Bioinformatics analysis of differentially expressed genes in diabetic foot ulcer and preliminary experimental verification
Highlight box
Key findings
• Bioinformatics analysis was performed in this study to search for signaling pathways that may be involved in the development of diabetic foot ulcers (DFUs).
What is known and what is new?
• It was observed that some genes were differentially expressed in the DFU and control groups, and these genes played different roles in the occurrence and development of diseases through various mechanisms of action. However, molecular genetics research on DFU is still lacking.
• With the widespread application of DNA microarray data, we can obtain the simultaneous expression of tens of thousands of genes in cells or tissues. The identification of differentially expressed genes can be made more reliable by integrating datasets from multiple microarrays.
What is the implication, and what should change now?
• We also found that the BNIP3 gene may be a new biomarker and intervention target for DFU.
Introduction
Diabetic foot ulcer (DFU) is one of the main complications of diabetes mellitus (DM) and is related to peripheral vascular disease, peripheral neuropathy, trauma, and high plantar pressure. DFU is the result of interaction between multiple risk factors, including smoking, age, blood pressure, blood glucose level, DM course, blocked vascular circulation, nerve injury, etc. (1), and its incidence in DM patients is as high as 25% (2). In 2016, there were 18.6 million DFU patients worldwide, among which 36.55% required amputation (3). Amputation seriously reduces the quality of life of patients and leads to high mortality rates and medical costs (4). Molecular genetics, through the use of molecular biology technology, studies the mechanism of disease occurrence and development at the molecular level and plays an important role in the prevention, diagnosis, and treatment of diseases. From a molecular perspective, wound healing is a process that occurs after breaking through the skin barrier and is often mediated by growth factors and cytokines released by specialized cells activated by the immune response. Long-term high glucose induces abnormal expression of many molecules, such as vascular endothelial growth factor, hypoxia-inducible factor 1, and anti-inflammatory factors, leading to poor angiogenesis and persistent chronic inflammation, which are pathogenic factors for delayed wound healing. Studies have shown that the current mature clinical treatment methods can promote wound healing by interfering with the wound microenvironment during the occurrence and development of diabetic foot ulcers (5,6). For example, the method of negative pressure wound therapy can improve the wound microenvironment, change microvascular hemodynamics, control wound infection, promote endothelial cell regeneration (5), and accelerate wound healing (6). Therefore, it is potentially clinically and socially significant to explore the molecular changes and functions of DFU during its development.
With the progress of molecular genetics technology, DNA microarray data has been widely applied, which has stimulated new research directions in bioinformatics. DNA microarrays allow us to obtain the simultaneous expression of tens of thousands of genes in DFU disease cells or tissues. The identification of differentially expressed genes (DEGs) can be made more reliable by integrating multiple microarray datasets. In this study, the key DEGs in DFU were identified using the bioinformatics analysis method, their functions and mechanisms of action were analyzed, and preliminary experimental verification was conducted.
In this paper, we obtained the co-expressed DEGs (co-DEGs) of DFU from the Gene Expression Omnibus (GEO) database. Based on these genes, we conducted a series of analyses, including Gene Ontology (GO) functional enrichment analysis, protein interaction network analysis, Kyoto Encyclopedia of Genes and Genomes (KEGG) pathway enrichment analysis, miRNA-related database retrieval, etc. The expression of the gene encoding protein in high glucose high glucose (HG)-treated human umbilical vein endothelial cells (HUVECs) was also observed. We present the following article in accordance with the MDAR reporting checklist (available at https://atm.amegroups.com/article/view/10.21037/atm-22-6437/rc).
Methods
Data collection and processing
The study was conducted in accordance with the Declaration of Helsinki (as revised in 2013). The GEO is an international public repository of microarrays, second-generation sequencing, and other forms of high-throughput functional genomic datasets submitted by the research community. It was established and maintained by the National Center for Biotechnology Information (NCBI). We performed the NCBI database retrieval as follows: after the home page, we selected the GEO datasets and entered the GEO Home database (https://www.ncbi.nlm.nih.gov/geo/). Next, we entered the Diabetic foot ulcer search words, and expression profiling was selected by the array to further screen to download the related gene chip data from the obtained search results for subsequent bioinformatics functional analysis.
Two independent diabetic foot-related chip datasets, GSE68183 and GSE80178, were used in this study, both of which use the GPL16686 (Affymetrix Human Gene 2.0 ST Array) chip platform. The GSE68183 dataset included three diabetic foot (DF) patients and three non-diabetic foot (NDF) patients, and the GSE80178 dataset included six DFU patients, three DM patients, and three healthy people with non-diabetes mellitus (NDM). The Biobase, GEOquery, and Limma R packages (R 3.6.3) were used to obtain data from the GEO database. After data cleaning, the dplyr and ggplot2 R packages were used to draw a volcano map. GSE68183 set filter for |Log2|fold change (FC)| >1 and P<0.05, including Log2FC >1 and P<0.05 genes represents raised differences, Log2FC <1 and P<0.05 gene differences on behalf of the downgrade. GSE80178 set filter for |Log2FC| >1 and |P-adjusted (Padj)|<0.01, including Log2FC >1 and Padj <0.01 genes represents raised differences, Log2FC <1 and Padj <0.01 gene differences on behalf of the downgrade.
Acquisition of DEGs
DEGs were obtained from the GSE80178 dataset data matrix, and the DAVID database (https://david.ncifcrf.gov/) was employed to convert the NCBI reference sequence for the gene, the Excel (WPS Office) data sorting and cleaning, and converted Txt file. After standardized processing by R, the ggplot2 and ComplexHeatmap packages were used to display the expressions of different genes in the different groups, and automatic clustering was performed to draw the heat maps. GSM2114231-GSM2114236 was the DFU group, GSM2114237-GSM2114239 was the DM group, and GSM2114240-GSM2114242 was the healthy control group.
Acquisition of the co-DEGs
The GEO2R tool on the GEO website (R 3.2.3, Biobase 2.30.0, GEOquery 2.40.0, limma 3.26.8) was used for the two datasets. The experimental group was first defined, followed by the control group, and screening adjustment was set as Padj <0.05. The top 250 DEGs were screened and derived. The DAVID database was then used to convert the NCBI reference sequence into a gene name, and the VennDiagram R package was applied to draw a Venn diagram. Data from the GSE80178 and GSE68183 datasets were defined as G1 and G2, respectively. The FC cutoff was set at <−1.2, with Padj <0.05 in G1 and P<0.05 in G2.
The co-DEGs data matrix obtained from the GSE80178 dataset was standardized using R, and heat maps were then drawn using the ggplot2 and ComplexHeatmap packages to show the expression of co-DEGs among the different groups.
GO function analysis of co-DEGs
By WebGestalt database (http://www.webgestalt.org/) enrichment of co-DEGs GO function analysis, less quantity of co-DEGs false discovery rate (FDR) acuity 0.05, but P values <0.05. The functional enrichment of the co-DEGs gene was analyzed at the biological process (BP), molecular function (MF), and cellular component (CC) levels.
Protein-protein interaction (PPI) network analysis
The protein interaction network was constructed using the STRING database (https://string-db.org/), and the interaction score was set to ≥0.9, with 20 first-level interacting proteins. The data were exported using STRING and analyzed by Cytoscape software, and the top 10 hub genes in the relational network data were searched using the cytoHubba plug-in. We then searched the Genecards database, checked the gene illustration and function, and the hub gene was determined for further analysis.
GO functional analysis of the hub genes and KEGG enrichment analysis and verification
The GO function of BNIP3 (BCL2 interacting protein 3) and its related hub genes were analyzed and visualized at the BP, MF, and CC levels using the cluster profile and org.hs.eg. db packages of R language.
KEGG enrichment analysis of the BNIP3 gene and its related hub gene was performed using WebGestalt. Also, the KEGG database (https://www.kegg.jp/) was used to search for BNIP3-related signaling pathways.
Targeted regulation of microRNA acquisition of hub genes
Related miRNAs regulating the BNIP3 gene were searched using the miRDB database, and miRNAs with more than 90 points were obtained according to the target score. BNIP3-related miRNAs were searched using the TargetScan database.
Experimental groups
The damage of endothelial cell function is the initial factor of vascular complications in diabetic patients, and human umbilical vein endothelial cells (HUVECs) have the potential of stem cells. Therefore, this study selected HUVECs were cultured and grouped according to different glucose concentrations and different intervention times to verify the differential expression of the hub gene. The HUVEC cell line was purchased from Wuhan Pnuosai Life Technology Co., Ltd. (China). Cell culture and intervention were performed in the Central Laboratory of Shanxi Provincial People’s Hospital. A HUVEC cell culture flask was added with HUVEC special complete medium (Gemme Gene Medical Technology Co., Ltd., Shanghai, China) containing dulbecco’s modified eagle medium (DMEM) HG, 0.01 mg/mL insulin, 1% non-essential amino acids (NEAA), 10% fetal bovine serum (FBS), and 1% penicillin-streptomycin (P/S). The conventional culture was carried out at 37 ℃ in a 5% carbon dioxide (CO2) incubator. When the cell density reached 80–90%, a 50% glucose solution (Otsuka Pharmaceutical Co., Ltd., China) was added to the medium to obtain final medium glucose concentrations of 5.6, 11.2, 16.8, and 33.6 mmol/L, respectively. They were then divided into two groups according to the incubation time (24, 72 h). The growth state of the cells under the different glucose concentrations was observed at 24 and 72 h, respectively, and the protein was extracted.
Western blot
Samples were extracted using enhanced Radio Immunoprecipitation Assay (RIPA) lysate (Relying on Bio, Hebei, China) and loaded with 30 µg of sample protein and 5 µL of pre-stained Mark (Polymers Biotechnology Co., Ltd., Beijing, China) on a 12% sodium dodecyl sulphate-polyacrylamide gel electrophoresis (SDS-PAGE) (Baushde Bioengineering Co., Ltd., Wuhan, China). Electrophoresis was performed at a constant pressure of 80 V for about 30 min and then continued at 120 V for about 60 min until the proteins were completely separated or electrophoresed to the lower edge of the glass plate.
Next, we transferred the samples to a polyvinylidene fluoride (PVDF) membrane (Merck Millipore, USA) at 250 mA for 90 min. The PVDF membrane was placed in a tris-buffered saline and Tween 20 (TBST) solution (Solarbio, Beijing, China) containing 5% skim milk powder and blocked on a shaker for 1h at room temperature. PVDF membranes were then cut according to the molecular weights of BNIP3 (Abcam, UK) and β-actin (Jinqiao Biotechnology Co., Ltd., Beijing, China) into a diluted BNIP3 monoclonal antibody and β-actin monoclonal antibody, respectively, and incubated at 4 ℃ overnight (12–16 h). A diluted secondary antibody (SAB, USA) was added and incubated for 1 h on a shaker at room temperature.
The enhanced chemiluminescence (ECL) color solution (Baude Bioengineering Co., Ltd., Wuhan, China) was prepared with a chemical luminant liquid A and liquid B at an A ratio of 1:1. Subsequently, the solution was evenly dropped onto the surface of the PVDF membrane, and the phase was scanned using the gel imaging system. The relative semi-quantitative value of each target protein could then be obtained by comparing the gray value of each target protein with that of β-actin.
Statistical analysis
GraphPad Prism 9 (GraphPad Software, Inc., San Diego, CA, USA) was used for statistical analysis of the experimental data, and one-way analysis of variance (ANOVA) was used for data analysis between multiple subjects. The experimental results were expressed as (standard deviation). P<0.05 was considered statistically significant.
Results
Screening results of DEGs in the two datasets
The GSE68183 and GSE80178 datasets were obtained using R and volcano maps were drawn (Figure 1A,1B). In the GSE68183 dataset, there was no statistically significant significance in gene expression between the DF and NDF groups under Padj <0.05; however, the difference in gene expression between the DF and NDF groups under P<0.05 was significant, indicating an obvious up-regulation and down-regulation trend in which 121 genes were down-regulated and 92 genes were up-regulated in DF. In the GSE80178 dataset, the gene expression in the DFU group was markedly different from that in DM and NDM groups under Padj <0.05. A total of 880 genes were down-regulated and 42 genes were up-regulated in the DFU group.
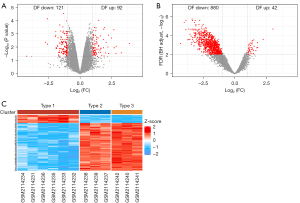
The data matrix of GSE80178 was downloaded from the GEO database, and the heat map was drawn using R (Figure 1C). The samples in Type 1 were the DFU group, samples in Type 2 were the DM group, and samples in Type 3 were the NDM group. In the three groups, the gene expression of the DFU group was substantially different from that of the DM and NDM groups, and the expression of most genes in the DFU group was lower than those in the other two groups. The gene expression in the DM and NDM groups also showed a stepwise progression compared with that in the DFU group.
Screening of the co-DEGs
A Venn diagram was drawn using R 3.6.3 and the VennDiagram package, and data from the GSE80178 and GSE68183 datasets were defined as G1 and G2, respectively (Figure 2A). The FC cutoff was set at <−1.2, with Padj <0.05 in G1 and P<0.05 in G2. A total of 322 DEGs were screened from the GSE80178 dataset, including 47 up-regulated genes and 275 down-regulated genes. A total of 96 DEGs were screened from the GSE68183 dataset, including 40 up-regulated genes and 56 down-regulated genes. Also, six DEGs were screened from the two datasets, including three up-regulated genes (BNIP3, HEPHL1, and KLK10) and three down-regulated genes (FRZB, KLHDC1, and NHLRC3).
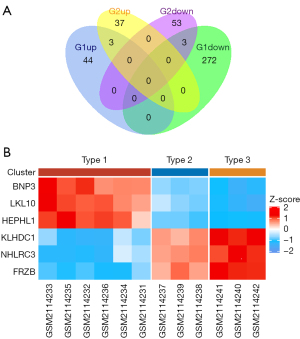
After standardized processing of the co-DEGs data matrix obtained from the GSE80178 dataset, the ggplot2 and ComplexHeatmap R language packages were used to show the co-DEGs expression among different groups (Figure 2B). We observed that the co-DEGs were significantly differentially expressed in each group, and there were regular increasing high expression and decreasing low expression between the three groups.
GO function analysis of the co-expressed differential genes
The co-DEGs were imported into WebGestalt for GO enrichment analysis at the BP, CC, and MF levels. In terms of BP, the ten statistically significant BPs included the mitochondrial protein catabolic BNIP3-related process, the granzyme-mediated apoptotic signaling pathway, negative regulation of mitochondrial fusion, negative regulation of the mitochondrial membrane, the intrinsic apoptotic signaling pathway in response to hypoxia, positive regulation of necrotic cell death, autophagic cell death, negative regulation of membrane permeability, frizzled-related protein (FRZB)-related convergent extension involved in organogenesis, and fat cell differentiation associated with BNIP3 and FRZB (Table 1).
Table 1
Gene set | Description | Gene symbol | P value |
---|---|---|---|
GO:0035694 | Mitochondrial protein catabolic process | BNIP3 | 0.0014995 |
GO:0045444 | Fat cell differentiation | BNIP3, FRZB | 0.0015415 |
GO:0008626 | Granzyme-mediated apoptotic signaling pathway | BNIP3 | 0.0017992 |
GO:0010637 | Negative regulation of mitochondrial fusion | BNIP3 | 0.0017992 |
GO:0035795 | Negative regulation of mitochondrial membrane permeability | BNIP3 | 0.0017992 |
GO:1990144 | Intrinsic apoptotic signaling pathway in response to hypoxia | BNIP3 | 0.0017992 |
GO:0060029 | Convergent extension involved in organogenesis | FRZB | 0.0017992 |
GO:0010940 | Positive regulation of necrotic cell death | BNIP3 | 0.0020988 |
GO:0048102 | Autophagic cell death | BNIP3 | 0.0020988 |
GO:1905709 | Negative regulation of membrane permeability | BNIP3 | 0.0023984 |
GO, Gene Ontology; DEG, differentially expressed gene.
The 10 statistically significant CCs were also retrieved, including the BNIP3-related components of the mitochondrial outer membrane, the intrinsic components of the outer mitochondrial membrane, the components of the mitochondrial membrane, the intrinsic components of the mitochondrial membrane, the NHL repeat containing 3 (NHLRC3)-related azurophil granule lumen, the primary lysosome, the azurophil granule, the vacuolar lumen, the secretory granule, and the secretory vesicle of KLK10 and NHLRC3 (Table 2).
Table 2
Gene set | Description | Gene symbol | P value |
---|---|---|---|
GO:0031307 | Integral component of mitochondrial outer membrane | BNIP3 | 0.0034068 |
GO:0031306 | Intrinsic component of mitochondrial outer membrane | BNIP3 | 0.0035769 |
GO:0030141 | Secretory granule | KLK10, NHLRC3 | 0.0064756 |
GO:0099503 | Secretory vesicle | KLK10, NHLRC3 | 0.0088835 |
GO:0032592 | Integral component of mitochondrial membrane | BNIP3 | 0.011721 |
GO:0098573 | Intrinsic component of mitochondrial membrane | BNIP3 | 0.011890 |
GO:0035578 | Azurophil granule lumen | NHLRC3 | 0.015270 |
GO:0005766 | Primary lysosome | NHLRC3 | 0.026200 |
GO:0042582 | Azurophil granule | NHLRC3 | 0.026200 |
GO:0005775 | Vacuolar lumen | NHLRC3 | 0.028711 |
GO, Gene Ontology; DEG, differentially expressed gene.
Moreover, five statistically significant MFs were found, including hephaestin-like 1 (HEPHL1)-related ferroxidase activity, oxidoreductase activity, oxidizing metal ions, oxygen as an acceptor, and copper ion binding. Binding Wnt proteins associated with FRZB (Table 3).
Table 3
Gene set | Description | Gene symbol | P value |
---|---|---|---|
GO:0004322 | Ferroxidase activity | HEPHL1 | 0.0026972 |
GO:0016724 | Oxidoreductase activity, oxidizing metal ions, oxygen as acceptor | HEPHL1 | 0.0026972 |
GO:0016722 | Oxidoreductase activity, oxidizing metal ions | HEPHL1 | 0.0053886 |
GO:0017147 | Wnt-protein binding | FRZB | 0.011051 |
GO:0005507 | Copper ion binding | HEPHL1 | 0.016984 |
GO, Gene Ontology; DEG, differentially expressed gene.
PPI network analysis of the co-DEGs
STRING was utilized to construct the protein interaction network (Figure 3A). We found that the six co-DEGs were independent of each other. BNIP3 is correlated with BCL2, BCL2L1, RHEB, GABARAPL1, GABARAP, and GABARAPL2, suggesting that these six genes are closely related to the function of BNIP3. Also, NHLRC3 is correlated with ACTR10, TXNDC5, PSMD1, and C3, suggesting that these four genes may be involved in the role of NHLRC3.
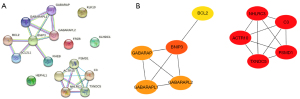
The data were derived using STRING, and the cytoHubba plug-in in Cytoscape was used to screen the top 10 core (hub) genes in the co-DEGs PPI network (Figure 3B). NHLRC3 and its interacting genes had the same scores and ranked from 1–5, respectively. The BNIP3 gene was ranked sixth. The scores of GABARAPL1, GABARAP, and GABARAPL2 ranked 7–9, and BCL2 was ranked tenth. Also, NHLRC3 and BNIP3 were up-regulated in DFU compared with the control group.
The NHLRC3 and BNIP3 genes were searched using the Genecards database. NHLRC3 is a protein-coding gene associated with innate immune system pathways; this gene encodes proteins containing NCL-1, HT2A, and LIN-41 (NHL) family repeats. Proteins containing mammalian NHL repeats may be involved in a variety of enzymatic processes, including protein modification by ubiquitination. BNIP3 encodes a mitochondrial protein that contains the BH3 domain and acts as a proapoptotic factor. The encoding protein E1B 19 kDa interacts with the anti-apoptotic protein Bcl2, and DNA methylation silences this gene in tumors. Combined with the above GO function analysis, the key genes of BNIP3 were selected for further analysis.
GO function analysis, KEGG enrichment analysis, and verification
GO function analysis of BNIP3 and its interacting genes
The R language cluster profile and org.hs.eg. db packages were used to analyze and visualize the GO functions of BNIP3 and its five interacting genes at the CC, MF, and BP levels (Figure 4). The CC level was significantly enriched in the autophagosome membrane, autophagosome, and vacuolar membrane, and the rest were significantly enriched in the outer membrane of mitochondria, organelle, plasma membrane, cytoplasm binding cell projection, microtubules, nuclear capsule, and the mitochondrial outer membrane. The MF level was significantly enriched in the ubiquitin-binding protein ligase and ubiquitin-like protein ligase, the rest were significantly enriched in γ-aminobutyric acid (GABA) receptor binding, β-tubulin-binding, microtubulin binding, Tat protein binding, the Bcl-2 homo (BH) domain, and the death domain-binding protein phosphatase 2A. At the BP level, significant enrichment was mainly observed in the cell response to external stimuli, autophagy, and the utilization of the autophagy mechanism, the rest were significant enrichment was observed in mitochondrial autophagy, mitochondrial decomposition, organelle decomposition, the cell starvation response, hunger response, the cell response to nitrogen starvation, and the cell response to nitrogen level.
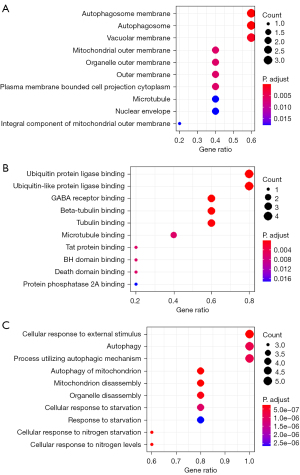
KEGG enrichment analysis and verification of BNIP3 and its interacting hub gene
KEGG enrichment analysis of BNIP3 and its interacting genes
KEGG pathway enrichment analysis of the BNIP3 gene and its related hub gene was conducted using WebGestalt, and it was found that BNIP3 gene and its related hub gene was significantly correlated with autophagy, mitochondrial autophagy, and the FoxO signaling pathway. The residue was significantly correlated with the nod-like receptor signaling pathway, γ-aminobutyl-synapse, the Apelin signaling pathway, and Kaposi sarcoma-associated herpesvirus infection, but not with apoptosis and the Hedgehog signaling pathway (Table 4).
Table 4
Gene set | Description | Overlap | P value | FDR | Gene symbol |
---|---|---|---|---|---|
hsa04140 | Autophagy | 5 | 1.56e-09 | 5.10e-07 | BNIP3; GABARAP; GABARAPL1; GABARAPL2; BCL2 |
hsa04137 | Mitophagy | 4 | 2.89e-08 | 4.71e-06 | BNIP3; GABARAP; GABARAPL1; GABARAPL2 |
hsa04068 | FoxO signaling pathway | 4 | 5.12e-07 | 5.56e-05 | BNIP3; GABARAP; GABARAPL1; GABARAPL2 |
hsa04136 | Autophagy | 3 | 7.70e-07 | 6.27e-05 | GABARAP; GABARAPL1; GABARAPL2 |
hsa04621 | NOD-like receptor signaling pathway | 4 | 1.35e-06 | 8.81e-05 | GABARAP; GABARAPL1; GABARAPL2; BCL2 |
hsa04727 | GABAergic synapse | 3 | 1.68e-05 | 9.15e-04 | GABARAP; GABARAPL1; GABARAPL2 |
Hsa04371 | Apelin signaling pathway | 3 | 6.37e-05 | 0.00296497 | GABARAP; GABARAPL1; GABARAPL2 |
hsa05167 | Kaposi sarcoma-associated herpesvirus infection | 3 | 1.59e-04 | 0.00646364 | GABARAP; GABARAPL1; GABARAPL2 |
hsa04215 | Apoptosis | 1 | 0.021818334 | 0.79030855 | BCL2 |
hsa04340 | Hedgehog signaling pathway | 1 | 0.031913697 | 0.9492984 | BCL2 |
KEGG, Kyoto Encyclopedia of Genes and Genomes; FDR, false discovery rate.
Pathway verification of BNIP3 and its interacting genes
The KEGG database was used to search for the BNIP3-related signaling pathways, and it was again verified to be correlated with the FoxO signaling pathway, mitochondrial autophagy, autophagy, and Shigella and Legionella (Figure 5).
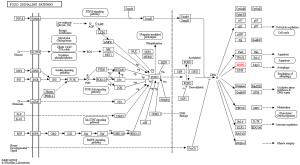
microRNA targeting regulation of hub genes
The miRDB database was used to search for related miRNAs regulating the BNIP3 gene. A total of 96 related miRNAs were searched, and 15 miRNAs with more than 90 points were obtained according to the target score (Table 5).
Table 5
Target rank | Target score | miRNA name | Gene symbol | Gene description |
---|---|---|---|---|
1 | 97 | hsa-miR-4717-5p | BNIP3 | BCL2 interacting protein 3 |
2 | 96 | hsa-miR-411-5p | BNIP3 | BCL2 interacting protein 3 |
3 | 96 | hsa-miR-182-5p | BNIP3 | BCL2 interacting protein 3 |
4 | 95 | hsa-miR-4753-5p | BNIP3 | BCL2 interacting protein 3 |
5 | 94 | hsa-miR-320c | BNIP3 | BCL2 interacting protein 3 |
6 | 94 | hsa-miR-320b | BNIP3 | BCL2 interacting protein 3 |
7 | 94 | hsa-miR-4429 | BNIP3 | BCL2 interacting protein 3 |
8 | 94 | hsa-miR-320a-3p | BNIP3 | BCL2 interacting protein 3 |
9 | 94 | hsa-miR-320d | BNIP3 | BCL2 interacting protein 3 |
10 | 91 | hsa-miR-583 | BNIP3 | BCL2 interacting protein 3 |
11 | 91 | hsa-miR-548av-5p | BNIP3 | BCL2 interacting protein 3 |
12 | 91 | hsa-miR-548k | BNIP3 | BCL2 interacting protein 3 |
13 | 90 | hsa-miR-145-5p | BNIP3 | BCL2 interacting protein 3 |
14 | 90 | hsa-miR-3180-5p | BNIP3 | BCL2 interacting protein 3 |
15 | 90 | hsa-miR-5195-3p | BNIP3 | BCL2 interacting protein 3 |
Also, the TargetScan database was used to search for BNIP3-related miRNAs, and a total of three conserved sites and several low-conserved sites were found, namely hsa-miR-182-5p, hsa-miR-96-5p, and hsa-miR-1271-5p (Figure 6A). Among them, the context++ score percentile and probability of conserved targeting (Pct) of hsa-miR-182-5p were optimal (Figure 6B).
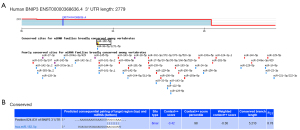
The expression level of BNIP3 protein in HUVECs in each group
The relative protein expression of BNIP3 in HUVECs under different glucose concentrations and different treatment times was detected by western blot (Figure 7A,7B). Groups a, b, c, and d received glucose treatment for 24 h at concentrations of 5.6, 11.2, 16.8, and 33.6 mmol/L, respectively, while groups A, B, C, and D received glucose treatment for 72 h (at the same concentrations listed above). There were no significant differences in the relative expression levels between the 24 and 72 h control groups, between group A and the 24 h control group (P<0.05), or between group A and the 72 h control group and group a. These results indicated that there was no significant difference in the expression of BNIP3 at 5.6 mmol/L glucose treatment for 24 and 72 h, and the difference was not statistically significant compared with the control group. Moreover, there was no significant difference between groups a and b, and between groups b and c. However, the differences between groups a and c (P<0.05) and those between groups c and d (P<0.0001) were significant. Furthermore, the differences between groups A and B, groups B and C, and groups C and D, (P<0.0001) were significant, as were those between groups b and B, groups c and C, and groups d and D (P<0.0001).
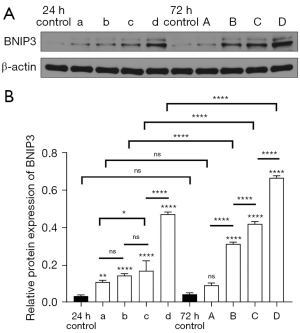
Discussion
In 2016, approximately 18.6 million people were suffering from DFU worldwide, which seriously affects the life quality and expectancy of patients and is also a major public health problem with a heavy societal burden (7). In previous studies, it was observed that some genes were differentially expressed in DFU and control groups, which played different roles in the occurrence and development of diseases through different mechanisms of action (8-13). For example, rs1024611 polymorphism of the monocyte chemoattractant protein-1 (MCP-1) gene is significantly correlated with MCP-1 expression and individual susceptibility to DFU (8). Also, silent mating-type information regulator 2 homolog 1 (SIRT1) is significantly down-regulated in DF patients, and the C allele of rs12778366 polymorphism of the SIRT1 gene may be a protective factor for DF (9). Moreover, the overexpression of taurine-upregulated genes 1 (TUG1) can restore endothelial progenitor cells (EPC) function after HG treatment by regulating the miR-29c-3p /PDGF-BB/Wnt signaling pathway (10). The upregulation of active-matrix metalloproteinase (MMP-9) results in diabetic wound healing difficulties in mice, and the inhibitor of MMP-9 is expected to be an effective treatment (11). It has also been shown that lysine oxidase gene polymorphism is correlated with DM and DFU patients compared with the healthy control group (12). Furthermore, the expression of NRF2 was found to be significantly decreased in type 2 diabetes (T2DM) and DFU patient cohorts, and NRF2 polymorphism rs182428269 was associated with the pathogeneses of T2DM and DFU (13).
However, molecular genetics research on DFU is still lacking. The study of molecular genetic changes in the pathogenesis of DFU will help to identify more effective prevention and treatment measures and potentially provide benefits to both patients and society. At the same time, with the widespread application of DNA microarray data, the simultaneous expression of tens of thousands of genes in cells or tissues can be obtained. Thus, the identification of DEGs can be made more reliable by integrating datasets from multiple microarrays. The GEO database is an international public repository containing microarray, second-generation sequencing, and other forms of high-throughput functional genomic datasets, which can facilitate online analysis, visualization, and free access to raw data, and is a common database for non-tumor data mining (14). Some other commonly used databases for molecular function analysis have been continuously updated and improved, and are increasingly used for bioinformatics analysis. For example, WebGestalt can be used for various enrichment analyses of different organisms and has been updated to WebGestalt 2019 (15). Additionally, the STRING database integrates all known and predicted associations between proteins, and Cytoscape can aggregate intermolecular interaction networks, high-throughput data, and other molecular states (16). R language through a variety of packages can enable statistical analysis, data mining, and data visualization. Therefore, from the perspective of molecular biology, this study used bioinformatics analysis to explore the key genes and their functional modes of action, which may affect the disease development of DFU patients.
Two datasets, GSE68183 and GSE80178, containing DF-related gene-level detection data were obtained from the GEO database. Both of these were chip datasets that used the GPL16686 chip platform. GSE68183 included three DF and three NDF patients; while GSE80178 included six DFU patients, three DM patients, and three healthy people with NDM. The GEO2R tool of the GEO website was used respectively to screen out the top 250 DEGs and export the data from both datasets. A total of 322 DEGs were screened from the GSE80178 data set, including 47 up-regulated genes and 275 down-regulated genes. Furthermore, a total of 96 DEGs were screened from the GSE68183 dataset, including 40 up-regulated genes and 56 down-regulated genes. The intersection of the two datasets was then analyzed and six DEGs were obtained, including three up-regulated genes (BNIP3, HEPHL1, and KLK10) and three down-regulated genes (FRZB, KLHDC1, and NHLRC3).
Through GO function and PPI network analyses, two key genes of NHLRC3 and BNIP3 were obtained. The NHLRC3 and BNIP3 genes were retrieved using the Genecards database. NHLRC3 is a protein-coding gene associated with the innate immune system pathway; this gene encodes proteins containing NHL family repeats. Proteins containing mammalian NHL repeats may be involved in a variety of enzymatic processes, including protein modification by ubiquitination. Meanwhile, BNIP3 encodes a mitochondrial protein that contains the BH3 domain and acts as a proapoptotic factor. The encoded protein E1B 19 kDa protein interacts with the anti-apoptotic protein, BCL2. At present, there are many studies related to BNIP3, most of which are related to DM, and only a few on NHLRC3. Therefore, the BNIP3 gene was selected for further analysis.
In this study, the expression level of co-DEGs obtained from the GSE80178 dataset in each group was displayed using a heat map. We observed that there is a significant difference in the expression of BNIP3 between the groups, and there is a marked increase in the expression of BNIP3 in NDM, DM, and DFU patients. In addition, vascular disease is one of the main causes of DFU, and the DFU healing process also requires neovascularization. HUVECs have stem cell differentiation potential. In this study, HUVECs were cultured in medium containing glucose concentrations of 5.6, 11.2, 16.8, and 33.6 mmol/L for 24 and 72 h, respectively. The expression of the BNIP3 protein in HUVECs increased significantly with the increase in glucose concentration in the HG intervention group at 72 h. Compared with the 24 and 72 h groups, the expression of the BNIP3 protein increased considerably with the increased intervention duration of different HG concentrations. At present, the differential expression and role of BNIP3 in diabetes complications are mostly concentrated in diabetic kidney disease (DKD), diabetic cardiomyopathy (DCM), and vascular endothelial protection; however, there are no reports in DFU.
A study (17) has shown that the expressions of BNIP3 and Nip3-like protein X (NIX) in HG-induced mesangial cells are slightly increased, suggesting that the mesangial cells may try to activate mitochondrial phagocytosis by sensing HG-induced injury, but it is not enough to alleviate mitochondrial damage. Intervention with tilapia skin peptides can significantly up-regulate the protein expressions of BNIP3 and NIX. Furthermore, mitochondrial phagocytosis activity is enhanced, the HG-induced glomerular mesangial fibrosis is alleviated by removing the damaged mitochondria, and there was a renal protective effect on DKD. In addition, compared with non-diabetic mice, BNIP3 can be significantly induced in diabetic mice, and blocking the calcium-activated potassium channel (KCa3.1) can markedly reduce the up-regulation of BNIP3 expression in diabetic mice and improve the mitochondrial phagocytosis disorder, thereby alleviating diabetic renal fibrosis (18). The upregulation of BNIP3 expression in HG-induced mesangial cells and diabetic mouse kidneys was observed in the two abovementioned studies (17,18). Yet, the upregulation and downregulation of BNIP3 alleviated diabetic renal fibrosis following different interventions. However, the specific mechanism of this effect needs to be further studied and elucidated.
HG can also lead to the overexpression of BNIP3 in human renal tubular epithelial cells, resulting in decreased cell viability and increased apoptosis, while deletion of BNIP3 can improve this situation (19). Another study (20) showed that HG significantly increased the expression of BNIP3 in human renal tubular epithelial cells, while thioredoxin interacting protein (TXNIP) silencing weakened the expression of BNIP3 and eliminated glucose-induced collagen deposition and oxidative stress. In addition, the BNIP3 protein and messenger RNA (mRNA) levels were found to be significantly increased in HG-treated mouse proximal renal tubular cells and Leprdb/db (DB/dB) mice, and stanniocalcin-1 (STC-1) inhibited the expression of BNIP3 by activating the AMPK/Sirt3 pathway to eliminate HG-induced oxidative stress and cell apoptosis (21). Taken together, the findings reported in these studies indicate that BNIP3 is highly expressed in the presence of HG, and the down-regulation of BNIP3 in different ways could improve renal tubular cell viability and reduce cell apoptosis and oxidative stress. Oxidative stress and decreased cell viability of vascular endothelial cells are also involved in the progression of DFU. However, whether BNIP3 is differentially expressed in DFU and whether the same improvement can be observed through down-regulation of BNIP3 remains to be verified by further experiments. Studies on DCM found similar results: the expression level of BNIP3 in primary myocardial cells of diabetic rats increased by 10 times compared with the control group. BNIP3 overexpression of BNIP3 can reduce the mitochondrial membrane potential and induce cell apoptosis, and BNIP3 knockout reverses the effect on cell apoptosis (22).
In addition, diabetic patients are extremely vulnerable to hypoxic injury. Both HG and hypoxia lead to increased BNIP3 expression, which affects the cell viability of rat cardiomyocytes and activates apoptosis. The inhibition of hypoxia inducible factor (HIF-1α) and BNIP3 expressions can improve the survival of rat cardiomyocytes and effectively inhibit apoptosis (23). Most DFU patients also face local poor blood supply, local hypoxia in the wound, and an early increase in the expression of HIF-1α. Therefore, the incidence of differential expression and the role of BNIP3 in DFU requires more detailed studies. In db/db mice, BNIP3 is significantly up-regulated in HG-treated HUVECs and retinal vascular endothelial cells, and metformin can down-regulate the expression of BNIP3 by activating the hedgehog (Hh) pathway to protect the vascular endothelium (24). In conclusion, the differential expression of BNIP3 in DKD, DCM, and vascular endothelial protection may also be prevalent in DFU and exert a similar role, and the development of DFU may be inhibited to some extent by regulating its expression.
In this study, GO functional enrichment analysis showed six co-DEGs; BNIP3-related BPs were enriched in mitochondrial protein catabolism, negative regulation of mitochondrial fusion and mitochondrial membrane permeability, the granular enzyme-mediated apoptosis signaling pathways, the intrinsic apoptosis signaling pathway of anaerobic reaction, positive adjustment of necrotic cell death, autophagy cell death, and adipocyte differentiation. Furthermore, the BNIP3-related CCs were mainly in the mitochondrial membrane. GO functional analysis of BNIP3 and its interacting genes showed that the CC level was significantly enriched in the autophagosome membrane, the autophagosome, and the vacuolar membrane. At the MF level, the ubiquitin-binding protein ligase and ubiquitin-like protein ligase were notably enriched. Also, the BP level was significantly enriched in the response of cells to external stimuli, autophagy, and the utilization of the autophagy mechanism. The GO functional analysis suggested that BNIP3 was primarily enriched in the mitochondrial membrane and autophagosome membrane, exerting its role mainly through mitochondrial autophagy and apoptosis. BNIP3 is encoded by the BNIP3 gene on human chromosome 10Q26.3, with a molecular weight of 21.5kDa, and its peptide chain consists of 194 amino acids (25). It is a member of the BCL-2 family of cell death regulators but only contains the BH3 homologous domain (26,27). BNIP3 is widely expressed in a variety of cells and participates in cell functions through a variety of cell signaling pathways, including mitochondrial dysfunction, mitochondrial autophagy, and apoptosis (28-30).
KEGG pathway enrichment analysis further suggested that the role of BNIP3 was mainly related to mitochondrial autophagy and autophagy. Autophagy is primarily involved in the degradation of long-lived proteins and damaged organelles, and the mitochondria, peroxisome, or endoplasmic reticulum are separated from the cytoplasm by the two-membrane-structure autophagosomes. The autophagosomes then fuse with lysosomes, resulting in the destruction of their inclusions by hydrolases in lysosomes (31). Autophagy is generally non-specific but can degrade specific organelles in some cases, such as mitochondrial autophagy (32). When nutrients or oxygen are scarce, autophagy is rapidly upregulated; however, the protective or harmful effects of its regulation are unclear. Autophagy can be employed for cell survival by recovering fatty acids and amino acids in lipids and proteins in the absence of nutrients (33), and can also promote cell survival by removing protein aggregates and damaged mitochondria that may be harmful to cells (34). Conversely, autophagy has also been considered a mechanism of death in response to cardiac pressure overload and post-ischemia reperfusion (35). The function of BNIP3 can be regulated by modulating mitochondrial membrane potential, the permeability of mitochondrial intima, interaction with microtubule-associated protein light chain 3 (LC-3), as well as by HIF-1α, inflammatory response, and multiple signaling pathways. Cardiomyocytes overexpressing the BNIP3 gene induce mitochondrial membrane potential (δ ψ M) deletion and mitochondrial permeability transition pore (mPTP) opening, resulting in mitochondrial abnormality and cardiomyocyte death (36). BNIP3, as a transcription target responding to dysfunctional mitochondria, regulates mitochondrial autophagy mainly by interacting with LC-3 and its related molecular receptors (37). In hypoxia, HIF-1α can be upregulated to promote BNIP3 expression and limit reactive oxygen species (ROS) production through mitochondrial phagocytosis (38).
KEGG pathway enrichment analysis suggested that BNIP3 was mainly enriched in the FoxO signaling pathway. Both the PI3K/AKT signaling pathway and AMPK can inhibit FoxO3a activity, thereby down-regulating the abnormal BNIP3 and autophagy levels (39). Through the miRDB and TargetScan databases, we found that miRNA-182 may be involved in the targeted regulation of BNIP3. Numerous studies have confirmed the targeted regulatory relationship between miR-182 and BNIP3. For example, the downregulation of BNIP3 expression caused by miRNA-182-5P overexpression inhibits the apoptosis of mouse hippocampal neurons induced by oxygen-glucose deprivation/reoxygenation (40). Small molecule inducers of miR-182 reduce myocardial cell death caused by ischemia/reperfusion by down-regulating BNIP3 (41). Ligustrazine significantly increases miR-182-5P expression, decreases BNIP3, increased BCL-2 expression, inhibits ROS production and malondialdehyde levels, up-regulates superoxide dismutase, and protects retinal ganglion cells by inhibiting apoptosis and oxidative stress (42). In addition, miR-182-5P promotes the radiation resistance of nasopharyngeal carcinoma cells by down-regulating BNIP3 (43).
In this study, the BNIP3 gene was found to be highly expressed in DF and DFU, and its expression was significantly up-regulated in HG-treated HUVECs. BNIP3 is mainly involved in autophagy and mitochondrial autophagy. It can exert its functions by regulating the mitochondrial membrane potential, mitochondrial inner membrane permeability, and interaction with microtubule-associated protein light chain 3 (LC-3). It is believed that BNIP3 is regulated by the FoxO signaling pathway, and miR-182 may be involved in the targeted regulation of BNIP3. These findings may provide new molecular markers for disease severity and prognosis in DFU patients, and may also provide a potentially effective treatment strategy for DFU.
At the same time, there are also some limitations in this study that should be noted. Firstly, the data on gene expression levels in DFU and the number of datasets were lacking. Secondly, DFU patients were not included in the GSE68183 dataset and the sample size was small, meaning that the expression difference of differential genes in DF and NDF may not be as obvious as that between DFU and NDF, and the credibility of differential expression caused by the small sample size was insufficient. This research also lacks biological experimental studies and clinical parameters to support the above findings. In the future, biological and clinical experiments can be carried out to verify the differential expression, function, and regulation of BNIP3 in DFU, and determine whether the intervention of BNIP3 expression level is beneficial to the development of the disease.
Conclusions
In our study, bioinformatics analysis was used to screen out the differentially expressed BNIP3 gene in DFU from the GEO microarray public database, and its differential expression was verified by western blot assay. According to bioinformatics analysis, BNIP3 functions mainly by participating in autophagy, mitophagy, and the FoxO pathways, and miR-182 may be involved in the targeted regulation of BNIP3 expression. These findings may provide novel molecular markers for disease severity and prognosis in patients with DFU, and may also provide a potentially effective treatment strategy for these patients.
Acknowledgments
Funding: This research was sponsored by the Shanxi Provincial Natural Science Foundation of China (No. 201901D111434) and the National Natural Science Foundation of China (No. 32171339).
Footnote
Reporting Checklist: The authors have completed the MDAR reporting checklist. Available at https://atm.amegroups.com/article/view/10.21037/atm-22-6437/rc
Data Sharing Statement: Available at https://atm.amegroups.com/article/view/10.21037/atm-22-6437/dss
Conflicts of Interest: All authors have completed the ICMJE uniform disclosure form (available at https://atm.amegroups.com/article/view/10.21037/atm-22-6437/coif). The authors have no conflicts of interest to declare.
Ethical Statement: The authors are accountable for all aspects of the work, including ensuring that any questions related to the accuracy or integrity of any part of the work have been appropriately investigated and resolved. The study was conducted in accordance with the Declaration of Helsinki (as revised in 2013).
Open Access Statement: This is an Open Access article distributed in accordance with the Creative Commons Attribution-NonCommercial-NoDerivs 4.0 International License (CC BY-NC-ND 4.0), which permits the non-commercial replication and distribution of the article with the strict proviso that no changes or edits are made and the original work is properly cited (including links to both the formal publication through the relevant DOI and the license). See: https://creativecommons.org/licenses/by-nc-nd/4.0/.
References
- Barrett T, Wilhite SE, Ledoux P, et al. NCBI GEO: archive for functional genomics data sets--update. Nucleic Acids Res 2013;41:D991-5. [Crossref] [PubMed]
- Bowling FL, Rashid ST, Boulton AJ. Preventing and treating foot complications associated with diabetes mellitus. Nat Rev Endocrinol 2015;11:606-16. [Crossref] [PubMed]
- Chen G, Ray R, Dubik D, et al. The E1B 19K/Bcl-2-binding protein Nip3 is a dimeric mitochondrial protein that activates apoptosis. J Exp Med 1997;186:1975-83. [Crossref] [PubMed]
- Deng Z, Ou H, Ren F, et al. LncRNA SNHG14 promotes OGD/R-induced neuron injury by inducing excessive mitophagy via miR-182-5p/BINP3 axis in HT22 mouse hippocampal neuronal cells. Biol Res 2020;53:38. [Crossref] [PubMed]
- Huang C, Leavitt T, Bayer LR, et al. Effect of negative pressure wound therapy on wound healing. Curr Probl Surg 2014;51:301-31. [Crossref] [PubMed]
- Chen L, Zhang S, Da J, et al. A systematic review and meta-analysis of efficacy and safety of negative pressure wound therapy in the treatment of diabetic foot ulcer. Annals of palliative medicine 2021;10:10830-9. [Crossref] [PubMed]
- Dhingra A, Jayas R, Afshar P, et al. Ellagic acid antagonizes Bnip3-mediated mitochondrial injury and necrotic cell death of cardiac myocytes. Free Radic Biol Med 2017;112:411-22. [Crossref] [PubMed]
- Fordjour PA, Wang L, Gao H, et al. Targeting BNIP3 in inflammation-mediated heart failure: a novel concept in heart failure therapy. Heart Fail Rev 2016;21:489-97. [Crossref] [PubMed]
- Hanna RA, Quinsay MN, Orogo AM, et al. Microtubule-associated protein 1 light chain 3 (LC3) interacts with Bnip3 protein to selectively remove endoplasmic reticulum and mitochondria via autophagy. J Biol Chem 2012;287:19094-104. [Crossref] [PubMed]
- He W, Jin H, Liu Q, et al. miR 182 5p contributes to radioresistance in nasopharyngeal carcinoma by regulating BNIP3 expression. Mol Med Rep 2021;23:130. [Crossref] [PubMed]
- Huang C, Yi H, Shi Y, et al. KCa3.1 Mediates Dysregulation of Mitochondrial Quality Control in Diabetic Kidney Disease. Front Cell Dev Biol 2021;9:573814. [Crossref] [PubMed]
- Huang C, Zhang Y, Kelly DJ, et al. Thioredoxin interacting protein (TXNIP) regulates tubular autophagy and mitophagy in diabetic nephropathy through the mTOR signaling pathway. Sci Rep 2016;6:29196. [Crossref] [PubMed]
- Jin L, Zheng D, Yang G, et al. Tilapia Skin Peptides Ameliorate Diabetic Nephropathy in STZ-Induced Diabetic Rats and HG-Induced GMCs by Improving Mitochondrial Dysfunction. Mar Drugs 2020;18:363. [Crossref] [PubMed]
- Jin Q, Li R, Hu N, et al. DUSP1 alleviates cardiac ischemia/reperfusion injury by suppressing the Mff-required mitochondrial fission and Bnip3-related mitophagy via the JNK pathways. Redox Biol 2018;14:576-87. [Crossref] [PubMed]
- Kim I, Rodriguez-Enriquez S, Lemasters JJ. Selective degradation of mitochondria by mitophagy. Arch Biochem Biophys 2007;462:245-53. [Crossref] [PubMed]
- Kubasiak LA, Hernandez OM, Bishopric NH, et al. Hypoxia and acidosis activate cardiac myocyte death through the Bcl-2 family protein BNIP3. Proc Natl Acad Sci U S A 2002;99:12825-30. [Crossref] [PubMed]
- Kubli DA, Quinsay MN, Huang C, et al. Bnip3 functions as a mitochondrial sensor of oxidative stress during myocardial ischemia and reperfusion. Am J Physiol Heart Circ Physiol 2008;295:H2025-31. [Crossref] [PubMed]
- Lee SY, Lee S, Choi E, et al. Small molecule-mediated up-regulation of microRNA targeting a key cell death modulator BNIP3 improves cardiac function following ischemic injury. Sci Rep 2016;6:23472. [Crossref] [PubMed]
- Levine B, Klionsky DJ. Development by self-digestion: molecular mechanisms and biological functions of autophagy. Dev Cell 2004;6:463-77. [Crossref] [PubMed]
- Liao Y, Wang J, Jaehnig EJ, et al. WebGestalt 2019: gene set analysis toolkit with revamped UIs and APIs. Nucleic Acids Res 2019;47:W199-205. [Crossref] [PubMed]
- Liu SP, Shibu MA, Tsai FJ, et al. Tetramethylpyrazine reverses high-glucose induced hypoxic effects by negatively regulating HIF-1α induced BNIP3 expression to ameliorate H9c2 cardiomyoblast apoptosis. Nutr Metab (Lond) 2020;17:12. [Crossref] [PubMed]
- Liu Z, Liu H, Xiao L, et al. STC-1 ameliorates renal injury in diabetic nephropathy by inhibiting the expression of BNIP3 through the AMPK/SIRT3 pathway. Lab Invest 2019;99:684-97. [Crossref] [PubMed]
- Li X, Wang Q, Ren Y, et al. Tetramethylpyrazine protects retinal ganglion cells against H2O2 induced damage via the microRNA 182/mitochondrial pathway. Int J Mol Med 2019;44:503-12. [Crossref] [PubMed]
- Li Y, Zhi K, Han S, et al. TUG1 enhances high glucose-impaired endothelial progenitor cell function via miR-29c-3p/PDGF-BB/Wnt signaling. Stem Cell Res Ther 2020;11:441. [Crossref] [PubMed]
- Lv L, Li D, Tian F, et al. Silence of lncRNA GAS5 alleviates high glucose toxicity to human renal tubular epithelial HK-2 cells through regulation of miR-27a. Artif Cells Nanomed Biotechnol 2019;47:2205-12. [Crossref] [PubMed]
- Mammucari C, Schiaffino S, Sandri M. Downstream of Akt: FoxO3 and mTOR in the regulation of autophagy in skeletal muscle. Autophagy 2008;4:524-6. [Crossref] [PubMed]
- Matsui Y, Takagi H, Qu X, et al. Distinct roles of autophagy in the heart during ischemia and reperfusion: roles of AMP-activated protein kinase and Beclin 1 in mediating autophagy. Circ Res 2007;100:914-22. [Crossref] [PubMed]
- Nguyen TT, Ding D, Wolter WR, et al. Validation of Matrix Metalloproteinase-9 (MMP-9) as a Novel Target for Treatment of Diabetic Foot Ulcers in Humans and Discovery of a Potent and Selective Small-Molecule MMP-9 Inhibitor That Accelerates Healing. J Med Chem 2018;61:8825-37. [Crossref] [PubMed]
- Niu C, Chen Z, Kim KT, et al. Metformin alleviates hyperglycemia-induced endothelial impairment by downregulating autophagy via the Hedgehog pathway. Autophagy 2019;15:843-70. [Crossref] [PubMed]
- Otasek D, Morris JH, Bouças J, et al. Cytoscape Automation: empowering workflow-based network analysis. Genome Biol 2019;20:185. [Crossref] [PubMed]
- Park CW, Hong SM, Kim ES, et al. BNIP3 is degraded by ULK1-dependent autophagy via MTORC1 and AMPK. Autophagy 2013;9:345-60. [Crossref] [PubMed]
- Peng Y, Zhang G, Tang H, et al. Influence of SIRT1 polymorphisms for diabetic foot susceptibility and severity. Medicine (Baltimore) 2018;97:e11455. [Crossref] [PubMed]
- Pichu S, Sathiyamoorthy J, Vimalraj S, et al. Impact of lysyl oxidase (G473A) polymorphism on diabetic foot ulcers. Int J Biol Macromol 2017;103:242-7. [Crossref] [PubMed]
- Sinha R, van den Heuvel WJ, Arokiasamy P. Factors affecting quality of life in lower limb amputees. Prosthet Orthot Int 2011;35:90-6. [Crossref] [PubMed]
- Sowter HM, Ratcliffe PJ, Watson P, et al. HIF-1-dependent regulation of hypoxic induction of the cell death factors BNIP3 and NIX in human tumors. Cancer Res 2001;61:6669-73. [PubMed]
- Su N, Zhao N, Wang G, et al. Association of MCP-1 rs1024611 polymorphism with diabetic foot ulcers. Medicine (Baltimore) 2018;97:e11232. [Crossref] [PubMed]
- Tapp RJ, Shaw JE, de Courten MP, et al. Foot complications in Type 2 diabetes: an Australian population-based study. Diabet Med 2003;20:105-13. [Crossref] [PubMed]
- Teena R, Dhamodharan U, Jayasuriya R, et al. Analysis of the Exonic Single Nucleotide Polymorphism rs182428269 of the NRF2 Gene in Patients with Diabetic Foot Ulcer. Arch Med Res 2021;52:224-32. [Crossref] [PubMed]
- Webster KA, Graham RM, Bishopric NH. BNip3 and signal-specific programmed death in the heart. J Mol Cell Cardiol 2005;38:35-45. [Crossref] [PubMed]
- Woodworth-Hobbs ME, Hudson MB, Rahnert JA, et al. Docosahexaenoic acid prevents palmitate-induced activation of proteolytic systems in C2C12 myotubes. J Nutr Biochem 2014;25:868-74. [Crossref] [PubMed]
- Yuan C, Pu L, He Z, et al. BNIP3/Bcl-2-mediated apoptosis induced by cyclic tensile stretch in human cartilage endplate-derived stem cells. Exp Ther Med 2018;15:235-41. [PubMed]
- Zhang Y, Lazzarini PA, McPhail SM, et al. Global Disability Burdens of Diabetes-Related Lower-Extremity Complications in 1990 and 2016. Diabetes Care 2020;43:964-74. [Crossref] [PubMed]
- Zhou W, Yang J, Zhang DI, et al. Role of Bcl-2/adenovirus E1B 19 kDa-interacting protein 3 in myocardial cells in diabetes. Exp Ther Med 2015;10:67-73. [Crossref] [PubMed]
(English Language Editor: A. Kassem)