Systematic pan-cancer analysis identifies cGAS as an immunological and prognostic biomarker
Highlight box
Key findings
• cGAS may be a novel biomarker for diagnosing and treating COVID-19-infected tumor patients.
What is known and what is new?
• Cancer patients are more susceptible to SARS-CoV-2. cGAS could be a potential SARS-CoV-2 sensor.
• This study discovered that SARS-CoV-2 infected cancer patients might experience changes in their tumor environment due to cGAS, increasing the susceptibility of patients with high cGAS-expressing tumors to COVID-19 and possibly worsening their prognosis. Furthermore, cGAS may be a novel biomarker for diagnosing and treating COVID-19-infected tumor patients.
What is the implication, and what should change now?
• The results of this study would be more convincing if combined with experimental validation, such as IHC or large prospective clinical studies. According to our findings, cGAS was both a protective and a risk factor in some tumors, and thus, its mechanisms of action need to be investigated further.
Introduction
The coronavirus disease 2019 (COVID-19) pandemic was triggered by the severe acute respiratory syndrome coronavirus 2 (SARS-CoV-2) virus, which had caused 475 million infections and 6.1 million deaths as of March 2022. SARS-CoV-2 infection can present with various manifestations, including asymptomatic, mild, or fatal (1). As with other pathogenic coronaviruses, such as Middle East respiratory syndrome (MERS) and severe acute respiratory syndrome (SARS), SARS-CoV-2 has been linked to human-bat cross-species transmission (2). The high transmission rate of SARS-CoV-2 in the human population is attributed to the increased affinity of the SARS-CoV-2 spike protein for the cyclic GMP-AMP synthase (cGAS) cellular receptor in humans (3-5).
In the early stages of RNA virus infection, viral genomic RNA is known to activate the RLR pathway when exposed to the cytoplasm of infected cells (6-10). Furthermore, there is growing evidence that RNA virus infection can activate cGAS/stimulator of interferon genes (STING) pathway via virus-cell membrane fusion and virus-induced mitochondrial damage (11,12). The virion-associated accessory proteins and viral structural proteins of SARS-CoV-2 are more likely to be involved in inhibiting the innate immune response of the host during the early stages of viral infection. The SARS-CoV-2 spike protein induces cell fusion and nuclear rupture, resulting in the leakage of DNA into the cytoplasm, and activates the DNA sensor protein cytoplasmic cGAS and its downstream effector STING, which stimulates the expression of interferon-β (IFN-β), revealing a previously unknown mechanism of IFN response to SARS-CoV-2 infection (1).
The protein-coding gene cGAS serves as a key DNA sensor; it binds directly to double-stranded DNA (dsDNA), causing liquid droplets to form where cGAS is activated, inducing the synthesis of 2',3'-cyclic GMP-AMP (2',3'-cGAMP), a second messenger that binds to and activates STING1, resulting in the production of type I IFN (13-18). In the cytoplasm, the presence of dsDNA is a key exogenous DNA sensor as a danger signal that induces an immune response (14). Antiviral activity is detected via dsDNA from DNA viruses in the cytoplasm (14).
Cancer patients who are immunocompromised are thought to be especially susceptible to the COVID-19 epidemic (19). According to recent clinical research (20), tumor patients infected with COVID-19 are more likely to have clinical complications and death than non-tumor patients. This mechanism may be that COVID-19 activates cGAS/STING and then activates its downstream noncanonical nuclear factor-κB (NF-κB) signal pathway to promote cancer cell metastasis (21). Although it has been reported that in pan carcinoma, the expression level of cGAS is related to methylation and infiltration of single types of immune cells (CD4, CD8 T cells and dendritic cell) (22). However, the relationship between cGAS gene and tumor immunotherapy related biomarkers and drug sensitivity has not been reported, and the abnormal expression of cGAS gene in human cancer has not been fully studied. Therefore, we applied a bioinformatics approach in this study to assess the relationship between cGAS expression, prognosis, tumor mutational burden (TMB), immune infiltration, immune neoantigens, microsatellite instability (MSI), and drug sensitivity in pan-cancer data. We present the following article in accordance with the MDAR reporting checklist (available at https://atm.amegroups.com/article/view/10.21037/atm-22-6318/rc).
Methods
cGAS sample information and pan-cancer expression analysis
The Cancer Genome Atlas (TCGA) (https://portal.gdc.cancer.gov/) and Genotype-Tissue Expression (GTEx) database (https://gtexportal.org/) databases were used to obtain the gene expression matrix and clinical data for both normal and tumor samples. Also, data on tumor cell line expression was downloaded from the Cancer Cell Line Encyclopedia (CCLE) database (https://portals.broadinstitute.org/). The study was conducted in accordance with the Declaration of Helsinki (as revised in 2013).
Pan-cancer prognostic analysis of cGAS expression
Kaplan-Meier (KM) forest plots (https://kmplot.com/analysis/) were constructed to illustrate the associations between cGAS expression and patient outcomes, including overall survival (OS). The log-rank P values, hazard ratios (HRs), and 95% confidence intervals (CIs) were calculated using univariate survival analysis.
cGAS genomic alterations in pan-cancer
TMB refers to the total number of non-synonymous mutations found in a tumor genome’s coding regions (23). In patients with higher TMB, immunotherapy [immune checkpoint inhibitors (ICIs)] is more effective (24,25).
The data were downloaded from the University of California, Santa Cruz (UCSC) Xena, and the relationship between cGAS expression and the TMB was investigated using Spearman’s correlation. The cBioPortal database (https://www.cbioportal.org/) was used to analyze gene changes in cGAS in the pan-cancer dataset. The “oncoprint”, “cancer type summary”, and “mutations” modules provided the cGAS gene alteration and mutation site information.
Analysis of mismatch repair (MMR) and MSI in cancer
The process of repairing mismatches within cells is known as MMR. When key genes are rendered inactive due to this process, errors in DNA replication cannot be corrected, which leads to numerous somatic mutations. We investigated the relationship between cGAS expression and several essential MMR genes, including MutL homologs (MLH1) and MutS homologs (MSH2, MSH6), as well as increased post-meiotic segregation (PMS2) and the epithelial cell adhesion molecule (EPCAM). MSI, another immunotherapy biomarker, is an inherited hypermutation state caused by MMR gene inactivation (26,27). Tumor data were obtained from UCSC Xena, and the relationship between cGAS expression and MSI was examined using Spearman’s correlation.
Association analysis of cGAS and methyltransferases
DNA methylation describes a chemical modification of DNA that can alter genetic properties without changing the DNA sequence. The relationship between cGAS levels and the expression of the four methyltransferases was investigated using the ggplot data visualization tool. The correlations were considered significant at P<0.05 and R>0.20.
Relationship between cGAS expression and immune cell infiltration
We also used the expression data (ESTIMATE) to estimate stromal and immune cells in malignant tissue, generating three scores—stromal score, immune score, and estimated score—representing the level of immune cell infiltration in the tumor, stromal cells, and tumor purity tissue (28). Next, we explored the relationship between cGAS expression and the ESTIMATE score.
Association analysis of cGAS with immune neoantigens and immune checkpoint genes
Neoantigen is a new antigen encoded by tumor cell mutation gene. It is a new abnormal protein produced by gene point mutation, deletion mutation and gene fusion that is different from the protein expressed by normal cells. Neoantigens were ranked according to their antigenicity index value, variant allele frequency, and docking affinity score, and the number of neoantigens present in each tumor sample was determined independently using a tool similar to ScanNeo (29). Additionally, we extracted the immune checkpoint genes to examine the relationship between their levels of expression and cGAS expression to analyze the relationship between immune checkpoint genes and cGAS expression. A significant positive correlation was shown by P<0.05 and R>0.20.
Subcellular localization and protein expression of cGAS
Retrieve the microarray data set from GEO under the number of accesses of GSE30589. These microarray data are generated by the Affymetrix GPL570 platform.
Furthermore, the distribution and subcellular localization of cGAS protein expression were identified using immunofluorescence staining based on the Human Protein Atlas (HPA) (https://www.proteinatlas.org/) database. Meanwhile, the Clinical Proteome Tumor Analysis Consortium and the HPA databases were used to analyze the protein-level expression of cGAS in various tumors.
Genes, disease network, enrichment analysis
GeneMANIA (http://www.genemania.org) was an online research tool, which imported the cGAS gene and presented the protein expression and inheritance in the network (30). The OpenTarget platform was used to conduct genetic association-based cGAS gene-disease network analysis. To investigate the potential biological functions of cGAS-interacting proteins and co-expressed genes in pan-cancer, Gene Ontology (GO) enrichment analysis, including molecular functions (MFs), cellular components (CCs), and biological processes (BPs), was employed to determine the enriched genes. The “ClusterProfiler” package was used to perform both the GO and Kyoto Encyclopedia of Genes and Genomes (KEGG) analyses.
Toll-like receptor (TLR) gene drug sensitivity analysis
Gene and drug susceptibility studies in the NCI-60 cell line were available via the Cell Miner Resource Collection (https://discover.nci.nih.gov/cellminer/) (31,32). Cell sensitivity to a drug was indicated by the z-score in the compound activity profile; the drug’s anticancer activity increased as the z-score value increased. The relationship between the cGAS gene expression levels and the z-score for each chemical was ascertained using Pearson correlation analysis. It must be emphasized that only Food and Drug Administration (FDA)-approved medications and substances that have undergone clinical validation were included in the correlation analysis. The identified molecular structures of drugs were determined using the PubChem database (https://pubchem.ncbi.nlm.nih.gov/).
Statistical analyses
Use R for all statistical analyses (https://www.rproject.org/). Univariate Cox regression analysis was used to examine the relationship between cGAS expression and cancer prognosis. KM survival analysis was conducted using survival and survminer packages. Spearman correlation test was used to infer the correlation between the two parameters. Student’s t-test and Wilcoxon test were used for comparison between the two groups, and one-way analysis of variance (ANOVA) and Kruskal-Wallis test were used for comparison between three or more groups. Bilateral P value <0.05 is statistically significant.
Results
Differential expression analysis of cGAS in pan-cancer and normal tissues
In this investigation, we used the GTEx database to identify the human cGAS expression in various tissues and conducted a systematic analysis of cGAS expression in pan-cancer (Figure 1A). Next, cGAS expression levels were measured in 21 different human cancer cell lines based on the CCLE repository (Figure 1B). The specificity of cGAS expression was later demonstrated by findings from TCGA database, which showed variations in cGAS expression levels in tumors and nearby normal tissues within a single tumor sample (Figure 1C). We then combined the normal and tumor tissue data from TCGA and GTEx databases to assess the differential expression of cGAS in 33 cancer types, avoiding errors caused by TCGA’s small sample size of normal tissues (Figure 1D). With the exception of adrenocortical carcinoma (ACC), kidney chromophobe (KICH), prostate adenocarcinoma (PRAD), and thymoma (THYM), this analysis revealed that cGAS was abnormally overexpressed in cancer tissues compared to normal tissues.
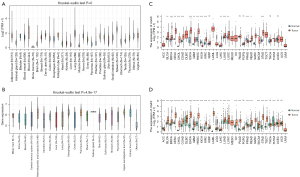
Pan-cancer analysis of the prognostic value of cGAS expression
Next, we performed a univariate Cox regression analysis to examine the relationship between cGAS expression and cancer prognosis. The cGAS expression levels were used to divide each type of cancer case into two subgroups. According to the forest plots of 33 tumors, cGAS expression had a significant impact on OS in patients with ACC, kidney renal clear cell carcinoma (KIRC), kidney renal papillary cell carcinoma (KIRP), brain lower grade glioma (LGG), liver hepatocellular carcinoma (LIHC), mesothelioma (MESO), pancreatic adenocarcinoma (PAAD), skin cutaneous melanoma (SKCM), and uveal melanoma (UVM). The findings also revealed that, with the exception of SKCM, the cGAS expressions of most tumor types were primarily correlated with a poor prognosis (Figure 2A).
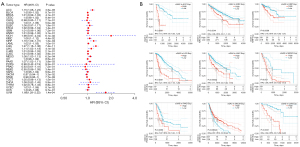
Using the KM plotter portal and the log-rank method (Figure 2B), we further evaluated the relationship between cGAS expression levels and patient outcomes. These results indicated that significantly worse OS was associated with a high expression of cGAS in patients with ACC (HR =1.15, P<0.0001), KIRC (HR =1.06, P=0.0044), KIRP (HR =1.19, P=0.0089), LGG (HR =1.27, P<0.0001), LIHC (HR =1.11, P=0.00022), PAAD (HR =1.07, P=0.0065), and UVM (HR =1.69, P<0.0001). However, higher cGAS levels suggested that SKCM had a better prognosis (HR =0.97, P=0.00042). According to the aforementioned data, a worse prognosis in various tumors is predicted by higher cGAS expression levels in addition to SKCM. Overall, these findings implied that cGAS may be a prognostic biomarker linked to OS in cancer patients.
Genetic variation analysis of cGAS in pan-cancer
We also investigated the correlation between the TMB and cGAS expression in cancer. Although the cGAS messenger RNA (mRNA) expression was negatively correlated with the TMB in LIHC, PRAD, thyroid carcinoma (THCA), and UVM, it was positively correlated with the TMB in bladder urothelial carcinoma (BLCA), breast invasive carcinoma (BRCA), colon adenocarcinoma (COAD), LGG, lung adenocarcinoma (LUAD), ovarian serous cystadenocarcinoma (OV), PAAD, sarcoma (SARC), stomach adenocarcinoma (STAD), THYM, and uterine carcinosarcoma (UCS) (Figure 3A). Gene mutations were then analyzed using the cBioPortal database. It was found that among pan-cancer patients, the gene alteration rates of cGAS were the highest among malignancies including UVM, prostate adenocarcinoma, LIHC, and uterine corpus endometrial, with deep deletion being the main alteration type (Figure 3B).
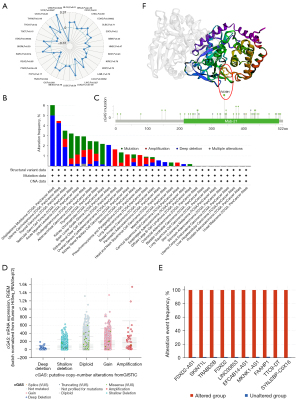
Additionally, we also determined the kind, location, and quantity of cGAS gene modifications (Figure 3C). cGAS missense was the predominant type of alteration. The most common putative copy number alterations in cGAS were diploid, gain, and shallow deletion (Figure 3D). Gene alterations were more frequent in the altered group than in the unaltered group in the following areas: FOXD2-AS1, SKINT1L, TRABD2B, FOXD2, LINC00853, EFCAB14-AS1, MKNK1-AS1, FAAHP1, TTC9-DT, and SYNJ2BP-COX16 (Figure 3E). We detected changes in R339H in 6 patients and obtained three-dimensional structural map of cGAS at the 339 mutation site (Figure 3F).
Correlation between cGAS expression and MMR/MSI in cancer
Microsatellites are straightforward repeats of nucleotide bases that cause mistakes in DNA replication, which can be detected and corrected by MMR genes. Microsatellite mutations can occur in tumors lacking the MMR system, resulting in high levels of MSI, which then induce the accumulation of cancer-related gene mutational burdens and worsening of the TMB (33). We examined the relationship between cGAS expression and a number of crucial MMR genes. With the exception of esophageal carcinoma (ESCA), lung squamous cell carcinoma (LUSC), and UCS, almost all of the 33 cancers showed that the cGAS expression was strongly correlated with MMR genes (Figure 4A). Our study also evaluated the relationship between MSI and cGAS expression as another biomarker related to ICI response (Figure 4B). According to our analysis, cGAS expression and MSI were positively correlated in BRCA, COAD, and UCS but negatively correlated in cholangiocarcinoma (CHOL), lymphoid neoplasm diffuse large B-cell lymphoma (DLBC), PAAD, PRAD, and SKCM.
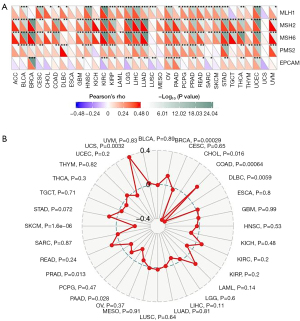
cGAS influences DNA MMR genes and methyltransferase expression in pan-cancer
The covalent attachment of methyl groups to DNA is known as DNA methylation via DNA methyltransferase (DNMT) to the cytosine 5' carbon site in the CpG dinucleotide of the genome. We visually analyzed the correlation between cGAS content and the expression of the four methyltransferases and found that most cancers had a positive relationship with cGAS content (Figure 5). These findings implied that cGAS may control tumorigenesis by altering the epigenetic conditions and the development of human pan-cancer.
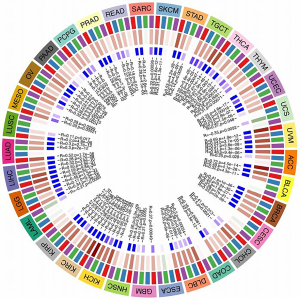
Correlation between cGAS and the level of immune infiltration
Using the ESTIMATE method, we separately calculated the immune, stroma, and estimated scores. Next, we explored the relationship between pan-cancer cGAS expression and the ESTIMATE score. Figure 6 demonstrated a significant correlation between cGAS expression and the estimated scores for the majority of cancers. These findings implied that as cGAS expression increases, stromal or immune cells become more prevalent and the tumor’s purity declines.
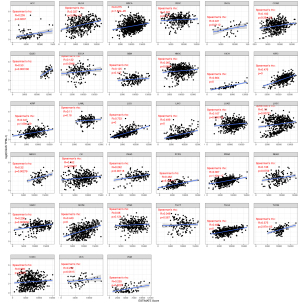
cGAS expression is associated with immune neoantigens and immune checkpoint genes
We examined data from more than 40 immune checkpoint genes that are frequently found in various malignancies (Figure 7A) to examine the connection between cGAS content and checkpoint gene expression. According to the data, there was a positive correlation between cGAS content in malignant tumors and immune checkpoint genes such as BRCA, KICH, KIRC, LGG, LIHC, THCA, and UVM. We also discovered that cGAS controls the expression of a large number of immune checkpoint genes, which are essential for modifying tumor immunity. To determine whether cGAS content and their abundance are related, the number of these neoantigens was independently counted in each tumor: OV (R=0.214, P=0.05), LUAD (R=0.262, P=0.05), BRCA (R=0.122, P=0.05), and PRAD (R=−0.127, P=0.05) (Figure 7B).
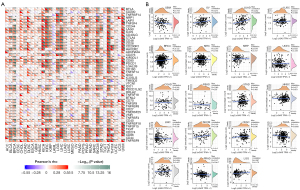
Intracellular localization and protein expression of cGAS
We then examined the subcellular distribution of cGAS in A-431, U-2 OS, and U-251 MG cells by immunofluorescence staining in the endoplasmic reticulum (ER) and nucleus based on the HPA database to ascertain the intracellular localization of cGAS. Figure 8A demonstrates that in the A-431, U-2 OS, and U-251 MG cells, cGAS was co-localized with 4',6-diamidino-2-phenylindole (DAPI)-labeled nuclei, indicating that cGAS was primarily found in the nucleoplasm. Additionally, we obtained the immunohistochemistry (IHC) results from the HPA database and contrasted them with the cGAS gene expression data obtained from TCGA to evaluate cGAS expression at the protein level. As seen in Figure 8B, the data analysis outcomes of the two databases were comparable. The amount of cGAS IHC staining in normal breast, liver, and lung tissue was minimal, whereas the amount in tumor tissue was more than moderate.
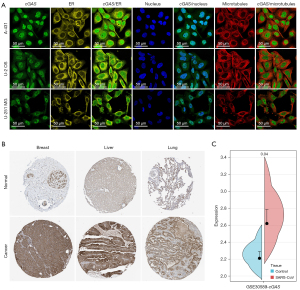
Additionally, it was important to research the modifications of cGAS in tumors following SARS-CoV-2 infection. Changes in cGAS expression following SARS-CoV infection of cells or animals can be used as a reference for SARS-CoV-2, as SARS-CoV-2 and SARS-CoV are highly homologous (2). We then analyzed the cGAS expression changes in SARS-CoV-infected Vero E6 cells using the GSE30589 dataset. These findings demonstrated that Vero E6 cells had higher levels of cGAS expression than the control group (Figure 8C). Based on this finding, cGAS expression may increase following SARS-CoV-2 infection.
Gene, disease network, and functional enrichment analysis
To investigate the potential relationship between cGAS genes, we built a gene-gene network using the GeneMANIA database. As shown in Figure 9A, the gene of cGAS were surrounded by 20 nodes, which shared protein domains, genetic interactions, colocalization, coexpression, and coexpression predictions with cGAS. The correlations between STING1, PQBP1, and BLVRA were among the strongest.
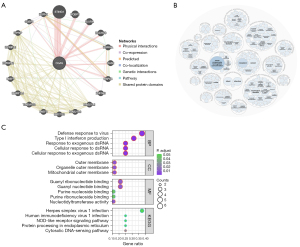
Subsequently, we investigated cGAS gene-related diseases using the OpenTarget database, and the findings revealed that cGAS was linked to immune system diseases, cancers or benign tumors, as well as hereditary, familial, or congenital diseases (Figure 9B). According to the GO and KEGG enrichment analysis results of cGAS interacting genes (Figure 9C), the predominant BPs included the regulation of cellular response to exogenous double-stranded RNA (dsRNA), the cellular response to dsRNA, the viral defense response, the response to exogenous dsRNA, and type I IFN production. Also, MFs were significantly enriched in nucleotidyltransferase activity, guanyl ribonucleotide binding, guanyl nucleotide binding, purine ribonucleoside binding, and purine nucleoside binding. CCs were typically found in the mitochondrial outer membrane, organelle outer membrane, and outer membrane. KEGG enrichment analysis indicated that cGAS mutual genes were significantly enriched in the DNA-sensing pathway of the cytosol, Herpes simplex virus 1 infection, ER protein processing, NOD-like receptor signaling pathway, and human immunodeficiency virus 1 infection.
Drug sensitivity analysis
The drug sensitivity (z-score) data from the Cell Miner database and cGAS were used for the correlation analysis. Figure 10 shows the results of the 11 highest correlation coefficients, sorted according to the P value. A previous studies has shown that cGAS expression levels alter tumor cell sensitivity to certain drugs. Patients with high cGAS expression were found to be more sensitive to curcumin, alectinib, fludarabine, and 6-thioguanine, implying that patients with high cGAS expression may be more likely to receive antitumor therapy (34). Yet, the cGAS expression levels were negatively correlated with the sensitivity of mithramycin, MI-219, AFP464, aminoflavone, kahalide F, AT-13387, and doxorubicin, indicating that the risk of drug resistance increases with the elevation of cGAS expression levels.
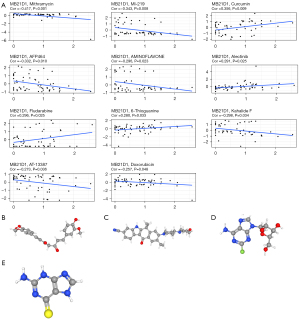
Discussion
COVID-19 is a global epidemic with no effective treatment at present (35). Smokers, obese people, and cancer patients are more likely to contract SARS-CoV-2 and have a worse prognosis (20,36-40). Understanding other potential SARS-CoV-2 sensors/receptors may help to offer new insights and identify potential therapeutic targets to develop better treatment options for COVID-19 (41-43). cGAS is a key DNA sensor that activates STING1 to cause type I IFN production (13-18), and the varicella-zoster virus ORF9 exerts an antagonistic effect on the cGAS DNA sensor (44). As a result, cGAS is a key factor in COVID-19 infection and progression, and it may be a potential new target for effective COVID-19 treatment.
At present, the study of cGAS gene in pancarcinoma is not sufficient.. Our analyses of the GTEx, CCLE, and TCGA databases confirmed that cGAS was overexpressed in most cancers compared to the adjacent normal tissues, and we demonstrated that cGAS played a significant role as a biomarker in various cancers, which was consistent with the findings of previous studies (45,46). Yet, the upstream factors regulating cGAS expression, as well as the reasons for cGAS’s persistent upregulation in various types of cancer, remain unknown and require further investigation. We discovered that cGAS was overexpressed in various cancers and confirmed this finding at the protein level. Furthermore, cGAS expression was associated with the prognosis of certain tumor types. In patients with ACC, KIRC, KIRP, LGG, LIHC, MESO, PAAD, and UVM, higher cGAS was linked to a poor prognosis. This discovery had never been reported before, highlighting the uniqueness of our study, which showed that the prognostic and discriminative value of cGAS expression in cancer was significant.
We also examined the correlation between cGAS and the TMB, MSI, MMR gene, and DNMTs to further explore the potential mechanism of the relationship between cGAS and cancer. MSI is a molecular fingerprint that develops as a result of MMR gene mutation (47,48). According to new evidence, most tumors with MSI-high (MSI-H)/deficiency in MMR (dMMR) status have a high TMB (23,49). The TMB status is becoming more widely recognized as a promising pan-cancer biomarker for predicting the efficacy of immune checkpoint blockade (ICB) therapy (50). MMR has received considerable attention as an important factor in genome stability and integrity (51,52). Furthermore, the TMB and MSI are new immunotherapy susceptibility predictors (33,53,54). These characteristics are associated with increased neoantigens, which affect tumor-infiltrating lymphocytes and the response to ICB, and thus, independently predict the immunotherapy response (55-57). At the pan-cancer level, our findings showed more correlations between cGAS expression and MSI/TMB in a variety of other cancer types.
However, in some cancers, cGAS expression was found to be inconsistently correlated with MSI and TMB. The association between MSI and TMB is complicated by other characteristics, which may explain why studies have shown a higher TMB in tumors with MSI-H status. MSI and TMB status must be integrated to predict the response to ICB reports. Secondly, the use of different datasets and the uniqueness of each data collection method might have resulted in disparities between the associations of cGAS with MSI and TMB in the same cancer type. In addition to genetic mutations, epigenetic changes have a significant impact on tumor growth, proliferation, metastasis, and immunosuppression.
One type of epigenetic regulation is DNA methylation. Abnormal DNA methylation levels have been linked to tumorigenesis and immune evasion in cancer (58-60). Our study discovered some positive and negative correlations between the expressions of DNMTs and cGAS in various cancer types, implying that DNA methylation might also play a role in cGAS regulation. This mechanism reduced tumor suppression by hypermethylating immune genes, leading to tumorigenesis and immunosuppression due to DNA hypomethylation (61,62). Possible strategies for targeting these checkpoints with methylation modulators or combining methylation modulators with ICBs have been proposed to improve the response rates. In summary, different methylation patterns regulate different types of tumors and their immune status, which is highly complex and would require more in-depth research in the future.
SARS-CoV-2 is highly homologous to SARS-CoV (2). As a result, we used the GSE30589 dataset to investigate the cGAS changes in Vero E6 cells infected with SARS-CoV. The findings revealed that cGAS expression increased following SARS-CoV infection, which suggested that cGAS levels might be elevated in tumor tissue following SARS-CoV-2 infection.
cGAS expression was linked to MMR genes in almost all of the cancers examined in this study. Furthermore, cGAS was also linked to TMB and MSI in certain types of cancer. We then compared the association between cGAS expression with multiple checkpoint markers in different cancer types. cGAS was found to be highly positively correlated with almost all checkpoint genes in the majority of cancers, implying that cGAS might play an important role in tumor immunity and might be a promising candidate for immune-targeted therapy. These findings could help researchers better understand the potential role of cGAS in tumor immunology.
Immune cell function continuously promoted the body’s antiviral and antitumor BPs. Lymphopenia was identified by previous research as an indicator in patients with COVID-19 and other cancers (63,64). Moreover, immunosuppression and a weakened immune system were major contributors to the severe disease course and high mortality rate of COVID-19 among cancer patients (65).
By binding to PD-1, PD-L1 inhibits T-cell proliferation and cytokine secretion at a given T-cell receptor attack threshold, resulting in immune tolerance and impaired T-cell immune function (66,67). LAG3 is a T-cell negative regulator; previous research has shown that blocking LAG3 can increase cytotoxic T-lymphocyte activity. Also, cGAS expression was found to be significantly correlated with PD-L1 function, and cGAS is associated with PDCD1, which encodes the PD-1 protein. LAG3 inhibition combined with PD-1 inhibition slows tumor progression and increases regression (68,69). We discovered that cGAS levels were significantly related to LAG3 expression in various cancers. Similar expression patterns of cGAS, PD-L1, and LAG3 in tumors suggested that these proteins promote tumor aggressiveness via a common cascade.
To our knowledge, this was the first pan-cancer analysis of cGAS combined with subcellular localization, protein expression and drug sensitivity, which showed that cGAS played a significant role in tumorigenesis and progression and is a promising potential marker for specific cancers. Moreover, this study not only settled the debate regarding whether cGAS was overexpressed or underexpressed in cancer but also revealed that cGAS plays a non-negligible role in tumor immunity, paving the way forward for tumor research. Nevertheless, a few limitations were present in this study that should be noted. Firstly, since we did not collect samples from patients with COVID-19 and cancer, we could not directly determine whether these cGAS patients had a poor prognosis. Secondly, more research is needed to confirm the underlying mechanisms of cGAS in various cancers. Our results would be more convincing if combined with experimental validation, such as IHC or large prospective clinical studies. Finally, according to our findings, cGAS was both a protective and risk factor in some tumors, and thus, the mechanism of action still needs to be investigated further.
Conclusions
This study demonstrated that cGAS was associated with COVID-19 and was a marker of multiple cancers. We also found that it was differentially expressed in different human cancers, and was strongly associated with poor prognosis in various malignancies. Additionally, we observed that the expression of cGAS in pan-cancer was dysregulated by TMB, MSI, MMR, and DNA methylation. In conclusion, cGAS might represent a novel approach for the detection and treatment of cancer.
Acknowledgments
The authors thank reviewers for helpful comments on the manuscript.
Funding: This study was supported by funding from the National Natural Science Foundation of China (No. 82002497); the Science and Technology Program of Fujian Province (Nos. 2020J011125, 2021J01442).
Footnote
Reporting Checklist: The authors have completed the MDAR reporting checklist. Available at https://atm.amegroups.com/article/view/10.21037/atm-22-6318/rc
Conflicts of Interest: All authors have completed the ICMJE uniform disclosure form (available at https://atm.amegroups.com/article/view/10.21037/atm-22-6318/coif). The authors have no conflicts of interest to declare.
Ethical Statement: The authors are accountable for all aspects of the work in ensuring that questions related to the accuracy or integrity of any part of the work are appropriately investigated and resolved. The study was conducted in accordance with the Declaration of Helsinki (as revised in 2013).
Open Access Statement: This is an Open Access article distributed in accordance with the Creative Commons Attribution-NonCommercial-NoDerivs 4.0 International License (CC BY-NC-ND 4.0), which permits the non-commercial replication and distribution of the article with the strict proviso that no changes or edits are made and the original work is properly cited (including links to both the formal publication through the relevant DOI and the license). See: https://creativecommons.org/licenses/by-nc-nd/4.0/.
References
- Liu X, Wei L, Xu F, et al. SARS-CoV-2 spike protein-induced cell fusion activates the cGAS-STING pathway and the interferon response. Sci Signal 2022;15:eabg8744. [Crossref] [PubMed]
- Zhou P, Yang XL, Wang XG, et al. A pneumonia outbreak associated with a new coronavirus of probable bat origin. Nature 2020;579:270-3. [Crossref] [PubMed]
- Lan J, Ge J, Yu J, et al. Structure of the SARS-CoV-2 spike receptor-binding domain bound to the ACE2 receptor. Nature 2020;581:215-20. [Crossref] [PubMed]
- Shang J, Wan Y, Luo C, et al. Cell entry mechanisms of SARS-CoV-2. Proc Natl Acad Sci U S A 2020;117:11727-34. [Crossref] [PubMed]
- Yan R, Zhang Y, Li Y, et al. Structural basis for the recognition of SARS-CoV-2 by full-length human ACE2. Science 2020;367:1444-8. [Crossref] [PubMed]
- Ishikawa H, Barber GN. STING is an endoplasmic reticulum adaptor that facilitates innate immune signalling. Nature 2008;455:674-8. [Crossref] [PubMed]
- Streicher F, Jouvenet N. Stimulation of Innate Immunity by Host and Viral RNAs. Trends Immunol 2019;40:1134-48. [Crossref] [PubMed]
- Brubaker SW, Bonham KS, Zanoni I, et al. Innate immune pattern recognition: a cell biological perspective. Annu Rev Immunol 2015;33:257-90. [Crossref] [PubMed]
- Holm CK, Rahbek SH, Gad HH, et al. Influenza A virus targets a cGAS-independent STING pathway that controls enveloped RNA viruses. Nat Commun 2016;7:10680. [Crossref] [PubMed]
- Holm CK, Jensen SB, Jakobsen MR, et al. Virus-cell fusion as a trigger of innate immunity dependent on the adaptor STING. Nat Immunol 2012;13:737-43. [Crossref] [PubMed]
- Rehwinkel J, Gack MU. RIG-I-like receptors: their regulation and roles in RNA sensing. Nat Rev Immunol 2020;20:537-51. [Crossref] [PubMed]
- Ablasser A, Hur S. Regulation of cGAS- and RLR-mediated immunity to nucleic acids. Nat Immunol 2020;21:17-29. [Crossref] [PubMed]
- Wang Y, Ning X, Gao P, et al. Inflammasome Activation Triggers Caspase-1-Mediated Cleavage of cGAS to Regulate Responses to DNA Virus Infection. Immunity 2017;46:393-404. [Crossref] [PubMed]
- Tao J, Zhang XW, Jin J, et al. Nonspecific DNA Binding of cGAS N Terminus Promotes cGAS Activation. J Immunol 2017;198:3627-36. [Crossref] [PubMed]
- Du M, Chen ZJ. DNA-induced liquid phase condensation of cGAS activates innate immune signaling. Science 2018;361:704-9. [Crossref] [PubMed]
- Uggenti C, Lepelley A, Depp M, et al. cGAS-mediated induction of type I interferon due to inborn errors of histone pre-mRNA processing. Nat Genet 2020;52:1364-72. [Crossref] [PubMed]
- Song ZM, Lin H, Yi XM, et al. KAT5 acetylates cGAS to promote innate immune response to DNA virus. Proc Natl Acad Sci U S A 2020;117:21568-75. [Crossref] [PubMed]
- Zhou W, Mohr L, Maciejowski J, et al. cGAS phase separation inhibits TREX1-mediated DNA degradation and enhances cytosolic DNA sensing. Mol Cell 2021;81:739-55.e7. [Crossref] [PubMed]
- Yang S, Zhang Y, Cai J, et al. Clinical Characteristics of COVID-19 After Gynecologic Oncology Surgery in Three Women: A Retrospective Review of Medical Records. Oncologist 2020;25:e982-5. [Crossref] [PubMed]
- Liang W, Guan W, Chen R, et al. Cancer patients in SARS-CoV-2 infection: a nationwide analysis in China. Lancet Oncol 2020;21:335-7. [Crossref] [PubMed]
- Bakhoum SF, Ngo B, Laughney AM, et al. Chromosomal instability drives metastasis through a cytosolic DNA response. Nature 2018;553:467-72. [Crossref] [PubMed]
- An X, Zhu Y, Zheng T, et al. An Analysis of the Expression and Association with Immune Cell Infiltration of the cGAS/STING Pathway in Pan-Cancer. Mol Ther Nucleic Acids. 2019;14:80-89. [Crossref] [PubMed]
- Chalmers ZR, Connelly CF, Fabrizio D, et al. Analysis of 100,000 human cancer genomes reveals the landscape of tumor mutational burden. Genome Med 2017;9:34. [Crossref] [PubMed]
- Meléndez B, Van Campenhout C, Rorive S, et al. Methods of measurement for tumor mutational burden in tumor tissue. Transl Lung Cancer Res 2018;7:661-7. [Crossref] [PubMed]
- Marabelle A, Fakih M, Lopez J, et al. Association of tumour mutational burden with outcomes in patients with advanced solid tumours treated with pembrolizumab: prospective biomarker analysis of the multicohort, open-label, phase 2 KEYNOTE-158 study. Lancet Oncol 2020;21:1353-65. [Crossref] [PubMed]
- Ward R, Meagher A, Tomlinson I, et al. Microsatellite instability and the clinicopathological features of sporadic colorectal cancer. Gut 2001;48:821-9. [Crossref] [PubMed]
- Boussios S, Ozturk MA, Moschetta M, et al. The Developing Story of Predictive Biomarkers in Colorectal Cancer. J Pers Med 2019;9:12. [Crossref] [PubMed]
- Becht E, Giraldo NA, Lacroix L, et al. Estimating the population abundance of tissue-infiltrating immune and stromal cell populations using gene expression. Genome Biol 2016;17:218. [Crossref] [PubMed]
- Xu C, Zang Y, Zhao Y, et al. Comprehensive Pan-Cancer Analysis Confirmed That ATG5 Promoted the Maintenance of Tumor Metabolism and the Occurrence of Tumor Immune Escape. Front Oncol 2021;11:652211. [Crossref] [PubMed]
- Warde-Farley D, Donaldson SL, Comes O, et al. The GeneMANIA prediction server: biological network integration for gene prioritization and predicting gene function. Nucleic Acids Res 2010;38:W214-20. [Crossref] [PubMed]
- Shankavaram UT, Varma S, Kane D, et al. CellMiner: a relational database and query tool for the NCI-60 cancer cell lines. BMC Genomics 2009;10:277. [Crossref] [PubMed]
- Reinhold WC, Sunshine M, Liu H, et al. CellMiner: a web-based suite of genomic and pharmacologic tools to explore transcript and drug patterns in the NCI-60 cell line set. Cancer Res 2012;72:3499-511. [Crossref] [PubMed]
- Yarchoan M, Hopkins A, Jaffee EM. Tumor Mutational Burden and Response Rate to PD-1 Inhibition. N Engl J Med 2017;377:2500-1. [Crossref] [PubMed]
- Hoong BYD, Gan YH, Liu H, et al. cGAS-STING pathway in oncogenesis and cancer therapeutics. Oncotarget 2020;11:2930-55. [Crossref] [PubMed]
- Song C, Li Z, Li C, et al. SARS-CoV-2: The Monster Causes COVID-19. Front Cell Infect Microbiol 2022;12:835750. [Crossref] [PubMed]
- Op den Brouw ML, de Jong MA, Ludwig IS, et al. Branched oligosaccharide structures on HBV prevent interaction with both DC-SIGN and L-SIGN. J Viral Hepat 2008;15:675-83. [Crossref] [PubMed]
- van Liempt E, Bank CM, Mehta P, et al. Specificity of DC-SIGN for mannose- and fucose-containing glycans. FEBS Lett 2006;580:6123-31. [Crossref] [PubMed]
- Al Heialy S, Hachim MY, Senok A, et al. Regulation of Angiotensin- Converting Enzyme 2 in Obesity: Implications for COVID-19. Front Physiol 2020;11:555039. [Crossref] [PubMed]
- Patanavanich R, Glantz SA. Smoking Is Associated With COVID-19 Progression: A Meta-analysis. Nicotine Tob Res 2020;22:1653-6. [Crossref] [PubMed]
- Zhang Z, Zheng Y, Niu Z, et al. SARS-CoV-2 spike protein dictates syncytium-mediated lymphocyte elimination. Cell Death Differ 2021;28:2765-77. [Crossref] [PubMed]
- Iyer GR, Samajder S, Zubeda S, et al. Infectivity and Progression of COVID-19 Based on Selected Host Candidate Gene Variants. Front Genet 2020;11:861. [Crossref] [PubMed]
- Anisul M, Shilts J, Schwartzentruber J, et al. A proteome-wide genetic investigation identifies several SARS-CoV-2-exploited host targets of clinical relevance. Elife 2021;10:e69719. [Crossref] [PubMed]
- Dos Santos ACM, Dos Santos BRC, Dos Santos BB, et al. Genetic polymorphisms as multi-biomarkers in severe acute respiratory syndrome (SARS) by coronavirus infection: A systematic review of candidate gene association studies. Infect Genet Evol 2021;93:104846. [Crossref] [PubMed]
- Hertzog J, Zhou W, Fowler G, et al. Varicella-Zoster virus ORF9 is an antagonist of the DNA sensor cGAS. EMBO J 2022;41:e109217. [Crossref] [PubMed]
- Nyui T, Yoshino H, Nunota T, et al. cGAS Regulates the Radioresistance of Human Head and Neck Squamous Cell Carcinoma Cells. Cells 2022;11:1434. [Crossref] [PubMed]
- Duan D, Shang M, Han Y, et al. EZH2-CCF-cGAS Axis Promotes Breast Cancer Metastasis. Int J Mol Sci 2022;23:1788. [Crossref] [PubMed]
- Vilar E, Tabernero J. Molecular dissection of microsatellite instable colorectal cancer. Cancer Discov 2013;3:502-11. [Crossref] [PubMed]
- Vilar E, Gruber SB. Microsatellite instability in colorectal cancer-the stable evidence. Nat Rev Clin Oncol 2010;7:153-62. [Crossref] [PubMed]
- Hechtman JF, Middha S, Stadler ZK, et al. Universal screening for microsatellite instability in colorectal cancer in the clinical genomics era: new recommendations, methods, and considerations. Fam Cancer 2017;16:525-9. [Crossref] [PubMed]
- Chan TA, Yarchoan M, Jaffee E, et al. Development of tumor mutation burden as an immunotherapy biomarker: utility for the oncology clinic. Ann Oncol 2019;30:44-56. [Crossref] [PubMed]
- Fishel R. Mismatch repair. J Biol Chem 2015;290:26395-403. [Crossref] [PubMed]
- Russo M, Crisafulli G, Sogari A, et al. Adaptive mutability of colorectal cancers in response to targeted therapies. Science 2019;366:1473-80. [Crossref] [PubMed]
- Boland CR, Goel A. Microsatellite instability in colorectal cancer. Gastroenterology 2010;138:2073-87.e3.
- Lee DW, Han SW, Bae JM, et al. Tumor Mutation Burden and Prognosis in Patients with Colorectal Cancer Treated with Adjuvant Fluoropyrimidine and Oxaliplatin. Clin Cancer Res 2019;25:6141-7. [Crossref] [PubMed]
- Mlecnik B, Bindea G, Angell HK, et al. Integrative Analyses of Colorectal Cancer Show Immunoscore Is a Stronger Predictor of Patient Survival Than Microsatellite Instability. Immunity 2016;44:698-711. [Crossref] [PubMed]
- Yarchoan M, Johnson BA 3rd, Lutz ER, et al. Targeting neoantigens to augment antitumour immunity. Nat Rev Cancer 2017;17:209-22. [Crossref] [PubMed]
- Li K, Luo H, Huang L, et al. Microsatellite instability: a review of what the oncologist should know. Cancer Cell Int 2020;20:16. [Crossref] [PubMed]
- Jung H, Kim HS, Kim JY, et al. DNA methylation loss promotes immune evasion of tumours with high mutation and copy number load. Nat Commun 2019;10:4278. [Crossref] [PubMed]
- Sasidharan Nair V, Toor SM, Taha RZ, et al. DNA methylation and repressive histones in the promoters of PD-1, CTLA-4, TIM-3, LAG-3, TIGIT, PD-L1, and galectin-9 genes in human colorectal cancer. Clin Epigenetics 2018;10:104. [Crossref] [PubMed]
- Gowrishankar K, Gunatilake D, Gallagher SJ, et al. Inducible but not constitutive expression of PD-L1 in human melanoma cells is dependent on activation of NF-κB. PLoS One 2015;10:e0123410. [Crossref] [PubMed]
- Estécio MR, Issa JP. Dissecting DNA hypermethylation in cancer. FEBS Lett 2011;585:2078-86. [Crossref] [PubMed]
- Toraño EG, Petrus S, Fernandez AF, et al. Global DNA hypomethylation in cancer: review of validated methods and clinical significance. Clin Chem Lab Med 2012;50:1733-42. [Crossref] [PubMed]
- Li M, Guo W, Dong Y, et al. Elevated Exhaustion Levels of NK and CD8(+) T Cells as Indicators for Progression and Prognosis of COVID-19 Disease. Front Immunol 2020;11:580237. [Crossref] [PubMed]
- Tan L, Wang Q, Zhang D, et al. Lymphopenia predicts disease severity of COVID-19: a descriptive and predictive study. Signal Transduct Target Ther 2020;5:33. [Crossref] [PubMed]
- Xia P, Dubrovska A. Tumor markers as an entry for SARS-CoV-2 infection? FEBS J 2020;287:3677-80. [Crossref] [PubMed]
- Parsa AT, Waldron JS, Panner A, et al. Loss of tumor suppressor PTEN function increases B7-H1 expression and immunoresistance in glioma. Nat Med 2007;13:84-8. [Crossref] [PubMed]
- Ansell SM, Lesokhin AM, Borrello I, et al. PD-1 blockade with nivolumab in relapsed or refractory Hodgkin's lymphoma. N Engl J Med 2015;372:311-9. [Crossref] [PubMed]
- Grosso JF, Kelleher CC, Harris TJ, et al. LAG-3 regulates CD8+ T cell accumulation and effector function in murine self- and tumor-tolerance systems. J Clin Invest 2007;117:3383-92. [Crossref] [PubMed]
- Woo SR, Turnis ME, Goldberg MV, et al. Immune inhibitory molecules LAG-3 and PD-1 synergistically regulate T-cell function to promote tumoral immune escape. Cancer Res 2012;72:917-27. [Crossref] [PubMed]
(English Language Editor: A. Kassem)