trait, WGCNA focuses on measuring the significance between a module and the information of the external clinical phenotype because the intramodular genes are densely correlated. Thereafter, several options such as module eigengenes (MEs) and intramodular connectivity measures are available for describing the clinically significant modules. Intramodular hub genes which have a high module membership and gene significance are identified. Gene Ontology (GO) annotation and Kyoto Encyclopedia of Genes and Genomes (KEGG) pathway information can be helpful in further describing the intramodular genes by predicting the potential functions.
In this study, the top 50% genes with the greatest variance among primary UCEC samples in The Cancer Genome Atlas (TCGA) database were used for WGCNA network construction. DEG analysis between UCEC tumor and normal control endometrial tissues was subsequently conducted in TCGA-UCEC cohort with matched normal controls. After identifying hub genes in hub modules, key genes were identified by intersecting the hub genes and DEGs. These overlapping key genes were then applied for further survival analysis. The univariable and multivariate Cox proportional hazards regression analyses generated a prognostic risk model consisting of 2 genes, GINS complex subunit 4 (GINS4) and estrogen receptor 1 (ESR1). This risk model was demonstrated to be an independent prognostic indicator according to Kaplan-Meier curves and receiver operating characteristic (ROC) curves as well as the univariate and multivariate Cox proportional hazards regression analyses. Expression patterns of GINS4 and ESR1 were also performed and validated in samples from TCGA and GSE17025, respectively. Protein expression levels of these genes were acquired from the Human Protein Atlas (HPA). Although GINS4 has been rarely studied in UCEC, the protein encoded by ESR1 has been shown to be negatively associated with epithelial-mesenchymal transition in UCEC (26). Thus, this study identified GINS4 and ESR1 to be key genes associated with UCEC progression and prognosis, thus offering an opportunity for further therapeutic optimization. We present the following article in accordance with the STREGA reporting checklist (available at https://atm.amegroups.com/article/view/10.21037/atm-22-6461/rc).
Methods
Data collection and preparation
RNA high-throughput sequencing counts and clinical information for patients with UCEC were directly downloaded from TCGA data repository (https://portal.gdc.cancer.gov/; Project ID: TCGA-UCEC). The “GDCRNATools” R package (The R Foundation for Statistical Computing) was used for data preprocessing, which facilitated the organization and integrative analysis of RNA expression data in the Genomic Data Commons (GDC) Data Portal (27). After removal of the duplicated samples, raw count data of 543 primary UCEC tumor samples and 35 solid tissue normal samples were normalized and transformed by running the “gdcVoomNormalization” function in R. The ensemble ID was converted based on Ensembl 90 annotation (Cambridge, UK). The study was conducted in accordance with the Declaration of Helsinki (as revised in 2013).
Screening for differentially expressed genes
To identify the significant DEGs between primary UCEC tumor samples and normal solid tissue samples, the “gdcDEAnalysis” package in R was applied, which provided 3 methods, “Limma”, “edgeR”, and “DESeq2” for analysis. In this study, the Limma method was adopted. The DEGs were defined as genes with |log2FC| >1 and false discovery rate (FDR) <0.05.
Construction of a weighted coexpression network
WGCNA has been widely used to identify gene modules and genes of interest. The “WGCNA” package in R facilitates weighted correlation network construction and analysis (14). To explore the modules of interest related to UCEC, the top 50% genes with the greatest variance among primary TCGA-UCEC samples were selected to generate the weighted coexpression network via the “WGCNA” R package. The power of β was chosen by the function “pickSoftThreshold” to produce a scale-free network. Genes with a high similarity of expression profile were grouped into a module based on the “blockwiseModules” function. The minimum module size was set at 30, and “mergeCutHeight” was set at 0.25.
Identification of clinically relevant and functional modules
Module eigengene (ME), could represent the gene expression profile of an indicated module. To further identify key modules associated with UCEC, the relationships between MEs and clinical traits (histologic type, grade, clinical stage, and age at initial diagnosis) were calculated by Pearson correlation coefficient. Modules with a higher absolute value of module significance (MS) were considered to be more biologically significant and were chosen for further analysis.
Genes of modules with high trait significance were selected to undergo GO and KEGG analyses with “clusterProfiler” R package. The cutoff criteria were set as P values <0.05.
Identification of key genes
The gene significance (GS) refers to the biological significance of an intramodular gene and is measured by the correlation between an indicated gene and a clinical phenotype. The module membership (MM), which represents the intramodular connectivity between an intramodular gene and the ME, is measured by Pearson correlation coefficient. In this study, hub genes were defined as |GS| >0.3 and |MM| >0.7, and were further selected to intersect with DEGs. The overlapping genes were obtained and defined as the key genes.
Survival analyses of key genes
Survival analyses according to both RNA expression and survival information were performed on 536 patients with UCEC in TCGA. We selected key genes associated with patients’ overall survival (OS) through univariable Cox proportional hazards regression analysis. Genes significantly associated with OS were obtained for further multivariate Cox proportional hazards regression analysis. A risk score was then calculated as follows: risk score = coef1 × expression of gene1 + coef2 × expression of gene2 + coef3 × expression of gene3 + coefi × expression of genei, in which coef is the gene’s regression coefficient in the multivariate Cox hazard model analysis. To estimate the predictive efficiency of the risk score, Kaplan-Meier curves and the receiver operating characteristic (ROC) curves were drawn.
Furthermore, the risk score and clinical characteristics were subjected to univariate and multivariate Cox proportional hazards regression analyses. These analyses were conducted with the “survival” and “survminer” R packages.
Gene set enrichment analysis
All patient with UCEC from TCGA were separated into high-risk and low-risk groups based on the median risk score. Gene set enrichment analysis (GSEA) software was then used to identify the underlying functions of the key genes. We chose c2.cp.kegg.v7.0.symbols.gmt as the reference gene sets. NOM (nominal) P<0.05 and FDR q<0.25 were set as the cutoff criteria.
Clinical correlation analysis based on The University of ALabama at Birmingham CANcer data analysis Portal (UALCAN)
UALCAN web (http://ualcan.path.uab.edu/) facilitates researchers to analyze protein-coding gene expression and survival data in various cancer types (28,29). With the assistance of UALCAN, the correlation between gene expression levels (in the form of transcripts per million) with different histologic subtypes, clinical stages, and TP53 mutation status in patients with UCEC were determined. Based on Clinical Proteomic Tumor Analysis Consortium (CPTAC) samples, GINS4 and ESR1 expression in UCEC cases with p53/Rb-related pathway alteration, MYC/MYCN alteration, mTOR pathway alteration, WNT pathway alteration, or HIPPO pathway alteration were compared with those in normal tissues at the protein level. Moreover, the overall survival curve for patients with UCEC with different gene expression profiles in TCGA cohort was generated.
Validation of gene expression
Gene expression files and clinical data of GSE17025 (contributed by Risinger et al.) were extracted from the Gene Expression Omnibus (GEO) database (https://www.ncbi.nlm.nih.gov/geo/) (30-32). Gene expression data from 12 noncancer endometrial samples and 91 samples of stage I endometrial cancers with different histologic types and grades were collected from the GSE17025 data set. The raw CEL files were preprocessed using the robust multichip average (RMA) algorithm. Gene annotation was based on GPL570 platform. The difference of gene expression in samples with different histologic grades (normal, G1/2, and G3) and histologic types (normal, endometrioid, and papillary serous cancers) were calculated with analysis of variance (ANOVA) at a P value <0.05 using GraphPad Prism version 9.0 (GraphPad Software, San Diego, CA, USA).
In addition, immunohistochemistry was used to measure the expression of key genes in the control endometrial samples and UCEC samples in the HPA (http://www.proteinatlas.org).
Statistical analysis
All data were performed using R or GraphPad Prism software. Descriptive data were represented as mean ± standard derivation (SD). One-way ANOVA was used to compare the means of three or more groups. Kaplan-Meier survival curves were calculated and compared. A P value less than 0.05 would be considered to be statistically significant.
Results
Weighted coexpression network of UCEC
The overall workflow of this study is presented in Figure 1. After removal of the duplicated samples, the top 50% [7,592] of messenger RNAs (mRNAs) with the greatest variance from the 543 UCEC samples were analyzed by WGCNA. Figure 2A shows the sample clustering dendrogram for detecting outliers, and there were no obvious outliers. To achieve a scale-free network distribution, we chose a soft threshold power of 4 (scale-free R2=0.87; Figure 2B,2C). Highly coexpressed mRNAs were clustered into the same module, and 19 modules were finally identified after merging similar modules (Figure 2D). The gray module included mRNAs that were not assigned to any of the modules.
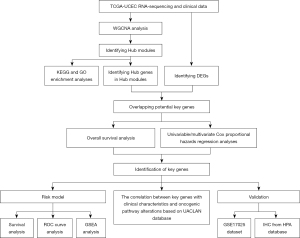
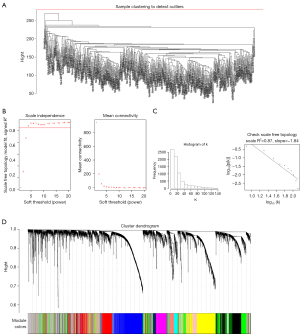
Identification of clinically relevant modules
Correlations between MEs and clinical traits were calculated to identify modules with clinical significance. The eigengene dendrogram and eigengene adjacency heatmap demonstrated 2 clusters. Five highly correlated MEs in 1 cluster were associated with histologic grade, histologic type, clinical stage, and age. Among them, the yellow ME was densely correlated with histologic grade (Figure 3). The yellow module showed the highest positive association with histologic grade (r=0.53; P=2e-41), while the red module showed the highest negative association with histologic grade (r=–0.5; P=5e-36). The ME of the yellow module also had a positive correlation with histologic type (r=0.31; P=1e-13) and clinical stage (r=0.22; P=2e-7). The ME of the red module negatively correlated with histologic type (r=–0.7; P=2e-80) and clinical stage (r=–0.35; P=8e-17) (Figure 4A).
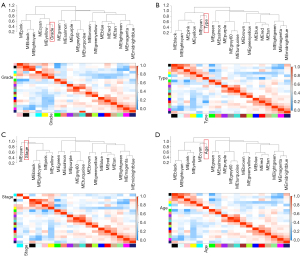
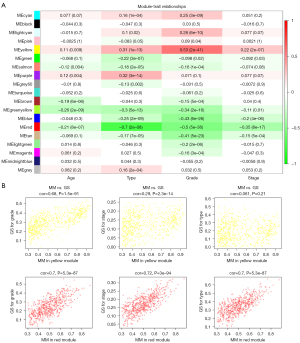
MM the yellow module and red module was highly correlated with gene significance for histologic grade [yellow module: correlation (cor) =0.68, P=1.5e-91; red module: cor =0.7, P=5.3e-87]. These data indicated strong relationships between histologic grade and these 2 MEs. Moreover, there were significant correlations between MM in the red module versus GS for clinical stage (cor =0.72; P=3e-94) and type (cor =0.7; P=5.3e-87). The correlation between GS for clinical stage and MM in yellow module was low (cor =0.29, P=2.3e-14; Figure 4B). Thus, the yellow module containing 666 genes and the red module containing 583 genes were defined as modules of interest and were selected for further analysis.
To further describe yellow and red modules, GO functional and KEGG pathway analyses were conducted. KEGG pathway analysis indicated that the genes in the yellow module were involved in cancer-associated pathways, including cell cycle pathway, cellular senescence pathway, Fanconi anemia pathway, DNA replication pathway, and p53 signaling pathway (Figure 5A). As shown in Figure 5B, the enriched GO terms in the yellow module, according to biological process (BP) analysis, were primarily nuclear division, organelle fission and chromosome segregation, mitotic nuclear division, DNA replication, nuclear chromosome segregation, sister chromatid segregation, mitotic sister chromatid segregation, DNA-dependent DNA replication, and regulation of chromosome segregation. According to cellular component (CC) analysis, these genes were enriched in the chromosomal region, and according to molecular function (MF) analysis, they were enriched in DNA-dependent ATPase activity. As indicated by KEGG pathway analysis, genes in the red module were mainly enriched in pathways of axon guidance, growth hormone synthesis, secretion and action, inflammatory mediator regulation of transient receptor potential (TRP) channels, relaxin signaling pathway, and estrogen signaling pathway (Figure 5C). Additionally, red module genes were most enriched in axonogenesis for the BP group, glutamatergic synapse for the CC group, and secondary active transmembrane transporter activity for the MF group (Figure 5D).
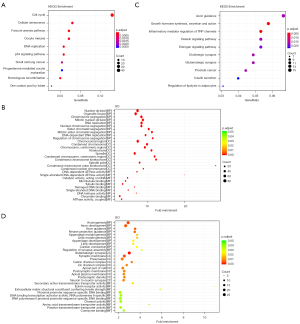
Identification of key genes in the yellow and red modules
The higher GS indicated higher biological significance, and the higher MM suggested higher intramodular connectivity. Hub genes with high GS and MM were selected for further analysis. We measured the GS and MM of every gene in the yellow and red modules. In total, 177 genes in the yellow module and 21 genes in the red module were identified as genes with high biological significance and high intramodular connectivity. Moreover, 4,003 DEGs were screened out between 543 TCGA-UCEC samples and 35 normal samples according to the cutoff criteria (Figure 6A). By combining the hub genes in yellow and red modules with DEGs, we could identify the 164 overlapping genes as the key genes (Figure 6B).
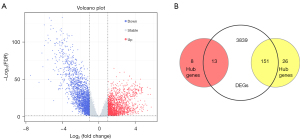
Survival analysis of key genes
As the prognosis of UCEC is largely affected by cancer progression, the association between overlapping key genes with OS was analyzed by a univariable Cox proportional hazards regression analysis. Results showed that 15 genes were significantly associated with the OS (Table 1). Among them, higher expression of 13 key genes in the yellow module predicted poor OS; conversely, increased expression of 2 key genes in the red module predicted good OS. These 15 genes were then further selected to for multivariate Cox proportional hazards regression analysis (Table 1). Finally, GINS4 and ESR1 were obtained to build the risk score, which was calculated as follows: risk score = (0.40863 × GINS4 value) + (–0.18708 × ESR1 value). Therefore, we focused on GINS4 and ESR1 in next analyses.
Table 1
Symbol | Univariate analysis | Multivariate analysis | |||||
---|---|---|---|---|---|---|---|
P | HR | 95% CI | P | HR | 95% CI | ||
PIMREG | 0.0084 | 1.4995 | 1.1094–2.0267 | 0.5345 | 1.1901 | 0.6873–2.0606 | |
PRR11 | 0.0139 | 1.4591 | 1.0798–1.9716 | 0.8700 | 1.0472 | 0.6029–1.8189 | |
CDC25A | 0.0346 | 1.4184 | 1.0257–1.9716 | 0.6251 | 0.8666 | 0.4880–1.5390 | |
MCM4 | 0.0205 | 1.4881 | 1.0632–2.0829 | 0.6637 | 0.8556 | 0.4237–1.7279 | |
HMMR | 0.0177 | 1.4235 | 1.0632–1.9058 | 0.3638 | 1.2186 | 0.7953–1.8673 | |
ESPL1 | 0.0270 | 1.3926 | 1.0385–1.8675 | 0.4203 | 0.8042 | 0.4734–1.3662 | |
E2F2 | 0.0497 | 1.3518 | 1.0005–1.8264 | 0.9917 | 1.0029 | 0.5837–1.7232 | |
CENPO | 0.0151 | 1.6508 | 1.1016–2.4737 | 0.5466 | 0.7753 | 0.3390–1.7733 | |
GINS4 | 0.0014 | 1.5783 | 1.1922–2.0894 | 0.0237 | 1.6961 | 1.0730–2.6810 | |
CKS1B | 0.0336 | 1.3569 | 1.0239–1.7980 | 0.6900 | 0.9024 | 0.5449–1.4946 | |
PLK1 | 0.0235 | 1.4254 | 1.0488–1.9372 | 0.6864 | 1.1374 | 0.6088–2.1250 | |
E2F1 | 0.0258 | 1.3359 | 1.0356–1.7232 | 0.8275 | 1.0556 | 0.6487–1.7178 | |
MCM2 | 0.0368 | 1.3559 | 1.0189–1.8043 | 0.9695 | 1.0125 | 0.5367–1.9100 | |
PGR | 0.0013 | 0.8652 | 0.7920–0.9453 | 0.9580 | 0.9957 | 0.8483–1.1687 | |
ESR1 | 0.0001 | 0.8110 | 0.7279–0.9036 | 0.0251 | 0.8001 | 0.6583–0.9725 |
TCGA, The Cancer Genome Atlas; UCEC, uterine corpus endometrial carcinoma; HR, hazard ratio; CI, confidence interval.
The Kaplan-Meier survival curve indicated that the OS rates in patients with higher GINS4 expression were worse compared with patients with low or medium expression levels. On the contrary, patients with low or medium ESR1 expression levels had good OS rates (Figure 7A). The patients with UCEC and survival information were stratified into high- (n=268) and low-risk (n=268) groups according to the median risk score. To estimate the prognostic value of the 2-gene signature, the Kaplan-Meier curve was applied. Results showed patients in the low-risk group tended to survive longer than those in high-risk group (P=0.0033; Figure 7B). In addition, the AUCs for the prediction of 1-, 2-, 3-, and 5-year OS were 0.8, 0.74, 0.7, and 0.72, respectively (Figure 7C). We also conducted GSEA to explore the potential mechanism of GINS4 and ESR1 on the OS of UCEC. The results revealed that several important pathways, including cell cycle pathway, pathways in several cancers, DNA replication pathway, basal transcriptional factors pathway, RNA degradation pathway, oocyte meiosis, and progesterone-mediated oocyte maturation pathway, were highly enriched in the high-risk group (Figure 7D). In summary, our 2-gene signature (containing GINS4 and ESR1) appeared to serve as a predictor for OS in TCGA-UCEC patients. Further prognostic analysis of the risk score built with the expression of GINS4 and ESR1 in different subsets of TCGA-UCEC was performed. Kaplan-Meier survival analysis indicated that the 2-gene signature risk score could also serve as a good prognostic marker for younger patients with UCEC or patients with low and high grade endometrioid type or advanced stage disease (Figure 8). ROC analysis demonstrated good performance of the 2-gene signature risk score in predicting 1-year OS for patients with UCEC in all subgroups as well as for the 1-, 2-, 3-, and 5-year survival of younger patients or patients with low-grade UCEC (Figure 9).
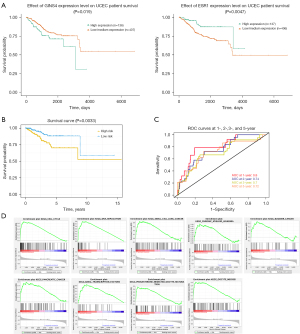
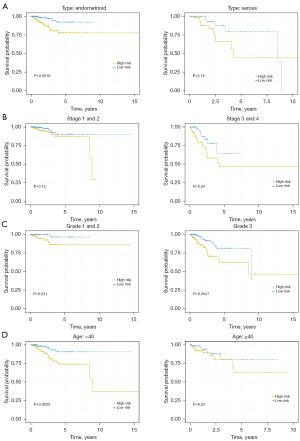
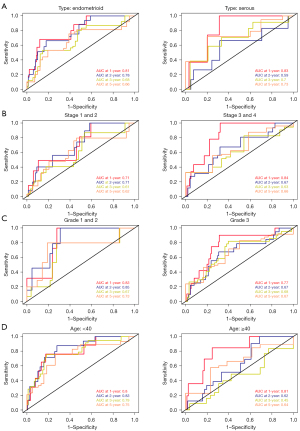
To further determine whether the 2-gene signature was an independent prognostic marker among clinical characteristics, univariable Cox proportional hazards regression analysis was conducted. Data indicated that a high-risk score, late stage, high histologic grade, and serous subtype were significantly associated with poor OS, while age at diagnosis could not predict survival. Meanwhile, the multivariate Cox proportional hazards regression analysis indicated clinical stage and risk score to be independent prognostic factors in patients with UCEC (Table 2).
Table 2
Variables | Univariate analysis | Multivariate analysis | |||||
---|---|---|---|---|---|---|---|
P | HR | 95% CI | P | HR | 95% CI | ||
Risk score | <0.0001 | 1.5434 | 1.2764–1.8662 | 0.0263 | 1.3487 | 1.0360–1.7559 | |
Stage | <0.0001 | 1.9287 | 1.4953–2.4876 | 0.0002 | 1.6598 | 1.2761–2.1588 | |
Grade | 0.0009 | 2.8795 | 1.5428–5.3742 | 0.0655 | 1.8854 | 0.9602–3.7018 | |
Type | 0.0133 | 1.4755 | 1.0843–2.0081 | 0.7974 | 0.9576 | 0.6881–1.3328 |
TCGA, The Cancer Genome Atlas; UCEC, uterine corpus endometrial carcinoma; HR, hazard ratio; CI, confidence interval.
The correlation between GINS4 and ESR1 with clinical characteristics and oncogenic pathway alterations
To explore the association between gene expression and clinical characteristics, including tumor stage, histologic subtype, pathological grade, and TP53 mutation status, TCGA-UCEC patients were divided into different subsets according to the common characteristics.
Results showed that GINS4 was expressed at a significantly higher level in tumors with advanced disease stage, histologic grade, and serous endometrial carcinoma. In contrast, the expression of ESR1 and clinical traits (disease stage, histologic grade, and serous endometrial adenocarcinoma) was inversely correlated. The overexpression of GINS4 was associated with TP53-mutant status. In contrast, in the UACLAN database, ESR1 expression was negatively correlated with TP53-mutant status (Figure 10A). Moreover, in CPTAC samples, UCEC tissues with p53/Rb-related pathway alteration, MYC/MYCN alteration, mTOR pathway alteration, WNT pathway alteration, or HIPPO pathway alteration had higher protein levels of GINS4 and ESR1 than did normal tissues (Figure 10B). These data indicated that overexpression of GINS4 and decreased expression of ESR1 were associated with progression in UCEC.
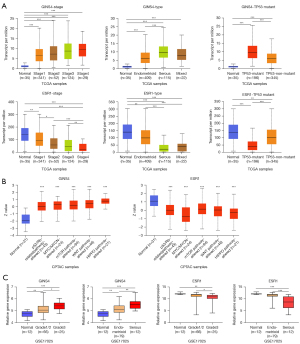
Validation of the key genes of GINS4 and ESR1
GINS4 and ESR1 were subsequently selected for validation in the GSE17025 cohort. The expression levels of GINS4 and ESR1 in 12 control samples and 91 endometrial cancer samples with stage I and different histologic grades and types were compared. The results demonstrated that both GINS4 and ESR1 were closely related to histologic grade and histologic type, suggesting their potential for identifying high-grade and papillary serous endometrial cancers from stage I endometrial cancers (Figure 10C). In addition, immunohistochemistry showed a high expression of GINS4 and low expression of ESR1 in UCEC tissues from the HPA (data not shown).
Discussion
The prognosis of patients with advanced and recurrent UCEC remains unsatisfactory. UCEC has traditionally been divided into 2 subgroups. Type I endometrioid endometrial carcinoma accounts for about 70–80% of UCEC. This type occurs mainly in young women and is characterized by estrogen excess, obesity, and positivity of estrogen and progesterone receptors. Uterine serous carcinoma, as the major subset of type II UCEC, is observed in relatively older and nonobese women and has low levels of estrogen or progesterone receptors and frequent mutations in TP53 (17,33,34). Endometrioid endometrial carcinoma usually has a significantly better outcome than does serous haplotype, which is often less well differentiated (33-36). TCGA Research Network carried out array and sequencing analyses in 373 UCEC samples, and classified UCEC into 4 subsets according to the genomic features. It also found that about a quarter of high-grade type I tumors and serous tumors have a similar molecular phenotype. Oncogenic mutant p53 (mtp53) occurred frequently in serous carcinoma (>90%) and in about a third of grade 3 endometrioid endometrial carcinomas (34,37,38). The new classification based on genomic features may lead to more appropriate cancer treatments (34). As it is hard for pathologists to classify high-grade endometrioid endometrial carcinomas and serous carcinomas, discovering novel biomarkers that may help improve patient management is of great importance.
In this study, 19 modules were identified through WGCNA based on data from TCGA-UCEC. The yellow module and red module displayed high correlation with clinical traits. We next performed KEGG and GO enrichment analyses. Results showed that genes in the yellow module were mainly enriched in cancer-associated pathways. It is well documented that pathways of cell cycle, cellular senescence, DNA replication, p53 signaling pathway, and homologous recombination are associated with cell growth and proliferation, both of which are crucial mechanisms of UCEC tumorigenesis and tumor progression (39-43). Recent studies have also shown that Fanconi anemia pathway can mediate DNA repair and is involved in many human cancers including UCEC (44,45). Consistent with the involvement of these cancer-associated pathways, GO enrichment analysis also suggested yellow module genes mainly participate in UCEC tumor cell growth and proliferation. Red module genes were mainly associated with axon guidance pathway, inflammatory mediator regulation of TRP channels, and some hormone-related signaling pathways. Several axonal guidance proteins, such as Slits and L1 cell adhesion molecule, have been reported to be associated with tumor cell proliferation, migration, and metastasis (46,47). Some iron channels, especially TRP channels, could be highly expressed in several cancers and may serve as promising pharmacological target in UCEC (48-50). Previous studies have indicated that the growth hormone axis, relaxin signaling pathway, and estrogen signaling pathway play important roles in UCEC growth and invasion and are associated with survival outcome (51-56). It was thus reasonable to speculate that exploring these enriched pathways may assist in understanding the mechanisms of UCEC progression and developing novel antitumor therapies.
Among the 198 hub genes we identified in these 2 modules, 164 genes were shared DEGs. Univariate and multivariate Cox regression analyses indicated that GINS4 and ESR1 were significantly associated with survival. Based on the GINS4 and ESR1 expression pattern, the risk score, with the potential to be an independent prognostic factor, was generated. GSEA indicated that oncogenic associated pathways, including cell cycle, DNA replication, basal transcription factors, small cell lung cancer, pancreatic cancer, and chronic myeloid leukemia were enriched in the high-risk group. Both RNA expression data from the GEO and TCGA databases as well as protein expression data based on UALCAN and the HPA website suggested that GINS4 was overexpressed and ESR1 was underexpressed in UCEC. High expression of GINS4 and loss of ESR1 were associated with high grade, late stage, serous cancer subtype, and poor prognosis.
GINS4, the GINS complex subunit 4, can form the hetero-tetrameric GINS complex together with GINS1, GINS2 and GINS3. Notably, the GINS complex plays a key role in the initiation and elongation process of DNA and chromosome replication (57). The “CMG (Cdc45-MCM-GINS)” complex, consisting of the GINS complex, CDC45, and MCM2-7, serves as a complex and highly conserved eukaryotic replicative ring helicase, which is responsible for unwinding double-strand DNA and recruiting other important elements during DNA replication (58-60). It has been suggested that CMG might be a potential drug target (61). Multiple studies have proven that the expression of human GINS (hGINS) is associated with cell proliferation. When hGINS expression is downregulated, cell circle progression can be prevented (62). It was reported that most of the mutations that occur in human cancers can be attributed to DNA replication errors and that genomic stability can be preserved by normal DNA replication forks (63,64).
An accumulating amount of evidence indicates that GINS4 figures prominently in several cancer types. Elevated levels of GINS4 have been detected in various cancers, for example, cervical squamous cell carcinoma, pancreatic cancer, glioblastoma, and lung adenocarcinoma. GINS4 is also widely studied as a prognostic marker in a multiple cancers (65-68). Liu et al. not only were the first to identify GINS4 as an independent prognostic predictor for glioma, but also showed GINS4 to be associated with the glioma immune microenvironment through immune cells and immune checkpoints (69). Another study demonstrated that GINS4 could promote tumor cell proliferation and metastasis in gastric cancer. Potential molecular mechanisms could involve GINS4 binding Rac1/CDC42 directly to activate Rac1/CDC42, leading to the activation of downstream pathways; the study further indicated that GINS4 could be a promising treatment target and early diagnostic marker for gastric cancer (70). Similarly, GINS4 has been demonstrated to be able to promote tumorigenesis, migration, and metastasis in other cancer types, such as lung cancer and colorectal cancer (67). MAPK/ERK pathway, PI3K/AKT pathway, and PTEN pathway have been reported to be affected by GINS4. Knockdown of GINS4 has been shown to inhibit cancer proliferation and progression in lung cancer, colorectal cancer, and pancreatic cancer (67,68,71). However, to the best of our knowledge, the effect of GINS4 on the UCEC cells has rarely been studied.
Consistent with previous studies, our analyses revealed that GINS4 was overexpressed in UCEC tissues. In this study, the results also showed that GINS4 expression had a positive relationship with UCEC cancer stage, pathological grade, serous subtype, and TP53-mutant status. Patients with overexpression of GINS4 had significantly shortened survival times than did patients with low GINS4 expression.
The ESR1 gene encodes estrogen receptor alpha (ERα). The protein encoded by this gene acts as a key mediator of estrogen responses and takes a part in growth, sexual development, gestation and other functions by regulating estrogen-inducible gene expression (72). ESR1 gene amplification has been widely reported in some cancer types, especially breast carcinoma (73,74). Compared with breast cancer patients without ESR1 amplification, patients with ESR1 amplification could benefit from endocrine therapy (75). Although ESR1 amplification has been reported to occur in endometrial carcinomas by some studies, Yu et al. and Rahman et al. suggest ESR1 amplification to not be associated with clinicopathological characteristics of clinical stage, histologic grade, metastasis, or invasion (75,76). Rahman et al. also reported that that estrogen receptor expression correlated with better progression-free survival, OS, and disease nonrecurrence, suggesting ESR1 amplification as an independent prognostic marker (76). Moreover, the loss of ER has been reported to be associated with alternated phosphoinositide 3 kinase/mammalian target of Rapamycin (PI3K/mTOR) pathway, epithelial-mesenchymal transition, lymph node metastasis, and poor survival even in low-grade UCEC, while positive ER expression has been associated with good differentiation and longer survival in several studies (26,77-79). Liu et al. identified 4 distinct transcriptome subsets according to clinicopathologic features and mutation profiles in 271 endometrioid endometrial carcinomas. Although both cluster I and II tumors were mainly low-grade and early-stage tumors, their molecular profiles were different. Cluster I tumors had higher expression levels of ESR1 and tended to have a better prognosis compared to cluster II tumors with the CTNNB1 exon 3 mutation (73). In another study, Guan et al. used immunohistochemistry to assess ER expression on grade I–II endometrioid endometrial carcinoma and found negativity of ER expression to be associated with severe stage, deeper myometrial infiltration, metastasis, and shorter progression-free survival and OS (79). Data from these studies implied that ESR1 might serve as a protective factor in UCEC.
Phenotypic factors like the clinical stage, histologic type, and grade are critically involved in the prognosis of UCEC. Therefore, our findings indicate that GINS4 and ESR1 may be key genes associated with UCEC progression and prognosis. Integration of GINS4 and ESR1 expression into clinical risk estimation may improve prognostic prediction and treatment options for UCEC. GINS4 and ESR1 have the potential to be applied for the diagnostic biomarker for UCEC, which should be further validated on external and large cohorts.
We should note the principal limitation of our study, which is that the prognostic prediction was carried out in the TCGA-UCEC cohort and GSE17025 cohort in which some information on the clinical features was unavailable. This 2-gene signature should be validated in multiple and larger UCEC cohorts in future studies, and the possible molecular mechanisms involved should be further investigated.
Conclusions
GINS4 and ESR1 were associated with clinicopathological features. Overexpression of GINS4 and downregulation of ESR1 were unfavorable prognostic marker in UCEC. These genes might be potential molecular targets for cancer therapy.
Acknowledgments
We would like to acknowledge the GEO, TCGA, UALCAN, CPTAC, and HPA for access to their databases, and thank the contributors for uploading their valuable data sets.
Funding: This research was funded, in part, by grants from Project of National Key Research and Development Plan “National Key R&D Program of China” (No. 2019YFE0117300), the Guangxi Science and Technology Base and Talents Project (Nos. GuiKe-AD20238062 and GuiKe-AA20325001), the Key Research and Development Project of Guangxi (No. GuiKe-AAB21196024), and the Guangxi Key Laboratory of Nanobody Research (No. 21-220-16).
Footnote
Reporting Checklist: The authors have completed the STREGA reporting checklist. Available at https://atm.amegroups.com/article/view/10.21037/atm-22-6461/rc
Conflicts of Interest: All authors have completed the ICMJE uniform disclosure form (available at https://atm.amegroups.com/article/view/10.21037/atm-22-6461/coif). The authors have no conflicts of interest to declare.
Ethical Statement: The authors are accountable for all aspects of the work in ensuring that questions related to the accuracy or integrity of any part of the work are appropriately investigated and resolved. The study was conducted in accordance with the Declaration of Helsinki (as revised in 2013).
Open Access Statement: This is an Open Access article distributed in accordance with the Creative Commons Attribution-NonCommercial-NoDerivs 4.0 International License (CC BY-NC-ND 4.0), which permits the non-commercial replication and distribution of the article with the strict proviso that no changes or edits are made and the original work is properly cited (including links to both the formal publication through the relevant DOI and the license). See: https://creativecommons.org/licenses/by-nc-nd/4.0/.
References
- Vinuesa L, Webster RM. The endometrial carcinoma market. Nat Rev Drug Discov 2022;21:255-6. [Crossref] [PubMed]
- Huvila J, Pors J, Thompson EF, et al. Endometrial carcinoma: molecular subtypes, precursors and the role of pathology in early diagnosis. J Pathol 2021;253:355-65. [Crossref] [PubMed]
- Sung H, Ferlay J, Siegel RL, et al. Global Cancer Statistics 2020: GLOBOCAN Estimates of Incidence and Mortality Worldwide for 36 Cancers in 185 Countries. CA Cancer J Clin 2021;71:209-49. [Crossref] [PubMed]
- Zhang S, Gong TT, Liu FH, et al. Global, Regional, and National Burden of Endometrial Cancer, 1990-2017: Results From the Global Burden of Disease Study, 2017. Front Oncol 2019;9:1440. [Crossref] [PubMed]
- Morice P, Leary A, Creutzberg C, et al. Endometrial cancer. Lancet 2016;387:1094-108. [Crossref] [PubMed]
- Navarria I, Usel M, Rapiti E, et al. Young patients with endometrial cancer: how many could be eligible for fertility-sparing treatment? Gynecol Oncol 2009;114:448-51. [Crossref] [PubMed]
- Colombo N, Creutzberg C, Amant F, et al. ESMO-ESGO-ESTRO Consensus Conference on Endometrial Cancer: diagnosis, treatment and follow-up. Ann Oncol 2016;27:16-41. [Crossref] [PubMed]
- Zheng M, Hu Y, Gou R, et al. Development of a seven-gene tumor immune microenvironment prognostic signature for high-risk grade III endometrial cancer. Mol Ther Oncolytics 2021;22:294-306. [Crossref] [PubMed]
- Bian J, Xu Y, Wu F, et al. Identification of a five-gene signature for predicting the progression and prognosis of stage I endometrial carcinoma. Oncol Lett 2020;20:2396-410. [Crossref] [PubMed]
- Sorbe B, Juresta C, Ahlin C. Natural history of recurrences in endometrial carcinoma. Oncol Lett 2014;8:1800-6. [Crossref] [PubMed]
- Sohaib SA, Houghton SL, Meroni R, et al. Recurrent endometrial cancer: patterns of recurrent disease and assessment of prognosis. Clin Radiol 2007;62:28-34; discussion 35-6. [Crossref] [PubMed]
- Zhou M, Zhang Z, Zhao H, et al. A novel lncRNA-focus expression signature for survival prediction in endometrial carcinoma. BMC Cancer 2018;18:39. [Crossref] [PubMed]
- Akobeng AK. Understanding type I and type II errors, statistical power and sample size. Acta Paediatr 2016;105:605-9. [Crossref] [PubMed]
- Langfelder P, Horvath S. WGCNA: an R package for weighted correlation network analysis. BMC Bioinformatics 2008;9:559. [Crossref] [PubMed]
- Zhang B, Horvath S. A general framework for weighted gene co-expression network analysis. Stat Appl Genet Mol Biol 2005;4:Article17.
- Fuller TF, Ghazalpour A, Aten JE, et al. Weighted gene coexpression network analysis strategies applied to mouse weight. Mamm Genome 2007;18:463-72. [Crossref] [PubMed]
- Liang L, Yu J, Li J, et al. Integration of scRNA-Seq and Bulk RNA-Seq to Analyse the Heterogeneity of Ovarian Cancer Immune Cells and Establish a Molecular Risk Model. Front Oncol 2021;11:711020. [Crossref] [PubMed]
- Zhou J, Guo H, Liu L, et al. Construction of co-expression modules related to survival by WGCNA and identification of potential prognostic biomarkers in glioblastoma. J Cell Mol Med 2021;25:1633-44. [Crossref] [PubMed]
- Tan R, Zhang G, Liu R, et al. Identification of Early Diagnostic and Prognostic Biomarkers via WGCNA in Stomach Adenocarcinoma. Front Oncol 2021;11:636461. [Crossref] [PubMed]
- Ling B, Liao X, Huang Y, et al. Identification of prognostic markers of lung cancer through bioinformatics analysis and in vitro experiments. Int J Oncol 2020;56:193-205. [Crossref] [PubMed]
- Rezaei Z, Ranjbaran J, Safarpour H, et al. Identification of early diagnostic biomarkers via WGCNA in gastric cancer. Biomed Pharmacother 2022;145:112477. [Crossref] [PubMed]
- Wang Y, Chen L, Ju L, et al. Novel Biomarkers Associated With Progression and Prognosis of Bladder Cancer Identified by Co-expression Analysis. Front Oncol 2019;9:1030. [Crossref] [PubMed]
- Xia L, Wang Y, et al. Integrated Bioinformatic Analysis of a Competing Endogenous RNA Network Reveals a Prognostic Signature in Endometrial Cancer. Front Oncol 2019;9:448. [Crossref] [PubMed]
- Cai Y, Cui J, Wang Z, et al. Comprehensive bioinformatic analyses of lncRNA-mediated ceRNA network for uterine corpus endometrial carcinoma. Transl Cancer Res 2022;11:1994-2012. [Crossref] [PubMed]
- Besso MJ, Montivero L, Lacunza E, et al. Identification of early stage recurrence endometrial cancer biomarkers using bioinformatics tools. Oncol Rep 2020;44:873-886. [Crossref] [PubMed]
- Wik E, Ræder MB, Krakstad C, et al. Lack of estrogen receptor-α is associated with epithelial-mesenchymal transition and PI3K alterations in endometrial carcinoma. Clin Cancer Res 2013;19:1094-105. [Crossref] [PubMed]
- Li R, Qu H, Wang S, et al. GDCRNATools: an R/Bioconductor package for integrative analysis of lncRNA, miRNA and mRNA data in GDC. Bioinformatics 2018;34:2515-7. [Crossref] [PubMed]
- Chandrashekar DS, Karthikeyan SK, Korla PK, et al. UALCAN: An update to the integrated cancer data analysis platform. Neoplasia 2022;25:18-27. [Crossref] [PubMed]
- Chandrashekar DS, Bashel B, Balasubramanya SAH, et al. UALCAN: A Portal for Facilitating Tumor Subgroup Gene Expression and Survival Analyses. Neoplasia 2017;19:649-58. [Crossref] [PubMed]
- Barrett T, Wilhite SE, Ledoux P, et al. NCBI GEO: archive for functional genomics data sets--update. Nucleic Acids Res 2013;41:D991-5. [Crossref] [PubMed]
- Day RS, McDade KK, Chandran UR, et al. Identifier mapping performance for integrating transcriptomics and proteomics experimental results. BMC Bioinformatics 2011;12:213. [Crossref] [PubMed]
- Day RS, McDade KK. A decision theory paradigm for evaluating identifier mapping and filtering methods using data integration. BMC Bioinformatics 2013;14:223. [Crossref] [PubMed]
- Setiawan VW, Yang HP, Pike MC, et al. Type I and II endometrial cancers: have they different risk factors? J Clin Oncol 2013;31:2607-18. [Crossref] [PubMed]
- Cancer Genome Atlas Research Network. Integrated genomic characterization of endometrial carcinoma. Nature 2013;497:67-73. [Crossref] [PubMed]
- Hsiang M, Chobrutskiy BI, Diaz M, et al. Chemical complementarity between immune receptors and cancer mutants, independent of antigen presentation protein binding, is associated with increased survival rates. Transl Oncol 2021;14:101069. [Crossref] [PubMed]
- Kośla K, Orzechowska M, Jędroszka D, et al. A Novel Set of WNT Pathway Effectors as a Predictive Marker of Uterine Corpus Endometrial Carcinoma-Study Based on Weighted Co-expression Matrices. Front Oncol 2019;9:360. [Crossref] [PubMed]
- Meng B, Hoang LN, McIntyre JB, et al. POLE exonuclease domain mutation predicts long progression-free survival in grade 3 endometrioid carcinoma of the endometrium. Gynecol Oncol 2014;134:15-9. [Crossref] [PubMed]
- Nelson GS, Pink A, Lee S, et al. MMR deficiency is common in high-grade endometrioid carcinomas and is associated with an unfavorable outcome. Gynecol Oncol 2013;131:309-14. [Crossref] [PubMed]
- Qiu P, Jie Y, Ma C, et al. Paired box 8 facilitates the c-MYC related cell cycle progress in TP53-mutation uterine corpus endometrial carcinoma through interaction with DDX5. Cell Death Discov 2022;8:276. [Crossref] [PubMed]
- Kong D, Hou Y, Li W, et al. LncRNA-ZXF1 stabilizes P21 expression in endometrioid endometrial carcinoma by inhibiting ubiquitination-mediated degradation and regulating the miR-378a-3p/PCDHA3 axis. Mol Oncol 2022;16:813-29. [Crossref] [PubMed]
- Li Q, Lei Y, Du W. A Novel Target of p53, TCF21, Can Respond to Hypoxia by MAPK Pathway Inactivation in Uterine Corpus Endometrial Carcinoma. DNA Cell Biol 2018;37:473-80. [Crossref] [PubMed]
- Musacchio L, Caruso G, Pisano C, et al. PARP Inhibitors in Endometrial Cancer: Current Status and Perspectives. Cancer Manag Res 2020;12:6123-35. [Crossref] [PubMed]
- Alnafakh RAA, Adishesh M, Button L, et al. Telomerase and Telomeres in Endometrial Cancer. Front Oncol 2019;9:344. [Crossref] [PubMed]
- Bi J, Areecheewakul S, Li Y, et al. MTDH/AEG-1 downregulation using pristimerin-loaded nanoparticles inhibits Fanconi anemia proteins and increases sensitivity to platinum-based chemotherapy. Gynecol Oncol 2019;155:349-58. [Crossref] [PubMed]
- Zheng C, Ren Z, Chen H, et al. FANCD2 promotes the malignant behavior of endometrial cancer cells and its prognostic value. Exp Cell Res 2022;421:113388. [Crossref] [PubMed]
- Sirohi VK, Popli P, Sankhwar P, et al. Curcumin exhibits anti-tumor effect and attenuates cellular migration via Slit-2 mediated down-regulation of SDF-1 and CXCR4 in endometrial adenocarcinoma cells. J Nutr Biochem 2017;44:60-70. [Crossref] [PubMed]
- Pasanen A, Tuomi T, Isola J, et al. L1 Cell Adhesion Molecule as a Predictor of Disease-Specific Survival and Patterns of Relapse in Endometrial Cancer. Int J Gynecol Cancer 2016;26:1465-71. [Crossref] [PubMed]
- Costa BP, Nunes FB, Noal FC, et al. Ion Channels in Endometrial Cancer. Cancers (Basel) 2022;14:4733. [Crossref] [PubMed]
- Stewart JM. TRPV6 as A Target for Cancer Therapy. J Cancer 2020;11:374-87. [Crossref] [PubMed]
- Chen X, Sooch G, Demaree IS, et al. Transient Receptor Potential Canonical (TRPC) Channels: Then and Now. Cells 2020;9:1983. [Crossref] [PubMed]
- Jeffery PL, Herington AC, Chopin LK. The potential autocrine/paracrine roles of ghrelin and its receptor in hormone-dependent cancer. Cytokine Growth Factor Rev 2003;14:113-22. [Crossref] [PubMed]
- Michalczyk K, Niklas N, Rychlicka M, et al. The Influence of Biologically Active Substances Secreted by the Adipose Tissue on Endometrial Cancer. Diagnostics (Basel) 2021;11:494. [Crossref] [PubMed]
- Fue M, Miki Y, Takagi K, et al. Relaxin 2/RXFP1 Signaling Induces Cell Invasion via the β-Catenin Pathway in Endometrial Cancer. Int J Mol Sci 2018;19:2438. [Crossref] [PubMed]
- Thanasupawat T, Glogowska A, Nivedita-Krishnan S, et al. Emerging roles for the relaxin/RXFP1 system in cancer therapy. Mol Cell Endocrinol 2019;487:85-93. [Crossref] [PubMed]
- Miki Y, Iwabuchi E, Takagi K, et al. Co-expression of nuclear heterogeneous nuclear ribonucleic protein K and estrogen receptor α in endometrial cancer. Pathol Res Pract 2022;231:153795. [Crossref] [PubMed]
- van Weelden WJ, van der Putten LJM, Inda MA, et al. Oestrogen receptor pathway activity is associated with outcome in endometrial cancer. Br J Cancer 2020;123:785-92. [Crossref] [PubMed]
- Labib K, Gambus A. A key role for the GINS complex at DNA replication forks. Trends Cell Biol 2007;17:271-8. [Crossref] [PubMed]
- Wasserman MR, Schauer GD, O'Donnell ME, et al. Replication Fork Activation Is Enabled by a Single-Stranded DNA Gate in CMG Helicase. Cell 2019;178:600-611.e16. [Crossref] [PubMed]
- Burnham DR, Kose HB, Hoyle RB, et al. The mechanism of DNA unwinding by the eukaryotic replicative helicase. Nat Commun 2019;10:2159. [Crossref] [PubMed]
- Onesti S, MacNeill SA. Structure and evolutionary origins of the CMG complex. Chromosoma 2013;122:47-53. [Crossref] [PubMed]
- Seo YS, Kang YH. The Human Replicative Helicase, the CMG Complex, as a Target for Anti-cancer Therapy. Front Mol Biosci 2018;5:26. [Crossref] [PubMed]
- Aparicio T, Guillou E, Coloma J, et al. The human GINS complex associates with Cdc45 and MCM and is essential for DNA replication. Nucleic Acids Res 2009;37:2087-95. [Crossref] [PubMed]
- Tomasetti C, Vogelstein B. Cancer etiology. Variation in cancer risk among tissues can be explained by the number of stem cell divisions. Science 2015;347:78-81. [Crossref] [PubMed]
- Tomasetti C, Li L, Vogelstein B. Stem cell divisions, somatic mutations, cancer etiology, and cancer prevention. Science 2017;355:1330-4. [Crossref] [PubMed]
- Lian YF, Li SS, Huang YL, et al. Up-regulated and interrelated expressions of GINS subunits predict poor prognosis in hepatocellular carcinoma. Biosci Rep 2018;38:BSR20181178. [Crossref] [PubMed]
- Usman M, Okla MK, Asif HM, et al. A pan-cancer analysis of GINS complex subunit 4 to identify its potential role as a biomarker in multiple human cancers. Am J Cancer Res 2022;12:986-1008.
- Yang R, Liu N, Chen L, et al. LSH interacts with and stabilizes GINS4 transcript that promotes tumourigenesis in non-small cell lung cancer. J Exp Clin Cancer Res 2019;38:280. [Crossref] [PubMed]
- Bu F, Zhu X, Yi X, et al. Expression Profile of GINS Complex Predicts the Prognosis of Pancreatic Cancer Patients. Onco Targets Ther 2020;13:11433-44. [Crossref] [PubMed]
- Liu B, Liu Z, Wang Y, et al. Overexpression of GINS4 is associated with poor prognosis and survival in glioma patients. Mol Med 2021;27:117. [Crossref] [PubMed]
- Zhu Z, Yu Z, Rong Z, et al. The novel GINS4 axis promotes gastric cancer growth and progression by activating Rac1 and CDC42. Theranostics 2019;9:8294-311. [Crossref] [PubMed]
- Rong Z, Luo Z, Zhang J, et al. GINS complex subunit 4, a prognostic biomarker and reversely mediated by Krüppel-like factor 4, promotes the growth of colorectal cancer. Cancer Sci 2020;111:1203-17. [Crossref] [PubMed]
- Deroo BJ, Korach KS. Estrogen receptors and human disease. J Clin Invest 2006;116:561-70. [Crossref] [PubMed]
- Liu Y, Patel L, Mills GB, et al. Clinical significance of CTNNB1 mutation and Wnt pathway activation in endometrioid endometrial carcinoma. J Natl Cancer Inst 2014;106:dju245. [Crossref] [PubMed]
- Holst F, Stahl PR, Ruiz C, et al. Estrogen receptor alpha (ESR1) gene amplification is frequent in breast cancer. Nat Genet 2007;39:655-60. [Crossref] [PubMed]
- Yu KD, Shao ZM. ESR1 gene amplification: another mechanism regulating the cellular levels of ERα. Nat Rev Cancer 2011;11:823-author reply 823. [Crossref] [PubMed]
- Rahman MT, Nakayama K, Rahman M, et al. ESR1 gene amplification in endometrial carcinomas: a clinicopathological analysis. Anticancer Res 2013;33:3775-81.
- Backes FJ, Walker CJ, Goodfellow PJ, et al. Estrogen receptor-alpha as a predictive biomarker in endometrioid endometrial cancer. Gynecol Oncol 2016;141:312-7. [Crossref] [PubMed]
- Lee CJ, Sung PL, Kuo MH, et al. Crosstalk between SOX2 and cytokine signaling in endometrial carcinoma. Sci Rep 2018;8:17550. [Crossref] [PubMed]
- Guan J, Xie L, Luo X, et al. The prognostic significance of estrogen and progesterone receptors in grade I and II endometrioid endometrial adenocarcinoma: hormone receptors in risk stratification. J Gynecol Oncol 2019;30:e13. [Crossref] [PubMed]
(English Language Editor: J. Gray)