Screening and identification of susceptibility genes for osteosarcoma based on bioinformatics analysis
Highlight box
Key findings
• Changes in the ECM and growth factors play a key role in the development of OS.
What is known and what is new?
• Gene changes play an important role in the occurrence and development of various malignant tumors.
• The study analyzes the gene expression profile of OS using bioinformatics, and explore the pathogenesis of OS at the molecular level.
What is the implication, and what should change now?
• Changes in the ECM and growth factors play a key role in the development of OS. The leukocyte transendothelial migration pathway and the PI3K-AKT pathway are closely related to OS, and the related molecular mechanism is worthy of further study.
Introduction
Osteosarcoma (OS) is a serious public health issue (1). It derives from primitive transformed cells of mesenchymal origin, showing osteoblastic differentiation and producing malignant osteoid (2). OS is characterized by high malignancy and invasiveness, rapid growth, and early metastasis, leading to poor prognosis and high mortality. The main symptoms of OS are local pain and swelling, mainly in the long bones of the limbs, especially the knees. Nearly 20% of OS patients have distant metastases at initial diagnosis, 90% of which are lung metastases. Although the etiology and pathogenesis of OS are unclear, some studies have shown that it is closely related to genetic factors (3-5). In the field of OS research, the challenge is to elucidate its pathogenesis at the molecular level (6-10).
At present, the clinical treatment of OS is mostly chemotherapy, radiotherapy, and tumor resection, but about 50% of patients still have tumor recurrence and distal metastasis after surgery. The 5-year survival rate for patients with OS remains low. Some target molecules have been reported to play an important role in the progression of OS (11-13). There is an urgent need to find diagnostic or prognostic markers with high specificity and sensitivity for disease diagnosis and treatment, to provide a basis for the optimization of clinical treatment and improve patient survival rate. Greater attention is being given to epigenetics, DNA methylation, histone modification, and chromatin remodeling involved in the formation of tumors, so in-depth understanding of the genetic and molecular basis of OS will the provide a theoretical basis for molecular targeted therapy research (14).
In recent years, the use of high-throughput hybrid arrays and sequencing-based technologies has increased because they can measure the molecular abundance of messenger RNA (mRNA) and genomic DNA (15,16). Researchers increasingly expect high-throughput data sets to be published in the scientific literature, and there is an international effort to ensure microarray experimental results are properly interpreted and comparable to each other. The Gene Expression Omnibus (GEO), located in the National Institutes of Health (Bethesda, MD, USA) is a resource for storing and retrieving public high-throughput gene-expression and genomic hybridization data (17). It now stores inventory files from original research deposited by the scientific community, and data on more than 1 million samples are currently available in the public domain. GEO currently accepts data from a variety of assays, including serial analysis of gene expression (SAGE) and real-time polymerase chain reaction (RT-PCR) data in addition to some gene-expression matrix data, and data from various omics in the current field of disease research (18).
Susceptibility gene refers to the gene that can code genetic disease or obtain disease susceptibility under appropriate environmental stimulation, that is, the gene carried by genetically determined organisms prone to certain diseases or diseases. At present, studies have explored the role of differentially expressed genes (DEGs) in patients with OS, but there is a lack of research to explore the interaction between different genes. Therefore, we aimed to analyze gene expression profile chip data for OS, and the DEGs between OS and normal tissues were obtained by screening. In addition, DEGs Gene Ontology (GO) and Kyoto Encyclopedia of Genes and Genomes (KEGG) pathway enrichment analyses were performed to build a protein-protein interaction (PPI) network, for exploration of possible molecular mechanisms and potential therapeutic targets of OS. We present the following article in accordance with the TRIPOD reporting checklist (available at https://atm.amegroups.com/article/view/10.21037/atm-22-6369/rc).
Methods
Data download
The gene expression profile of microarray data GSE16088 was obtained from the GEO database (https://www.ncbi.nlm.nih.gov/geo/). The microarray expression extracted profiles from 17 samples, including 14 OS samples and 3 normal control tissue samples. In this study, Affymetrix Human Genome U133A was used to genomeu133a on the GPL96 platform (HG-U133A) array [transcript (gene) version], expression data type for expression profiling by array, Homosapiens between species. The study was conducted in accordance with the Declaration of Helsinki (as revised in 2013).
Data processing and DEGs analysis
The original data were analyzed by the affy software package in R language (R Foundation for Statistical Computing, Vienna, Austria). The original CEL file was processed by robust multiarray analysis (RMA) algorithm for background correction, bootstrap correction of the original data, and then transformed into a probe table reach matrix. Then, according to the corresponding file GPL96 platform R language hugene10sttranscriptcluster.db package to name a probe into gene; DEGs also met |log2fold change (log2FC)| >2 and P<0.01. Heat maps of the top 100 significantly DEGs (defined as |log2FC|) were drawn using the gplots: heatmap.2 toolkit to visualize the expression of each DEG in each sample.
GO and KEGG analyses
KEGG is a knowledge library that systematically analyzes gene functions and enzyme pathways and connects the information of genes with higher-order functional information. GO is a powerful tool for integrating genes and product characteristics from all species. It is mainly used to integrate proteome data of different species, classify different proteins, predict the function of specific protein domains, and identify genes involved in specific diseases. In this study, GO and KEGG pathway enrichment analyses were performed on DEGs using the enrich-plot package of R language, and P<0.01 and false discovery rate (FDR) <0.05 were set as the critical values of significant gene enrichment.
Weighted analysis and construction of central gene network map
Search Tool for the Retrieval of Interacting Genes/Proteins (STRING) is an online database that retrieves interactions between proteins. Network system cluster tree construction was conducted by dynamic shear method, and gene modules with height below 0.25 were merged.
Gene set enrichment analysis
The DEG list file was imported to the left functional area of the Database for Annotation, Visualization, and Integrated Discovery (DAVID) website (https://david.ncifcrf.gov/) for GO analysis of the genes, including molecular function (MF), biological process (BP), and cellular component (CC), and the specific functional categories and CCs involved in the DEGs were analyzed. Using the R language clusterProfiler package of variance analysis of enrichment of gene analysis and visualization, through the analysis of gene expression profile data, the results of the analysis of DEGs reveal which ones may be involved in signaling pathways, and reveal how well they are expressed in specific functional gene sets, and whether that expression is statistically significant.
Further analysis of the core genes
The 10 core genes obtained were further analyzed in depth, and the direct differential expression of these core genes in metastatic and non-metastatic OS patients within 5 years was extracted and compared in GSE21257.
Prognostic analysis
Of the 36 OS samples in the GSE39055 data set, 1 case was excluded, 35 cases were retained for survival analysis, and the expression of 10 core genes was extracted, as well as the clinical data of the 35 OS patients. Sangerbox online software (http://www.sangerbox.com/tool.html) was used to analyze the relationship between the 10 core genes and overall survival of OS patients. The 35 OS samples were divided into a high expression group and a low expression group according to the best isolation value of each core gene. Kaplan-Meier (K-M) survival curves were drawn, and P<0.05 was considered statistically significant.
Statistical analysis
Statistical data were analyzed by SPSS 23.0 software (IBM Corp., Armonk, NY, USA), quantitative data were represented as mean ± standard deviation, the independent sample t-test was applied for comparisons between groups, one-way analysis of variance (ANOVA) was used for multiple groups, and the least significant difference (LSD) t-test was used for pairwise comparisons between groups. Prism 7 statistical analysis was used for target genes (the 10 core genes), and one-way ANOVA test was used for comparison between groups. P<0.05 indicated that the differences were significantly different (two-tailed).
Results
Screening for DEGs
A volcano map was drawn (Figure 1), which showed that the data quality of all samples was evenly distributed. Moreover, the data of each group were closely distributed, and there was no overflow value. The gene expression of each sample was mapped onto a heat map (Figure 2), and the gene expression differences between groups were statistically significant (P<0.05).
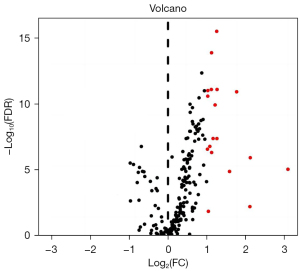
Weighted analysis and construction of central gene network map
A total of 4 gene co-expression modules were obtained after excluding representatives that failed to be assigned to any one known module. Among them, the blue module had the highest correlation with renal fibrosis, and the top 10 genes with the highest connectivity were selected from the blue module and blue module, respectively, and the interaction network was drawn, and these genes may play an important role in the disease process of fibrosis (Figure 3).
Analysis of subcomponent type
Consensus clustering cumulative distribution function (CDF) and relative change in the area under the CDF curve (CDF delta area) were used for analysis when cluster number varied from k−1 to k. The abscissa represents category number k, and the ordinate represents the relative change in the area. In the consistency analysis of the clustering results heatmap, rows and columns represented samples, and the different colors represent different types. In the expression heatmap of genes in different subgroups, red represented high expression, and blue represented low expression (Figure 4).
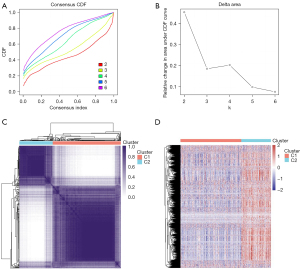
DEG analysis associated with OS prognosis
From the OS data, the heatmap of the DEG expression showed different colors representing the trend in gene expression in different tissues. The top 50 upregulated genes and top 50 downregulated genes are shown in Figure 5. DEGs were mainly involved in the regulation of leukocyte chemotaxis and migration, vascular development, and other BPs; mediation of receptor ligand activity, growth factor binding, growth factor activity, integrin binding, and other MFs; and were enriched in the extracellular matrix (ECM). The enriched KEGG signaling pathways were selected to demonstrate the primary biological actions of major potential mRNA. The abscissa indicates the gene ratio and the enriched pathways are presented in the ordinate. In the GO analysis of potential targets of mRNAs, the BP, CC, and MF of potential targets were clustered based on cluster Profiler package in R software version 3.18.0 (Figure 5).
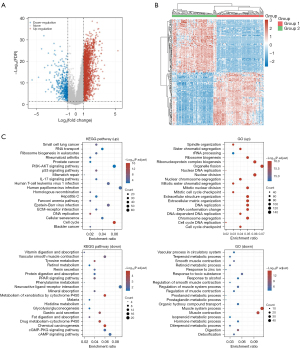
Further analysis of core genes
The 10 core genes identified were GAS6, IL-6, IGFBP4, IGFBP3, CYR61, LAMC1, FSTL1, FSTL3, APOE, and CSPP1 (Figure 6). Further analysis of the 10 core genes in the GSE21257 data set showed that the expressions of GAS6, IL-6, APOE, and IGFBP4 in the OS metastasis group were significantly lower than in the non-metastasis group (P values 0.0189, 0.0455, 0.0045, and 0.0068, respectively). The expression of IGFBP3 in the OS metastasis group was significantly higher than that in the non-metastasis group (P=0.0424). There was no significant difference in the expressions of CYR61, LAMC1, FSTL1, and FSTL3 between the two groups. Finally, we found that the expression of GAS6, IL-6, and IGFBP4 were always low in the development of OS.
Discussion
OS is a highly aggressive primary bone tumor with a high incidence in young adults (19). Despite the continuous improvement in neoadjuvant chemotherapy regimens, the survival rate of OS patients has not improved, and the 5-year survival rate is still below 20%. Metastasis is considered one of the main causes of mortality (20). In order to find potential drug therapeutic targets and new tumor markers, the pathogenesis of OS needs to be elucidated. With the rapid development of gene chip technology, it is being widely used in disease diagnosis, treatment, and prognosis assessment through bioinformatics (21). In this study, bioinformatics was used to screen DEGs in the GSE16088 gene chip dataset of OS and normal bone tissue along with GO analysis, KEGG rich set analysis, and PPI analysis of selected DEGs. The GO analysis showed that DEGs were mainly involved in mitotic mitosis, sister staining monosomy separation, mitotic microtubule cytoskeleton organization, organelle fission, and other BPs, and mediated histone kinase activity, chemokine receptor binding, microtubule movement, tubulin binding, and other MFs. DEGs gene products were mainly enriched in chromosome regions, spindle microtubules, kinetochores, and centromere regions (22). KEGG pathway enrichment analysis showed that DEGs were enriched in the PI3K-AKT, chemokine, p53, NF-κB, and other signaling pathways (23).
At present, it is a research hotspot to study the biological characteristics of various malignant tumors (24-27). Invasion and metastasis are important biological characteristics of OS, and one of the main reasons for the poor survival rate of OS patients. Therefore, it is urgent to thoroughly explore the molecular mechanism of tumor invasion and metastasis. KEGG pathway enrichment analysis has shown that the ECM-receptor interaction pathway, leukocyte transendothelial migration signaling pathway, PI3K-AKT signaling pathway, and actin cytoskeleton regulation pathways play certain roles in OS (28). Dysregulation of the PI3K-AKT signaling pathway is found in many diseases such as cancer, diabetes, cardiovascular diseases, and neurological diseases (29). Leukocyte transendothelial migration signaling pathway can activate the transcription factor 3 signal transduction pathway in OS cells, thereby promoting their proliferation and metastasis. In cancer, two mutations have been identified that enhance the intrinsic kinase activity of PI3K. Numerous studies have also shown that this signaling pathway also plays an important role in OS, and that the ECM-receptor interaction pathway also plays a certain role in OS (30,31). Therefore, the PI3K-AKT signaling pathway and ECM-receptor interaction pathway are expected to become potential drug therapeutic targets.
Limitations
We did not validate the expression of representative DEGs by experiments.
Conclusions
In this study, bioinformatic methods were used to analyze the relationship between OS-related genes, explore the genetic factors affecting the mechanism of OS, and provide a genetic basis for the prevention, diagnosis, and treatment of OS. Although the research basis of cancer gene therapy is broad, there are still problems and challenges.
Acknowledgments
Funding: None.
Footnote
Reporting Checklist: The authors have completed the TRIPOD reporting checklist. Available at https://atm.amegroups.com/article/view/10.21037/atm-22-6369/rc
Conflicts of Interest: All authors have completed the ICMJE uniform disclosure form (available at https://atm.amegroups.com/article/view/10.21037/atm-22-6369/coif). The authors have no conflicts of interest to declare.
Ethical Statement: The authors are accountable for all aspects of the work in ensuring that questions related to the accuracy or integrity of any part of the work are appropriately investigated and resolved. The study was conducted in accordance with the Declaration of Helsinki (as revised in 2013).
Open Access Statement: This is an Open Access article distributed in accordance with the Creative Commons Attribution-NonCommercial-NoDerivs 4.0 International License (CC BY-NC-ND 4.0), which permits the non-commercial replication and distribution of the article with the strict proviso that no changes or edits are made and the original work is properly cited (including links to both the formal publication through the relevant DOI and the license). See: https://creativecommons.org/licenses/by-nc-nd/4.0/.
References
- Rojas GA, Hubbard AK, Diessner BJ, et al. International trends in incidence of osteosarcoma (1988-2012). Int J Cancer 2021;149:1044-53. [Crossref] [PubMed]
- Mutsaers AJ, Walkley CR. Cells of origin in osteosarcoma: mesenchymal stem cells or osteoblast committed cells? Bone 2014;62:56-63. [Crossref] [PubMed]
- Yonemoto T, Tatezaki S, Ishii T, et al. Multiple primary cancers in patients with osteosarcoma: influence of anticancer drugs and genetic factors. Am J Clin Oncol 2004;27:220-4. [Crossref] [PubMed]
- Maximov VV, Aqeilan RI. Genetic factors conferring metastasis in osteosarcoma. Future Oncol 2016;12:1623-44. [Crossref] [PubMed]
- Liu W, Wang R, Zhang Y, et al. Whole-exome sequencing in osteosarcoma with distinct prognosis reveals disparate genetic heterogeneity. Cancer Genet 2021;256-257:149-57. [Crossref] [PubMed]
- Jiang H, Du H, Liu Y, et al. Identification of novel prognostic biomarkers for osteosarcoma: a bioinformatics analysis of differentially expressed genes in the mesenchymal stem cells from single-cell sequencing data set. Transl Cancer Res 2022;11:3841-52. [Crossref] [PubMed]
- Liang J, Chen J, Hua S, et al. Bioinformatics analysis of the key genes in osteosarcoma metastasis and immune invasion. Transl Pediatr 2022;11:1656-70. [Crossref] [PubMed]
- Lu D, Huang H, Zheng L, et al. Identification of Candidate MicroRNA-mRNA Subnetwork for Predicting the Osteosarcoma Progression by Bioinformatics Analysis. Comput Math Methods Med 2022;2022:1821233. [Crossref] [PubMed]
- Li G, Huang B, Wu H, et al. Development of novel gene signatures for the risk stratification of prognosis and diagnostic prediction of osteosarcoma patients using bioinformatics analysis. Transl Cancer Res 2022;11:2374-87. [Crossref] [PubMed]
- Liu W, Hao Y, Tian X, et al. The Role of NR4A1 in the Pathophysiology of Osteosarcoma: A Comprehensive Bioinformatics Analysis of the Single-Cell RNA Sequencing Dataset. Front Oncol 2022;12:879288. [Crossref] [PubMed]
- Chen C, Hou J, Tanner JJ, et al. Bioinformatics Methods for Mass Spectrometry-Based Proteomics Data Analysis. Int J Mol Sci 2020;21:2873. [Crossref] [PubMed]
- Huang X, Liu S, Wu L, et al. High Throughput Single Cell RNA Sequencing, Bioinformatics Analysis and Applications. Adv Exp Med Biol 2018;1068:33-43. [Crossref] [PubMed]
- Gong L, Zhang D, Dong Y, et al. Integrated Bioinformatics Analysis for Identificating the Therapeutic Targets of Aspirin in Small Cell Lung Cancer. J Biomed Inform 2018;88:20-8. [Crossref] [PubMed]
- Wu L, Zhong Y, Wu D, et al. Immunomodulatory Factor TIM3 of Cytolytic Active Genes Affected the Survival and Prognosis of Lung Adenocarcinoma Patients by Multi-Omics Analysis. Biomedicines 2022;10:2248. [Crossref] [PubMed]
- Wu L, Zheng Y, Ruan X, et al. Long-chain noncoding ribonucleic acids affect the survival and prognosis of patients with esophageal adenocarcinoma through the autophagy pathway: construction of a prognostic model. Anticancer Drugs 2022;33:e590-603. [Crossref] [PubMed]
- Pittard WS, Villaveces CK, Li S. A Bioinformatics Primer to Data Science, with Examples for Metabolomics. Methods Mol Biol 2020;2104:245-63. [Crossref] [PubMed]
- Costa MC, Gabriel AF, Enguita FJ. Bioinformatics Research Methodology of Non-coding RNAs in Cardiovascular Diseases. Adv Exp Med Biol 2020;1229:49-64. [Crossref] [PubMed]
- Noor Z, Ranganathan S. Bioinformatics approaches for improving seminal plasma proteome analysis. Theriogenology 2019;137:43-9. [Crossref] [PubMed]
- Oulas A, Minadakis G, Zachariou M, et al. Systems Bioinformatics: increasing precision of computational diagnostics and therapeutics through network-based approaches. Brief Bioinform 2019;20:806-24. [Crossref] [PubMed]
- Auslander N, Gussow AB, Koonin EV. Incorporating Machine Learning into Established Bioinformatics Frameworks. Int J Mol Sci 2021;22:2903. [Crossref] [PubMed]
- Rombo SE, Ursino D. Integrative bioinformatics and omics data source interoperability in the next-generation sequencing era-Editorial. Brief Bioinform 2021;22:1-2. [Crossref] [PubMed]
- Agyei D, Tsopmo A, Udenigwe CC. Bioinformatics and peptidomics approaches to the discovery and analysis of food-derived bioactive peptides. Anal Bioanal Chem 2018;410:3463-72. [Crossref] [PubMed]
- Sohail A, Arif F. Supervised and unsupervised algorithms for bioinformatics and data science. Prog Biophys Mol Biol 2020;151:14-22. [Crossref] [PubMed]
- Teng D, Xia S, Hu S, et al. miR-887-3p Inhibits the Progression of Colorectal Cancer via Downregulating DNMT1 Expression and Regulating P53 Expression. Comput Intell Neurosci 2022;2022:7179733. [Crossref] [PubMed]
- Chen Y, Wang J, Zhang X, et al. Correlation between apparent diffusion coefficient and pathological characteristics of patients with invasive breast cancer. Ann Transl Med 2021;9:143. [Crossref] [PubMed]
- Qi A, Li Y, Sun H, et al. Incidence and risk factors of sexual dysfunction in young breast cancer survivors. Ann Palliat Med 2021;10:4428-34. [Crossref] [PubMed]
- Qi A, Li Y, Yan S, et al. Effect of postoperative chemotherapy on blood glucose and lipid metabolism in patients with invasive breast cancer. Gland Surg 2021;10:1470-7. [Crossref] [PubMed]
- Chavali AK, Rhee SY. Bioinformatics tools for the identification of gene clusters that biosynthesize specialized metabolites. Brief Bioinform 2018;19:1022-34. [Crossref] [PubMed]
- Spurney R, Schwartz M, Gobble M, et al. Spatiotemporal Gene Expression Profiling and Network Inference: A Roadmap for Analysis, Visualization, and Key Gene Identification. Methods Mol Biol 2021;2328:47-65. [Crossref] [PubMed]
- Jiang B, Kang X, Zhao G, et al. miR-138 Reduces the Dysfunction of T Follicular Helper Cells in Osteosarcoma via the PI3K/Akt/mTOR Pathway by Targeting PDK1. Comput Math Methods Med 2021;2021:2895893. [Crossref] [PubMed]
- Lv M, Xu Q, Zhang B, et al. Imperatorin induces autophagy and G0/G1 phase arrest via PTEN-PI3K-AKT-mTOR/p21 signaling pathway in human osteosarcoma cells in vitro and in vivo. Cancer Cell Int 2021;21:689. [Crossref] [PubMed]
(English Language Editor: J. Jones)