Association between fasting plasma glucose variability and maternal and infant outcomes in patients with hyperglycemia during pregnancy: a retrospective cohort study
Highlight box
Key findings
• FPG-CV was independently associated with low-birth-weight infants, preterm birth, and preeclampsia in patients with gestational hyperglycemia.
• FPG-CV was positively correlated with SGA and low birth weight infants when at HbA1c levels of <5.9% during the third trimester of pregnancy.
• An FPG-CV value greater than 9.178 has good sensitivity and specificity for predicting preeclampsia in patients with gestational diabetes.
What is known and what is new?
• Blood GV is closely related to the occurrence of adverse obstetric outcomes.
• After adjusting for the effect of HbA1c, FPG-CV was independently associated with the occurrence of preeclampsia.
What is the implication, and what should change now?
• Patients with gestational hyperglycemia should undergo regular routine monitoring of FPG from diagnosis to production, and the impact of blood glucose fluctuation on adverse outcomes in mothers and infants should be considered in the clinical treatment of patients with gestational hyperglycemia.
Introduction
Hyperglycemia during pregnancy is a common metabolic complication and can increase the risk of adverse pregnancy outcomes in mothers and their offspring in the short and long term (1). Hyperglycemia increases the incidence of fetal complications, such as macrosomia, intrauterine growth retardation, premature delivery, stillbirths, fetal respiratory distress syndrome, and neonatal hypoglycemia. It also leads to metabolic syndrome in the offspring, as well as an increase in the possibility of maternal postpartum hemorrhage and the postpartum onset of type 2 diabetes mellitus (2). Hyperglycemia during pregnancy is classified into gestational diabetes mellitus (GDM), overt diabetes mellitus (ODM), and pre-GDM (PGDM), according to the 2020 guidelines of the Chinese Diabetes Society (CDS) (3). Using these guidelines, more pregnant women with mild hyperglycemia are diagnosed with GDM, which has subsequently increased the prevalence of GDM.
Studies have shown that blood glucose variability (GV) is closely related to the occurrence of adverse obstetric outcomes (4-6). Previous study has shown that the mean amplitude of glycemic excursions is an independent risk factor for adverse neonatal outcomes, such as large for gestational age (LGA), small for gestational age (SGA), and neonatal respiratory distress syndrome, and it can predict the occurrence of adverse outcomes including preeclampsia and neonatal hypoglycemia (4). Some studies have reported that a minimal increase in blood GV also affects fetal growth in utero (5). We have previously reviewed the importance of GV in patients with GDM and their related progress and found that well-controlled GV has greater benefits for patients with GDM (7). However, few studies have investigated the relationship between the change in fasting plasma glucose (FPG), an easily available and frequently-used indicator, from diagnosis to delivery, [as determined by the fasting plasma glucose coefficient of variation (FPG-CV)] and the occurrence of maternal and infant outcomes in patients with hyperglycemia during pregnancy. Current research suggests that the possible pathophysiological mechanisms of FPG variability and GDM are oxidative stress and β-cell dysfunction. Hyperglycemia variability enhances oxidative stress and promotes the production of cellular inflammatory factors. Intermittent hyperglycemia can also induce the formation of mitochondrial superoxide and reduce the secretion of adiponectin, indicating reduced glucose sensitivity. These processes promote the occurrence of diabetes. In addition, changes in blood sugar cause the pancreas β apoptosis may lead to poor blood glucose control and the further development of diabetes (8). These potential mechanisms lead to various pathological changes in GDM patients based on the high variability of FPG, which leads to poor maternal and infant outcomes. GDM is closely related to adverse pregnancy outcomes. The incidence of short- and long-term adverse maternal and infant events is high in patients with gestational hyperglycemia and poor blood glucose control (9). A study of hyperglycemia and adverse pregnancy outcomes showed that pregnant women with untreated blood glucose control developed preeclampsia compared with pregnant women with treated blood glucose control. Moreover, the proportion of newborns admitted to the intensive care unit increased and the Apgar 5-minute score of newborns decreased significantly (10). Therefore, to better control blood glucose levels, indicators reflecting blood glucose control should be adopted. Blood GV is a novel indicator of glucose control, which is associated with an increased risk of complications and mortality in patients with diabetes (11,12).
The commonly used evaluation indicators for the variability of FPG include the FPG-CV, FPG standard deviation (FPG-SD), and FPG variability independent of the mean (FPG-VIM). The present study mainly evaluated the relationship between FPG-CV and maternal and infant outcomes in patients with gestational hyperglycemia. Studies have shown that high hemoglobin A1c (HbA1c) levels in the middle and late trimesters of pregnancy are related to the occurrence of LGA (13). Moreover, HbA1c ≥5.9% has been reported to Increase the risk of premature delivery in GDM patients (14). Considering the correlation between HbA1c and maternal and infant outcomes, we adjusted the bias of HbA1c to evaluate the correlation between FPG-CV and maternal and infant outcomes of patients with gestational hyperglycemia at different HbA1c levels. Concurrently, we also attempted to determine an ideal boundary value in patients with hyperglycemia during pregnancy to predict the occurrence of maternal and infant outcomes. We present the following article in accordance with the STARD reporting checklist (available at https://atm.amegroups.com/article/view/10.21037/atm-22-6476/rc).
Methods
Study population
This retrospective cohort study included pregnant hyperglycemic patients who were hospitalized and had delivered in the Obstetrics Department of Shengjing Hospital Affiliated to China Medical University between June 2019 and December 2021. Specifically, the following patients were included: (I) patients who met the diagnostic criteria of ODM or GDM based on the 2020 CDS guidelines; (II) pregnant women aged 20 years or older with a single pregnancy; and (III) patients for whom venous blood fasting glucose monitoring was conducted at least four times in our hospital during the period from the diagnosis of ODM or GDM at 24–28 weeks of gestation to delivery, with the interval between two adjacent rounds of venous blood fasting glucose monitoring being >7 days. The exclusion criteria were as follows: (I) patients with pre-pregnancy diabetes mellitus based on the 2020 CDS diagnostic criteria for PGDM; (II) patients with incomplete clinical data; (III) patients with previous autoimmune diseases, tumors, severe infections, severe liver and kidney insufficiency, hematological diseases, and GDM; and (IV) patients with smoking or drinking habits.
The sample size calculation formula of the cohort study and NCSS-PASS 15 software were used to estimate the sample size with estimation parameters. The calculated sample size should be greater than 550 people. The formula used is as follows:
Diagnostic basis of GDM and ODM
GDM based on the 2020 CDS guidelines is diagnosed as follows: 75 g oral glucose tolerance test (OGTT) blood glucose at any time during pregnancy meets any of the following requirements: 5.1 mmol/L ≤ FPG <7.0 mmol/L, OGTT 1 h blood glucose ≥10.0 mmol/L, and 8.5 mmol/L ≤ OGTT 2 h blood glucose <11.1 mmol/L. Meanwhile, the diagnostic criteria of ODM are as follows: the blood glucose value at any time during pregnancy meets any one or more of the following: FPG ≥7.0 mmol/L, 75 g OGTT 2 h blood glucose ≥11.1 mmol/L, and random blood glucose ≥11.1 mmol/L.
Data collection and follow-up
The basic information and clinical characteristics of the patients, including age, height, weight before delivery, systolic blood pressure (SBP) on the first day of admission, diastolic blood pressure (DBP) on the first day of admission, if they were primipara, family history, abortion history, blood glucose 2 hours after meal after admission, the method of controlling blood glucose during pregnancy (insulin or oral hypoglycemic drugs), blood lipids before production, HbA1c in the third trimester fetal age, fetal sex, fetal birth weight, and neonatal blood glucose at delivery were collected. The basic information and clinical characteristics of the patients were obtained by HIS.
The body mass index (BMI) was calculated based on height and weight. Blood lipids included triglycerides, total cholesterol, high-density lipoprotein cholesterol (HDL-C), and low-density lipoprotein cholesterol (LDL-C). Maternal outcomes obtained at delivery included preeclampsia, emergency cesarean section, and dystocia. Neonatal outcomes included LGA, SGA, macrosomia, low birth weight, premature delivery, stillbirth, fetal malformation, and neonatal hypoglycemia.
Preeclampsia is defined as the occurrence of blood pressure >140/90 mmHg and proteinuria >300 mg/day after 20 weeks of pregnancy. Preterm birth is defined as the number of live birth weeks of pregnancy greater than 28 weeks and less than 37 weeks, and stillbirth is defined as the intrauterine death of the fetus after 20 weeks of pregnancy. LGA is defined as the 90th percentile of fetal birth weight greater than the average weight at the same gestational age, and SGA is defined as the 10th percentile of fetal birth weight less than the average weight at the same gestational age. The birth weight of newborns in 2020 was assessed according to the SGA Chinese birth age (15). Macrosomia is defined as birth weight >4,000 g, low birth weight is defined as birth weight <2,500 g, and neonatal hypoglycemia is defined as blood glucose <2.6 mmol/L at birth (16).
The study was conducted in accordance with the Declaration of Helsinki (as revised in 2013). The study was approved by Shengjing Hospital of China Medical University Ethics Committee (No. 2022PS629K), and individual consent for this retrospective analysis was waived.
Assessment of FPG variability
Participants who had at least four venous FPG measurements between the diagnosis of ODM or GDM at 24–28 weeks of gestation and delivery, and whose interval between two adjacent venous FPG monitoring was greater than 7 days were finally included in the calculation of FPG changes. The individual mean and SD of all the recorded FPG measurements were calculated for each included object. FPG-CV is defined as the average value of FPG-SD/FPG × 100%, which was used as the evaluation index of FPG variability.
FPG-VIM was defined as 100 × SD/mean β, where β is the regression coefficient, based on the natural log (ln) of the SD over the ln of the mean (8).
Statistical analysis
For the analysis of general data, the continuity variables were tested for normality, and the conformance test was compared by analysis of variance, which was expressed as the mean ± SD. The non-parametric test was used to compare the non-conforming normal distribution, which was expressed as the median and quartile. Categorical variables were compared using the chi-squared test and expressed as frequencies (percentages). All patients were divided into four groups based on the quartile of FPG-CV (group Q1: < P25; group Q2: P25–P50; group Q3: P50–P75; group Q4: > P75). The chi-square test or Fisher’s exact test was used to compare the relationship between maternal and infant outcomes in the four groups of FPG-CV.
Univariate binary logistic regression was used to evaluate the relationship between FPG-CV and the maternal and infant outcomes. Two multivariate binary logistic regression models were used to adjust for confounding factors. The first model was adjusted for age and BMI. The second model was adjusted for SBP, DBP, 2-hour postprandial blood glucose, triglyceride, cholesterol, HDL-C, LDL-C, family history, and abortion history based on the first model. Subgroup analysis was performed according to the HbA1c levels (≥5.9% and <5.9%) and insulin injection (unused and used) in the third trimester. The receiver operating characteristic (ROC) curve was used to evaluate the ability of FPG-CV to identify and predict the maternal and infant outcomes related to it, and the prediction limit of FPG-CV was determined using the Youden index. SPSS software version 27 (IBM Corp., Armonk, NY, USA) was applied for data sorting and statistical analysis. Odds ratio (OR) reflects the correlation strength between a certain exposure and the outcome, OR >1, indicating that exposure can promote the occurrence of the outcome, or that this factor is a risk factor for disease; OR <1, indicating that exposure can inhibit the occurrence of outcomes, or that this factor is a protective factor for disease; OR =1, indicating that exposure factors have no effect on the occurrence of disease. All statistical tests were bilateral tests, and statistical significance was set at P<0.05.
Results
Participants’ characteristics
A total of 7,145 patients with GDM and diabetes that occurred during pregnancy were discharged from the Hospital Information System (HIS) Obstetric Inpatient Ward of Shengjing Hospital China Medical University (Obstetric 1–Obstetric 6) from June 2019 to December 2021. A total of 608 patients were finally included according to the inclusion and exclusion criteria (Figure 1). Table 1 shows the basic information and clinical characteristics of patients with gestational hyperglycemia according to the FPG-CV quartile. The FPG-CV values of Q1, Q2, Q3, and Q4 group were <6.01%, 6.01–8.88%, 8.88–11.98%, and >11.98%, respectively. The proportion of FPG-SD and insulin injections differed among the groups (P<0.05). There were no significant differences in other clinical features among the GDM patients with different FPG-CV quartile arrays.
Table 1
Variables | Quartile of FPG-CV | P | |||
---|---|---|---|---|---|
Q1 (<6.01) | Q2 (6.01–8.88) | Q3 (8.88–11.98) | Q4 (>11.98) | ||
N | 152 | 152 | 152 | 152 | |
General data | |||||
Age† (years), M [P25, P50] | 32 [30, 35] | 32.5 [30.0, 36.0] | 32 [29, 35] | 32 [29, 35] | 0.968 |
BMI† (kg/m2), M [P25, P50] | 25.59 [22.52, 28.22] | 25.75 [22.76, 28.67] | 26.23 [23.01, 29.74] | 26.05 [23.45, 29.30] | 0.317 |
SBP† (mmHg), M [P25, P50] | 120 [111, 129] | 120 [112, 128] | 120 [110, 130] | 120 [112, 131] | 0.587 |
DBP† (mmHg), M [P25, P50] | 78 [69, 84] | 78 [68, 85] | 80 [69, 86] | 80 [70, 88] | 0.223 |
Unipara, n (%) | 114 (75.00) | 120 (78.95) | 122 (80.26) | 114 (75.00) | 0.591 |
Blood biochemical indices, M [P25, P50] | |||||
Triglyceride† (mmol/L) | 3.41 [2.46, 4.79] | 3.68 [2.97, 4.69] | 4.03 [2.86, 5.20] | 3.68 [2.81, 5.37] | 0.078 |
Total cholesterol† (mmol/L) | 5.68 [4.86, 6.74] | 5.76 [4.85, 6.65] | 5.85 [4.99, 6.70] | 5.63 [4.70, 6.40] | 0.400 |
HDL-C† (mmol/L) | 1.78 [1.45, 2.12] | 1.78 [1.51, 2.06] | 1.71 [1.40, 1.97] | 1.68 [1.43, 1.99] | 0.950 |
LDL-C† (mmol/L) | 3.22 [2.47, 3.95] | 3.05 [2.43, 3.79] | 3.17 [2.49, 3.89] | 3.25 [2.54, 3.77] | 0.681 |
Lifestyles, n (%) | |||||
Family history | 74 (48.68) | 75 (49.34) | 68 (44.74) | 67 (44.08) | 0.724 |
Abortion history | 3 (1.97) | 1 (0.66) | 2 (1.32) | 2 (1.32) | 0.750 |
Diabetes-related variables, M [P25, P50] | |||||
Mean of FPG† (mmol/L) | 4.98 [4.71, 5.24] | 4.96 [4.68, 5.26] | 4.90 [4.64, 5.23] | 4.89 [4.67, 5.29] | 0.578 |
FPG-SD† (mmol/L) | 0.23 [0.17, 0.26] | 0.37 [0.34, 0.41] | 0.51 [0.47, 0.55] | 0.75 [0.64, 0.89] | <0.001* |
2-hour postprandial blood glucose† (mmol/L) | 7.65 [7.00, 8.80] | 8.10 [6.90, 9.10] | 7.85 [6.93, 8.90] | 8.10 [6.90, 9.00] | 0.544 |
Medications, n (%) | |||||
Insulin | 18 (11.84) | 17 (11.18) | 33 (21.71) | 31 (20.39) | 0.016* |
Oral hypoglycemic drugs | 2 (1.32) | 3 (1.97) | 1 (0.66) | 1 (0.66) | 0.396 |
Fetus | |||||
Gestational age† (weeks), M [P25, P50] | 39.00 [38.32, 39.71] | 39.00 [38.29, 39.57] | 39.14 [38.43, 40.00] | 39.00 [38.04, 39.71] | 0.396 |
Male fetus, n (%) | 87 (57.24) | 82 (53.95) | 81 (53.29) | 74 (48.68) | 0.518 |
Fetal weight† (kg), M [P25, P50] | 3.34 [3.01, 3.64] | 3.36 [3.07, 3.70] | 3.37 [3.09, 3.72] | 3.36 [3.00, 3.68] | 0.588 |
The quartiles of FPG-CV were divided into four groups according to the quartile method. Group Q1: < P25; group Q2: P25–P50; group Q3: P50–P75; group Q4: > P75. Categorical variables are expressed as n (%) and were compared using the chi-square test. †, it does not conform to the normal distribution; therefore, it is expressed by the median [interquartile spacing] and was compared using a non-parametric test; *, P<0.05. FPG, fasting plasma glucose; CV, coefficient of variation; BMI, body mass index; SBP, systolic blood pressure; DBP, diastolic blood pressure; HDL-C, high-density lipoprotein cholesterol; LDL-C, low-density lipoprotein cholesterol; SD, standard deviation.
Comparison of maternal and infant outcomes in different FPG-CV quartiles
Patients with hyperglycemia during pregnancy were grouped according to the FPG-CV quartile, and the maternal and infant outcomes in each group are shown in Table 2. The maternal and infant outcomes between each group were then compared in pairs, and the results are shown in Figure 2. There were differences the in instances of preterm birth and preeclampsia among patients with hyperglycemia during pregnancy in the different FPG-CV quartiles (P<0.05), but there were no significant differences in the instances of LGA, SGA, macrosomia, low birth weight infants, emergency cesarean section, stillbirth, dystocia, fetal malformation, and neonatal hypoglycemia.
Table 2
Pregnancy outcomes | Q1 (<6.01), n | Q2 (6.01–8.88), n | Q3 (8.88–11.98), n | Q4 (>11.98), n | P |
---|---|---|---|---|---|
Summary† | 60 | 69 | 69 | 78 | 0.231 |
LGA | 35 | 42 | 40 | 43 | 0.732 |
SGA | 6 | 8 | 11 | 12 | 0.454 |
Macrosomia | 12 | 10 | 15 | 15 | 0.679 |
Low birth weight | 7 | 12 | 5 | 15 | 0.076 |
Premature delivery | 16 | 10 | 7 | 20 | 0.037* |
Preeclampsia | 2 | 4 | 11 | 16 | 0.001* |
Urgent cesarean section | 12 | 10 | 15 | 15 | 0.679 |
Stillbirth | 0 | 1 | 1 | 0 | 0.999 |
Dystocia | 1 | 3 | 0 | 4 | 0.340 |
Birth defects | 1 | 2 | 3 | 4 | 0.154 |
Neonatal hypoglycemia | 3 | 4 | 7 | 3 | 0.742 |
The quartiles of FPG-CV were divided into four groups according to the quartile method. Group Q1: < P25; group Q2: P25–P50; group Q3: P50–P75; group Q4: > P75. †, patients with hyperglycemia during pregnancy can have two or more maternal and infant outcomes at the same time; *, P<0.05. FPG, fasting plasma glucose; CV, coefficient of variation; LGA, large for gestational age; SGA, small for gestational age.
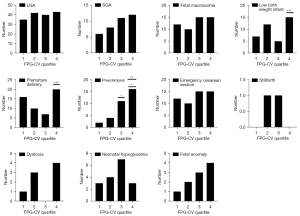
Correlation analysis between FPG variability and maternal and infant outcomes
Univariate logistic regression analysis showed that FPG-CV values were significantly correlated with SGA (OR =1.067, P=0.029), low birth weight infants (OR =1.077, P=0.009), preterm birth (OR =1.069, P=0.009), and preeclampsia (OR =1.159, P<0.001) (Table 3). FPG-SD values were significantly correlated with LGA (OR =2.022, P=0.041), low birth weight infants (OR 3.727, P=0.012), preterm birth (OR =4.160, P=0.002), and preeclampsia (OR =17.555, P<0.001) (Table 4).
Table 3
Dependent variables | Univariate | Model 1a | Model 2b | |||||
---|---|---|---|---|---|---|---|---|
OR (95% CI) | P | OR (95% CI) | P | OR (95% CI) | P | |||
LGA | 1.023 (0.987–1.060) | 0.222 | 1.017 (0.981–1.055) | 0.367 | 1.018 (0.980–1.056) | 0.362 | ||
SGA | 1.067 (1.007–1.130) | 0.029* | 1.066 (1.006–1.129) | 0.032 | 1.055 (0.993–1.121) | 0.083 | ||
Macrosomia | 1.015 (0.960–1.073) | 0.600 | 1.006 (0.951–1.065) | 0.830 | 1.007 (0.948–1.070) | 0.821 | ||
Low birth weight | 1.077 (1.018–1.138) | 0.009* | 1.079 (1.020–1.141) | 0.008* | 1.086 (1.023–1.152) | 0.007* | ||
Premature delivery | 1.069 (1.016–1.124) | 0.009* | 1.071 (1.019–1.127) | 0.008* | 1.069 (1.015–1.127) | 0.012* | ||
Preeclampsia | 1.159 (1.092–1.229) | <0.001* | 1.152 (1.085–1.222) | <0.001* | 1.180 (1.091–1.276) | <0.001* | ||
Urgent cesarean section | 1.048 (0.995–1.104) | 0.076 | 1.047 (0.994–1.104) | 0.082 | 1.045 (0.989–1.103) | 0.118 | ||
Stillbirth | 1.004 (0.759–1.328) | 0.978 | 0.994 (0.745–1.326) | 0.965 | 1.050 (0.736–1.499) | 0.787 | ||
Dystocia | 1.019 (0.892–1.166) | 0.778 | 1.025 (0.894–1.174) | 0.723 | 1.034 (0.905–1.182) | 0.622 | ||
Birth defects | 1.083 (0.983–1.193) | 0.107 | 1.084 (0.984–1.194) | 0.103 | 1.116 (0.999–1.246) | 0.052 | ||
Neonatal hypoglycemia | 1.028 (0.939–1.126) | 0.545 | 1.021 (0.932–1.118) | 0.657 | 1.026 (0.936–1.124) | 0.589 |
The correlation between FPG-CV and LGA, SGA, macrosomia, low birth weight infants, premature delivery, preeclampsia, emergency cesarean section, stillbirth, dystocia, fetal malformation, and neonatal hypoglycemia was examined using binary logistic regression analysis. a, adjusted for age and BMI index; b, SBP, DBP, 2-hour postprandial blood glucose, triglyceride, cholesterol, HDL-C, LDL-C, family history, and abortion history were further adjusted; *, P<0.05. Multivariate binary logistic regression was used for models 1 and 2. FPG, fasting plasma glucose; CV, coefficient of variation; OR, odds ratio; CI, confidence interval; LGA, large for gestational age; SGA, small for gestational age; BMI, body mass index; SBP, systolic blood pressure; DBP, diastolic blood pressure; HDL-C, high-density lipoprotein cholesterol; LDL-C, low-density lipoprotein cholesterol.
Table 4
Dependent variables | Univariate | Model 1a | Model 2b | |||||
---|---|---|---|---|---|---|---|---|
OR (95% CI) | P | OR (95% CI) | P | OR (95% CI) | P | |||
LGA | 2.022 (1.030–3.969) | 0.041* | 1.754 (0.884–3.481) | 0.108 | 1.783 (0.881–3.607) | 0.108 | ||
SGA | 2.682 (0.897–8.021) | 0.077 | 2.635 (0.871–7.976) | 0.086 | 2.101 (0.668–6.606) | 0.204 | ||
Macrosomia | 1.467 (0.519–4.147) | 0.469 | 1.185 (0.406–3.463) | 0.756 | 1.241 (0.389–3.954) | 0.715 | ||
Low birth weight | 3.727 (1.332–10.428) | 0.012* | 3.949 (1.391–11.208) | 0.010* | 4.092 (1.365–12.267) | 0.012* | ||
Premature delivery | 4.160 (1.668–10.380) | 0.002* | 4.475 (1.766–11.335) | 0.002* | 4.125 (1.567–10.864) | 0.004* | ||
Preeclampsia | 17.555 (6.034–51.075) | <0.001* | 15.186 (5.191–44.428) | <0.001* | 23.754 (5.813–97.067) | <0.001* | ||
Urgent cesarean section | 2.532 (0.967–6.624) | 0.058 | 2.483 (0.940–6.563) | 0.067 | 2.238 (0.812–6.169) | 0.120 | ||
Stillbirth | 0.418 (0.001–211.507) | 0.784 | 0.335 (0.001–204.673) | 0.738 | 0.463 (0.000–563.751) | 0.832 | ||
Dystocia | 1.106 (0.077–15.784) | 0.941 | 1.275 (0.087–18.712) | 0.859 | 1.469 (0.107–20.133) | 0.773 | ||
Birth defects | 4.354 (0.740–25.618) | 0.104 | 4.477 (0.755–26.547) | 0.099 | 7.489 (1.008–55.640) | 0.049* | ||
Neonatal hypoglycemia | 2.083 (0.411–10.555) | 0.376 | 1.730 (0.340–8.798) | 0.509 | 1.962 (0.374–10.289) | 0.425 |
The correlation between FPG-SD and LGA, SGA, macrosomia, low birth weight infants, premature delivery, preeclampsia, emergency cesarean section, stillbirth, dystocia, fetal malformation, and neonatal hypoglycemia was investigated using binary logistic regression analysis. a, adjusted for age and BMI index; b, SBP, DBP, 2-hour postprandial blood glucose, triglyceride, cholesterol, HDL-C, LDL-C, family history, and abortion history were further adjusted; *, P<0.05. Multivariate binary logistic regression was used for models 1 and 2. FPG, fasting plasma glucose; SD, standard deviation; OR, odds ratio; CI, confidence interval; LGA, large for gestational age; SGA, small for gestational age; BMI, body mass index; SBP, systolic blood pressure; DBP, diastolic blood pressure; HDL-C, high-density lipoprotein cholesterol; LDL-C, low-density lipoprotein cholesterol.
We then adjusted for age and BMI (model 1). FPG-CV remained independently associated with SGA (OR =1.066, P=0.032), low birth weight (OR =1.079, P=0.008), preterm birth (OR =1.071, P=0.008), and preeclampsia (OR =1.152, P<0.001). Also, FPG-SD remained independently associated with low birth weight (OR =3.949, P=0.010), preterm birth (OR =4.475, P=0.002), and preeclampsia (OR =15.186, P<0.001). Moreover, FPG-CV was consistently independently associated with low birth weight (OR =1.086, P=0.007), preterm birth (OR =1.069, P=0.012), and preeclampsia (OR =1.180, P<0.001). There was no notable correlation between LGA, macrosomia, emergency cesarean section, stillbirth, dystocia, fetal malformation, neonatal hypoglycemia, and FPG-CV in the univariate and multivariate logistic regression analyses (P>0.05). Moreover, FPG-SD was consistently independently associated with low birth weight (OR =4.092, P=0.012), preterm birth (OR =4.125, P=0.004), and preeclampsia (OR =23.754, P<0.001). In model 2, FPG-VIM was significantly correlated with SGA (OR =1.083, P=0.048), low birth weight infants (OR =1.123, P=0.004), premature delivery (OR =1.101, P=0.006), and preeclampsia (OR =1.245, P<0.001) (Table 5).
Table 5
Dependent variables | Univariate | Model 1a | Model 2b | |||||
---|---|---|---|---|---|---|---|---|
OR (95% CI) | P | OR (95% CI) | P | OR (95% CI) | P | |||
LGA | 1.023 (0.976–1.072) | 0.340 | 1.014 (0.967–1.063) | 0.573 | 1.014 (0.966–1.065) | 0.575 | ||
SGA | 1.097 (1.018–1.182) | 0.015* | 1.096 (1.017–1.182) | 0.017* | 1.083 (1.001–1.172) | 0.048* | ||
Macrosomia | 1.014 (0.942–1.091) | 0.712 | 1.001 (0.929–1.079) | 0.979 | 0.999 (0.923–1.081) | 0.981 | ||
Low birth weight | 1.105 (1.028–1.188) | 0.007* | 1.110 (1.031–1.195) | 0.006* | 1.123 (1.038–1.216) | 0.004* | ||
Premature delivery | 1.095 (1.025–1.169) | 0.007* | 1.100 (1.029–1.175) | 0.005* | 1.101 (1.028–1.181) | 0.006* | ||
Preeclampsia | 1.214 (1.123–1.312) | <0.001* | 1.201 (1.111–1.298) | <0.001* | 1.245 (1.122–1.382) | <0.001* | ||
Urgent cesarean section | 1.062 (0.992–1.137) | 0.084 | 1.060 (0.990–1.136) | 0.095 | 1.057 (0.984–1.136) | 0.128 | ||
Stillbirth | 1.002 (0.694–1.447) | 0.991 | 0.991 (0.678–1.448) | 0.961 | 1.072 (0.663–1.733) | 0.776 | ||
Dystocia | 1.029 (0.865–1.225) | 0.743 | 1.039 (0.870–1.241) | 0.673 | 1.051 (0.883–1.252) | 0.573 | ||
Birth defects | 1.117 (0.984–1.266) | 0.086 | 1.119 (0.985–1.271) | 0.083 | 1.161 (1.006–1.340) | 0.041* | ||
Neonatal hypoglycemia | 1.055 (0.942–1.182) | 0.354 | 1.042 (0.930–1.167) | 0.477 | 1.046 (0.933–1.173) | 0.437 |
The correlation between FPG-VIM and LGA, SGA, macrosomia, low birth weight infants, premature delivery, preeclampsia, emergency cesarean section, stillbirth, dystocia, fetal malformation, and neonatal hypoglycemia was studied using binary logistic regression analysis. a, adjusted for age and BMI index; b, SBP, DBP, 2-hour postprandial blood glucose, triglyceride, cholesterol, HDL-C, LDL-C, family history, and abortion history were further adjusted; *, P<0.05. Multivariate binary logistic regression was used for models 1 and 2. OR, odds ratio; CI, confidence interval; FPG, fasting plasma glucose; VIM, variability independent of the mean; LGA, large for gestational age; SGA, small for gestational age; BMI, body mass index; SBP, systolic blood pressure; DBP, diastolic blood pressure; HDL-C, high-density lipoprotein cholesterol; LDL-C, low-density lipoprotein cholesterol.
To reduce the impact of HbA1c on maternal and infant outcomes, we further performed subgroup regression analysis on the HbA1c level in the third trimester (≥5.9% and <5.9%), and the results are shown in Table 6. In the multivariate logistic regression models 1 and 2, FPG-CV was independently and positively correlated with low birth weight infants (OR =1.083, P=0.015) in the subgroup with HbA1c <5.9%; however, there was no significant correlation in the subgroup with HbA1c ≥5.9%. In the subgroup with HbA1c ≥5.9%, FPG-CV was independently and positively correlated with preterm birth (OR =1.292, P=0.013); however, there was no marked correlation in the subgroup with HbA1c <5.9%. In the subgroups with HbA1c <5.9% and HbA1c ≥5.9%, FPG-CV was independently and positively correlated with preeclampsia (OR =1.181, P=0.001 vs. OR =1.165, P=0.040).
Table 6
Dependent variables | Univariate | Model 1a | Model 2b | |||||
---|---|---|---|---|---|---|---|---|
OR (95% CI) | P | OR (95% CI) | P | OR (95% CI) | P | |||
LGA | ||||||||
HbA1c <5.9% | 1.013 (0.970–1.057) | 0.558 | 1.008 (0.965–1.053) | 0.716 | 1.003 (0.961–1.047) | 0.897 | ||
HbA1c ≥5.9% | 1.033 (0.962–1.111) | 0.370 | 1.031 (0.958–1.109) | 0.415 | 1.006 (0.963–1.052) | 0.779 | ||
SGA | ||||||||
HbA1c <5.9% | 1.073 (1.009–1.141) | 0.024* | 1.071 (1.007–1.139) | 0.029* | 1.066 (0.999–1.138) | 0.054 | ||
HbA1c ≥5.9% | 1.041 (0.871–1.244) | 0.658 | 1.054 (0.873–1.273) | 0.584 | 1.052 (0.848–1.305) | 0.645 | ||
Macrosomia | ||||||||
HbA1c <5.9% | 1.022 (0.951–1.098) | 0.561 | 1.017 (0.945–1.094) | 0.658 | 1.015 (0.936–1.101) | 0.719 | ||
HbA1c ≥5.9% | 0.985 (0.900–1.079) | 0.750 | 0.977 (0.891–1.072) | 0.622 | 1.000 (0.907–1.102) | 0.999 | ||
Low birth weight | ||||||||
HbA1c <5.9% | 1.087 (1.024–1.155) | 0.006* | 1.080 (1.016–1.148) | 0.014* | 1.083 (1.016–1.154) | 0.015* | ||
HbA1c ≥5.9% | 1.079 (1.015–1.146) | 0.014* | 1.083 (0.934–1.256) | 0.289 | 1.101 (0.926–1.309) | 0.278 | ||
Premature delivery | ||||||||
HbA1c <5.9% | 1.055 (0.996–1.117) | 0.067 | 1.056 (0.997–1.118) | 0.065 | 1.057 (0.997–1.121) | 0.063 | ||
HbA1c ≥5.9% | 1.128 (1.005–1.264) | 0.040* | 1.158 (1.020–1.314) | 0.023* | 1.292 (1.057–1.580) | 0.013* | ||
Preeclampsia | ||||||||
HbA1c <5.9% | 1.147 (1.072–1.228) | <0.001* | 1.143 (1.068–1.224) | <0.001* | 1.181 (1.076–1.295) | 0.001* | ||
HbA1c ≥5.9% | 1.174 (1.047–1.316) | 0.006* | 1.177 (1.044–1.327) | 0.008* | 1.165 (1.007–1.349) | 0.040* | ||
Urgent cesarean section | ||||||||
HbA1c <5.9% | 1.046 (0.986–1.109) | 0.133 | 1.042 (0.982–1.105) | 0.172 | 1.030 (0.970–1.095) | 0.335 | ||
HbA1c ≥5.9% | 1.056 (0.940–1.185) | 0.359 | 1.073 (0.948–1.215) | 0.264 | 1.108 (0.952–1.289) | 0.186 |
The correlation between FPG-CV and LGA, SGA, macrosomia, low birth weight infants, premature delivery, preeclampsia, emergency cesarean section, stillbirth, dystocia, fetal malformation, and neonatal hypoglycemia was studied using binary logistic regression analysis. a, adjusted for age and BMI index; b, SBP, DBP, 2-hour postprandial blood glucose, triglyceride, cholesterol, HDL-C, LDL-C, family history, and abortion history were further adjusted; *, P<0.05. Multivariate binary logistic regression was used for models 1 and 2. HbA1c, hemoglobin A1c; OR, odds ratio; CI, confidence interval; LGA, large for gestational age; SGA, small for gestational age; BMI, body mass index; SBP, systolic blood pressure; DBP, diastolic blood pressure; HDL-C, high-density lipoprotein cholesterol; LDL-C, low-density lipoprotein cholesterol.
To reduce the impact of insulin on maternal and infant outcomes, we further performed subgroup regression analysis on the insulin injection in the third trimester (unused and used), and the results are shown in Table 7. In the multivariate logistic regression models 1 and 2, FPG-CV was positively correlated with low birth weight infants (OR =1.801, P=0.016) and preterm delivery (OR =1.210, P=0.015) in the subgroup using insulin, while there was no notable correlation in the subgroup not using insulin. In the multivariate logistic regression model 1, FPG-CV was independently and positively correlated with preeclampsia (OR =1.119, P=0.001 vs. OR =1.300, P=0.001) in the subgroups without and with insulin. In model 2, FPG-CV was still independently and positively correlated with preeclampsia in the subgroup without insulin, while there was no significant correlation in the subgroup with insulin.
Table 7
Dependent variables | Univariate | Model 1a | Model 2b | |||||
---|---|---|---|---|---|---|---|---|
OR (95% CI) | P | OR (95% CI) | P | OR (95% CI) | P | |||
LGA | ||||||||
Insulin (unused) | 1.010 (0.970–1.052) | 0.624 | 1.002 (0.962–1.044) | 0.920 | 1.000 (0.958–1.044) | 0.994 | ||
Insulin (used) | 1.069 (0.981–1.165) | 0.126 | 1.075 (0.985–1.174) | 0.105 | 1.064 (0.967–1.171) | 0.202 | ||
SGA | ||||||||
Insulin (unused) | 1.062 (0.996–1.131) | 0.064 | 1.065 (0.998–1.136) | 0.056 | 1.052 (0.984–1.124) | 0.138 | ||
Insulin (used) | 1.103 (0.947–1.285) | 0.207 | 1.137 (0.948–1.363) | 0.165 | 1.615 (0.979–2.665) | 0.061 | ||
Macrosomia | ||||||||
Insulin (unused) | 1.023 (0.964–1.086) | 0.454 | 1.013 (0.953–1.076) | 0.686 | 1.007 (0.943–1.074) | 0.844 | ||
Insulin (used) | 0.981 (0.836–1.151) | 0.811 | 0.980 (0.832–1.155) | 0.811 | 0.993 (0.844–1.169) | 0.936 | ||
Low birth weight | ||||||||
Insulin (unused) | 1.039 (0.973–1.110) | 0.250 | 1.037 (0.969–1.109) | 0.296 | 1.043 (0.973–1.118) | 0.237 | ||
Insulin (used) | 1.315 (1.106–1.564) | 0.002* | 1.324 (1.100–1.593) | 0.003* | 1.801 (1.114–2.912) | 0.016* | ||
Premature delivery | ||||||||
Insulin (unused) | 1.034 (0.972–1.100) | 0.286 | 1.031 (0.969–1.097) | 0.340 | 1.029 (0.996–1.095) | 0.377 | ||
Insulin (used) | 1.170 (1.045–1.309) | 0.006* | 1.170 (1.034–1.325) | 0.013* | 1.210 (1.037–1.412) | 0.015* | ||
Preeclampsia | ||||||||
Insulin (unused) | 1.127 (1.055–1.203) | <0.001* | 1.119 (1.047–1.196) | 0.001* | 1.127 (1.034–1.227) | 0.006* | ||
Insulin (used) | 1.266 (1.100–1.458) | 0.001* | 1.300 (1.115–1.515) | 0.001* | 104109.914 (0.000–)# | 0.984 | ||
Urgent cesarean section | ||||||||
Insulin (unused) | 1.030 (0.967–1.097) | 0.361 | 1.029 (0.966–1.096) | 0.378 | 1.021 (0.958–1.089) | 0.516 | ||
Insulin (used) | 1.081 (0.970–1.204) | 0.159 | 1.078 (0.996–1.203) | 0.182 | 1.140 (0.990–1.314) | 0.068 |
The correlation between FPG-CV and LGA, SGA, macrosomia, low birth weight infants, premature delivery, preeclampsia, emergency cesarean section, stillbirth, dystocia, fetal malformation, and neonatal hypoglycemia was studied using binary logistic regression analysis. a, adjusted for age and BMI index; b, SBP, DBP, 2-hour postprandial blood glucose, triglyceride, cholesterol, HDL-C, LDL-C, family history, and abortion history were further adjusted; *, P<0.05; #, the large OR value is due to the large deviation between the two groups of sample data. Multivariate binary logistic regression was used for models 1 and 2. OR, odds ratio; CI, confidence interval; LGA, large for gestational age; SGA, small for gestational age; FPG, fasting plasma glucose; CV, coefficient of variation; BMI, body mass index; SBP, systolic blood pressure; DBP, diastolic blood pressure; HDL-C, high-density lipoprotein cholesterol; LDL-C, low-density lipoprotein cholesterol.
Prediction of maternal and infant outcomes by FPG-CV
We used ROC curves to evaluate the ability of FPG-CV to identify and predict SGA, low birth weight infants, preterm birth, and preeclampsia, and the results are presented in Table 8 and Figure 3. For the predictive diagnosis of SGA, the optimal FPG-CV boundary value was 7.9, with a sensitivity of 78.4% and specificity of 41.7% [area under the curve (AUC) =0.587]. For the predictive diagnosis of low birth weight infants, the best FPG-CV boundary value was 14.375, with a sensitivity of 33.3% and specificity of 85.9% (AUC =0.564). For the predictive diagnosis of preterm delivery, the best FPG-CV boundary value was 14.189, with a sensitivity of 34% and specificity of 85.6% (AUC =0.537). For the predictive diagnosis of preeclampsia, the optimal FPG-CV boundary value was 9.178, with a sensitivity of 94.9% and a specificity of 44.1% (AUC =0.725).
Table 8
Dependent variables | AUC | 95% CI lower limit | 95% CI upper limit | Sensitivity | Specificity | Best cutoff point |
---|---|---|---|---|---|---|
SGA | 0.587 | 0.495 | 0.679 | 0.784 | 0.417 | 7.900 |
Low birth weight | 0.564 | 0.462 | 0.667 | 0.333 | 0.859 | 14.375 |
Premature delivery | 0.537 | 0.443 | 0.632 | 0.340 | 0.856 | 14.189 |
Preeclampsia | 0.725 | 0.644 | 0.805 | 0.949 | 0.441 | 9.178 |
ROC, receiver operating characteristics; FPG, fasting plasma glucose; CV, coefficient of variation; AUC, area under the curve; CI, confidence interval; SGA, small for gestational age.
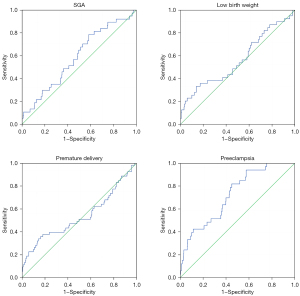
Discussion
This study showed that FPG-CV, as an evaluation index of FPG variability, was associated with SGA, low birth weight infants, preterm birth, and preeclampsia in patients with hyperglycemia during pregnancy. After excluding other conventional risk factors, FPG-CV was associated with low birth weight infants, preterm birth, and preeclampsia. Our results suggest that patients with gestational hyperglycemia should undergo regular routine monitoring of FPG from diagnosis to delivery, and the impact of blood glucose fluctuation on adverse outcomes in mothers and infants should be considered in the clinical treatment of patients with gestational hyperglycemia.
In recent decades, the incidence rate of GDM has been increasing, with a rate of approximately 14% worldwide in 2017 (17). A meta-analysis of the prevalence of GDM in East and Southeast Asia in 2018 showed that the prevalence of GDM in China was 11.91% (18). With the complete implementation of the “two-child policy” in China in January 2016, the number of older adults and overweight or obese pregnant women increased significantly, which further increased the number of patients with hyperglycemia during pregnancy (19). A retrospective study involving 79,064 Chinese participants showed that the total prevalence of GDM in mainland China was 14.8% (20). There is sufficient evidence that hyperglycemia during pregnancy can increase the risk of adverse pregnancy outcomes in mothers and their offspring in both the short and long term compared with normal pregnant women (1). A recent systematic review in South Asia showed that GDM is positively correlated with the occurrence of preeclampsia and has an impact on the occurrence of macrosomia, neonatal hyperglycemia, intrauterine growth retardation, stillbirth, and low birth weight (21). Some studies on LGA in China have shown that the incidence of LGA in patients with GDM ranges from 10–47.3% (22,23). In this study, the incidence of LGA in patients with hyperglycemia was 26.3%, and no correlation was observed between FPG-CV and FPG-CV. A higher incidence rate was considered for gestational weight gain, and BMI was associated with its occurrence (24). It is well known that hyperglycemia will increase the risk of macrosomia. In this study, the incidence of macrosomia in patients with gestational hyperglycemia was 8.5%, which was not related to FPG-CV. Also, the incidence of low birth weight infants was 6.4%. The possible reason related to FPG-CV is that pregnant women with high blood sugar and large fluctuations usually reduce their diet. Excessive diet control may cause malnutrition, and poor blood sugar control may affect the growth and development of the fetus. These factors may lead to a small fetus and poor development. The results of subgroup analysis regarding whether to use insulin in this study showed that after eliminating the influence of confounding factors, FPG-CV had an independent positive correlation with low birth weight infants (model 1: OR =1.324, P=0.003; model 2: OR =1.801, P=0.016) and preterm labor (model 1: OR =1.170, P=0.013; model 2: OR =1.210, P=0.015). A possible reason for this was that the hyperglycemic pregnant women who used insulin in this study had poor blood glucose control, which in turn increased the risk of low fetal weight and premature delivery. At the same time, the research participants included in this study were obstetric inpatients at our hospital. The sample size was large, the pregnancy situation of the inpatients was more complex, and the incidence of maternal and infant outcomes was higher, which can explain the high incidence of LGA in this study. There were differences between the maternal and infant outcomes and those of the research group. The research group included endocrine outpatients in our hospital for prospective research, and our research included obstetric inpatients. So, the different results may be related to the patient group.
In order to reduce the adverse consequences caused by poor blood glucose control, we use blood GV, a new evaluation index of blood glucose control (11,12). Studies have shown that blood GV is closely related to the occurrence of adverse obstetric outcomes (4-6). However, some studies have reported no correlation between blood GV and adverse outcomes in mothers and infants (25). Panyakat et al. conducted a 72-h continuous glucose monitoring (CGM) on GDM women in the third trimester of pregnancy. The study showed no correlation between blood GV and adverse pregnancy outcomes (25). As a traditional monitoring index, FPG level is closely related to maternal and infant outcomes. Continuous fasting hyperglycemia is more likely to lead to adverse maternal and infant outcomes in patients with gestational hyperglycemia (8,26). FPG in early pregnancy can predict the occurrence of LGA and is positively correlated with it (27). However, there are few studies on the correlation between FPG-CV and maternal and infant outcomes in patients with gestational hyperglycemia. This study showed that FPG-CV was positively correlated with SGA, low birth weight infants, preterm birth, and preeclampsia in patients with gestational hyperglycemia. After adjusting for common confounding factors, FPG-CV was still independently associated with the occurrence of low birth weight infants, preterm birth, and preeclampsia. Studies have shown that CGM can better measure the blood glucose levels of pregnant women and accurately determine blood GV. Compared to self-monitoring of blood glucose (SMBG), CGM can also reduce the occurrence of preeclampsia (4,28,29). However, in current relevant studies, CGM was used from 72 h to 7 days, and the sample size was small. Few studies have obtained blood glucose values throughout pregnancy, and CGM is expensive and difficult to popularize (30). The use of SMBG makes it easier to obtain the FPG-CV values during pregnancy; it only requires the pricking of a finger every day to measure the blood glucose levels, and has the advantages of low price, convenient operation, and is a popular way to measure blood glucose levels.
HbA1c has high specificity and low sensitivity and can reflect the average blood glucose level in the past 2–3 months. Studies have shown that HbA1c can predict the occurrence of adverse reactions in women with GDM (31). Moreover, women with GDM with HbA1c levels ≥5.9% have a higher risk of preeclampsia, premature delivery, and neonatal admission to the neonatal intensive care unit (NICU) (32). Barbry et al. revealed that HbA1c ≥5.9% increases the risk of premature delivery in women with GDM (14). Considering the effect of HbA1c level on maternal and infant outcomes, we adjusted for HbA1c bias. Based on our findings, after adjusting for HbA1c levels in patients with hyperglycemia during pregnancy, FPG-CV was independently associated with preeclampsia. FPG-CV was also positively correlated with SGA and low birth weight infants when HbA1c levels in the third trimester of pregnancy were <5.9%.
To better predict the occurrence of maternal and infant outcomes, this study further evaluated the ability of FPG-CV to identify and predict SGA, low birth weight infants, preterm birth, and preeclampsia. Patients with gestational hyperglycemia who had an FPG-CV value >9.178 were more likely to develop preeclampsia. However, few studies have investigated the correlation between FPG-CV and maternal and infant outcomes in patients with gestational hyperglycemia from diagnosis to delivery and we discovered that there was no accurate reference range for FPG-CV. In the future, a larger sample size and more standardized correlation studies are needed to determine the actual impact of FPG-CV during pregnancy on maternal and infant outcomes. It is also important to control blood GV to improve maternal and infant outcomes in patients with gestational hyperglycemia. Inositol supplementation during pregnancy can effectively reduce insulin resistance and stabilize blood GV in women with GDM (33). With increasing gestational age, insulin resistance also gradually increases. The rational application of hypoglycemic treatment can stabilize blood glucose levels in patients with hyperglycemia during pregnancy (34). However, the effects of different hypoglycemic regimens on blood GV and maternal and infant outcomes should be studied further.
Our study had several limitations that should be noted. Firstly, the measurement of FPG was based on the retrospective acquisition of venous FPG during obstetric hospitalization. The frequency and interval of FPG measurements varied from person to person and the differences in this regard have not been resolved. Therefore, we cannot rule out the impact of the rising and falling trends of FPG during pregnancy on the outcome; however, we did not find an appropriate index to define whether the FPG during pregnancy is rising or falling. Secondly, patients who measured the total number of FPG ≥4 times during pregnancy paid more attention to their own blood glucose control, and the degree of blood glucose control was relatively good, which would have produced a certain bias. Finally, considering that there were many groups of adverse outcomes in this study and the incidence of adverse outcomes (such as fetal malformation and neonatal hypoglycemia) was low, a larger sample size is needed to confirm the actual impact of blood GV on the outcome.
Conclusions
Our study showed that FPG-CV, as an evaluation index of FPG variability, was independently associated with low birth weight infants, preterm birth, and preeclampsia in patients with gestational hyperglycemia. After adjusting for the effect of HbA1c, FPG-CV was independently associated with the occurrence of preeclampsia and was also positively correlated with SGA and low birth weight infants when the HbA1c levels in the third trimester of pregnancy were <5.9%. An FPG-CV value greater than 9.178 showed good sensitivity and specificity for predicting preeclampsia in patients with gestational hyperglycemia.
Acknowledgments
Funding: This work was supported by the National Natural Science Foundation of China (No. 81700706); the 345 Talent Project of Shengjing Hospital, the Clinical Research Project of Liaoning Diabetes Medical Nutrition Prevention Society (No. LNSTNBYXYYFZXH-RS01B); the Natural Science Foundation of Liaoning Province (No. 2021-MS-182); the Science Foundation of Liaoning Education Department (No. LK201603); and the Virtual Simulation Experiment Teaching Project of China Medical University (No. 2020-47).
Footnote
Reporting Checklist: The authors have completed the STARD reporting checklist. Available at https://atm.amegroups.com/article/view/10.21037/atm-22-6476/rc
Data Sharing Statement: Available at https://atm.amegroups.com/article/view/10.21037/atm-22-6476/dss
Conflicts of Interest: All authors have completed the ICMJE uniform disclosure form (available at https://atm.amegroups.com/article/view/10.21037/atm-22-6476/coif). The authors have no conflicts of interest to declare.
Ethical Statement: The authors are accountable for all aspects of the work in ensuring that questions related to the accuracy or integrity of any part of the work are appropriately investigated and resolved. The study was conducted in accordance with the Declaration of Helsinki (as revised in 2013). The study was approved by Shengjing Hospital of China Medical University Ethics Committee (No. 2022PS629K), and individual consent for this retrospective analysis was waived.
Open Access Statement: This is an Open Access article distributed in accordance with the Creative Commons Attribution-NonCommercial-NoDerivs 4.0 International License (CC BY-NC-ND 4.0), which permits the non-commercial replication and distribution of the article with the strict proviso that no changes or edits are made and the original work is properly cited (including links to both the formal publication through the relevant DOI and the license). See: https://creativecommons.org/licenses/by-nc-nd/4.0/.
References
- Jolly MC, Sebire NJ, Harris JP, et al. Risk factors for macrosomia and its clinical consequences: a study of 350,311 pregnancies. Eur J Obstet Gynecol Reprod Biol 2003;111:9-14. [Crossref] [PubMed]
- Murtaugh MA, Jacobs DR Jr, Moran A, et al. Relation of birth weight to fasting insulin, insulin resistance, and body size in adolescence. Diabetes Care 2003;26:187-92. [Crossref] [PubMed]
- Diabetes Branch of Chinese Medical Association. Guidelines for the Prevention and Treatment of Type 2 Diabetes in China (2020 Edition) (Part 2). Chinese Journal of Practical Internal Medicine 2021;41:757-84.
- Yu F, Lv L, Liang Z, et al. Continuous glucose monitoring effects on maternal glycemic control and pregnancy outcomes in patients with gestational diabetes mellitus: a prospective cohort study. J Clin Endocrinol Metab 2014;99:4674-82. [Crossref] [PubMed]
- Dalfrà MG, Chilelli NC, Di Cianni G, et al. Glucose Fluctuations during Gestation: An Additional Tool for Monitoring Pregnancy Complicated by Diabetes. Int J Endocrinol 2013;2013:279021. [Crossref] [PubMed]
- Hyperglycemia and Adverse Pregnancy Outcome (HAPO) Study: associations with neonatal anthropometrics. Diabetes 2009;58:453-9. [Crossref] [PubMed]
- Yu W, Wu N, Li L, et al. A Review of Research Progress on Glycemic Variability and Gestational Diabetes. Diabetes Metab Syndr Obes 2020;13:2729-41. [Crossref] [PubMed]
- Kim JA, Kim J, Roh E, et al. Association of fasting plasma glucose variability with gestational diabetes mellitus: a nationwide population-based cohort study. BMJ Open Diabetes Res Care 2020;8:e001084. [Crossref] [PubMed]
- Tobias DK, Stuart JJ, Li S, et al. Association of History of Gestational Diabetes With Long-term Cardiovascular Disease Risk in a Large Prospective Cohort of US Women. JAMA Intern Med 2017;177:1735-42. [Crossref] [PubMed]
- Maryns AS, Dehaene I, Page G. Maternal and neonatal outcomes in a treated versus non- treated cohort of women with Gestational Diabetes Mellitus according to the HAPO 5 and 4 criteria. Facts Views Vis Obgyn 2017;9:133-40. [PubMed]
- Lee CL, Sheu WH, Lee IT, et al. Trajectories of fasting plasma glucose variability and mortality in type 2 diabetes. Diabetes Metab 2018;44:121-8. [Crossref] [PubMed]
- Tang X, Li S, Wang Y, et al. Glycemic variability evaluated by continuous glucose monitoring system is associated with the 10-y cardiovascular risk of diabetic patients with well-controlled HbA1c. Clin Chim Acta 2016;461:146-50. [Crossref] [PubMed]
- Fonseca L, Saraiva M, Amado A, et al. Third trimester HbA1c and the association with large-for-gestational-age neonates in women with gestational diabetes. Arch Endocrinol Metab 2021;65:328-35. [Crossref] [PubMed]
- Barbry F, Lemaitre M, Ternynck C, et al. HbA1c at the time of testing for gestational diabetes identifies women at risk for pregnancy complications. Diabetes Metab 2022;48:101313. [Crossref] [PubMed]
- Li H. Growth standard curves of birth weight, length and head circumference of Chinese newborns of different gestation. Chinese Journal of Pediatrics 2020;58:738-46. [PubMed]
- Expert consensus on standard clinical management of neonatal hypoglycemia in China (2021). Zhongguo Dang Dai Er Ke Za Zhi 2022;24:1-13. [PubMed]
- Cho NH, Shaw JE, Karuranga S, et al. IDF Diabetes Atlas: Global estimates of diabetes prevalence for 2017 and projections for 2045. Diabetes Res Clin Pract 2018;138:271-81. [Crossref] [PubMed]
- Nguyen CL, Pham NM, Binns CW, et al. Prevalence of Gestational Diabetes Mellitus in Eastern and Southeastern Asia: A Systematic Review and Meta-Analysis. J Diabetes Res 2018;2018:6536974. [Crossref] [PubMed]
- Juan J, Yang H. Prevalence, Prevention, and Lifestyle Intervention of Gestational Diabetes Mellitus in China. Int J Environ Res Public Health 2020;17:9517. [Crossref] [PubMed]
- Gao C, Sun X, Lu L, et al. Prevalence of gestational diabetes mellitus in mainland China: A systematic review and meta-analysis. J Diabetes Investig 2019;10:154-62. [Crossref] [PubMed]
- Mistry SK, Das Gupta R, Alam S, et al. Gestational diabetes mellitus (GDM) and adverse pregnancy outcome in South Asia: A systematic review. Endocrinol Diabetes Metab 2021;4:e00285. [Crossref] [PubMed]
- Liu FF. The study on risk factors of gestational diabetes mellitus and its impact on large gestational age infants. Tianjin: Tianjin Medical University, 2016.
- Harvey L, van Elburg R, van der Beek EM. Macrosomia and large for gestational age in Asia: One size does not fit all. J Obstet Gynaecol Res 2021;47:1929-45. [Crossref] [PubMed]
- Yang W, Liu J, Li J, et al. Interactive effects of prepregnancy overweight and gestational diabetes on macrosomia and large for gestational age: A population-based prospective cohort in Tianjin, China. Diabetes Res Clin Pract 2019;154:82-9. [Crossref] [PubMed]
- Panyakat WS, Phatihattakorn C, Sriwijitkamol A, et al. Correlation Between Third Trimester Glycemic Variability in Non-Insulin-Dependent Gestational Diabetes Mellitus and Adverse Pregnancy and Fetal Outcomes. J Diabetes Sci Technol 2018;12:622-9. [Crossref] [PubMed]
- Zheng Y, Shen Y, Jiang S, et al. Maternal glycemic parameters and adverse pregnancy outcomes among high-risk pregnant women. BMJ Open Diabetes Res Care 2019;7:e000774. [Crossref] [PubMed]
- Sesmilo G, Prats P, Garcia S, et al. First-trimester fasting glycemia as a predictor of gestational diabetes (GDM) and adverse pregnancy outcomes. Acta Diabetol 2020;57:697-703. [Crossref] [PubMed]
- Cappon G, Vettoretti M, Sparacino G, et al. Continuous Glucose Monitoring Sensors for Diabetes Management: A Review of Technologies and Applications. Diabetes Metab J 2019;43:383-97. [Crossref] [PubMed]
- Voormolen DN, DeVries JH, Sanson RME, et al. Continuous glucose monitoring during diabetic pregnancy (GlucoMOMS): A multicentre randomized controlled trial. Diabetes Obes Metab 2018;20:1894-902. [Crossref] [PubMed]
- Yu Q, Aris IM, Tan KH, et al. Application and Utility of Continuous Glucose Monitoring in Pregnancy: A Systematic Review. Front Endocrinol (Lausanne) 2019;10:697. [Crossref] [PubMed]
- Yin B, Hu L, Meng X, et al. Association of higher HbA1c within the normal range with adverse pregnancy outcomes: a cross-sectional study. Acta Diabetol 2021;58:1081-9. [Crossref] [PubMed]
- Rowan JA, Budden A, Ivanova V, et al. Women with an HbA1c of 41-49 mmol/mol (5.9-6.6%): a higher risk subgroup that may benefit from early pregnancy intervention. Diabet Med 2016;33:25-31. [Crossref] [PubMed]
- Pintaudi B, Di Vieste G, Corrado F, et al. Effects of myo-inositol on glucose variability in women with gestational diabetes. Eur Rev Med Pharmacol Sci 2018;22:6567-72. [PubMed]
- Jayasingh S Sr, Nanda S, Misra S, et al. Comparison of Fetomaternal Outcomes in Patients With Gestational Diabetes Mellitus Treated With Insulin Versus Acarbose: Results of a Prospective, Open Label, Controlled Study. Cureus 2020;12:e12283. [Crossref] [PubMed]
(English Language Editor: A. Kassem)