Association between heavy metal mercury in body fluids and tissues and diabetes mellitus: a systematic review and meta-analysis
Highlight box
Key findings
• No significant relationship was observed between mercury exposure and risk of diabetes mellitus.
What is known and what is new?
• Given the high incidence of diabetes and widespread exposure to mercury, as well as the inconsistent findings on the association between mercury and diabetes risk, it is essential that meta-analyses be conducted to assess this association.
• This is the first meta-analysis to focus on mercury exposure and the risk of diabetes mellitus.
What is the implication, and what should change now?
• Due to the unavoidable risk of bias, more well designed studies focusing on mercury exposure and the risk of diabetes mellitus are still needed.
Introduction
Diabetes mellitus is a metabolic disease characterized by chronic hyperglycemia, insufficient insulin secretion, or insensitivity to insulin receptors. The International Diabetes Federation (IDF) published the 10th edition of its “IDF Diabetes Atlas” on its website on December 6, 2021, and statistics reveal that in 2021, approximately 537 million adults aged 20 to 79 years worldwide, or one in 10 people, might have diabetes (1). The total number of diabetics is expected to reach 643 million by 2023 and 783 million by 2045 (1). The exploration and intervention of diabetes risk factors is the key to reducing the incidence of diabetes. Known risk factors for diabetes include genetic predisposition, old age, obesity, lack of physical activity, environmental chemicals, etc. (2). Recently, environmental chemicals are receiving increasing attention (2-4). Humans are exposed to a variety of metal substances as a result of environmental contamination. Their multiple applications in industry, households, agriculture, medicine, and technology have led to their widespread distribution in the environment (5,6). Most countries restricted the levels of hazardous heavy metals in the environment as industrialization progressed; however, it was unavoidable that people would be exposed to them through food, drinking water, and the surrounding air (7-9).
Mercury is a toxic, non-degradable heavy metal that exists widely in the environment in three forms: elemental mercury, inorganic mercury, and organic mercury. Mercury compounds are widely used in dry batteries, arc lamps, incandescent lamps, cosmetics, pharmaceuticals, mirrors, gold and silver extracted from ores, glass thermometers, sphygmomanometers, dental amalgam fillings, and vaccine preservatives (10,11). Elemental mercury is easily converted to methylmercury by microorganisms and accumulates in foods such as seafood and rice (12,13). As a result, mercury is an inevitable environmental contaminant in our lives that poses a significant hazard to human health. Further, high levels of mercury exposure can damage a variety of cells, including islet cells, through oxidative stress, ultimately affecting the central nervous system, cardiovascular system, urinary system, endocrine system, reproductive system, and immune system (14-17).
Some studies support a link between mercury exposure and diabetes, but reports have drawn conflicting conclusions. For example, a cross-sectional study in South Korea in 2010–2011 found a significant association between elevated blood mercury levels and increased fasting blood sugar (18). Similarly, a positive correlation between mercury and diabetes was observed in a cross-sectional study in China (19), but not in two studies in South Korea (8,20). Moreover, Roy et al. reported the relationship between heavy metal mercury and diabetes in a descriptive review paper, examining their possible association (21). Collectively, due to the mixed reports, little can be concluded about the relationship between mercury exposure and diabetes risk. The discrepancy is partly due to different countries of study, different sample sources, different types of studies, mercury exposure measured at only one time point, etc. Measuring mercury concentrations at only one time point is indeed problematic, and it would be better if there were multiple time points to be averaged. Unfortunately, most of the original studies did not address this issue. Furthermore, as mercury is not generally metabolized and excreted from the body, its concentration does not vary greatly from one time point to another, so the impact of this on the study has been ignored. Therefore, it is essential to explore the relationship between mercury exposure and diabetes using a systematic review and meta-analysis approach and to determine the relationship in different subgroups, which may complement new risk factors for diabetes and help delay or reduce the incidence of diabetes. We present the following article in accordance with the MOOSE reporting checklist (available at https://atm.amegroups.com/article/view/10.21037/atm-22-6404/rc).
Methods
Search strategy and selection criteria
The study protocol is registered on the International Prospective Register of Systematic Reviews (PROSPERO; No. CRD42022322375), where more details can be found.
We searched the PubMed, Web of Science, Cochrane, and Embase databases to retrieve relevant literature published before March 9, 2022 using the following keywords: (‘‘Diabetes Mellitus’’ OR ‘‘DM’’ OR ‘‘T2DM’’ OR ‘‘Diabetes’’ OR ‘‘Type 1 diabetes’’ OR ‘‘Type 2 diabetes’’) AND (‘‘Mercury’’ OR ‘‘methylmercury ‘‘OR ‘‘Hg’’) (see Tables S1-S4). Endnote, a document management program, was used to organize all the retrieved literature information.
Two authors (Yunran Guo and Xinming Liu) independently screened the articles. After deleting the duplicate articles, the title and abstract of each article were used to determine each study’s relevance, and the full text then underwent further screening as necessary. We also evaluated the retrieved articles and manually retrieved references to supplement any missing investigations. Finally, the contents and methods of the articles were evaluated, and any corresponding articles were included in the meta-analysis. Any disagreements between the authors were resolved with the assistance of the third author.
Inclusion and exclusion criteria
After discussion among all the researchers, it was agreed that the studies had to meet the following inclusion criteria for inclusion in the meta-analysis: (I) studies were included if they were cross-sectional, case-control, or cohort studies; (II) comprise adult subjects who were aged >18 years; (III) report data on the relationship between heavy metal mercury exposure and diabetes using the odds ratio (OR) and 95% confidence intervals (CIs), or report mercury concentrations in diabetic and healthy populations as the mean ± standard deviations (SDs) and sample sizes; (IV) mercury exposure in body fluids and tissues is measured by an unrestricted method; and (V) diabetes is defined as fasting serum glucose concentration ≥7.0 mmol/L and/or 2-h glucose concentration ≥11.1 mmol/L or other criterion-justified ranges. Studies were excluded from the meta-analysis if they met any of the following exclusion criteria: (I) were not of interest to us (e.g., concerned a review, report, systematic review and meta-analysis, letter, guide, conference abstract, or book); (II) had been published in a language other than English; (III) did not provide the results of interest to us; and/or (IV) the data of the study could not be accurately extracted or were missing.
Data extraction
The data, including details of the first author, study design, gender, year of publication, source of detection, study site, the main point of view, specimen, age, the exposure level of mercury, potential confounders for adjustment, OR and their associated 95% CIs, was extracted using a pre-designed Excel spreadsheet. If an article reported data on males and females separately, the OR and their 95% CIs were extracted by sex. We identified the studies with the most adjustment potential confounders from those with multiple risk estimates of adjustment reports based on distinct confounders. In addition, to make the most of the available data to enable us to conduct the most comprehensive meta-analysis possible, we also extracted heavy metal mercury concentrations from diabetic and healthy control groups to explore whether the mercury levels were higher in the biological samples of the diabetic patients than the healthy control patients. Standardized mean difference (SMD) was calculated using the available means and SDs of mercury concentrations and specimens as an effect estimate. If the articles reported standard errors (SEs) rather than SDs, the SDs were estimated based on their SEs and samples.
Quality assessment
Guo and Liu independently performed the quality assessment. The Newcastle-Ottawa Scale (NOS) was employed to assess the quality of the cohort and case-control studies. Each cohort study was evaluated in terms of its cohort selection, comparability, and outcome measurements. Each case-control study was evaluated based on the following three aspects: selection, comparability, and exposure assessment method. The widely used score cut-off of ≥7 was used in this study as a score for high-quality studies, as formal criteria for high quality have not yet been universally established, as shown in the previously published meta-analysis (22-24). Each cross-sectional study was evaluated using the Agency for Healthcare Research and Quality (AHRQ) scale recommended by the United States. A total of 11 items were evaluated by the AHRQ scale, including sources of information, inclusion and exclusion criteria, study participants’ time cycles and continuity, personnel blindness, quality assurance assessments, confusion and missing data, and patient response rates and completeness. The quality of each study was graded as follows: low quality = 0–3; moderate quality = 4–7; and high quality = 8–11, as shown in the previously published meta-analysis (25-28).
Statistical analysis
We analyzed the data using Stata version 15.0 (Stata Corporation, College Station, TX, USA). The study was performed using random-effects models to calculate the pooled OR, SMD, and their 95% CIs. As the ORs extracted for each study represent how many times the risk of diabetes at the highest level of mercury exposure is the lowest level, we calculated pooled effect values (28,29). We calculated I2 to assess the heterogeneity between the studies, with significant heterogeneity expressed at I2>50%, P<0.1. To examine heterogeneity, we performed subgroup analyses by study type, country, sex, and specimen. A sensitivity analysis was carried out by eliminating one article at a time and re-calculating the pooled OR. Publication bias was statistically assessed by Begg’s test and Egger’s test. A two-tailed P value < 0.05 was considered statistically significant.
Results
Literature search and study characteristics
We retrieved 8,430 articles from our database searches. After removing the duplicate articles, we screened the 4,605 articles and reviewed the full text of 31 articles by reviewing the headlines and abstracts. A further 23 articles were eliminated based on the inclusion and exclusion criteria, and ultimately eight articles were included in the meta-analysis. Five articles (six studies) were used to investigate the relationship between high and low mercury exposure and diabetes, and three articles (three studies) were used to examine mercury concentration differences between diabetics and controls (Figure 1) (8,9,19,20,30-33). The baseline characteristics of the included studies are shown in Table 1.
Table 1
Study | Country | Design | Specimen | Population | Age (years) | Gender | Exposure level (values or mean ± standard deviation) | Adjustment | OR (95% CI) |
---|---|---|---|---|---|---|---|---|---|
Flores et al. (30), 2011 | Mexico | Cross-sectional | Blood | 88 | 42–67 | M/F | (1.12±0.97) vs. (0.18±0.35) | NA | NA |
Moon (20), 2013 | South Korea | Cross-sectional | Blood | 3,184 | ≥30 | M/F | Q1: 2.10±1.01; Q2: 3.53±1.00; Q3: 5.16±1.01; Q4: 9.62±1.01 | Age, sex, region, smoking, alcohol consumption and regular exercise | 1.076 (0.758, 1.526) |
Pal et al. (32), 2013 | Canada | Cross-sectional | Hair | 72 | >18 | M/F | (1763.8±2218.9) vs. (982.6±918.46) | NA | NA |
Hansen et al. (31), 2017 | Norway | Case-control | Blood | 883 | ≥20 | M/F | 3.47 vs. 3.18 | Age, sex, body mass index, waist-to-hip ratio, education, income, smoking and family history of diabetes | 1.66 (0.79, 3.47) |
Tsai et al. (19), 2019 | China | Cross-sectional | RBC | 646 | ≥19 | M/F | T1: <10.66; T2: 10.66–18.80; T3: >18.80 | Age, sex, BMI, educational status, hypertension, total cholesterol, fasting glucose, cigarette smoking, alcohol consumption, saltwater fish consumption, total calorie intake, protein and fat intake, geographical strata, seasonality, C-reactive protein and hemoglobin | 4.71 (1.86, 11.93) |
Wang et al. (9), 2020 | America | Cohort | Urine | 1,237 | 45–56 | M/F | (1.22±1.11) vs. (1.46±1.32) | NA | NA |
Zhang et al. (33), 2021 | China | Cross-sectional | Blood | 30,994 | >20 | F | Q1: ≤0.44; Q2: 0.45–0.84; Q3: 0.85–1.69; Q4: >1.69 | Data release cycle, age, sex, race/ethnicity, body mass index, hypertension, poverty-income ratio, education, marital status, and daily intakes of protein, total fat, sugar, fiber | 0.76 (0.63, 0.92) |
Moon et al. (8), 2022 | South Korea | Cross-sectional | Blood | 3,787 | ≥19 | M/F | NA | Age, sex, cigarette smoking, alcohol drinking, exercise, and education levels | 0.87 (0.59, 1.29) |
Moon et al. (8), 2022 | South Korea | Cross-sectional | Urine | 3,787 | ≥19 | M/F | NA | Age, sex, cigarette smoking, alcohol drinking, exercise, and education levels | 0.97 (0.62, 1.51) |
M, male; F, female; NA, not available; OR, odds ratio; CI, confidence interval; Q1/Q2/Q3/Q4, quartile 1/2/3/4; T1/T2/T3, tertile1/2/3; BMI, body mass index.
Quality assessment of the included studies
Two studies were rated as high quality and six as medium quality according to the NOS Quality Evaluation and AHRQ Checklist (Tables S5-S7 of the Supplementary material)
Meta-analyses and subgroup analyses
The meta-analyses using random-effects models revealed no statistically significant association between high mercury exposure and diabetes as compared to lower mercury exposure (OR: 1.11, 95% CI: 0.80, 1.55, n=6, I2=73.7%) (Figure 2).
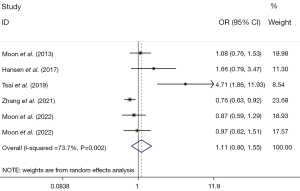
As the I2 analysis revealed significant heterogeneity, we conducted a subgroup analysis based on sex, country of study, type of study, and specimen, and found the following: (I) Gender: a significant association was only found in the male subgroup (pooled OR: 0.71, 95% CI: 0.57, 0.90, n=3, I2=0.0%), but no such correlation was found in the female subgroup (pooled OR: 1.11, 95% CI: 0.69, 1.79, n=3, I2=67.7%) (Table 2). (II) Study countries: no statistical associations were found in South Korea (pooled OR: 0.98, 95% CI: 0.78, 1.22, n=3, I2=0.0%), China (pooled OR: 1.78, 95% CI: 0.30, 10.62, n=2, I2=93.0%), or Norway (pooled OR: 1.66, 95% CI: 0.79, 3.48, n=1) (Table 2). (III) Study design: no statistical associations were detected in the cross-sectional studies (pooled OR: 1.05, 95% CI: 0.75, 1.49, n=5, I2=75.4%), or case-control studies (pooled OR: 1.66, 95% CI: 0.79, 3.48, n=1) (Table 2). (IV) Specimen: no statistical associations were detected in the blood (pooled OR: 0.93, 95% CI: 0.72, 1.21, n=4, I2=51.9%), urine (OR: 0.97, 95% CI: 0.62, 1.51, n=1), or red blood cell (RBC) (OR: 4.71, 95% CI: 1.86, 11.93, n=1) (Table 2).
Table 2
Subgroups | No. of studies | OR (95% CI) | Heterogeneity test | |
---|---|---|---|---|
I2 | P value | |||
Gender | ||||
Male | 3 | 0.71 (0.57, 0.90) | 0.0% | 0.912 |
Female | 3 | 1.11 (0.69, 1.79) | 67.7% | 0.045 |
Study countries | ||||
South Korea | 3 | 0.98 (0.78, 1.22) | 0.0% | 0.729 |
China | 2 | 1.78 (0.30, 10.62) | 93.0% | 0.002 |
Norway | 1 | 1.66 (0.79, 3.48) | – | – |
Study types | ||||
Cross-sectional study | 5 | 1.05 (0.75, 1.49) | 75.4% | 0.003 |
Case-control | 1 | 1.66 (0.79, 3.48) | – | – |
Specimen | ||||
Blood | 4 | 0.93 (0.72, 1.21) | 51.9% | 0.100 |
RBC | 1 | 4.71 (1.86, 11.93) | – | – |
Urine | 1 | 0.97 (0.62, 1.51) | – | – |
OR, odds ratio; CI, confidence interval; RBC, red blood cell.
In three studies, comprising 1,798 participants, no statistically significant difference was observed in the heavy metal levels between the diabetic and control groups (SMD: 0.41, 95% CI: –0.32, 1.14) (Figure 3).
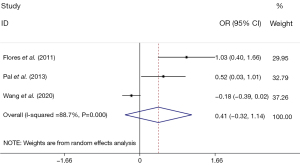
Sensitivity analysis
The sensitivity analysis showed no significant change in the overall effects when each study was sequentially removed, and the pooled OR was recalculated, demonstrating the robustness of the results (Figure 4).
Publication bias
Begg’s test was used to examine publication bias, and none of the studies included in this study demonstrated potential publication bias (P=0.060) (Figure 5). However, the Egger’s test results showed publication bias (P=0.013) (Figure 6). After a trim-and-fill correction for publication bias, no missing studies were found, and the results did not change (Figure 7).
Discussion
Diabetes mellitus is a chronic metabolic disorder caused by a variety of factors. Some metals in the environment are regarded as risk factors for diabetes (34). Mercury is a redox passivating metal, and current research suggests that it may induce oxidative stress by decreasing the enzymatic activity of superoxide dismutase, lowering antioxidants, or binding to protein-Sulfhydryl (-SH) groups (35,36). Some of the toxic effects of mercury have also been found to be related to mitochondrial dysfunction, which has been found to be related to oxidative damage and the inflammation of the β-cell apoptotic signaling pathway, lowering β-cell function, and increasing susceptibility to diabetes (34). In summary, oxidative stress, apoptosis, and inflammation play roles in mercury-induced diabetes (16,17,35,37,38). However, their exact roles and mechanisms in islet beta-cell function and glucose regulation are unclear.
Some epidemiological studies support a link between mercury and diabetes. A cross-sectional study in Greenland found that high whole-blood mercury is a risk factor for impaired fasting blood sugar (OR: 1.03, 95% CI: 1.02, 1.05) and type 2 diabetes (OR: 1.02, 95% CI: 1.01, 1.04) (39). In a prospective observational study, He et al. found that individuals exposed to high levels of mercury during their youth may have an increased risk of diabetes later in life (OR: 1.65, 95% CI: 1.07, 2.56) (40). Tsai et al. discovered that increased levels of mercury in red blood cells were substantially related to the risk of type 2 diabetes in the studies included in their meta-analysis (19). Studies by Flores et al. and Pal et al. also showed that the mercury levels in biological samples were higher in diabetics than in healthy people (30,32). All this evidence suggests that mercury may be a risk factor for diabetes, but our meta-analysis results did not support this conclusion.
It is well known that the heterogeneity and suboptimal quality of a study may affect the final outcome. Since our study had a high degree of heterogeneity, we performed subgroup analyses to understand the origin of the heterogeneity. In subgroups with both genders (the data from the original study were not grouped by sex), there was no association between mercury and diabetes. Despite the limited number of studies, the results demonstrated a negative correlation between mercury and diabetes in the male subgroup but no association in the female subgroup. There is no (published) plausible mechanism to explain this phenomenon. Thus, further research should be conducted to collect gender-specific data to better understand the role of gender in the relationship between mercury exposure and diabetes. The different study designs of the studies we included may result in clinical heterogeneity. In subgroups with both case-control and cross-sectional studies, there was no association between mercury and diabetes. However, more well-designed studies are needed to verify the results in the future. Low-quality studies might reduce the credibility of results, whereas this meta-analysis did not include low-quality studies.
In some of the studies analyzed in this article, the dominance ratio was calculated based on the total mercury concentration’s quartile or tertile, and the highest concentration’s dominance ratio was extracted. Moon et al. found no correlation between mercury exposure and diabetes in the fourth quartile, but found that mercury exposure was negatively correlated to diabetes in the third quartile (8). This suggests a possible link between mercury exposure and diabetes at specific concentrations. As a non-biodegradable, non-essential heavy metal, mercury can accumulate to toxic levels in organisms by various means and can be highly toxic even at low concentrations (35). In addition, a study has linked elevated mercury levels in the brains of mice to increased neurobehavioral effects (41). This suggests that future studies should focus on the effect of various concentrations of mercury exposure on the risk of diabetes.
Mercury exists in three forms in the environment, each with its own source of exposure and hazardous effects (42). Notably, methylmercury is the most toxic and dangerous mercury in the environment (43). The long-term consumption of methylmercury in fish and marine mammals is unavoidable due to its accumulation in the food chain (44). Methylmercury toxicity to the liver has been reported to affect the activity of key enzymes involved in glucose metabolism (45). A previous study has found that methylmercury can impair the development and function of pancreatic beta cells, resulting in insulin resistance and elevated blood sugar levels (46). However, this study measured the total mercury concentration in biological samples, making it impossible to analyze data on various types of mercury further.
Our study had some limitations. First, there was publication bias in the included studies. As no literature search in non-English language databases and no non-English articles were included in this meta-analysis, there may be language bias. We await the subsequent publication of high-quality articles to address this deficiency. Second, in addition to the insufficient number of articles included in the study, other factors, including the type of mercury (i.e., elemental, inorganic, and organic), exposure time and dose, type of biological specimen, and the population’s sex and age, may have affected our judgment of the genuine relationship between mercury and diabetes, which needs to be addressed in future studies. Third, adjusting for confounding factors has an important influence on the results of our study. Adjusted OR and their 95% CI were directly extracted from most of the included original studies in this meta-analysis. However, three original studies only provided mean and SDs, but more specific data were not provided (9,30,32). Therefore, we could not adjust these results.
Conclusions
Based on the data of 40,891 patients collected from eight articles, our results revealed no association between heavy metal mercury exposure and diabetes. More research needs to be conducted to adjust for potential confounding factors and re-assess the relationship between mercury exposure and diabetes.
Acknowledgments
We would like to thank the researchers and study participants for their contributions.
Funding: None.
Footnote
Reporting Checklist: The authors have completed the MOOSE reporting checklist. Available at https://atm.amegroups.com/article/view/10.21037/atm-22-6404/rc
Conflicts of Interest: All authors have completed the ICMJE uniform disclosure form (available at https://atm.amegroups.com/article/view/10.21037/atm-22-6404/coif). The authors have no conflicts of interest to declare.
Ethical Statement: The authors are accountable for all aspects of the work in ensuring that questions related to the accuracy or integrity of any part of the work are appropriately investigated and resolved.
Open Access Statement: This is an Open Access article distributed in accordance with the Creative Commons Attribution-NonCommercial-NoDerivs 4.0 International License (CC BY-NC-ND 4.0), which permits the non-commercial replication and distribution of the article with the strict proviso that no changes or edits are made and the original work is properly cited (including links to both the formal publication through the relevant DOI and the license). See: https://creativecommons.org/licenses/by-nc-nd/4.0/.
References
- International Diabetes Federation. IDF Diabetes Atlas. 10th ed. Brussels, Belgium: International Diabetes Federation; 2021.
- Jeon JY, Ha KH, Kim DJ. New risk factors for obesity and diabetes: Environmental chemicals. J Diabetes Investig 2015;6:109-11. [Crossref] [PubMed]
- Tsimihodimos V, Gonzalez-Villalpando C, Meigs JB, et al. Hypertension and Diabetes Mellitus: Coprediction and Time Trajectories. Hypertension 2018;71:422-8. [Crossref] [PubMed]
- Hectors TL, Vanparys C, van der Ven K, et al. Environmental pollutants and type 2 diabetes: a review of mechanisms that can disrupt beta cell function. Diabetologia 2011;54:1273-90. [Crossref] [PubMed]
- Rahman Z, Singh VP. The relative impact of toxic heavy metals (THMs) (arsenic (As), cadmium (Cd), chromium (Cr)(VI), mercury (Hg), and lead (Pb)) on the total environment: an overview. Environ Monit Assess 2019;191:419. [Crossref] [PubMed]
- Zhang Y, Song Z, Huang S, et al. Global health effects of future atmospheric mercury emissions. Nat Commun 2021;12:3035. [Crossref] [PubMed]
- CDC. Fourth National Report on Human Exposure to Environmental Chemicals. 2019.
- Moon MK, Lee I, Lee A, et al. Lead, mercury, and cadmium exposures are associated with obesity but not with diabetes mellitus: Korean National Environmental Health Survey (KoNEHS) 2015-2017. Environ Res 2022;204:111888. [Crossref] [PubMed]
- Wang X, Karvonen-Gutierrez CA, Herman WH, et al. Urinary metals and incident diabetes in midlife women: Study of Women's Health Across the Nation (SWAN). BMJ Open Diabetes Res Care 2020;8:e001233. [Crossref] [PubMed]
- Thangavelu L, Veeraragavan GR, Mallineni SK, et al. Role of Nanoparticles in Environmental Remediation: An Insight into Heavy Metal Pollution from Dentistry. Bioinorg Chem Appl 2022;2022:1946724. [Crossref] [PubMed]
- Zhao M, Li Y, Wang Z. Mercury and Mercury-Containing Preparations: History of Use, Clinical Applications, Pharmacology, Toxicology, and Pharmacokinetics in Traditional Chinese Medicine. Front Pharmacol 2022;13:807807. [Crossref] [PubMed]
- Sharp D. Environmental toxins, a potential risk factor for diabetes among Canadian Aboriginals. Int J Circumpolar Health 2009;68:316-26. [Crossref] [PubMed]
- Lv W, Zhan T, Abdelhafiz MA, et al. Selenium-amended biochar mitigates inorganic mercury and methylmercury accumulation in rice (Oryza sativa L.). Environ Pollut 2021;291:118259. [Crossref] [PubMed]
- Choi J, Bae S, Lim H, et al. Mercury Exposure in Association With Decrease of Liver Function in Adults: A Longitudinal Study. J Prev Med Public Health 2017;50:377-85. [Crossref] [PubMed]
- Vas J, Monestier M. Immunology of mercury. Ann N Y Acad Sci 2008;1143:240-67. [Crossref] [PubMed]
- Chen YW, Huang CF, Tsai KS, et al. The role of phosphoinositide 3-kinase/Akt signaling in low-dose mercury-induced mouse pancreatic beta-cell dysfunction in vitro and in vivo. Diabetes 2006;55:1614-24. [Crossref] [PubMed]
- Tinkov AA, Ajsuvakova OP, Skalnaya MG, et al. Mercury and metabolic syndrome: a review of experimental and clinical observations. Biometals 2015;28:231-54. [Crossref] [PubMed]
- Eom SY, Choi SH, Ahn SJ, et al. Reference levels of blood mercury and association with metabolic syndrome in Korean adults. Int Arch Occup Environ Health 2014;87:501-13. [Crossref] [PubMed]
- Tsai TL, Kuo CC, Pan WH, et al. Type 2 diabetes occurrence and mercury exposure - From the National Nutrition and Health Survey in Taiwan. Environ Int 2019;126:260-7. [Crossref] [PubMed]
- Moon SS. Association of lead, mercury and cadmium with diabetes in the Korean population: the Korea National Health and Nutrition Examination Survey (KNHANES) 2009-2010. Diabet Med 2013;30:e143-8. [Crossref] [PubMed]
- Roy C, Tremblay PY, Ayotte P. Is mercury exposure causing diabetes, metabolic syndrome and insulin resistance? A systematic review of the literature. Environ Res 2017;156:747-60. [Crossref] [PubMed]
- Lyngsø J, Ramlau-Hansen CH, Bay B, et al. Association between coffee or caffeine consumption and fecundity and fertility: a systematic review and dose-response meta-analysis. Clin Epidemiol 2017;9:699-719. [Crossref] [PubMed]
- Wijs LA, Fusco MR, Doherty DA, et al. Asthma and allergies in offspring conceived by ART: a systematic review and meta-analysis. Hum Reprod Update 2021;28:132-48. [Crossref] [PubMed]
- Montazeri Z, Nyiraneza C, El-Katerji H, et al. Waterpipe smoking and cancer: systematic review and meta-analysis. Tob Control 2017;26:92-7. [Crossref] [PubMed]
- Mao DH, Miao JK, Zou X, et al. Risk Factors in Predicting Prognosis of Neonatal Bacterial Meningitis-A Systematic Review. Front Neurol 2018;9:929. [Crossref] [PubMed]
- Hu J, Dong Y, Chen X, et al. Prevalence of suicide attempts among Chinese adolescents: A meta-analysis of cross-sectional studies. Compr Psychiatry 2015;61:78-89. [Crossref] [PubMed]
- Wang Y, Yang X, Han Q, et al. Prevalence of Sialorrhea Among Amyotrophic Lateral Sclerosis Patients: A Systematic Review and Meta-Analysis. J Pain Symptom Manage 2022;63:e387-96. [Crossref] [PubMed]
- Hu Q, Li SJ, Chen QL, et al. Risk Factors for Acute Kidney Injury in Critically Ill Neonates: A Systematic Review and Meta-Analysis. Front Pediatr 2021;9:666507. [Crossref] [PubMed]
- Xu P, Liu A, Li F, et al. Associations between metabolic syndrome and four heavy metals: A systematic review and meta-analysis. Environ Pollut 2021;273:116480. [Crossref] [PubMed]
- Flores CR, Puga MP, Wrobel K, et al. Trace elements status in diabetes mellitus type 2: possible role of the interaction between molybdenum and copper in the progress of typical complications. Diabetes Res Clin Pract 2011;91:333-41. [Crossref] [PubMed]
- Hansen AF, Simić A, Åsvold BO, et al. Trace elements in early phase type 2 diabetes mellitus-A population-based study. The HUNT study in Norway. J Trace Elem Med Biol 2017;40:46-53. [Crossref] [PubMed]
- Pal S, Blais JM, Robidoux MA, et al. The association of type 2 diabetes and insulin resistance/secretion with persistent organic pollutants in two First Nations communities in northern Ontario. Diabetes Metab 2013;39:497-504. [Crossref] [PubMed]
- Zhang J, Wang J, Hu J, et al. Associations of total blood mercury and blood methylmercury concentrations with diabetes in adults: An exposure-response analysis of 2005-2018 NHANES. J Trace Elem Med Biol 2021;68:126845. [Crossref] [PubMed]
- Elmorsy E, Al-Ghafari A, Al Doghaither H, et al. Effects of environmental metals on mitochondrial bioenergetics of the CD-1 mice pancreatic beta-cells. Toxicol In Vitro 2021;70:105015. [Crossref] [PubMed]
- Kim JJ, Kim YS, Kumar V. Heavy metal toxicity: An update of chelating therapeutic strategies. J Trace Elem Med Biol 2019;54:226-31. [Crossref] [PubMed]
- Genchi G, Sinicropi MS, Carocci A, et al. Mercury Exposure and Heart Diseases. Int J Environ Res Public Health 2017;14:74. [Crossref] [PubMed]
- Al Doghaither H, Elmorsy E, Al-Ghafari A, et al. Roles of oxidative stress, apoptosis, and inflammation in metal-induced dysfunction of beta pancreatic cells isolated from CD1 mice. Saudi J Biol Sci 2021;28:651-63. [Crossref] [PubMed]
- Chen YW, Huang CF, Yang CY, et al. Inorganic mercury causes pancreatic beta-cell death via the oxidative stress-induced apoptotic and necrotic pathways. Toxicol Appl Pharmacol 2010;243:323-31. [Crossref] [PubMed]
- Jeppesen C, Valera B, Nielsen NO, et al. Association between whole blood mercury and glucose intolerance among adult Inuit in Greenland. Environ Res 2015;143:192-7. [Crossref] [PubMed]
- He K, Xun P, Liu K, et al. Mercury exposure in young adulthood and incidence of diabetes later in life: the CARDIA Trace Element Study. Diabetes Care 2013;36:1584-9. [Crossref] [PubMed]
- Yoshida M, Lee JY, Satoh M, et al. Neurobehavioral effects of postnatal exposure to low-level mercury vapor and/or methylmercury in mice. J Toxicol Sci 2018;43:11-7. [Crossref] [PubMed]
- Wells EM, Kopylev L, Nachman R, et al. Total Blood Mercury Predicts Methylmercury Exposure in Fish and Shellfish Consumers. Biol Trace Elem Res 2022;200:3867-75. [Crossref] [PubMed]
- Siedlewicz G, Korejwo E, Szubska M, et al. Presence of mercury and methylmercury in Baltic Sea sediments, collected in ammunition dumpsites. Mar Environ Res 2020;162:105158. [Crossref] [PubMed]
- Ceccatelli S, Daré E, Moors M. Methylmercury-induced neurotoxicity and apoptosis. Chem Biol Interact 2010;188:301-8. [Crossref] [PubMed]
- Maqbool F, Bahadar H, Hassani S, et al. Biochemical evidence on the potential role of methyl mercury in hepatic glucose metabolism through inflammatory signaling and free radical pathways. J Cell Biochem 2019;120:16195-205. [Crossref] [PubMed]
- Schumacher L, Abbott LC. Effects of methyl mercury exposure on pancreatic beta cell development and function. J Appl Toxicol 2017;37:4-12. [Crossref] [PubMed]