Development and validation of nomogram for the prediction of preterm delivery based on patient characteristics and circulating inflammatory cells in patients with gestational diabetes mellitus
Highlight box
Key recommendations
• The nomogram including data of patient characteristics and circulating inflammatory cells is an excellent model for predicting preterm delivery in women with gestational diabetes mellitus (GDM) at the time of GDM diagnosis.
What was recommended and what is new?
• Maternal age and insulin use were known predictors for preterm delivery;
• Currently, research on the association between these 2 inflammation-related indicators [neutrophil-lymphocyte ratio (NLR) and monocyte counts] and pregnancy outcome is rare. Our study suggested that NLR and monocyte counts were significantly associated with the incidence of preterm delivery in patients with GDM, and visualized the effect of maternal age, insulin use, NLR and monocyte counts on preterm delivery through the nomogram model.
What is the implication, and what should change now?
• The nomogram model in this study appears to be a reliable approach for the prediction of preterm delivery and deserves further investigation.
Introduction
Gestational diabetes mellitus (GDM), a common pregnancy complication with increasing incidence, is defined as hyperglycemia that arises during pregnancy (1,2). The incidence of adverse pregnancy outcomes, such as preterm delivery (<37 weeks’ gestation), is increased due to GDM (3,4). To avoid pregnancy complications caused by hyperglycemia, women with GDM must maintain strict glycemic control. Lifestyle interventions, including nutritional therapy and exercise, are first-line treatment, and if ideal blood glucose levels cannot be achieved, additional pharmacological treatment is required (2,5). Insulin is the primary pharmacological treatment of choice for women whose blood glucose is poorly controlled by lifestyle interventions (6). Some studies have shown that after lifestyle interventions alone or with additional insulin, the incidence of preterm delivery in women with GDM decreases, however, some women with GDM still experience preterm delivery (7,8). The health care for premature infants places a heavy financial burden on families, and preterm delivery is also the leading cause of death in children under 5 years old (9). If potential preterm delivery in patients with GDM can be diagnosed early and then prevented as soon as possible, not only can adverse pregnancy outcomes be improved, but the pressure on the health care system can also be relieved. Therefore, effective methods are needed for early prediction of preterm delivery in women with GDM.
The development of GDM is closely related to multiple patient characteristics including age, body mass index (BMI), physical activity, smoking history, family history of diabetes mellitus, and insulin use (10). Specific patient characteristics, such as age 35 years or older, higher BMI, no insulin use, and so on, can increase the incidence of GDM (10,11). Moreover, the positive association between maternal age and preterm delivery in GDM remained significant, even after adjusting for relevant confounders (12). It has also been reported that maternal obesity was related to an increased risk of preterm delivery, but obese women with diabetes had an increased risk of preterm delivery, suggesting that blood glucose also plays a role while BMI affects preterm delivery (13). A model showed that patient characteristics, including maternal age, maternal diabetes, smoking history, and so on, can predict preterm delivery, but with a sensitivity of only 51.5%, and the model needs to be improved (14). Some studies have suggested that GDM is associated with changes in circulating inflammatory cells, including lymphocyte count, neutrophil count, and others, which are routinely tested in perinatal health care. These studies also found that circulating inflammatory cells are involved in the development of GDM (15-18). Furthermore, Toll-like receptor (TLR), expressed in certain inflammatory cells, is a factor contributing to elevated blood glucose in pregnant women, and is also important in preterm delivery (19). Based on the above data, we speculate that the combination of clinical characteristics and circulating inflammatory cells may better predict preterm delivery in women with GDM.
Therefore, this study aimed to evaluate the patient characteristics and circulating inflammatory cells of patients with GDM and to develop a nomogram to help find novel and practical obstetric biomarkers for predicting the preterm deliveries, allowing clinicians to take timely measures to prevent the occurrence of preterm deliveries in women with GDM at the time of GDM diagnosis. We present the following article in accordance with the TRIPOD reporting checklist (available at https://atm.amegroups.com/article/view/10.21037/atm-22-6223/rc).
Methods
Subjects and study design
This was a retrospective cohort study. From January 2017 to January 2022, 1,008 patients with GDM from the First Affiliated Hospital of Wenzhou Medical University were consecutively screened in this retrospective study. The inclusion criteria were as follows: aged ≥18 years, with GDM defined as the presence of at least 1 plasma glucose abnormality at 24–28 weeks of pregnancy [fasting plasma glucose ≥5.1 mmol/L, 60-min ≥10.0 mmol/L, 120-min ≥8.5 mmol/L by 75 g oral glucose tolerance test (OGTT)], and singleton pregnancy. The exclusion criteria were as follows: diabetes mellitus before pregnancy, multiple pregnancy, history of preterm deliveries, loss of research information, and serious complications or comorbidities including kidney disease, liver disease, or heart disease. Based on the inclusion and exclusion criteria, 806 patients were involved in this study. The data (564 patients) recorded from January 2017 to June 2020 were named the training cohort and further assigned to subgroups (term delivery group and preterm delivery group), and the data (242 patients) obtained from patients with GDM, from July 2020 to January 2022, were named the validation cohort (Figure 1).
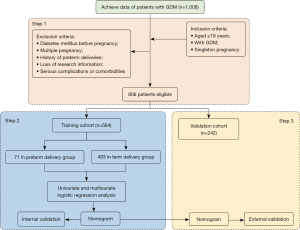
The study was conducted in accordance with the Declaration of Helsinki (as revised in 2013). The Ethics Committee of the First Affiliated Hospital of Wenzhou Medical University approved this study (No. KY2022-R201). Data collection was performed after written informed consents were provided by participants.
Data collection
The data collected in this study included age, BMI before pregnancy, family history of diabetes, smoking history, alcohol history, hypertension before pregnancy, parity, insulin use, OGTT result, lymphocyte count at GDM diagnosis, neutrophil count at GDM diagnosis, neutrophil-lymphocyte ratio (NLR) at GDM diagnosis, and monocyte count.
Construction of the nomogram
In the training cohort, univariate and multivariate logistic regression analyses were used to screen the independent predictors of the preterm deliveries in patients with GDM. The nomogram was established using the independent predictors from the multivariate logistic regression model to predict the risk of the preterm deliveries in patients with GDM.
Validation of the nomogram
Both the internal and external validation were conducted using 1,000 bootstrap resamples on the training and validation cohort, respectively. Concordance index (C-index) was conducted to internally and externally assess the discriminative power of the nomogram, respectively. The Hosmer-Lemeshow (HL) test and decision curve analysis (DCA) were conducted to internally and externally assess the calibration and the clinical applicability of the nomogram, respectively.
Statistical analysis
The sample size calculation was conducted using PASS 15 (NCSS Statistical Software, Kaysville, UT, USA). According to the result of sample size calculation with a power of 90%, the sample size was appropriate. Mann-Whitney U test and chi-square test were used to compare the skewed distributed (age, BMI before pregnancy, OGTT result, lymphocyte count at GDM diagnosis, neutrophil count at GDM diagnosis, NLR at GDM diagnosis, monocyte count), and categorical data (family history of diabetes, smoking history, alcohol history, hypertension before pregnancy, parity, insulin use), respectively. According to the results of univariate logistic regression analysis, the multivariate logistic regression model was developed in the training cohort. Then, the nomogram was established using the data from multivariate logistic regression model. The validation of the nomogram was conducted using 1,000 bootstrap resamples on the training and validation cohort, respectively. The area under the receiver operating characteristic (ROC) curve (AUC) with 95% confidence interval (95% CI), as well as the associated specificity and sensitivity, was used to assess the discrimination of the nomogram. The discrimination was also assessed with C-index which is roughly equivalent to the AUC. The calibration was evaluated by the calibration curves with the HL test. In addition, DCA was conducted to evaluate the clinical utility of nomogram. AUCs calculated from the ROC curve analyses were used to compare the diagnostic performances of each independent predictor and predictive model. Statistical analyses were performed using MedCalc version 18.2 (MedCalc Software Ltd., Ostend, Belgium) and R package version 4.2.1 (The R Foundation for Statistical Computing, Vienna, Austria). P<0.05 was considered significant, which was two-sided.
Results
In this study, the pregnancies of 71 patients in the training cohort (12.6%, 71/564) and 32 patients (13.2%, 32/242) in the validation cohort ended in preterm delivery. Baseline characteristics of patients in the 2 cohorts are summarized in Table 1. There were no significant differences in baseline characteristics, including patient characteristics and circulating inflammatory cells, between the 2 cohorts (all P>0.05) (Table 1).
Table 1
Variables | Training cohort (n=564) | Validation cohort (n=242) | P value |
---|---|---|---|
Patient characteristics | |||
Maternal age, years, median (IQR) | 28.7 (25.2, 33.9) | 28.4 (24.9, 32.8) | 0.308# |
BMI before pregnancy, kg/m2, median (IQR) | 23.5 (20.5, 25.8) | 23.4 (20.3, 25.9) | 0.631# |
Family history of diabetes mellitus, n (%) | 61 (10.8) | 28 (11.6) | 0.849* |
Smoking history, n (%) | 6 (1.1) | 2 (0.8) | 1.000* |
Alcohol drinking history, n (%) | 66 (11.7) | 26 (10.7) | 0.786* |
History of hypertension, n (%) | 36 (6.4) | 17 (7.0) | 0.856* |
Parity ≥1, n (%) | 206 (36.5) | 77 (31.8) | 0.229* |
Insulin use, n (%) | 59 (10.5) | 29 (12.0) | 0.609* |
Fasting PG at OGTT ≥5.1 mmol/L, median (IQR) | 5.2 (4.6, 6.0) | 5.3 (4.6, 6.0) | 0.955# |
1-h PG at OGTT ≥10.0 mmol/L, median (IQR) | 11.1 (10.0, 11.9) | 10.7 (9.7, 12.1) | 0.258# |
2-h PG at OGTT ≥8.5 mmol/L, median (IQR) | 10.2 (9.2, 10.8) | 10.2 (9.1, 10.9) | 0.769# |
Circulating inflammatory cells at GDM diagnosis, median (IQR) | |||
Lymphocyte count, ×109/L | 5.1 (3.1, 6.8) | 4.7 (3.0, 6.6) | 0.288# |
Neutrophil count, ×109/L | 20.4 (12.3, 27.3) | 18.6 (11.9, 25.5) | 0.135# |
NLR | 4.1 (3.6, 4.6) | 4.0 (3.4, 4.6) | 0.407# |
Monocyte count, ×109/L | 1.6 (1.3, 1.9) | 1.6 (1.3, 1.9) | 0.666# |
*, for chi-square test; #, for Mann-Whitney U-test. IQR, inter-quartile range; BMI, body mass index; PG, plasma glucose; OGTT, oral glucose tolerance test; GDM, gestational diabetes mellitus; NLR, neutrophil-lymphocyte ratio.
Independent predictors for preterm delivery
The results of the univariate and multivariate logistic analyses used to assess the relationship between potential factors and preterm delivery in the training cohort are shown in Table 2. On univariate analysis, maternal age, insulin use, neutrophil count, NLR, and monocyte count were associated with preterm delivery (P<0.001, P<0.001, P=0.049, P<0.001, and P<0.001, respectively), which was confirmed using the Mann-Whitney U test and chi-square test, respectively (Table S1). The multivariate analysis suggested that maternal age, insulin use, NLR, and monocyte count were the independent predictors of preterm delivery in patients with GDM (P<0.001, P=0.002, P<0.001, and P<0.001, respectively; Table 2).
Table 2
Variables | Univariate logistic regression | Multivariate logistic regression | |||||
---|---|---|---|---|---|---|---|
P value | OR | 95% CI | P value | OR | 95% CI | ||
Patient characteristics | |||||||
Maternal age | <0.001 | 1.088 | 1.053–1.123 | <0.001 | 1.093 | 1.049–1.140 | |
BMI before pregnancy | 0.332 | 1.035 | 0.966–1.109 | – | – | – | |
Family history of diabetes mellitus | 0.896 | 1.054 | 0.446–2.206 | – | – | – | |
Smoking history | 0.759 | 1.27 | 0.194–4.859 | – | – | – | |
Alcohol drinking history | 0.977 | 1.008 | 0.574–1.717 | – | – | – | |
History of hypertension | 0.078 | 2.109 | 0.865–4.636 | – | – | – | |
Parity ≥1 | 0.301 | 0.754 | 0.434–1.273 | – | – | – | |
Insulin use | <0.001 | 0.364 | 0.209–0.617 | 0.002 | 0.359 | 0.181–0.686 | |
Fasting PG at OGTT ≥5.1 mmol/L | 0.221 | 0.815 | 0.585–1.128 | – | – | – | |
1-h PG at OGTT ≥10.0 mmol/L | 0.573 | 1.065 | 0.857–1.327 | – | – | – | |
2-h PG at OGTT ≥8.5 mmol/L | 0.071 | 1.359 | 0.977–1.906 | – | – | – | |
Circulating inflammatory cells at GDM diagnosis | |||||||
Lymphocyte count | 0.495 | 0.962 | 0.860–1.075 | – | – | – | |
Neutrophil count | 0.049 | 1.026 | 1.000–1.054 | 0.762 | 0.995 | 0.945–1.030 | |
NLR | <0.001 | 4.281 | 2.828–6.713 | <0.001 | 3.431 | 2.153–5.762 | |
Monocyte count | <0.001 | 6.352 | 4.197–9.884 | <0.001 | 6.571 | 4.100–10.868 |
BMI, body mass index; PG, plasma glucose; OGTT, oral glucose tolerance test; GDM, gestational diabetes mellitus; NLR, neutrophil-lymphocyte ratio; OR, odds ratio; CI, confidence interval.
Nomogram establishment
A nomogram for predicting the probability of preterm delivery in patients with GDM was developed using the 4 independent predictors (maternal age, insulin use, NLR, and monocyte count) (Figure 2). For a 35-year-old woman with GDM who had insulin use during pregnancy, an NLR of 4, and a monocyte count of 2×109/L, the total point count was 124 (22.5+0+54+47.5); the risk was 10%.
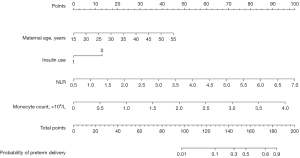
Nomogram verification
In the training cohort, the C-index was 0.885 (95% CI: 0.836–0.933), suggesting a good discrimination of this nomogram model, and the HL test (χ2=3.618, P=0.306) revealed a good calibration. Figure 3A,3B show the ROC (AUC =0.885, 95% CI: 0.855–0.910, sensitivity =83.0%, specificity =83.1%) and calibration curves of this nomogram used to assess the discrimination and calibration, respectively. The DCA revealed an obvious clinical net benefit (Figure 3C). In the validation cohort, the model also suggested good discrimination (AUC =0.919, 95% CI: 0.859–0.979) (Figure 3D) and good calibration (HL test: χ2=6.012, P=0.111). The ROC (AUC =0.919, 95% CI: 0.858–0.980, sensitivity =90.6%, specificity =84.8%), calibration and DCA curve were shown in Figure 3E,3F, respectively.
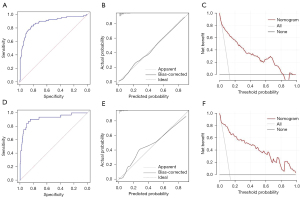
Predictive performances of each independent predictor and predictive model
Combined application of maternal age, insulin use, NLR, and monocyte count showed the highest AUC (AUC =0.885; 95% CI: 0.855–0.910; sensitivity =83.0%; specificity =83.1%; Table 3). This indicates that the predictive model had a better predictive power than the single predictors (maternal age, insulin use, NLR, and monocyte count) alone (Figure 4).
Table 3
Parameters | AUC (95% CI) | Sensitivity (%) | Specificity (%) | P value |
---|---|---|---|---|
Maternal age | 0.735 (0.696–0.771) | 80.3 | 69.2 | <0.001 |
Insulin use | 0.620 (0.578–0.660) | 70.4 | 53.5 | <0.001 |
NLR | 0.752 (0.714–0.787) | 36.6 | 98.2 | <0.001 |
Monocyte count | 0.846 (0.813–0.874) | 69.0 | 91.7 | <0.001 |
Predictive model | 0.885 (0.855–0.910) | 83.0 | 83.1 | <0.001 |
AUC, area under the curves; CI, confidence interval; NLR, neutrophil-lymphocyte ratio; ROC, receiver operating characteristic.
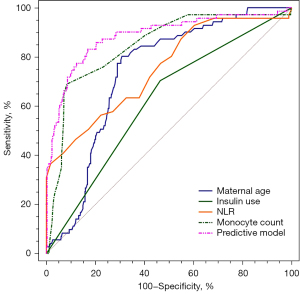
Discussion
Through accurate prediction of preterm deliveries in women with GDM at the time of GDM diagnosis, clinicians can take effective action to avoid the occurrence of preterm delivery as early as possible. To solve this problem, we constructed a nomogram using the 4 independent predictors (maternal age, insulin use, NLR, and monocyte count) to predict preterm deliveries in women with GDM at the time of GDM diagnosis. This nomogram model showed excellent performance and apparent clinical net benefit, suggesting that it might be a very good screening tool for preterm deliveries in women with GDM.
Over the past decades, delayed childbearing has developed an increasing trend worldwide (20). In China, pregnancies in women older than 35 years have increased by more than 10% over the past 10 years and account for approximately 30% of all pregnancies (21). Several studies have supported that advancing maternal age is an independent risk factor for the development of GDM, with a meta-analysis revealing that women aged 35–39 years had about twice the risk and those aged more than 40 years about 4 times the risk of women aged <35 years (20,22-25). In addition, a large study found an absolute risk of preterm delivery of 1.4% for women aged 35–39 and preterm delivery <34 weeks’ gestation of 2.0% (26). On the basis of previous studies, our study further confirmed that maternal age was an independent risk factor for preterm delivery in women with GDM, and visualized the effect of maternal age on preterm delivery through the nomogram model, which plays an important role in helping patients and clinicians to accurately assess pregnancy outcomes.
Women with GDM must maintain strict glycemic control to prevent pregnancy complications due to hyperglycemia (27). Insulin is regarded as the standard treatment for managing GDM when lifestyle interventions fail to achieve adequate glucose levels (28). A previous study revealed that the proportion of patients receiving insulin was significantly related to the proportion of patients experiencing adverse pregnancy outcomes, including preterm delivery (29). Our results showed that insulin use was an independent predictor for preterm delivery [odds ratio (OR) =0.359, P=0.002], consistent with the results of previous research, which might be caused by the positive correlation between the concentration of blood glucose and the incidence of adverse outcomes of pregnancy (29).
The development of GDM is complex. In addition to the impairment of the secretion of insulin, some studies have revealed that inflammation also plays a crucial role in the pathogenesis of GDM (15-18). As a subclinical inflammatory marker, the NLR demonstrates the state of inflammation and immune regulation. Decreased lymphocyte count and an abnormal inflammatory state may be responsible for increased NLR in diabetic patients (30,31). Currently, research on the association between NLR and pregnancy outcome is rare. This study confirmed that there was a significant increased risk of preterm delivery in women with GDM when NLR was increased (OR =3.431, P=0.000), explained by the fact that the higher level of inflammation is accompanied by the higher level of NLR.
Moreover, monocytes are an important marker of the inflammatory response and early prediction of GDM. An increased monocyte count was reported in pregnant women at different weeks of gestation (32-34). The importance of induction of insulin resistance via monocyte-secreted monocyte chemoattractant protein-1 (MCP-1) in the development of GDM has been reported (35). However, no studies have shown the relationship between monocytes and the pregnancy outcomes. In preclinical studies, toll-like receptor-4 (TLR-4), present in the monocytes, stimulated the release of inflammatory cytokines that caused the development of preterm delivery (36). To further confirm the conclusion of preclinical study in clinical practice, we observed the effect of monocytes on preterm delivery and found that monocyte counts were significantly associated with the incidence of preterm delivery in patients with GDM.
This study has 2 limitations. First, because the study was retrospective, information was partially missing for some patients. Second, all enrolled patients were from a single region, so studies involving patients in other regions need to be performed.
Conclusions
The visual nomogram including data of patient characteristics and circulating inflammatory cells is an excellent model for predicting preterm deliveries in women with GDM at the time of GDM diagnosis. This model appears to be a reliable approach for the prediction of preterm delivery and deserves further investigation.
Acknowledgments
Funding: The study was supported by Wenzhou Science and Technology Bureau, Basic Research Project (No. Y20210367).
Footnote
Reporting Checklist: The authors have completed the TRIPOD reporting checklist. Available at https://atm.amegroups.com/article/view/10.21037/atm-22-6223/rc
Data Sharing Statement: Available at https://atm.amegroups.com/article/view/10.21037/atm-22-6223/dss
Conflicts of Interest: All authors have completed the ICMJE uniform disclosure form (available at https://atm.amegroups.com/article/view/10.21037/atm-22-6223/coif). The authors have no conflicts of interest to declare.
Ethical Statement: The authors are accountable for all aspects of the work in ensuring that questions related to the accuracy or integrity of any part of the work are appropriately investigated and resolved. The Ethics Committee of the First Affiliated Hospital of Wenzhou Medical University approved the study (No. KY2022-R201). Data collection was performed after written informed consents were provided by participants. The study was conducted in accordance with the Declaration of Helsinki (as revised in 2013).
Open Access Statement: This is an Open Access article distributed in accordance with the Creative Commons Attribution-NonCommercial-NoDerivs 4.0 International License (CC BY-NC-ND 4.0), which permits the non-commercial replication and distribution of the article with the strict proviso that no changes or edits are made and the original work is properly cited (including links to both the formal publication through the relevant DOI and the license). See: https://creativecommons.org/licenses/by-nc-nd/4.0/.
References
- Shah NS, Wang MC, Freaney PM, et al. Trends in Gestational Diabetes at First Live Birth by Race and Ethnicity in the US, 2011-2019. JAMA 2021;326:660-9. [Crossref] [PubMed]
- ACOG Practice Bulletin No. 190: Gestational Diabetes Mellitus. Obstet Gynecol 2018;131:e49-64. [Crossref] [PubMed]
- Mottola MF, Artal R. Role of Exercise in Reducing Gestational Diabetes Mellitus. Clin Obstet Gynecol 2016;59:620-8. [Crossref] [PubMed]
- Chiefari E, Arcidiacono B, Foti D, et al. Gestational diabetes mellitus: an updated overview. J Endocrinol Invest 2017;40:899-909. [Crossref] [PubMed]
- 14. Management of Diabetes in Pregnancy: Standards of Medical Care in Diabetes-2019. Diabetes Care 2019;42:S165-72. [Crossref] [PubMed]
- Brown J, Grzeskowiak L, Williamson K, et al. Insulin for the treatment of women with gestational diabetes. Cochrane Database Syst Rev 2017;11:CD012037. [Crossref] [PubMed]
- HAPO Study Cooperative Research Group. Hyperglycemia and adverse pregnancy outcomes. N Engl J Med 2008;358:1991-2002. [Crossref] [PubMed]
- Teede HJ, Bailey C, Moran LJ, et al. Association of Antenatal Diet and Physical Activity-Based Interventions With Gestational Weight Gain and Pregnancy Outcomes: A Systematic Review and Meta-analysis. JAMA Intern Med 2022;182:106-14. [Crossref] [PubMed]
- Huang S, Tian J, Liu C, et al. Elevated C-reactive protein and complement C3 levels are associated with preterm birth: a nested case-control study in Chinese women. BMC Pregnancy Childbirth 2020;20:131. [Crossref] [PubMed]
- Ye W, Luo C, Huang J, et al. Gestational diabetes mellitus and adverse pregnancy outcomes: systematic review and meta-analysis. BMJ 2022;377:e067946. [Crossref] [PubMed]
- Schummers L, Hutcheon JA, Hacker MR, et al. Absolute risks of obstetric outcomes by maternal age at first birth: a population-based cohort. Epidemiology 2018;29:379-87. [Crossref] [PubMed]
- Lu L, Li JH, Dai XF, et al. Impact of advanced maternal age on maternal and neonatal outcomes in preterm birth. Ginekol Pol 2022; Epub ahead of print. [Crossref] [PubMed]
- Riley KL, Carmichael SL, Mayo JA, et al. Body Mass Index Change between Pregnancies and Risk of Spontaneous Preterm Birth. Am J Perinatol 2016;33:1017-22. [Crossref] [PubMed]
- Mehta-Lee SS, Palma A, Bernstein PS, et al. A Preconception Nomogram to Predict Preterm Delivery. Matern Child Health J 2017;21:118-27. [Crossref] [PubMed]
- Plows JF, Stanley JL, Baker PN, et al. The Pathophysiology of Gestational Diabetes Mellitus. Int J Mol Sci 2018;19:3342. [Crossref] [PubMed]
- Cinkajzlová A, Anderlová K, Šimják P, et al. Subclinical Inflammation and Adipose Tissue Lymphocytes in Pregnant Females With Gestational Diabetes Mellitus. J Clin Endocrinol Metab 2020;105:dgaa528. [Crossref] [PubMed]
- Wolf M, Sauk J, Shah A, et al. Inflammation and glucose intolerance: a prospective study of gestational diabetes mellitus. Diabetes Care 2004;27:21-7. [Crossref] [PubMed]
- Baz B, Riveline JP, Gautier JF. ENDOCRINOLOGY OF PREGNANCY: Gestational diabetes mellitus: definition, aetiological and clinical aspects. Eur J Endocrinol 2016;174:R43-51. [Crossref] [PubMed]
- Sun X, Sun H, Li P. Association of circulating inflammatory cells and platelets with gestational diabetes and pregnancy outcomes. Clin Chim Acta 2021;523:87-96. [Crossref] [PubMed]
- Lean SC, Derricott H, Jones RL, et al. Advanced maternal age and adverse pregnancy outcomes: A systematic review and meta-analysis. PLoS One 2017;12:e0186287. [Crossref] [PubMed]
- Shan D, Qiu PY, Wu YX, et al. Pregnancy Outcomes in Women of Advanced Maternal Age: a Retrospective Cohort Study from China. Sci Rep 2018;8:12239. [Crossref] [PubMed]
- Khalil A, Syngelaki A, Maiz N, et al. Maternal age and adverse pregnancy outcome: a cohort study. Ultrasound Obstet Gynecol 2013;42:634-43. [Crossref] [PubMed]
- Cleary-Goldman J, Malone FD, Vidaver J, et al. Impact of maternal age on obstetric outcome. Obstet Gynecol 2005;105:983-90. [Crossref] [PubMed]
- Jacobsson B, Ladfors L, Milsom I. Advanced maternal age and adverse perinatal outcome. Obstet Gynecol 2004;104:727-33. [Crossref] [PubMed]
- Waldenström U, Aasheim V, Nilsen ABV, et al. Adverse pregnancy outcomes related to advanced maternal age compared with smoking and being overweight. Obstet Gynecol 2014;123:104-12. [Crossref] [PubMed]
- Frederiksen LE, Ernst A, Brix N, et al. Risk of Adverse Pregnancy Outcomes at Advanced Maternal Age. Obstet Gynecol 2018;131:457-63. [Crossref] [PubMed]
- Picón-César MJ, Molina-Vega M, Suárez-Arana M, et al. Metformin for gestational diabetes study: metformin vs insulin in gestational diabetes: glycemic control and obstetrical and perinatal outcomes: randomized prospective trial. Am J Obstet Gynecol 2021;225:517.e1-517.e17. [Crossref] [PubMed]
- 14. Management of Diabetes in Pregnancy: Standards of Medical Care in Diabetes-2021. Diabetes Care 2021;44:S200-10. [Crossref] [PubMed]
- Farrar D, Simmonds M, Bryant M, et al. Hyperglycaemia and risk of adverse perinatal outcomes: systematic review and meta-analysis. BMJ 2016;354:i4694. [Crossref] [PubMed]
- Wang JR, Chen Z, Yang K, et al. Association between neutrophil-to-lymphocyte ratio, platelet-to-lymphocyte ratio, and diabetic retinopathy among diabetic patients without a related family history. Diabetol Metab Syndr 2020;12:55. [Crossref] [PubMed]
- Wang J, Zhu QW, Cheng XY, et al. Clinical significance of neutrophil-lymphocyte ratio and monocyte-lymphocyte ratio in women with hyperglycemia. Postgrad Med 2020;132:702-8. [Crossref] [PubMed]
- Sun T, Meng F, Zhao H, et al. Elevated First-Trimester Neutrophil Count Is Closely Associated With the Development of Maternal Gestational Diabetes Mellitus and Adverse Pregnancy Outcomes. Diabetes 2020;69:1401-10. [Crossref] [PubMed]
- Yang H, Zhu C, Ma Q, et al. Variations of blood cells in prediction of gestational diabetes mellitus. J Perinat Med 2015;43:89-93. [PubMed]
- Huang Y, Chen X, You ZS, et al. The value of first-trimester platelet parameters in predicting gestational diabetes mellitus. J Matern Fetal Neonatal Med 2022;35:2031-5. [Crossref] [PubMed]
- Nio Y, Yamauchi T, Iwabu M, et al. Monocyte chemoattractant protein-1 (MCP-1) deficiency enhances alternatively activated M2 macrophages and ameliorates insulin resistance and fatty liver in lipoatrophic diabetic A-ZIP transgenic mice. Diabetologia 2012;55:3350-8. [Crossref] [PubMed]
- Firmal P, Shah VK, Chattopadhyay S. Insight Into TLR4-Mediated Immunomodulation in Normal Pregnancy and Related Disorders. Front Immunol 2020;11:807. [Crossref] [PubMed]
(English Language Editor: J. Jones)