Metabolic signatures of lymphangioleiomyomatosis in biofluids: nuclear magnetic resonance (NMR)-based metabonomics of blood plasma: a case-control study
Highlight box
Key findings
• Methionine and acetic acid could be used as biomarkers for disease diagnosis.
Methionine was associated with pneumothorax and could be used as a risk marker
• What is known and what is new?
• The gold standard of previous diagnosis of LAM depends on histopathology;
NMR has good discriminatory power for LAM as a new non-invasive diagnostic method
• What is the implication, and what should change now?
• Noninvasive tests such as NMR could be used instead of pathological biopsy.
Introduction
Lymphangioleiomyomatosis (LAM) is a rare, low-grade malignant tumor, almost only affecting women (1,2). There are two types: sporadic LAM (S-LAM) without genetic background and LAM associated with the hereditary disease tuberous sclerosis complex (TSC-LAM) (2). The average incidence of S-LAM is about 4.9/1 million female population (3), and the incidence of TSC is ≈1/20,000 in the population, and ≈30–40% of adult female TSC patients are complicated with LAM. It has also been shown that 80% of female TSC patients aged 40 years show cystic changes in the lungs (4,5). Common symptoms include dyspnea, cough, chylous effusion, or pneumothorax, and with progression of the disease, lung function deteriorates. Extrapulmonary manifestations include renal angiomyolipoma (AML), and retroperitoneal lymphangioleiomyoma. TSC-LAM also has other multisystem clinical features of TSC, including effects on the nervous system and skin (4,6). At present, pulmonary or extrapulmonary pathological diagnosis is the gold standard for the diagnosis of LAM (2), but obtaining pathological specimens is traumatic for patients and a risk for adverse reactions such as pneumothorax and bleeding.
Excessive proliferation of LAM cells leads to abnormal lymphatic vessels, which is related to the involvement of vascular endothelial growth factor D (VEGF-D) in the occurrence of diseases (7,8). Serum VEGF-D level can be used as a non-invasive biomarker to distinguish LAM from other lung diseases (9). However, the limitation of VEGF-D is that it only increases in patients with severe lymphatic vessel involvement, and there are still many LAM patients whose VEGF-D does not increase (10). Cui et al. (11) found that about 14.5% of LAM patients did not have elevated levels of VEGF-D in their blood. This method has not been fully validated in Asian populations. Therefore, it is necessary to find new biomarkers to further improve the diagnostic efficacy of LAM and hopefully differentiate disease types.
The main molecular basis of LAM cell proliferation is TSC1 or TSC2 gene mutation, predominantly TSC2 mutation (12). The TSC1 and TSC2 proteins inhibit the activity of mammalian target of rapamycin (mTOR) in a complex manner in vivo. When TSC1 or TSC2 function is defective, mTOR is overactivated, leading to LAM cell proliferation and tumor development, which is the most critical pathogenesis of LAM and TSC (13,14). The mTOR signaling pathway plays a key role in the regulation of energy metabolism and cell proliferation. Because sirolimus has a specific inhibitory effect on mTOR targets it can be used for the treatment of LAM and TSC (15). Clinical study has shown that the main therapeutic effects of sirolimus include maintaining lung function, improving quality of life, reducing chylothorax, and reducing the volume of renal AML in LAM patients (16). However, not all patients benefit from sirolimus treatment, so new drugs for the treatment of this disease are needed.
The mTOR signaling pathway specifically plays an important role in lipid synthesis, nucleotide synthesis, and glucose metabolism. It regulates the expression of genes related to fatty acid and cholesterol biosynthesis by regulating the transcription factor sterol responsive element binding protein both through S6 kinase and phosphorylation of Lipin1 (17). mTOR complex 1 (mTORC1) supports cellular growth by regulating the balance between oxidative phosphorylation and glycolysis, increasing the activity of the pentose phosphate pathway. Lipids play a role in cancer cell growth and tumor progression, which leads to active metabolic reprogramming accompanied by the interaction of amino acid and lipid metabolism (18). The onset and progression of most diseases are accompanied by specific metabolic alterations. Therefore, metabolomics is helpful to explore the pathogenesis, diagnosis and treatment of diseases.
Nuclear magnetic resonance (NMR) is a new method for comprehensive and precise quantitative analysis of blood lipids and metabolites (19). NMR has considerable potential to identify clinically relevant biomarkers and monitor disease, which in turn presents opportunities to treat the disease with minimal trauma to the patient. Moreover, NMR detection is fast (12 minutes) and mature in technology. In this study, we used NMR detection technology to explore non-invasive biomarkers for the diagnosis and classification of LAM. We present the following article in accordance with the STARD reporting checklist (available at https://atm.amegroups.com/article/view/10.21037/atm-22-6420/rc).
Methods
Subjects and data collection
From January 2020 to January 2022, we consecutively enrolled 69 LAM patients from the outpatient department of the First Affiliated Hospital of Guangzhou Medical University. All subjects met the current standards of the American Thoracic Society/Japanese Respiratory Society (15). The diagnosis was mainly based on clinical history, lung high-resolution computed tomography (CT) (diffuse thin-walled cystic changes) and pathological (LAM cells) findings (15). Exclusion criteria were: (I) combined with other types of malignant tumor; (II) combined with rheumatic and endocrine diseases; and (III) incomplete data. Of the 69 LAM patients, 2 were excluded due to other comorbidities (1 with breast cancer, 1 with hyperthyroidism), so 67 patients entered the next stage of the study, and of them 4 patients were excluded for incomplete data and 2 patients refused blood sampling. Finally, a total of 61 LAM patients (51 S-LAM patients, 10 TSC-LAM patients) were included (Figure 1). The control group was 30 healthy women over 18 years old without a history of lung disease history recruited from the hospital’s physical examination center. LAM patients underwent clinical evaluation, including TSC clinical screening, pulmonary function examination, chest and abdominal CT to ascertain if AML and lymphoid tissue were involved.
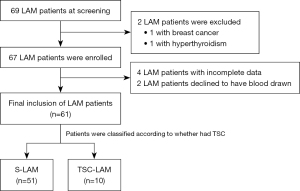
The purpose of this prospective study was to compare the plasma lipoproteins and metabolites between LAM patients and healthy subjects to determine the difference indicators, and the differences between the different types of LAM patients in order to provide a reference for the non-invasive diagnosis and classification of LAM. The physicians and laboratory staff who performed the tests were unaware of the details of the subjects in advance. The study was conducted in accordance with the Declaration of Helsinki (as revised in 2013). The study was approved by the Ethics Committee of the First Affiliated Hospital of Guangzhou Medical University (No. 2020-178) and informed consent was taken from all individual participants. The study was registered at http://www.chictr.org.cn (ChiCTR2100050460).
Plasma metabolomics assays
After all subjects had fasted for 10 hours, venous blood samples were drawn into vacuum tubes and stored at room temperature for 30 min before centrifugation (1,200 ×g for 10 min). Plasma samples were collected in vacuum tubes, stored at −80 ℃, and transported on dry ice. Samples were collected in the blood collection room of the hospital. Plasma samples were prepared and tested using Bruker IVDr Standard Operating Procedures (19) at ProteinT Biotechnology Co., Ltd. (Tianjin, China). Samples were thawed at room temperature, and 400-µL plasma samples were mixed thoroughly with 400 µL buffer (phosphate buffer pH 7.4 containing TSP-D4; Bruker, Rheinstetten, Germany), of which 600 µL was transferred to a 5-mm NMR tube for analysis.
The test was performed on a 600 MHz NMR AVANCE III HD spectrometer equipped with a BBI probe head and a SampleJet autosampler, adjusted to 6 ℃ during the test (Bruker Biospin). Before acquisition of sample data, each sample was automatically tuned and shimmed. The free induction decay signals (FIDs) were presented in the form of a Fourier-transformed spectrum, and automatic phase and baseline correction were performed in Toppin software as Bruker IVDr. The concentrations of metabolites were expressed as mmol/L (Figure S1).
Statistical analysis
Data were analyzed by the umbrella program (Protein T, Tianjin). Wilcoxon test, independent-sample t-test, or χ2 test were used to compare the data between two groups. The P value (<0.05) and fold change (FC) (<0.83 or >1.2) were used as screening criteria to find the difference indicators, which were subsequently modeled by logistic regression. A total of 99 models were constructed. Indicators with a frequency of 50-fold or more were defined as high-frequency indicators. The modeling process was random and divided into 7:3 sampling (i.e., 70% of the samples were training queues and 30% of the samples were verification queues). The model with strong stability was selected through multiple modeling. The model formula: total value = index 1*coef1 + index 2*coef2 + ... + intercept (intercept, constant; coef is the coefficient multiplied by the index). Finally, the diagnostic accuracy of the best predictive parameters was evaluated by the area under the receiver operating characteristic (ROC) curve (AUC), and the sensitivity and specificity were calculated. Indicators with a normal distribution are expressed as mean ± standard deviation. The Kruskal-Wallis test was used to analyze the correlation between multiple samples. P<0.05 was considered statistically significant, and all statistical tests were two-tailed. SPSS statistical package (version 21.0, IBM, Armonk, NY, USA) and R (version 4.0.3, R Foundation for Statistical Computing, Vienna, Austria) were used for data analysis and drawing.
Results
Basic demographic information and clinical characteristics (Table 1)
Table 1
Clinical characteristics | LAM (n=61) | S-LAM (n=51) | TSC-LAM (n=10) | P-value (S-LAM vs. TSC-LAM) |
---|---|---|---|---|
Age (years), mean ± SD | 40.70±8.59 | 40.90±8.68 | 39.70±8.49 | >0.05 |
Female, n (%) | 61 (100.00) | 51 (100.00) | 10 (100.00) | >0.05 |
Asian, n (%) | 61 (100.00) | 51 (100.00) | 10 (100.00) | >0.05 |
Smoking history | 0 | 0 | 0 | >0.05 |
Family history, n (%) | 3 (4.92) | 1 (1.96) | 2 (20.00) | 0.016 |
Biopsy, n (%) | 44 (72.13) | 37 (72.55) | 7 (70.00) | >0.05 |
Pneumothorax, n (%) | 28 (45.90) | 23 (45.10) | 5 (50.00) | >0.05 |
Chylothorax, n (%) | 5 (8.20) | 3 (5.88) | 2 (20.00) | >0.05 |
Extrapulmonary involvement, n (%) | 39 (63.93) | 29 (56.86) | 10 (100.00) | 0.009 |
Renal angiomyolipoma, n (%) | 23 (37.70) | 14 (27.45) | 9 (90.00) | <0.001 |
Liver, n (%) | 19 (31.15) | 13 (25.49) | 6 (60.00) | 0.031 |
Chylous ascites, n (%) | 4 (6.56) | 3 (5.88) | 1 (10.00) | >0.05 |
Hysteromyoma, n (%) | 9 (14.75) | 7 (13.73) | 2 (20.00) | >0.05 |
Retroperitoneal angiomyolipoma, n (%) | 13 (21.31) | 11 (21.57) | 2 (20.00) | >0.05 |
FEV1% predicted, mean ± SD | 70.26±27.50 | 66.93±28.51 | 87.66±10.99 | 0.001 |
FVC% predicted, mean ± SD | 90.63±16.03 | 89.47±16.68 | 96.68±10.79 | >0.05 |
FEV1/FVC, %, mean ± SD | 66.93±23.54 | 64.41±24.65 | 80.08±9.22 | 0.002 |
DLCO-SB% predicted, mean ± SD | 57.28±21.63 | 53.94±20.45 | 74.75±20.03 | 0.007 |
S, sporadic; TSC, tuberous sclerosis complex; LAM, lymphangioleiomyomatosis; DLCO-SB, diffusing capacity of the lungs for carbon monoxide-single breath; FEV1, forced expiratory volume in the first second; FVC, forced vital capacity; SD, standard deviation.
According to the clinical practice guidelines of the American Thoracic Society/Japanese Respiratory Society (15), the 61 stable female patients met the criteria (51 patients with S-LAM, 10 patients with TSC-LAM). All patients completed a CT scan of the lung before enrollment. Lung CT showed diffuse distribution of cysts in bilateral lungs, which was consistent with the characteristics of LAM. Blood samples were collected from patients within 1 month after diagnosis, and none had received any treatment at the time of blood drawing. The age and pulmonary function indexes of the patients were normally distributed data, expressed as mean ± standard deviation. There is no significant difference in most clinical indicators between S-LAM and TSC-LAM groups. All LAM patients and controls were Asian and their mean age was 40.70±8.59 years (40.90±8.68 years for S-LAM patients; 39.70±8.49 years for TSC-LAM patients). None had a smoking history, and 3 patients (4.92%) had a family history of LAM or TSC. Of the 61 patients, 44 (72.13%) underwent biopsy, 28 (45.90%) had pneumothorax, 5 (8.20%) had chylothorax, and 39 (63.93%) had extrapulmonary involvement. Among the extrapulmonary organs involved, renal AML accounted for the largest proportion (23 cases, 37.70%), followed by liver, retroperitoneal AMLs, hysteromyoma and chylous ascites. The proportion of extrapulmonary involvement in TSC-LAM group was significantly higher than that in S-LAM group, especially in kidney. We also tested lung function at baseline. Pulmonary ventilation function indexes included forced expiratory volume in the first second (FEV1)% predicted (70.26%±27.50%), forced vital capacity (FVC)% predicted (90.63%±16.03%), and FEV1/FVC% (66.93%±23.54%). The diffusion function [ diffusing capacity of the lungs for carbon monoxide-single breath% (DLCO-SB%) predicted] was 57.28%±21.63%.
Difference index analysis
Indicators with significant differences should simultaneously meet two criteria: P value of the comparison between the two groups <0.05, and FC >1.2 (upregulated) or <0.83 (downregulated). The full names corresponding to abbreviations of all indicators of differences are shown in Table S1.
Indicators of differences between LAM patients and healthy controls
There were 15 different lipoprotein indexes these groups, mainly the fifth and sixth components of low-density lipoprotein (LDL), specifically H2A2, V5FC, L6PL, L3TG, L6TG, L5FC, L6CH, L6FC, L6PN, L6AB, L5PN, L5AB, L5PL, L5CH, H1A2 (see Appendix for abbreviations). There were 18 differential metabolites, including methionine, acetic acid, alanine, histidine, N,N-dimethylglycine, sarcosine, acetoacetic acid, ornithine, dimethyl sulfone, glutamic acid, lysine, 3-hydroxybutyric acid, acetone, threonine, isoleucine, trimethylamine N-oxide, 2-aminobutyric acid, and creatine (all P<0.05 and FC >1.2 or <0.83, Table 2).
Table 2
Biochemical name | P value | FC |
---|---|---|
LAM vs. control | ||
Methionine | 3.263E−11 | 0.20 |
Acetic acid | 5.501E−13 | 4.25 |
Alanine | 1.107E−06 | 0.72 |
Histidine | 1.024E−06 | 0.81 |
N,N-dimethylglycine | 0.001 | 1.25 |
Sarcosine | 0.038 | 2.25 |
Acetoacetic acid | 0.001 | 1.60 |
Ornithine | 1.981E−04 | 0.35 |
Dimethyl sulfone | 2.670E−04 | 0.50 |
Glutamic acid | 0.009 | 1.70 |
Lysine | 3.008E−04 | 0.74 |
3-Hydroxybutyric acid | 7.266E−05 | 4.70 |
Acetone | 1.955E−04 | 1.71 |
Threonine | 0.015 | 0.24 |
Isoleucine | 0.015 | 0.82 |
Trimethylamine N-oxide | 0.020 | 1.29 |
2-Aminobutyric acid | 0.010 | 0.24 |
Creatine | 0.006 | 1.39 |
H2A2 | 1.501E−04 | 1.30 |
V5FC | 0.006 | 2.00 |
L6PL | 0.005 | 1.44 |
L3TG | 0.005 | 1.26 |
L6TG | 0.005 | 1.33 |
L5FC | 0.025 | 1.27 |
L6CH | 0.010 | 1.46 |
L6FC | 0.011 | 1.42 |
L6PN | 0.006 | 1.51 |
L6AB | 0.006 | 1.51 |
L5PN | 0.044 | 1.44 |
L5AB | 0.044 | 1.44 |
L5PL | 0.047 | 1.29 |
L5CH | 0.050 | 1.33 |
H1A2 | 0.023 | 1.60 |
S-LAM vs. control | ||
Methionine | 2.273E−10 | 0.20 |
Acetic acid | 3.995E−12 | 4.50 |
Alanine | 5.170E−06 | 0.72 |
Histidine | 2.066E−06 | 0.81 |
Acetoacetic acid | 4.555E−04 | 2.00 |
Dimethyl sulfone | 3.332E−04 | 0.50 |
N,N-Dimethylglycine | 0.001 | 1.25 |
3-Hydroxybutyric acid | 2.824E−05 | 4.87 |
Acetone | 7.109E−05 | 1.89 |
Ornithine | 0.001 | 0.39 |
Lysine | 0.001 | 0.75 |
Succinic acid | 0.003 | 1.33 |
Threonine | 0.025 | 0.56 |
Creatine | 0.011 | 1.35 |
Glutamic acid | 0.018 | 1.66 |
Trimethylamine N-oxide | 0.034 | 1.21 |
Isoleucine | 0.009 | 0.80 |
2-Aminobutyric acid | 0.003 | 0.24 |
L6PN | 0.015 | 1.42 |
V5FC | 0.016 | 1.89 |
L3TG | 0.008 | 1.27 |
L6TG | 0.017 | 1.27 |
L6CH | 0.027 | 1.38 |
L6FC | 0.024 | 1.41 |
L6PL | 0.014 | 1.41 |
L6AB | 0.015 | 1.42 |
H1A2 | 0.010 | 1.66 |
H2A2 | 5.03E−05 | 1.31 |
TSC-LAM vs. control | ||
Acetic acid | 2.80E−05 | 3.56 |
Creatinine | 2.57E−04 | 1.47 |
Methionine | 8.94E−05 | 0.19 |
Alanine | 0.003 | 0.80 |
Ornithine | 0.002 | 0.31 |
Lysine | 0.006 | 0.56 |
Trimethylamine N-oxide | 0.028 | 1.95 |
Glutamic acid | 0.045 | 2.20 |
V5FC | 0.023 | 3.39 |
V4CH | 0.019 | 1.53 |
IDPN | 0.013 | 1.71 |
IDAB | 0.013 | 1.71 |
V4PL | 0.031 | 1.32 |
H4PL | 0.015 | 1.24 |
L6PL | 0.009 | 1.61 |
L6CH | 0.013 | 1.65 |
L6TG | 0.011 | 1.68 |
L6PN | 0.019 | 1.75 |
S-LAM vs. TSC-LAM | ||
Creatinine | 0.0004 | 1.43 |
Acetone | 0.036 | 0.76 |
FC >1.2 = significantly upregulated, FC <0.83 = significantly downregulated, P<0.05 = significant difference. FC, fold change; S, sporadic; TSC, tuberous sclerosis complex; LAM, lymphangioleiomyomatosis.
Indicators of differences between S-LAM patients and healthy controls
There were 10 different lipoprotein indexes between these groups, mainly the sixth components of LDL, specifically V5FC, L3TG, L6CH, L6TG, L6FC, L6PL, L6PN, L6AB, H1A2, H2A2. There were 18 differential metabolites, including methionine, acetic acid, alanine, histidine, acetoacetic acid, dimethyl sulfone, N,N-dimethylglycine, 3-hydroxybutyric acid, acetone, ornithine, lysine, succinic acid, threonine, creatine, glutamic acid, trimethylamine N-oxide, isoleucine, and 2-aminobutyric acid (all P<0.05 and FC >1.2 or <0.83, Table 2).
Indicators of differences between TSC-LAM patients and healthy controls
There were 14 different lipoprotein indexes between these two groups, mainly the sixth components of LDL, specifically V4CH, V4PL, V5FC, IDPN, IDAB, L5FC, L6CH, L6TG, L6FC, L6PL, L6PN, L6AB, H4FC, H4PL. There were 8 differential metabolites, including acetic acid, creatinine, methionine, alanine, ornithine, lysine, trimethylamine N-oxide, and glutamic acid (all P<0.05 and FC >1.2 or <0.83, Table 2).
Indicators of differences between S-LAM and TSC-LAM
The metabolites with significant difference between S-LAM and TSC-LAM were creatinine and acetone (all P<0.05 and FC >1.2 or <0.83, Table 2). There was no significant difference in lipoprotein between the two subgroups.
Model building
Selection of indicators with significant difference between LAM patients and healthy controls for logistic regression analysis
The 15 differential lipoprotein indexes and 18 differential metabolites obtained by comparing LAM patients with healthy controls were modeled by logistic regression. The high-frequency indexes appearing in the modeling were acetic acid, alanine, methionine, sarcosine, creatine, V5FC, 2-aminobutyric acid, glutamic acid, histidine, H2A2, threonine, L3TG, and isoleucine (Figures 2,3).
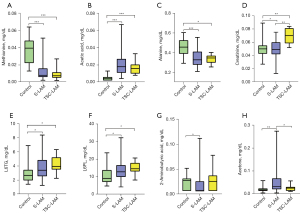
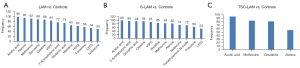
The indicators in the median model were histidine, methionine, 2-aminobutyric acid, alanine, threonine, isoleucine, L3TG, L6TG, H2A2, trimethylamine N-oxide, glutamic acid, creatine, ornithine, sarcosine, dimethyl sulfone, N,N-dimethylglycine, and acetic acid (Figure 2, Table 3).
Table 3
Group | Name | Coefficient |
---|---|---|
LAM vs. Control | Histidine | −56.25 |
Methionine | −51.27 | |
2-Aminobutyric acid | −46.00 | |
Alanine | −21.79 | |
Threonine | −7.20 | |
Isoleucine | −2.57 | |
L3TG | 0.31 | |
L6TG | 0.84 | |
H2A2 | 1.21 | |
Trimethylamine N-oxide | 2.46 | |
Glutamic acid | 8.78 | |
Creatine | 14.90 | |
Ornithine | 169.06 | |
Sarcosine | 255.47 | |
Dimethyl sulfone | 348.10 | |
N,N-dimethylglycine | 469.56 | |
Acetic acid | 746.67 | |
Intercept | −8.27 | |
S-LAM vs. Control | Creatinine | −115.30 |
Trimethylamine N-oxide | −43.66 | |
Alanine | −0.46 | |
L6TG | 0.12 | |
H4PL | 0.33 | |
V5FC | 3.88 | |
Ornithine | 7.44 | |
Glutamic acid | 11.14 | |
Acetic acid | 742.47 | |
Intercept | −11.47 | |
TSC-LAM vs. Control | Methionine | −37.07 |
Alanine | −0.79 | |
L6PL | 0.12 | |
Creatinine | 32.52 | |
Acetic acid | 107.40 | |
Intercept | −3.78 |
S, sporadic; TSC, tuberous sclerosis complex; LAM, lymphangioleiomyomatosis.
In the validation cohort, the AUC value of the median model was 0.9737, and the sensitivity and specificity of the model were 100% and 89%, respectively (Figure 4A). In all subjects in this group, ROC curves (Figure 4B) were drawn separately for the high-frequency indicators or indicators that appeared in the median model to distinguish patients from healthy people. Among them, the indicators with higher diagnostic efficacy were methionine (AUC =0.929, sensitivity =73.8%, specificity =100%, cut-off value =0.011 mmol/L) and acetic acid (AUC =0.966, sensitivity =95.1%, specificity =90%, cut-off value =0.006 mmol/L) (Table 4).
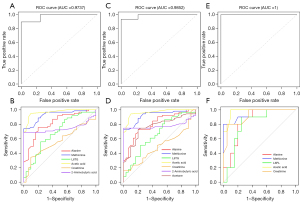
Table 4
Group | Name | AUC | Sensitivity (%) | Specificity (%) | Cut-off value (mmol/L) |
---|---|---|---|---|---|
LAM vs. control | Alanine | 0.816 | 75.4 | 80 | 0.387 |
Methionine | 0.929 | 73.8 | 100 | 0.011 | |
L6TG | 0.681 | 55.7 | 76.7 | 3.370 | |
Acetic acid | 0.966 | 95.1 | 90 | 0.006 | |
Creatinine | 0.565 | 34.4 | 90 | 0.059 | |
2-Aminobutyric acid | 0.744 | 68.9 | 100 | 0.003 | |
S-LAM vs. control | Alanine | 0.805 | 72.5 | 80 | 0.387 |
Methionine | 0.924 | 72.5 | 100 | 0.011 | |
L6TG | 0.660 | 86.3 | 43.3 | 2.330 | |
Acetic acid | 0.963 | 94.1 | 90 | 0.006 | |
Creatinine | 0.496 | 15.7 | 100 | 0.026 | |
2-aminobutyric acid | 0.780 | 74.5 | 100 | 0.004 | |
Acetone | 0.764 | 72.5 | 80 | 0.026 | |
TSC-LAM vs. control | Alanine | 0.840 | 90 | 75 | 0.382 |
Methionine | 0.948 | 80 | 100 | 0.011 | |
L6PL | 0.800 | 90 | 75 | 12.550 | |
Acetic acid | 0.975 | 100 | 90 | 0.007 | |
Creatinine | 0.917 | 80 | 95 | 0.059 |
AUC, area under the curve; S, sporadic; TSC, tuberous sclerosis complex; LAM, lymphangioleiomyomatosis.
The calculation formula of the median model was: total value = histidine*(−56.25) + methionine*(−51.27) + 2-aminobutyric acid*(−46) + alanine*(−21.79) + threonine*(−7.20) + isoleucine*(−2.57) + L3TG*0.31 + L6TG*0.84 + H2A2*1.21 + trimethylamine N-oxide*2.46 + glutamic acid*8.78 + creatine*14.90 + ornithine*169.06 + sarcosine*255.47 + dimethyl sulfone*348.10 + N,N-dimethylglycine*469.56 + acetic acid*746.67 + (−8.27).
Selection of indicators with significant difference between S-LAM patients and healthy controls for logistic regression analysis
The 10 differential lipoprotein indexes and 18 differential metabolites obtained by comparing S-LAM patients with healthy controls were modeled by logistic regression. The high-frequency indexes appearing in the modeling were acetic acid, 2-aminobutyric acid, glutamic acid, creatine, V5FC, histidine, methionine, alanine, isoleucine, trimethylamine N-oxide, threonine, and L6TG (Figures 2,3). The indicators in the median model were 2-aminobutyric acid, histidine, isoleucine, alanine, threonine, lysine, L6TG, H2A2, V5FC, trimethylamine N-oxide, creatine, acetone, glutamic acid, ornithine, N,N-dimethylglycine, and acetic acid (Figure 2, Table 3). In the validation cohort, the AUC value of the median model was 0.9852, and the sensitivity and specificity of the model were 100% and 93%, respectively (Figure 4C). In all subjects in this group, ROC curves (Figure 4D) were drawn separately for the high-frequency indicators or indicators that appeared in the median model to distinguish patients from healthy people. Among them, the indicators with higher diagnostic efficacy were methionine (AUC =0.924, sensitivity =72.5%, specificity =100%, cut-off value =0.011 mmol/L) and acetic acid (AUC =0.963, sensitivity =94.1%, specificity =90%, cut-off value =0.006 mmol/L) (Table 4).
The calculation formula of the median model was: total value = methionine*(−72.9901) + alanine*(−1.9661) + HDA2*0.0201 + H4A2*0.0283 + H2A1*0.1263 + L6TG*0.2449 + acetic acid*162.4706 + (−3.6679).
Selection of indicators with significant difference between TSC-LAM patients and healthy controls for logistic regression analysis
The 14 differential lipoprotein indexes and 8 differential metabolites obtained by comparing TSC-LAM patients with healthy controls were modeled by logistic regression. The high-frequency indexes appearing in the modeling were acetic acid, methionine, creatinine, and alanine (Figures 2,3). The indicators in the median model were methionine, alanine, L6PL, creatinine, and acetic acid (Figure 2, Table 3). In the validation cohort, the AUC value of the median model was 1, and the sensitivity and specificity of the model were 100% and 100%, respectively (Figure 4E). In all subjects in this group, ROC curves (Figure 4F) were drawn separately for the high-frequency indicators or indicators that appeared in the median model to distinguish patients from healthy people. Among them, the indicators with higher diagnostic efficacy were methionine (AUC =0.948, sensitivity =80%, specificity =100%, cut-off value =0.011 mmol/L), acetic acid (AUC =0.975, sensitivity =100%, specificity =90%, cut-off value =0.007 mmol/L) and creatinine (AUC =0.917, sensitivity =80%, specificity =95%, cut-off value =0.059 mmol/L) (Table 4).
The calculation formula of the median model was: total value = methionine*(−37.07) + alanine*(−0.79) + L6PL*0.12 + creatinine*32.52 + acetic acid*107.40 + (−3.78).
Correlation analysis between differential metabolites and clinical indicators
Clinical indicators of the 61 LAM patients were collected, including age, lung function (FEV1% predicted, FVC% predicted, FEV1/FVC, DLCO-SB% predicted), pneumothorax, chylothorax, renal AML, liver lesions, chylous ascites, hysteromyoma and retroperitoneal AMLs. The correlation between the clinical indicators and the differential indicators (alanine, methionine, L6TG, acetic acid, L6PL, creatinine, histidine, 2-aminobutyric acid, acetone, sarcosine) with high diagnostic efficiency was analyzed. Methionine was found to be significantly associated with the occurrence of pneumothorax (P<0.05), and creatinine was significantly correlated with hysteromyoma (P<0.05) (Figure 5).
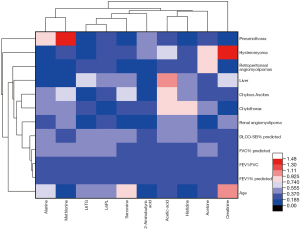
Discussion
This is the first metabonomic analysis of LAM patients in an Asian population using the NMR method. We conducted our study to search for blood biomarkers of LAM and to assess the efficacy of this non-invasive examination. Our study found that S-LAM and TSC-LAM patients, compared with the healthy control group, jointly showed upregulation of part of the sixth component of LDL (L6TG, LDL-6 triglycerides, L6PL LDL-6 phospholipids). We also found abnormal metabolism of some amino acids, such as the downregulation of methionine and alanine and upregulation of acetic acid and creatinine. These indicators were high-frequency indicators or indicators in the median model that appeared together in the three types of models. In addition, creatinine and acetone were found to be significantly different between the S-LAM and TSC-LAM subgroups.
We found that methionine levels were significantly lower in LAM patients than in healthy people. Methionine is an essential amino acid required for the maintenance of cell growth (20) and protein translation (21). Abnormal methionine metabolism (downregulation) had been observed in many tumors, such as glioma and lung cancer (22,23). mTORC1 is central to the ability of cells to adapt to microenvironments, and is a key signaling hub. It can be activated by a growth factor signal (24), and stimulates amino acid uptake and protein synthesis by phosphorylating multiple targets (25). It has been reported that in non-small cell lung cancer cells, methionine deficiency can activate mTORC1 to varying degrees, inhibiting AKT phosphorylation and affecting the proliferation of tumor cells (26). In the pathogenesis of LAM, the activation of mTORC1 and the inactivation of mTORC2 are key factors for the occurrence and progression of LAM. We speculate that the downregulation of methionine may play an important role in the occurrence and progress of LAM. In addition, another study found that methionine deficiency in the diet of mice significantly reduced the level of alveolar surfactant, leading to atelectasis and reversibly affecting lung function, resulting in a decrease in deep inspiratory capacity and compliance (27). The decrease in the rate of extracellular matrix turnover in the alveolar epithelium and pulmonary surfactant synthesis may be involved in the pathogenesis of LAM. Human smokers under the restrictions of methionine limitation were more likely to develop atelectasis and air trapping. The pulmonary imaging features of LAM include multiple cystic changes, which are similar to pulmonary air trapping in smokers from the perspective of pathophysiology. Our study also found a significant decrease in methionine in LAM patients, and in both LAM subtypes (S-LAM and TSC-LAM), suggesting that pulmonary cystic degeneration may be associated with downregulation of methionine. We also found that methionine could effectively distinguish LAM from healthy people, with an AUC value of 0.929 and a cut-off value of 0.011 mmol/L. It is noteworthy that we also found that methionine was significantly related to the occurrence of pneumothorax, indicating that the low level of methionine in patients’ blood is potentially an indicator for early prediction of pneumothorax risk. In the future, in-depth research is needed to confirm this possibility.
Another indicator with high diagnostic efficacy was acetic acid. It was significantly upregulated in LAM patients compared with healthy controls. The AUC value was 0.966 (sensitivity =95.1%, specificity =90%), and the cut-off value was 0.006 mmol/L. A previous study identified that in their metabolomic analysis of breast cancer patients, acetic acid was elevated compared with healthy controls, making it a potential biomarker (28). Acetic acid is significantly increased in lung and liver cancer, which confirms that acetic acid and its metabolites may have a role in promoting tumor growth (29,30). In addition, we also found that alanine was differentially expressed. Alanine levels were significantly lower in the plasma of cancer patients compared with healthy controls (31), most likely due to greater utilization as the main gluconeogenic precursor to meet the high glucose uptake and demand of tumor cells. Pancreatic cancer cells rely on extracellular alanine as the carbon source to promote the tricarboxylic acid cycle in a glutamic-pyruvic transaminase 2 (GPT2)-dependent manner (32). A recent study reestablished the role of alanine in T cell activation (33). GPT2 is responsible for alanine catabolism (34,35), converting alanine to pyruvic acid, which is the main substrate of mitochondrial metabolism. Pyruvic acid could compensate for glutamine consumption by supplementing the tricarboxylic acid cycle, and maintain the cancer anabolic process by utilizing the activities of different enzymes (e.g., pyruvate dehydrogenase complex or pyruvate carboxylase) (36-38). In the present study we found that acetone was significantly higher in S-LAM patients than in healthy controls and could distinguish S-LAM from TSC-LAM. A previous study found higher acetone production by lung cancer cells (39), and Pedersen et al. found that acetone was significantly elevated in the blood of small cell lung cancer patients (40). Ketone bodies are mainly responsible for delivering energy to cells and are involved in regulating various cellular processes (41). Increased ketogenesis in cancer cells may be due to alterations in lipogenesis, gluconeogenesis, and cholesterologenesis, all mechanisms intimately related to ketone body regulation, or due to high competition for primary energy compounds between cancerous and healthy cells (41,42).
Creatinine upregulation was also found in this study. Rouvière et al. reported that 48–80% of TSC patients had kidney disease, accompanied by AML, cysts, cancer and/or progression to renal insufficiency (43). The rate of AML involvement in the TSC-LAM group in our study was as high as 90%, consistent with the result of elevated creatinine. Previous studies have shown renal AMLs in 34–80% of TSC-LAM patients (44,45), with AMLs, cortical cysts, malignant lesions, and/or chronic renal dysfunction. We also found significantly higher creatinine in patients with TSC-LAM compared with healthy controls, reflecting the possibility of renal involvement in TSC-LAM patients. Moreover, this indicator was differentially expressed between S-LAM and TSC-LAM, showing promise as an indicator distinguishing between the two subtypes.
We analyzed the plasma lipid metabolic profile of LAM patients and in the comparison with healthy controls, plasma LDL-6 triglycerides were significantly increased in S-LAM patients, and LDL-6 phospholipids were significantly increased in TSC-LAM patients at baseline. Previous studies had shown that breast cancer was associated with lipid disorders, and that increased levels of LDL in the blood may be related to tumor lipid reprogramming metabolism (46,47), which is in line with our findings. Moreover, we further classified the elevated lipoprotein subgroups by the NMR platform and found that the sixth component of LDL was elevated, which is usually ignored in routine clinical lipid testing. Many studies have found that LDL promotes the proliferation, metastasis and angiogenesis of breast cancer cells (48-50). Bottolo et al. found that sphingolipid, fatty acid, and phospholipid metabolites were associated with disease severity and mTOR inhibition in LAM patients (17). The association between these metabolites and their potential biological roles in cell survival and signaling suggests that lipids may be both disease-relevant biomarkers and potential therapeutic targets for LAM. Taken together, our data are consistent with previous reports in other types of tumors, suggesting that LDL is significantly elevated in LAM patients, but that the AUC of LDL-6 triglycerides and LDL-6 phospholipids is not high. The exact mechanism linking lipoprotein abnormalities and the development of LAM remains unclear and needs to be further explored by more fundamental studies in the future.
Because LAM is a rare disease, the number of subjects included in this study was limited, especially TSC-LAM, which is a rare type of rare disease, so the number of cases was very small. It is hoped in the future that the number of patients as well as multicenter studies can be enlarged to overcome this limitation. In addition, although we found significant differences in some lipoproteins and metabolites between LAM patients and healthy controls, the underlying mechanisms remain unclear. In the future, studies in animal models or cell models could further confirm the related mechanisms, such as metabolic pathways, and make breakthroughs in therapeutic drugs.
Conclusions
In the present study, for the first time, we deeply explored the blood metabolic signatures of LAM patients by NMR. NMR had good discriminatory power for blood samples, thus opening up new possibilities for non-invasive diagnostic methods for LAM. Metabolic disorder is one of the manifestations of LAM. Methionine and acetic acid levels in the plasma of LAM patients could be used as biomarkers for disease diagnosis. Methionine was also found to be associated with pneumothorax in LAM patients. Acetone and creatinine were promising metabolic markers to distinguish S-LAM from TSC-LAM.
Acknowledgments
Funding: This work was supported by the joint funding project of Guangzhou Science and Technology Bureau and University (College) (Basic and Applied Basic Research Project) (project No. 202201020464), and the National Natural Science Foundation of China (grant Nos. 82270089, 82070063, and 81870068).
Footnote
Reporting Checklist: The authors have completed the STARD reporting checklist. Available at https://atm.amegroups.com/article/view/10.21037/atm-22-6420/rc
Data Sharing Statement: Available at https://atm.amegroups.com/article/view/10.21037/atm-22-6420/dss
Conflicts of Interest: All authors have completed the ICMJE uniform disclosure form (available at https://atm.amegroups.com/article/view/10.21037/atm-22-6420/coif). The authors have no conflicts of interest to declare.
Ethical Statement: The authors are accountable for all aspects of the work in ensuring that questions related to the accuracy or integrity of any part of the work are appropriately investigated and resolved. The study was conducted in accordance with the Declaration of Helsinki (as revised in 2013). The study was approved by the Ethics Committee of the First Affiliated Hospital of Guangzhou Medical University (No. 2020-178) and informed consent was taken from all individual participants.
Open Access Statement: This is an Open Access article distributed in accordance with the Creative Commons Attribution-NonCommercial-NoDerivs 4.0 International License (CC BY-NC-ND 4.0), which permits the non-commercial replication and distribution of the article with the strict proviso that no changes or edits are made and the original work is properly cited (including links to both the formal publication through the relevant DOI and the license). See: https://creativecommons.org/licenses/by-nc-nd/4.0/.
References
- McCormack FX, Travis WD, Colby TV, et al. Lymphangioleiomyomatosis: calling it what it is: a low-grade, destructive, metastasizing neoplasm. Am J Respir Crit Care Med 2012;186:1210-2. [Crossref] [PubMed]
- Johnson SR, Cordier JF, Lazor R, et al. European Respiratory Society guidelines for the diagnosis and management of lymphangioleiomyomatosis. Eur Respir J 2010;35:14-26. [Crossref] [PubMed]
- Harknett EC, Chang WY, Byrnes S, et al. Use of variability in national and regional data to estimate the prevalence of lymphangioleiomyomatosis. QJM 2011;104:971-9. [Crossref] [PubMed]
- Northrup H, Krueger DA. Tuberous sclerosis complex diagnostic criteria update: recommendations of the 2012 Iinternational Tuberous Sclerosis Complex Consensus Conference. Pediatr Neurol 2013;49:243-54. [Crossref] [PubMed]
- Cudzilo CJ, Szczesniak RD, Brody AS, et al. Lymphangioleiomyomatosis screening in women with tuberous sclerosis. Chest 2013;144:578-85. [Crossref] [PubMed]
- Krueger DA, Northrup H. Tuberous sclerosis complex surveillance and management: recommendations of the 2012 International Tuberous Sclerosis Complex Consensus Conference. Pediatr Neurol 2013;49:255-65. [Crossref] [PubMed]
- Taveira-DaSilva AM, Jones AM, Julien-Williams P, et al. Long-Term Effect of Sirolimus on Serum Vascular Endothelial Growth Factor D Levels in Patients With Lymphangioleiomyomatosis. Chest 2018;153:124-32. [Crossref] [PubMed]
- Young LR, Inoue Y, McCormack FX. Diagnostic potential of serum VEGF-D for lymphangioleiomyomatosis. N Engl J Med 2008;358:199-200. [Crossref] [PubMed]
- Young LR, Vandyke R, Gulleman PM, et al. Serum vascular endothelial growth factor-D prospectively distinguishes lymphangioleiomyomatosis from other diseases. Chest 2010;138:674-81. [Crossref] [PubMed]
- Banville N, Burgess JK, Jaffar J, et al. A quantitative proteomic approach to identify significantly altered protein networks in the serum of patients with lymphangioleiomyomatosis (LAM). PLoS One 2014;9:e105365. [Crossref] [PubMed]
- Cui H, Cheng C, Xu W, et al. The etiology of diffuse cystic lung diseases: an analysis of 1010 consecutive cases in a LAM clinic. Orphanet J Rare Dis 2021;16:273. [Crossref] [PubMed]
- Carsillo T, Astrinidis A, Henske EP. Mutations in the tuberous sclerosis complex gene TSC2 are a cause of sporadic pulmonary lymphangioleiomyomatosis. Proc Natl Acad Sci U S A 2000;97:6085-90. [Crossref] [PubMed]
- Kwiatkowski DJ, Zhang H, Bandura JL, et al. A mouse model of TSC1 reveals sex-dependent lethality from liver hemangiomas, and up-regulation of p70S6 kinase activity in Tsc1 null cells. Hum Mol Genet 2002;11:525-34. [Crossref] [PubMed]
- El-Hashemite N, Zhang H, Henske EP, et al. Mutation in TSC2 and activation of mammalian target of rapamycin signalling pathway in renal angiomyolipoma. Lancet 2003;361:1348-9. [Crossref] [PubMed]
- Gupta N, Finlay GA, Kotloff RM, et al. Lymphangioleiomyomatosis Diagnosis and Management: High-Resolution Chest Computed Tomography, Transbronchial Lung Biopsy, and Pleural Disease Management. An Official American Thoracic Society/Japanese Respiratory Society Clinical Practice Guideline. Am J Respir Crit Care Med 2017;196:1337-48. [Crossref] [PubMed]
- McCormack FX, Inoue Y, Moss J, et al. Efficacy and safety of sirolimus in lymphangioleiomyomatosis. N Engl J Med 2011;364:1595-606. [Crossref] [PubMed]
- Bottolo L, Miller S, Johnson SR. Sphingolipid, fatty acid and phospholipid metabolites are associated with disease severity and mTOR inhibition in lymphangioleiomyomatosis. Thorax 2020;75:679-88. [Crossref] [PubMed]
- Snaebjornsson MT, Janaki-Raman S, Schulze A. Greasing the Wheels of the Cancer Machine: The Role of Lipid Metabolism in Cancer. Cell Metab 2020;31:62-76. [Crossref] [PubMed]
- Jiménez B, Holmes E, Heude C, et al. Quantitative Lipoprotein Subclass and Low Molecular Weight Metabolite Analysis in Human Serum and Plasma by (1)H NMR Spectroscopy in a Multilaboratory Trial. Anal Chem 2018;90:11962-71. [Crossref] [PubMed]
- Sanderson SM, Gao X, Dai Z, et al. Methionine metabolism in health and cancer: a nexus of diet and precision medicine. Nat Rev Cancer 2019;19:625-37. [Crossref] [PubMed]
- Mazor KM, Dong L, Mao Y, et al. Effects of single amino acid deficiency on mRNA translation are markedly different for methionine versus leucine. Sci Rep 2018;8:8076. [Crossref] [PubMed]
- Chen K, Liu H, Liu Z, et al. Genetic variants in RUNX3, AMD1 and MSRA in the methionine metabolic pathway and survival in nonsmall cell lung cancer patients. Int J Cancer 2019;145:621-31. [Crossref] [PubMed]
- Wang K, Liu H, Liu J, et al. IL1RN mediates the suppressive effect of methionine deprivation on glioma proliferation. Cancer Lett 2019;454:146-57. [Crossref] [PubMed]
- Sancak Y, Bar-Peled L, Zoncu R, et al. Ragulator-Rag complex targets mTORC1 to the lysosomal surface and is necessary for its activation by amino acids. Cell 2010;141:290-303. [Crossref] [PubMed]
- Ma XM, Blenis J. Molecular mechanisms of mTOR-mediated translational control. Nat Rev Mol Cell Biol 2009;10:307-18. [Crossref] [PubMed]
- Jin HO, Hong SE, Kim JY, et al. Amino acid deprivation induces AKT activation by inducing GCN2/ATF4/REDD1 axis. Cell Death Dis 2021;12:1127. [Crossref] [PubMed]
- Jubinville É, Milad N, Maranda-Robitaille M, et al. Critical importance of dietary methionine and choline in the maintenance of lung homeostasis during normal and cigarette smoke exposure conditions. Am J Physiol Lung Cell Mol Physiol 2020;319:L391-402. [Crossref] [PubMed]
- Silva C, Perestrelo R, Silva P, et al. Volatomic pattern of breast cancer and cancer-free tissues as a powerful strategy to identify potential biomarkers. Analyst 2019;144:4153-61. [Crossref] [PubMed]
- Ho CL, Yu SC, Yeung DW. 11C-acetate PET imaging in hepatocellular carcinoma and other liver masses. J Nucl Med 2003;44:213-21. [PubMed]
- Nomori H, Shibata H, Uno K, et al. 11C-Acetate can be used in place of 18F-fluorodeoxyglucose for positron emission tomography imaging of non-small cell lung cancer with higher sensitivity for well-differentiated adenocarcinoma. J Thorac Oncol 2008;3:1427-32. [Crossref] [PubMed]
- Lai HS, Lee JC, Lee PH, et al. Plasma free amino acid profile in cancer patients. Semin Cancer Biol 2005;15:267-76. [Crossref] [PubMed]
- Sousa CM, Biancur DE, Wang X, et al. Pancreatic stellate cells support tumour metabolism through autophagic alanine secretion. Nature 2016;536:479-83. [Crossref] [PubMed]
- Ron-Harel N, Ghergurovich JM, Notarangelo G, et al. T Cell Activation Depends on Extracellular Alanine. Cell Rep 2019;28:3011-3021.e4. [Crossref] [PubMed]
- Hodakoski C, Hopkins BD, Zhang G, et al. Rac-Mediated Macropinocytosis of Extracellular Protein Promotes Glucose Independence in Non-Small Cell Lung Cancer. Cancers (Basel) 2019;11:37. [Crossref] [PubMed]
- Kim M, Gwak J, Hwang S, et al. Mitochondrial GPT2 plays a pivotal role in metabolic adaptation to the perturbation of mitochondrial glutamine metabolism. Oncogene 2019;38:4729-38. [Crossref] [PubMed]
- Sellers K, Fox MP, Bousamra M 2nd, et al. Pyruvate carboxylase is critical for non-small-cell lung cancer proliferation. J Clin Invest 2015;125:687-98. [Crossref] [PubMed]
- Woolbright BL, Rajendran G, Harris RA, et al. Metabolic Flexibility in Cancer: Targeting the Pyruvate Dehydrogenase Kinase:Pyruvate Dehydrogenase Axis. Mol Cancer Ther 2019;18:1673-81. [Crossref] [PubMed]
- Cheng T, Sudderth J, Yang C, et al. Pyruvate carboxylase is required for glutamine-independent growth of tumor cells. Proc Natl Acad Sci U S A 2011;108:8674-9. [Crossref] [PubMed]
- Ruzsányi V, Péter Kalapos M. Breath acetone as a potential marker in clinical practice. J Breath Res 2017;11:024002. [Crossref] [PubMed]
- Pedersen S, Hansen JB, Maltesen RG, et al. Identifying metabolic alterations in newly diagnosed small cell lung cancer patients. Metabol Open 2021;12:100127. [Crossref] [PubMed]
- Feng S, Wang H, Liu J, et al. Multi-dimensional roles of ketone bodies in cancer biology: Opportunities for cancer therapy. Pharmacol Res 2019;150:104500. [Crossref] [PubMed]
- Poff AM, Ari C, Arnold P, et al. Ketone supplementation decreases tumor cell viability and prolongs survival of mice with metastatic cancer. Int J Cancer 2014;135:1711-20. [Crossref] [PubMed]
- Rouvière O, Nivet H, Grenier N, et al. Kidney damage due to tuberous sclerosis complex: management recommendations. Diagn Interv Imaging 2013;94:225-37. [Crossref] [PubMed]
- O'Callaghan FJ, Noakes MJ, Martyn CN, et al. An epidemiological study of renal pathology in tuberous sclerosis complex. BJU Int 2004;94:853-7. [Crossref] [PubMed]
- Rakowski SK, Winterkorn EB, Paul E, et al. Renal manifestations of tuberous sclerosis complex: Incidence, prognosis, and predictive factors. Kidney Int 2006;70:1777-82. [Crossref] [PubMed]
- Chang SJ, Hou MF, Tsai SM, et al. The association between lipid profiles and breast cancer among Taiwanese women. Clin Chem Lab Med 2007;45:1219-23. [Crossref] [PubMed]
- Chen Z, Chen L, Sun B, et al. LDLR inhibition promotes hepatocellular carcinoma proliferation and metastasis by elevating intracellular cholesterol synthesis through the MEK/ERK signaling pathway. Mol Metab 2021;51:101230. [Crossref] [PubMed]
- Lu CW, Lo YH, Chen CH, et al. VLDL and LDL, but not HDL, promote breast cancer cell proliferation, metastasis and angiogenesis. Cancer Lett 2017;388:130-8. [Crossref] [PubMed]
- Antalis CJ, Arnold T, Rasool T, et al. High ACAT1 expression in estrogen receptor negative basal-like breast cancer cells is associated with LDL-induced proliferation. Breast Cancer Res Treat 2010;122:661-70. [Crossref] [PubMed]
- dos Santos CR, Domingues G, Matias I, et al. LDL-cholesterol signaling induces breast cancer proliferation and invasion. Lipids Health Dis 2014;13:16. [Crossref] [PubMed]
(English Language Editor: K. Brown)