Network and pathway-based analysis of genes associated with esophageal squamous cell carcinoma
Highlight box
Key findings
• At the molecular functional level, genes related to chemical response, response to stimuli, and cell proliferation were significantly enriched in ESCC.
• Pathway crosstalk analysis showed that pathways related to multiple malignancies, immune system, and metabolic processes were enriched among ESCC genes.
What is known and what is new?
• The 321 extracted genes, of which aberrant expression mediates biological processes such as immunometabolism, apoptosis, and cell proliferation, are involved in the development and progression of ESCC.
• By ESCC-specific network analysis, we discovered 39 new genes, most of which have never been linked to ESCC. The genes MUC13, RYK, and FIN may be potential prognostic indicators of ESCC. GRB2 and CDC25C may be potential targets of ESCC in proliferation.
What is the implication, and what should change now?
• The molecular mechanisms underlying the development and progression of ESCC require more extensive and in-depth studies.
Introduction
Esophageal squamous cell carcinoma (ESCC), originating from the esophageal mucosa or gland, is malignant and aggressive with a poor prognosis, and accounts for 90% of all cases of esophageal cancer globally. In some eastern Asian and African countries, the incidence of ESCC is extremely high (1,2). An extensive body of research has demonstrated that alcohol and smoking are major risk factors for ESCC. Meanwhile, environmental factors such as the intake of hot beverages, nutritional deficiencies, and limited intake of fruits and vegetables also play a role in the development of this cancer (3,4).
ESCC is clinically challenging and requires multidisciplinary approaches to diagnosis and treatment. The early detection of ESCC is difficult, and most patients are diagnosed with advanced ESCC when major symptoms such as progressive dysphagia and pain behind the sternum occur. Most patients with ESCC require extensive treatment, including chemotherapy, chemoradiotherapy, and/or surgical resection. Palliative treatments are adopted by patients with advanced or metastatic ESCC. However, the limited clinical approaches for the early diagnosis and treatment of ESCC lead to a 10% 5-year survival rate for patients (1,5,6).
Currently, countless genomic analyses have increased the notoriety of these 6 ESCC-implicated genes (TP53, RB1, CDKN2A, PIK3CA, NOTCH1, NFE2L2). TP53 Pro72 allele increases the risk of ESCC. In previous research, CDKN2A/RB1 was not expressed in the esophageal mucosa of patients without risk factors whereas p16/pRb expression increased in patients exposed to risk factors or with ESCC (7,8). Although numerous single genetic studies and genetic pathway studies have provided many important insights of the development and prognosis of ESCC, they have not provided clues from the perspective of systems biology. In this study, we conducted a comprehensive collection of ESCC-associated genes from the current studies on ESCC genetic association. Then, we detected the significant biological themes in these genetic factors by performing functional enrichment analyses. Moreover, to ulteriorly reveal the mechanisms of ESCC in a more effective manner, we analyzed the interactions between these biochemical pathways through pathway crosstalk and examined the topological characteristics of these ESCC-associated genes based on human protein-protein interaction (PPI) network. Besides, the ESCC-specific molecular network was deduced and evaluated using the Steiner minimal tree algorithm. This study should provide clues and directions for understanding the molecular mechanism of ESCC from the perspective of systems biology. We present the following article in accordance with the STREGA reporting checklist (available at https://atm.amegroups.com/article/view/10.21037/atm-22-6512/rc).
Methods
Identification of ESCC-related genes
Candidate genes related to ESCC (the standardized term found through medical subject headings [MeSH]) were curated by retrieving the genetic association studies in the PubMed database. We conducted literature retrieval related to ESCC with the terms (Esophageal Squamous Cell Carcinoma AND polymorphism) OR (Esophageal Squamous Cell Carcinoma AND genotype) OR (Esophageal Squamous Cell Carcinoma AND alleles). By 15 July 2022, a total of 1,131 publications were retrieved. After reviewing all 1,131 publications, only the genetic association studies were selected. From the selected publications, we narrowed our selection by focusing on those that reported 1 or more genes significantly associated with ESCC. In addition, the associated genes from several genome-wide association studies (GWAS), showing genetic association at a genome-wide significance level, were selected. To reduce the number of false-positive findings, we excluded studies that included negative or unrelated associations. We carefully read the full reports of selected studies to ensure that the conclusion was consistent with the content. In this way, we eventually screened out 321 genes from 736 articles. The study was conducted in accordance with the Declaration of Helsinki (as revised in 2013).
Functional enrichment analysis of ESCC-related genes
Applying the software the Database for Annotation, Visualization and Integrated Discovery 6.8 (DAVID 6.8; https://david.ncifcrf.gov/), we converted the names of 321 ESCC-related genes obtained from the literature into Entrez Gene IDs. To investigate the functional features of ESCC-related genes, Gene Ontology (GO) term analysis and pathway analysis were applied for functional enrichment analysis. The GO resource (http://www.geneontology.org/) is an international standard classification system of gene function. We performed gene enrichment analysis using GOseq in R Studio 3.4.2 (R Foundation for Statistical Computing, Vienna, Austria). Based on the Wallenius non-central hyper-geometric distribution, this method allows the estimation of gene length preferences and allows us to accurately calculate the probability of a GO term being enriched by the host gene. The enriched P values were corrected by the Benjamini-Hochberg (BH), procedure and we retained terms with P values less than 0.05 as significantly enriched terms. We used directed acyclic graphs (DAGs) as a graphical representation of the GO enrichment analysis of protein genes.
Kyoto Encyclopedia of Genes and Genomes (KEGG) pathway enrichment analysis
Upon analyzing the entirety of the KEGG database, the pathways in which the study genes were involved were identified and a network of these pathways was established with the aid of the KEGG database’s pathway topology. A gene network specific to a single pathway was created through pathway topology analysis. This network was then mapped to the reference gene network from KEGG, resulting in the study gene network. The Entrez Genes IDs were uploaded into the software KOBAS 2.1.1 (http://kobas.cbi.pku.edu.cn/) and were compared with the genes included in each canonical pathway based on the KEGG pathway database. Then, we obtained the corresponding KEGG pathway and P values for each pathway. KEGG pathway analysis was performed using tools in KOBAS, corrected P value [false discovery rate (FDR)] obtained by Fisher’s exact test, which were based on hypergeometric distribution, and the enriched P values were corrected by the BH method and a threshold of FDR <0.05 was used to select significantly enriched pathways.
Pathway crosstalk analysis
To further observe the interconnections and interactions between pathways, we conducted a pathway crosstalk analysis of the above-mentioned pathways of significant enrichment. Herein, to test for the overlap between any pair of given pathways, we imported two measurements: the and the , where A and B are the lists of genes included in the two tested pathways. To construct the pathway crosstalk, we implemented the following procedure:
- Select the pathways with PBH ≤0.05. Meanwhile, the number of candidate genes contained in each pathway was restricted to be bigger than or equal to 3, because pathways with too few candidate genes may insufficiently reflect the biological information.
- Calculate the number of candidate genes that overlap between any pair of pathways. Delete the pathway pair with less than 3 overlapping genes.
- Calculate the overlap of all the pathway pairs that meet the above conditions and rank them by
.
- Visualize the selected pathway crosstalk with the software Cytoscape 3.5.1 (9). Indicate the degree of pathways by the size of node, whereby the bigger the degree, the greater the size of the node. Use the thickness of the edge to indicate the score between the pathway pairs, whereby the rougher the edge, the higher the score.
Subnetwork extraction
We merged human protein interaction data downloaded from the Protein Interaction Network Analysis (PINA) platform (updated 21 May 2014) and protein interaction data reported in the recent literature. After removing redundant relationship and self-paired relationship we obtained the final proteins and relationship pairs (10). With these network relationships as a background, we applied Klein-Ravi algorithm in GenRev, a network-based software package to explore functional relevance of genes via the Steiner minimal tree algorithm that uses a greedy search strategy to merge the smaller trees into larger ones until only one tree connecting all input seeds is built, to extract a subnetwork from the human PPI network by using the 321 ESCC-related genes as seeds (11). In order to verify that this subnetwork was a non-random network, we used Erdos-Renyi algorithm in the igraph R package to generate 100 random background networks with the same number of nodes and edges, and combined the seed gene with the 100 random background networks to generate subnetworks by analyses. Then, we calculated the average values of the shortest-path distance and clustering coefficient. We calculated the number of the average shortest path in the random subnetwork that was shorter than the ESCC networks, denoted the number as nL; calculated the number of the average clustering coefficient in random subnetwork that was higher than the ESCC network, denoted the number as nC; calculated the values of pL and .
Results
Identification of Genes Reported to be Associated with ESCC
By searching PubMed, we only selected genetic association studies and related genes from several GWAS, which showed a significant degree of genome-wide genetic association. We excluded studies that included either negative or unrelated outcomes to reduce the number of false-positive results.
Altogether, we screened 321 genes reported to be significantly related with ESCC out of 736 articles, which had a range of diverse biological functions. For example, some genes were related to tumor necrosis factor (TNF) signaling (e.g., MMP9, CASP3, MMP3, CASP8, AKT1, FADD, IL6, LTA, PIK3CA, PIK3CB, ITCH, FAS, PTGS2, EDN1, IL1B, TNF, TNFRSF1A, IL18R1, and NFKBIA), and some genes were related to nucleotide excision repair (e.g., GTF2H3, ERCC2, XPA, ERCC5, ERCC4, XPC, and ERCC1), and some genes were related to choline metabolism in cancer (e.g., mTOR, Akt1, PIK3CA, RHEB, EGFR, EGF, CHKA, PIK3CB, and WASL). A detailed list of all genes we found to be associated with ESCC is provided in Table S1.
GO enrichment analysis in ESCC-related genes
The GO database provides a standardized description of the gene products from the function, the participating biological pathway, and cellular localization, which comprises the simple annotation of the gene products. Through GO enrichment analysis, we can gather a rough understanding of the biological functions, pathways, or cellular localization of the differential genes. DAGs of biological process (BP; Figure 1A), cellular component (CC; Figure 1B), and molecular function (MF; Figure 1C) were used to show the GO annotation results. In the GO DAG, annotation moved from more general to more specific as one moved from parent nodes to child nodes. Consequently, a DAG approach was used to provide a clearer understanding of which GO terms were specifically enriched and how these affected other GO terms through upper hierarchies. The top 10 terms with the lowest P value and their parent terms were shown in a GO DAG, the terms with pale marks were significantly enriched, and those with deeper red marks were more significantly enriched. In the DAG of BP, significant enrichment terms, namely, response to chemical (PBH=5.28E-47), cellular response to stimulus (PBH=1.56E-43), and cell proliferation (PBH=1.42E-42) were identified. An example of a significantly enriched term in the DAG of CC is the GO term “intracellular organelle part”. This GO term was enriched at a very low FDR (1.46E-08), and two GO terms at upper hierarchies: “nuclear part” and “nuclear lumen” were enriched as a result. These two GO terms together enriched the term “nucleoplasm.” The results were consistent with the pathophysiological background of ESCC, indicating that candidate genes were relatively reliable for subsequent bioinformatics analysis. In addition, we used a dot plot to visualize the P values enriched in the first 10 terms in BP, CC, and MF as well as the number of genes involved in each term. At the same time, intuitively, we also found that the genes previously found are in the GO term, which more distinctly showed the correlation. For example, in BP’s dot plot (Figure 2A), the term “response to stimulus” contained the largest number of genes but had the lowest rich factor. In CC’s dot plot (Figure 2B), the term “organelle” contained many genes and its p-value was minimal. In the MF’s dot plot (Figure 2C), we found that the term “damaged DNA binding” had the highest rich factor. Combining all dot plots, we found that there was a trend that the more genes ascribed to a term, the lower the rich factor. This may be because those terms had too many gene numbers. Moreover, we used the ggplot2 package in the Goseq software to graph the top 10 GO terms in BP, CC, and MF (Figure 3).
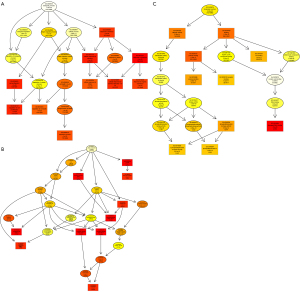
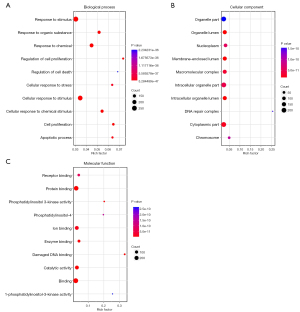
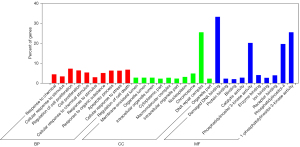
KEGG pathway enrichment analysis in ESCC-related genes
Pathway analysis enables researchers to map the genes, proteins, or molecules onto a particular class of metabolic or regulatory networks, or according to an individuals’ molecular set of functions, in order to form their own specific pathway. This is very important for elucidating the molecular mechanism of action and identifying biomarkers. We uploaded the differential genes into KOBAS 2.1.1 software and compared them to the genes contained in the canonical approaches based on the KEGG pathway database, and we enriched 240 pathways. A total of 31 pathways with corrected P value (FDR) <0.05 were retained as the significantly enriched pathways (Table S2). Most of the pathways were related to pathophysiological process of cancer. Among them, several pathways are closely related to certain types of malignant tumors, including bladder cancer (ranked 2nd), melanoma (ranked 4th), prostate cancer (ranked 5th), colorectal cancer (ranked 9th), non-small cell lung cancer (NSCLC; ranked 11th), endometrial cancer (ranked 14th), and glioma (ranked 20th). Many studies have clarified the role of p53 and TNF in the development of cancer. p53 is a well-known tumor suppressor gene. However, in ESCC, the p53 pathway is inactivated by TP53 mutations, so it has carcinogenic effects (12-14). Numerous studies have shown that TNF has antitumor activity and is also an endogenous tumor promoter (15). TNF is down-regulated in ESCC tissues and multivariate analysis has shown that down-regulation of TNF is independently associated with early local tumor recurrence (16,17). In cellular metabolism, we obtained pathways such as proteoglycans in cancer, endocrine resistance, HIF-1 signaling pathway, and microRNAs in cancer. It was worth mentioning that in the microRNAs in cancer pathway, MYC binding protein (MYCBP) was identified as a direct target of miR-26 (18). In drug metabolism, we found pathways such as platinum drug resistance (ranked 1st), metabolism of xenobiotics by cytochrome P450, and drug metabolism-cytochrome P450. Cisplatin is an important part of chemotherapy for esophageal cancer (19). However, in the use of platinum drugs, some challenges such as patients’ partial antitumor response, drug resistance development, and tumor recurrence limit the patient’s life expectancy (20). Furthermore, immune-associated biological processes such as graft-versus-host disease and allograft rejection were also significantly enriched.
Pathway crosstalk analysis among significantly enriched pathways
To further observe the interconnections and interactions between significantly enriched pathways, we conducted a pathway crosstalk analysis of the 31 significantly enriched pathways mentioned above. The approach was based on the assumption that two pathways were considered to crosstalk if they shared a proportion of ESCC-related genes (21). A total of 30 of the above 31 significantly enriched pathways were in accordance with the crosstalk analysis criteria, namely, each pathway contained 3 or more genes, each of which had at least two genes sharing with 1 or more other pathways. The base excision repair pathway was the only non-compliant pathway due to the number of genes overlapping with other pathways being less than or equal to 2. All paths formed by these approaches were used to construct pathway crosstalk and the level of overlap between the two pathways was measured on the basis of the average scores of the coefficients JC and OC. Based on their crosstalk, pathways could be broadly divided into three main parts, each containing more interactions than other pathways and might involve in the same or similar biological processes (Figure 4). The first part consisted of 16 pathways, including 9 of the top 10 significantly enriched pathways of KEGG. Most of these 16 pathways were specific cancer pathways, such as prostate cancer and NSCLC. Three of these pathways shared some genes with the platinum drug resistance pathway, and these three pathways were linked to drug metabolism and chemical carcinogenesis. The second part contained seven pathways, which connected the other two parts of pathways that shared no genes. These seven pathways were mainly involved in the regulation of many kinds of receptors and cytokines in cells. At the same time, they played an important role in the regulation of cell proliferation, apoptosis, inflammation in vivo, infection, and other physiological processes. The third pathway was mainly associated with immunity and inflammation. As indicated above, path crosstalk analysis can provide important clues to the development and prognosis of ESCC and provide insights into the ECSS mechanism.
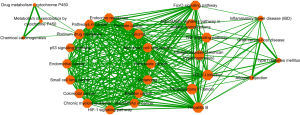
Subnetwork extraction
To refine the ESCC-related interactions, we used the Steiner’s minimal tree algorithm to extract the ESCC subnetwork from the human PPI network and recently published protein interaction pairs (232,866 pairs of 17,379 proteins were involved). This approach attempts to connect a maximum number of input nodes with a minimum number of linked nodes (we used 308 nodes). As shown in Figure 5, 347 nodes and 1,339 edges were contained. A total of 308 out of the 321 ESCC-related genes were included in the ESCC-specific network, accounting for about 95.95% of the candidate genes and 88.76% of the genes in the ESCC-specific network, demonstrating a high coverage of ESCC-related genes in the subnetwork. It was noteworthy that we found 39 additional proteins, some of which had been reported as associated with ESCC (Table 1). In addition, to verify that the sub-network was a non-random network, we used the Erdos-Renyi model in the igraph R package to generate 100 random sub-networks. We calculated the arithmetic average values of the shortest path distance and clustering coefficients in the random sub-networks. The average shortest path distance value and average clustering coefficient were compared with the corresponding values of the ESCC-specific network. For these random background networks, the average shortest path distance was 2.86, bigger than the shortest path distance of ESCC specific network (shortest path distance was 2.60; PL<0.01). The average clustering coefficient of random sub-networks was 0.19, which was significantly smaller than that of ESCC-specific networks (clustering coefficient was 0.33, PC<0.01). Therefore, the ESCC-specific network extracted from the entire PPI network was a non-random network.
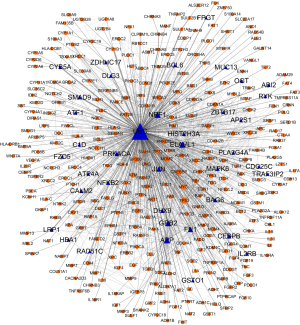
Table 1
Gene abbreviations | Gene ID | Species | Gene name |
---|---|---|---|
RAD51C | 5889 | Homo sapiens | RAD51 paralog C (RAD51C) |
GRB2 | 2885 | Homo sapiens | Growth factor receptor bound protein 2 (GRB2) |
AP2S1 | 1175 | Homo sapiens | Adaptor related protein complex 2 sigma 1 subunit (AP2S1) |
ABI2 | 10152 | Homo sapiens | Abl interactor 2 (ABI2) |
NFKB2 | 4791 | Homo sapiens | Nuclear factor kappa B subunit 2 (NFKB2) |
ZBTB17 | 7709 | Homo sapiens | Zinc finger and BTB domain containing 17 (ZBTB17) |
ATF1 | 466 | Homo sapiens | Activating transcription factor 1 (ATF1) |
APP | 351 | Homo sapiens | Amyloid beta precursor protein (APP) |
BAG6 | 7917 | Homo sapiens | BCL2 associated athanogene 6 (BAG6) |
DLG3 | 1741 | Homo sapiens | Discs large MAGUK scaffold protein 3 (DLG3) |
BCL6 | 604 | Homo sapiens | B-cell CLL/lymphoma 6 (BCL6) |
PRKACA | 5566 | Homo sapiens | Protein kinase camp-activated catalytic subunit alpha (PRKACA) |
OGT | 8473 | Homo sapiens | O-linked N-acetylglucosamine (glcnac) transferase (OGT) |
GSTO1 | 9446 | Homo sapiens | Glutathione S-transferase omega 1 (GSTO1) |
MUC13 | 56667 | Homo sapiens | Mucin 13, cell surface associated (MUC13) |
FN1 | 2335 | Homo sapiens | Fibronectin 1 (FN1) |
HIST2H3A | 333932 | Homo sapiens | Histone cluster 2 H3 family member a (HIST2H3A) |
DHX9 | 1660 | Homo sapiens | Dexh-box helicase 9 (DHX9) |
IL2RB | 3560 | Homo sapiens | Interleukin 2 receptor subunit beta (IL2RB) |
CEBPB | 1051 | Homo sapiens | CCAAT/enhancer binding protein beta (CEBPB) |
SMAD9 | 4093 | Homo sapiens | SMAD family member 9 (SMAD9) |
ATP4A | 495 | Homo sapiens | ATPase H+/K+ transporting alpha subunit (ATP4A) |
RYK | 6259 | Homo sapiens | Receptor-like tyrosine kinase (RYK) |
ELAVL1 | 1994 | Homo sapiens | ELAV like RNA binding protein 1 (ELAVL1) |
CYB5A | 1528 | Homo sapiens | Cytochrome b5 type A (CYB5A) |
HBA1 | 3039 | Homo sapiens | Hemoglobin subunit alpha 1 (HBA1) |
FZD5 | 7855 | Homo sapiens | Frizzled class receptor 5 (FZD5) |
CDC25C | 995 | Homo sapiens | Cell division cycle 25C (CDC25C) |
TRAF3IP2 | 10758 | Homo sapiens | TRAF3 interacting protein 2 (TRAF3IP2) |
NRF1 | 4899 | Homo sapiens | Nuclear respiratory factor 1 (NRF1) |
PLA2G4A | 5321 | Homo sapiens | Phospholipase A2 group IVA (PLA2G4A) |
ZDHHC17 | 23390 | Homo sapiens | Zinc finger DHHC-type containing 17 (ZDHHC17) |
LRP1 | 4035 | Homo sapiens | LDL receptor related protein 1 (LRP1) |
MAPK6 | 5597 | Homo sapiens | Mitogen-activated protein kinase 6 (MAPK6) |
JUN | 3725 | Homo sapiens | Jun proto-oncogene, AP-1 transcription factor subunit (JUN) |
UBC | 7316 | Homo sapiens | Ubiquitin C (UBC) |
FPGT | 8790 | Homo sapiens | Fucose-1-phosphate guanylyltransferase (FPGT) |
CALM2 | 805 | Homo sapiens | Calmodulin 2 (CALM2) |
C1D | 10438 | Homo sapiens | C1D nuclear receptor corepressor (C1D) |
ESCC, esophageal squamous cell carcinoma.
Discussion
As a highly malignant and aggressive tumor, ESCC is not sensitive to radiotherapy and chemotherapy. In the past few decades, studies of human participants, animals, or cell models have gained insight into the molecular mechanisms of ESCC. Although increasing numbers of genes/proteins are believed to be associated with this disease, with the development of high-throughput technologies, many genes/proteins are considered potential targets for detection or treatment. However, the understanding of the biological processes associated with the pathogenesis of ESCC at the molecular level is far from complete. Therefore, there is a need to decode the underlying pathogenesis of ESCC at the level of systems biology. In this study, we explored the interactions of these genes by collecting genes related to ESCC as well as utilizing pathway and network analysis systems. We have provided a comprehensive and systematic framework for mapping related biochemical processes.
Through biological function enrichment analysis, we obtained specific biological processes caused by ESCC-related genes. The GO enrichment analysis provided us with a rough interpretation of the biological functions, pathways, or cellular localization of the differential genes, convincingly demonstrating that these genes involved in immune response, metabolic regulation, cell proliferation, apoptosis, and drug response. In cluster analysis, GO terms relative to apoptosis, cell metabolism, regulation of cell development, DNA damage repair, angiogenesis, and xenobiotic metabolism turned out high enrichment scores, which was consistent with our perception of ESCC so far. For example, in GO MF, key terms such as catalytic activity, binding, protein binding, and damaged DNA binding, along with other enriched terms delineated that the cancer cell has an increased damage repair function and can fully repair the DNA damage caused by chemotherapeutic drugs, which at least partially provides a reason for the unsatisfactory effect of chemotherapy in the treatment of ESCC.
The results of pathway analysis showed that genes related to esophageal cancer contain multiple tumor pathways, revealing its commonality with other malignancies. We found that 7 pathways: bladder cancer, prostate cancer, melanoma, NSCLC, endometrial cancer, glioma, and pancreatic cancer, were in the same functional annotation clustering, and these pathways all contain the genes TP53, RB1, EGFR, EGF, and CCND1, which was highly consistent with our understanding of the mechanisms of cancer development. TP53 encodes a tumor suppressor protein containing transcriptional activation, DNA binding, and oligomerization domains. The encoded protein responds to diverse cellular stresses to regulate expression of target genes, thereby inducing cell cycle arrest, apoptosis, senescence, DNA repair, or changes in metabolism. In ESCC, over 90% of TP53 mutations and inactivation of the p53 pathway are associated with patient prognosis and resistance to radiotherapy and chemotherapy. CCND1 mutations exceeding 20%, deletions of CDKN2A, RB1, and CDKN2A, and abnormal methylation impair the cell cycle. EP300, CREBBP, and NOTCH (NOTCH1, NOTCH2, NOTCH3) are associated with epigenetic processes (12,22,23). Moreover, CCND1, EGFR, and ERBB2 are likely to be driver genes for the development of ESCC (24,25). Previous studies have shown that RASSF1 is a very promising tumor suppressor gene, and the extent of its transcript expression and methylation is related to tumor progression and survival of ESCC patients (26,27). In the RAS signaling pathway and the RAP1 signaling pathway, RASSF1 interacts with and influences PIK3CA, AKT1, EGF, and EGFR. These genes are involved in the formation, proliferation, migration, and angiogenesis of tumors. AKT1 is significantly associated with local recurrence of ESCC (28). Tumor inhibitors of the RASSF family act as RAS apoptosis and senescence effectors. It is speculated that inactivation of the RASSFlA tumor suppressor promotes K-RAS-mediated transformation by uncoupling it with apoptotic pathways such as the Hippo pathway (29,30). In addition, we observed that in the cell cycle, proliferation, the PI3K-AKT signaling pathway, allograft rejection reaction, MYC, COX-2, p53, and RB1 also showed associations. As indicated by these results, a variety of genes and signaling molecules serve as bridges and connect to each other, join to many signaling pathways and form a complex network of ESCC molecules.
In crosstalk analysis, we identified three major modules. The first module contains 16 pathways, most of which are specific cancer pathways, such as prostate cancer, NSCLC, pancreatic cancer, and the like. Among these 16 pathways, 3 share some genes with the platinum drug resistance pathway. These three pathways are related to drug metabolism and chemical carcinogenesis: drug metabolism—cytochrome P450, metabolism of xenobiotics by cytochrome P450, and chemical carcinogenesis. The CYP1B1 mutation was significantly associated with ESCC risk (31,32). Studies have shown that the most important issue associated with ESCC treatment is the intrinsic resistance of chemotherapeutic drugs, and multidrug resistance is increasingly common in patients with ESCC (33,34). Apoptosis is one of the most critical processes in cell proliferation and a key molecular mechanism for anticancer therapy. The PI3K/Akt/mTOR pathway is involved in cell proliferation, differentiation, survival, apoptosis, and metastasis (35-37). Sensitized drugs promote the action of oxaliplatin by significantly inducing apoptosis and modulating the PI3K/Akt/mTOR pathway, counteracting resistance to chemotherapy (38,39). The second module contains 7 pathways which connect other pathways that do not share genes. These 7 pathways are mainly involved in the regulation of various receptors and cytokines in cells, and they also play an important role in the regulation of cell proliferation, apoptosis, inflammation, infection, and other physiological processes. By analysis of module II and module III, pathways related to immune response, cell adhesion (i.e., HTLV-I infection), allograft rejection, graft-versus-host disease, the FoxO signaling pathway, shared genes such as IL6, FAS, END1, IL1B, TNF, TNFRSF1A, NFKBIA, HLA-A, HLA-B, and HLA-C. In these three pathways (graft-versus-host disease, type I diabetes mellitus, and allograft rejection), we found similar processes and common genes such as the HLA family (HLA-A, HLA-B, HLA-C, HAL-G, HLA- DQB1, HLA-DRB1), FAS, FASLG, and some cytokines (IL6, IL1B). By targeting the FASLG gene, miR-21 down-regulates cell growth, invades, and induces apoptosis, and by combining with FASLG treatment methods, the efficacy of radiotherapy can be enhanced, and unnecessary angiogenesis-promoting effects can be reduced (40-42). By modulating HLA-G expression, miR-148a is indicated to be involved in the carcinogenesis of primary ESCC. The current results indicate that miR-148a is a potential biomarker for ESCC (43). HLA class I is critical for tumor immunity, and its degree of expression is an independent prognostic factor for relapse-free survival (44,45). Studies have shown that HLA-G is highly expressed in ESCC and can be used as a novel tumor marker (43,46-48). HLA-II molecules are mainly encoded by DP, DQ, and DR genes, expressed in immune cells, and are responsible for presenting antigenic peptides to CD4+ T cells to trigger the expansion and differentiation of these T cells and induce a series of antigen-specific immunity responses. Studies have shown that aberrant methylation of HLA-DQB1 and HLA-DAB1 at different sites is significantly associated with ESCC differentiation and late stages, and their methylation is conducive to the abnormal expression of HLA-II (49-51). Therefore, abnormal expression of HLA-II may lead to immune response or autoimmunity deficiency in some diseases. Their abnormal expression inhibits the function of immune cells such as natural killer (NK) cells and T cells, which promotes the development of ESCC. These pathways related to the immune response and cell adhesion movement profoundly influence the occurrence and development of ESCC, and they also shed a new light for understanding diseases through molecular mechanisms.
Remarkably, in the ESCC-specific network, we discovered 39 new genes, most of which have never been linked to ESCC, such as APP, TRAF3IP2, ZBTB17, NRF1, CEBPB, HBA1, ABI2, CALM2, RAD51C, and AP2S1 (Table 1). Studies have shown that RYK may be an important predictor of ESCC clinical stage staging and prognosis (52). The combination of MUC13/MUC20 expression is a potential prognostic indicator of neoadjuvant chemotherapy and postoperative ESCC (53,54). GSTO1 may also be a potential biomarker for early detection (55). The drug resistance/response mechanism of ESCC is very complicated. A study found that differential genes are also key cancer-promoting genes related to chemotherapy resistance through PPI, such as BMP1 and DBF4 (56); prognosis-related genes, such as TTK and KIF18A. These studies can help improve the survival rate of patients (57).
JUN is a chromosome region of human malignancy translocations and deletions associated with various signal transduction pathways (58,59). In ESCC, BCL6 is up-regulated, and its acylation is controlled by HDAC and SIRT-dependent mechanisms (60). Interference with this process may lead to cell cycle arrest and apoptosis. Its biological function may indicate that it can become a new regulator in esophageal cancer cells (61,62). GRB2 is a prominent node involved in the naturally regulated cell proliferation-related pathways of the tyrosine kinase signaling pathway and Erk1/Erk2 Mapk signaling pathway. UBC participates in cell cycle progression and is upregulated in more than 70% of ESCC tissues (63-65). UEB2C directly interferes with the level of cyclin B1 protein and alters the proliferation and cell cycle characteristics of ESCC (66). Due to activation of the p53/p21 pathway, CDC25C is thus reduced, leading to cell cycle arrest (67,68). Although the quantity and quality of PPI data have greatly improved in recent years, the human PPI network is far from being completed. In addition, due to current technology limitations, there may be some false positive results in the PPI data. These potential deviations associated with human PPI networks may have affected our results.
Conclusions
The mechanism of occurrence and development of ESCC is extremely complex and involves a variety of factors, such as genetic and environmental factors. In this study, we used a systems biology framework to select candidate genes and performed a variety of analyses on ESCC. By integrating information from tandem analysis of GO, pathways, and pathway crosstalk, we found that ESCC was associated with a variety of cancer pathways, cell proliferation, apoptosis, immune responses, and drug metabolism. In addition, in ESCC-specific network, some of these additional genes have been reported to be associated with ESCC. In order to reveal the molecular mechanism of ESCC, we initially constructed its molecular network. Our research facilitates a more in-depth understanding of the mechanism of ESCC.
Acknowledgments
The primary results of the abstract in this study were presented as a meeting poster (No. PS02.037) in the 2018 International Society for Diseases of the Esophagus.
Funding: This work was funded by Bethune Charitable Foundation (No. HZB-20190528-19).
Footnote
Reporting Checklist: The authors have completed the STREGA reporting checklist. Available at https://atm.amegroups.com/article/view/10.21037/atm-22-6512/rc
Conflicts of Interest: All authors have completed the ICMJE uniform disclosure form (available at https://atm.amegroups.com/article/view/10.21037/atm-22-6512/coif). The authors have no conflicts of interest to declare.
Ethical Statement: The authors are accountable for all aspects of the work in ensuring that questions related to the accuracy or integrity of any part of the work are appropriately investigated and resolved. The study was conducted in accordance with the Declaration of Helsinki (as revised in 2013).
Open Access Statement: This is an Open Access article distributed in accordance with the Creative Commons Attribution-NonCommercial-NoDerivs 4.0 International License (CC BY-NC-ND 4.0), which permits the non-commercial replication and distribution of the article with the strict proviso that no changes or edits are made and the original work is properly cited (including links to both the formal publication through the relevant DOI and the license). See: https://creativecommons.org/licenses/by-nc-nd/4.0/.
References
- Smyth EC, Lagergren J, Fitzgerald RC, et al. Oesophageal cancer. Nat Rev Dis Primers 2017;3:17048. [Crossref] [PubMed]
- Tao Y, Chai D, Ma L, et al. Identification of distinct gene expression profiles between esophageal squamous cell carcinoma and adjacent normal epithelial tissues. Tohoku J Exp Med 2012;226:301-11. [Crossref] [PubMed]
- Testa U, Castelli G, Pelosi E. Esophageal Cancer: Genomic and Molecular Characterization, Stem Cell Compartment and Clonal Evolution. Medicines (Basel) 2017;4:67. [Crossref] [PubMed]
- Holmes RS, Vaughan TL. Epidemiology and pathogenesis of esophageal cancer. Semin Radiat Oncol 2007;17:2-9. [Crossref] [PubMed]
- Lagergren J, Smyth E, Cunningham D, et al. Oesophageal cancer. Lancet 2017;390:2383-96. [Crossref] [PubMed]
- Song Y, Li L, Ou Y, et al. Identification of genomic alterations in oesophageal squamous cell cancer. Nature 2014;509:91-5. [Crossref] [PubMed]
- Müller LB, Meurer L, Lopes AB, et al. Stepwise expression of CDKN2A and RB1 proteins in esophageal mucosa from patients at high risk for squamous cell carcinoma. Appl Immunohistochem Mol Morphol 2014;22:669-73. [Crossref] [PubMed]
- Ni X, Wu P, Wu J, et al. Hyperthermic intraperitoneal perfusion chemotherapy and response evaluation in patients with gastric cancer and malignant ascites. Oncol Lett 2017;14:1691-6. [Crossref] [PubMed]
- Shannon P, Markiel A, Ozier O, et al. Cytoscape: a software environment for integrated models of biomolecular interaction networks. Genome Res 2003;13:2498-504. [Crossref] [PubMed]
- Cowley MJ, Pinese M, Kassahn KS, et al. PINA v2.0: mining interactome modules. Nucleic Acids Res 2012;40:D862-5. [Crossref] [PubMed]
- Zheng S, Zhao Z. GenRev: exploring functional relevance of genes in molecular networks. Genomics 2012;99:183-8. [Crossref] [PubMed]
- Kishino T, Niwa T, Yamashita S, et al. Integrated analysis of DNA methylation and mutations in esophageal squamous cell carcinoma. Mol Carcinog 2016;55:2077-88. [Crossref] [PubMed]
- Wu Z, Zhou J, Zhang X, et al. Reprogramming of the esophageal squamous carcinoma epigenome by SOX2 promotes ADAR1 dependence. Nat Genet 2021;53:881-94. [Crossref] [PubMed]
- Li L, Xu J, Qiu G, et al. Epigenomic characterization of a p53-regulated 3p22.2 tumor suppressor that inhibits STAT3 phosphorylation via protein docking and is frequently methylated in esophageal and other carcinomas. Theranostics 2018;8:61-77. [Crossref] [PubMed]
- Scott KA, Moore RJ, Arnott CH, et al. An anti-tumor necrosis factor-alpha antibody inhibits the development of experimental skin tumors. Mol Cancer Ther 2003;2:445-51.
- Xie Y, Wang B. Downregulation of TNFAIP2 suppresses proliferation and metastasis in esophageal squamous cell carcinoma through activation of the Wnt/β-catenin signaling pathway. Oncol Rep 2017;37:2920-8. [Crossref] [PubMed]
- Chen Y, Wang Y, Song H, et al. Expression profile of apoptosis-related genes potentially explains early recurrence after definitive chemoradiation in esophageal squamous cell carcinoma. Tumour Biol 2014;35:4339-46. [Crossref] [PubMed]
- Li J, Liang Y, Lv H, et al. miR-26a and miR-26b inhibit esophageal squamous cancer cell proliferation through suppression of c-MYC pathway. Gene 2017;625:1-9. [Crossref] [PubMed]
- Lebwohl D, Canetta R. Clinical development of platinum complexes in cancer therapy: an historical perspective and an update. Eur J Cancer 1998;34:1522-34. [Crossref] [PubMed]
- Oberoi HS, Nukolova NV, Kabanov AV, et al. Nanocarriers for delivery of platinum anticancer drugs. Adv Drug Deliv Rev 2013;65:1667-85. [Crossref] [PubMed]
- Jia P, Kao CF, Kuo PH, et al. A comprehensive network and pathway analysis of candidate genes in major depressive disorder. BMC Syst Biol 2011;5:S12. [Crossref] [PubMed]
- Gao YB, Chen ZL, Li JG, et al. Genetic landscape of esophageal squamous cell carcinoma. Nat Genet 2014;46:1097-102. [Crossref] [PubMed]
- Huang YH, Cai K, Xu PP, et al. CREBBP/EP300 mutations promoted tumor progression in diffuse large B-cell lymphoma through altering tumor-associated macrophage polarization via FBXW7-NOTCH-CCL2/CSF1 axis. Signal Transduct Target Ther 2021;6:10. [Crossref] [PubMed]
- Sawada G, Niida A, Hirata H, et al. An Integrative Analysis to Identify Driver Genes in Esophageal Squamous Cell Carcinoma. PLoS One 2015;10:e0139808. [Crossref] [PubMed]
- Cui D, Zhu Y, Yan D, et al. Dual inhibition of cMET and EGFR by microRNA-338-5p suppresses metastasis of esophageal squamous cell carcinoma. Carcinogenesis 2021;42:995-1007. [Crossref] [PubMed]
- Perez-Janices N, Blanco-Luquin I, Torrea N, et al. Differential involvement of RASSF2 hypermethylation in breast cancer subtypes and their prognosis. Oncotarget 2015;6:23944-58. [Crossref] [PubMed]
- Haluskova J, Lachvac L, Nagy V. The investigation of GSTP1, APC and RASSF1 gene promoter hypermethylation in urine DNA of prostate-diseased patients. Bratisl Lek Listy 2015;116:79-82. [Crossref] [PubMed]
- Tian B, Liu J, Zhang N, et al. Oncogenic SNORD12B activates the AKT-mTOR-4EBP1 signaling in esophageal squamous cell carcinoma via nucleus partitioning of PP-1α. Oncogene 2021;40:3734-47. [Crossref] [PubMed]
- Schmidt ML, Hobbing KR, Donninger H, et al. RASSF1A Deficiency Enhances RAS-Driven Lung Tumorigenesis. Cancer Res 2018;78:2614-23. [Crossref] [PubMed]
- Yu W, Chu L, Zhao K, et al. A nomogram based on phosphorylated AKT1 for predicting locoregional recurrence in patients with oesophageal squamous cell carcinoma. J Cancer 2017;8:3755-63. [Crossref] [PubMed]
- Hyland PL, Freedman ND, Hu N, et al. Genetic variants in sex hormone metabolic pathway genes and risk of esophageal squamous cell carcinoma. Carcinogenesis 2013;34:1062-8. [Crossref] [PubMed]
- Malik MA, Upadhyay R, Mittal RD, et al. Association of xenobiotic metabolizing enzymes genetic polymorphisms with esophageal cancer in Kashmir Valley and influence of environmental factors. Nutr Cancer 2010;62:734-42. [Crossref] [PubMed]
- Zhou P, Zhang R, Wang Y, et al. Cepharanthine hydrochloride reverses the mdr1 (P-glycoprotein)-mediated esophageal squamous cell carcinoma cell cisplatin resistance through JNK and p53 signals. Oncotarget 2017;8:111144-60. [Crossref] [PubMed]
- Chi Y, Wang F, Zhang Y, et al. Apatinib inhibits tumour progression and promotes antitumour efficacy of cytotoxic drugs in oesophageal squamous cell carcinoma. J Cell Mol Med 2022;26:1905-17. [Crossref] [PubMed]
- Li T, Wang G. Computer-aided targeting of the PI3K/Akt/mTOR pathway: toxicity reduction and therapeutic opportunities. Int J Mol Sci 2014;15:18856-91. [Crossref] [PubMed]
- Furusawa S, Wu J. The effects of biscoclaurine alkaloid cepharanthine on mammalian cells: implications for cancer, shock, and inflammatory diseases. Life Sci 2007;80:1073-9. [Crossref] [PubMed]
- Wang L, Zhang Z, Yu X, et al. SOX9/miR-203a axis drives PI3K/AKT signaling to promote esophageal cancer progression. Cancer Lett 2020;468:14-26. [Crossref] [PubMed]
- Lin JJ, Su JH, Tsai CC, et al. 11-epi-Sinulariolide acetate reduces cell migration and invasion of human hepatocellular carcinoma by reducing the activation of ERK1/2, p38MAPK and FAK/PI3K/AKT/mTOR signaling pathways. Mar Drugs 2014;12:4783-98. [Crossref] [PubMed]
- Zhou J, Jiang YY, Chen H, et al. Tanshinone I attenuates the malignant biological properties of ovarian cancer by inducing apoptosis and autophagy via the inactivation of PI3K/AKT/mTOR pathway. Cell Prolif 2020;53:e12739. [Crossref] [PubMed]
- Blaes J, Thomé CM, Pfenning PN, et al. Inhibition of CD95/CD95L (FAS/FASLG) Signaling with APG101 Prevents Invasion and Enhances Radiation Therapy for Glioblastoma. Mol Cancer Res 2018;16:767-76. [Crossref] [PubMed]
- Wang N, Zhang CQ, He JH, et al. MiR-21 down-regulation suppresses cell growth, invasion and induces cell apoptosis by targeting FASL, TIMP3, and RECK genes in esophageal carcinoma. Dig Dis Sci 2013;58:1863-70. [Crossref] [PubMed]
- Du X, Hong L, Sun L, et al. miR-21 induces endothelial progenitor cells proliferation and angiogenesis via targeting FASLG and is a potential prognostic marker in deep venous thrombosis. J Transl Med 2019;17:270. [Crossref] [PubMed]
- Chen Q, Luo G, Zhang X. MiR-148a modulates HLA-G expression and influences tumor apoptosis in esophageal squamous cell carcinoma. Exp Ther Med 2017;14:4448-52. [Crossref] [PubMed]
- Ito S, Okano S, Morita M, et al. Expression of PD-L1 and HLA Class I in Esophageal Squamous Cell Carcinoma: Prognostic Factors for Patient Outcome. Ann Surg Oncol 2016;23:508-15. [Crossref] [PubMed]
- Jhunjhunwala S, Hammer C, Delamarre L. Antigen presentation in cancer: insights into tumour immunogenicity and immune evasion. Nat Rev Cancer 2021;21:298-312. [Crossref] [PubMed]
- Golyan FF, Abbaszadegan MR, Forghanifard MM. TWIST1, MMP-21, and HLAG-1 co-overexpression is associated with ESCC aggressiveness. J Cell Biochem 2019;120:14838-46. [Crossref] [PubMed]
- Zheng J, Xu C, Chu D, et al. Human leukocyte antigen G is associated with esophageal squamous cell carcinoma progression and poor prognosis. Immunol Lett 2014;161:13-9. [Crossref] [PubMed]
- Hu J, Li L, Liu Y, et al. Overexpression of HLA-G Is positively associated with Kazakh esophageal squamous cell carcinoma in Xinjiang, China. Viral Immunol 2013;26:180-4. [Crossref] [PubMed]
- Baccar A, Ferchichi I, Troudi W, et al. CD99 and HLA-II immunostaining in breast cancer tissue and their correlation with lymph node metastasis. Dis Markers 2013;34:363-71. [Crossref] [PubMed]
- De Lerma Barbaro A, De Ambrosis A, Banelli B, et al. Methylation of CIITA promoter IV causes loss of HLA-II inducibility by IFN-gamma in promyelocytic cells. Int Immunol 2008;20:1457-66. [Crossref] [PubMed]
- Jo YS, Lee JC, Li S, et al. Significance of the expression of major histocompatibility complex class II antigen, HLA-DR and -DQ, with recurrence of papillary thyroid cancer. Int J Cancer 2008;122:785-90. [Crossref] [PubMed]
- Lv J, Cao XF, Ji L, et al. Association of Wnt1/beta-catenin with clinical pathological characteristics and prognosis of esophageal squamous cell carcinoma. Genet Test Mol Biomarkers 2010;14:363-9. [Crossref] [PubMed]
- Shen LY, Wang H, Dong B, et al. Possible prediction of the response of esophageal squamous cell carcinoma to neoadjuvant chemotherapy based on gene expression profiling. Oncotarget 2016;7:4531-41. [Crossref] [PubMed]
- Wang H, Shen L, Lin Y, et al. The expression and prognostic significance of Mucin 13 and Mucin 20 in esophageal squamous cell carcinoma. J Cancer Res Ther 2015;11:C74-9. [Crossref] [PubMed]
- Li Y, Zhang Q, Peng B, et al. Identification of glutathione S-transferase omega 1 (GSTO1) protein as a novel tumor-associated antigen and its autoantibody in human esophageal squamous cell carcinoma. Tumour Biol 2014;35:10871-7. [Crossref] [PubMed]
- Xie F, Zhang D, Qian X, et al. Analysis of cancer-promoting genes related to chemotherapy resistance in esophageal squamous cell carcinoma. Ann Transl Med 2022;10:92. [Crossref] [PubMed]
- Yang Y, Sun Z, Shi Y, et al. Integrated bioinformatics analysis to identify key genes related to the prognosis of esophageal squamous cell carcinoma. Transl Cancer Res 2021;10:1679-91. [Crossref] [PubMed]
- Li LY, Jiang H, Xie YM, et al. Macrolide analog F806 suppresses esophageal squamous cell carcinoma (ESCC) by blocking β1 integrin activation. Oncotarget 2015;6:15940-52. [Crossref] [PubMed]
- Luo A, Yu X, Li G, et al. Differentiation-associated genes regulated by c-Jun and decreased in the progression of esophageal squamous cell carcinoma. PLoS One 2014;9:e96610. [Crossref] [PubMed]
- Jiang YY, Jiang Y, Li CQ, et al. TP63, SOX2, and KLF5 Establish a Core Regulatory Circuitry That Controls Epigenetic and Transcription Patterns in Esophageal Squamous Cell Carcinoma Cell Lines. Gastroenterology 2020;159:1311-1327.e19. [Crossref] [PubMed]
- Ahrens TD, Timme S, Hoeppner J, et al. Selective inhibition of esophageal cancer cells by combination of HDAC inhibitors and Azacytidine. Epigenetics 2015;10:431-45. [Crossref] [PubMed]
- Chen J, Guo L, Peiffer DA, et al. Genomic profiling of 766 cancer-related genes in archived esophageal normal and carcinoma tissues. Int J Cancer 2008;122:2249-54. [Crossref] [PubMed]
- Li X, Jiang Z, Feng J, et al. 2-Acetylamino-3-[4-(2-acetylamino-2-carboxyethylsulfanylcarbonylamino) phenyl carbamoylsulfanyl] propionic acid, a glutathione reductase inhibitor, induces G2/M cell cycle arrest through generation of thiol oxidative stress in human esophageal cancer cells. Oncotarget 2017;8:61846-60.
- Li LY, Zhang K, Jiang H, et al. Quantitative proteomics reveals the downregulation of GRB2 as a prominent node of F806-targeted cell proliferation network. J Proteomics 2015;117:145-55. [Crossref] [PubMed]
- Lu J, Xue L, Jin M, et al. Expression profiling of metastasis-related microRNAs in early esophageal squamous cell carcinoma. Zhonghua Bing Li Xue Za Zhi 2014;43:313-7.
- Palumbo A Jr, Da Costa NM, De Martino M, et al. UBE2C is overexpressed in ESCC tissues and its abrogation attenuates the malignant phenotype of ESCC cell lines. Oncotarget 2016;7:65876-87. [Crossref] [PubMed]
- Wang J, Zhang Z, Che Y, et al. Rabdocoestin B exhibits antitumor activity by inducing G2/M phase arrest and apoptosis in esophageal squamous cell carcinoma. Cancer Chemother Pharmacol 2018;81:469-81. [Crossref] [PubMed]
- Yin X, Zhang R, Feng C, et al. Diallyl disulfide induces G2/M arrest and promotes apoptosis through the p53/p21 and MEK-ERK pathways in human esophageal squamous cell carcinoma. Oncol Rep 2014;32:1748-56. [Crossref] [PubMed]
(English Language Editor: J. Jones)