Predicting the occurrence of stress urinary incontinence after prolapse surgery: a machine learning-based model
Highlight box
Key findings
• We developed and validated an extreme gradient boosting (XGBoost) model to predict postoperative stress urinary incontinence (SUI) in patients receiving pelvic organ prolapse (POP) surgeries.
What is known and what is new?
• Several prediction models for postoperative SUI had been established, which cannot be applied to patients receiving transvaginal mesh (TVM) surgery and colpocleisis or those with preoperative subject urinary incontinence (preSUI).
• Previous models had poor discrimination and calibration among a Chinese population. However, our XGBoost model performed well irrespective of preSUI and surgical methods. Body mass index, C, age, Aa, and TVM were the 5 most important predictors in the XGBoost model.
What is the implication, and what should change now?
• This model has the potential in clinical counseling among doctors and patients and may support them in tailored surgical decisions. Its efficacy still needs to be extensively verified under various scenarios.
Introduction
One study indicated that 8% to 40% of patients with pelvic organ prolapse (POP) develop bothersome stress urinary incontinence (SUI) after prolapse surgery (1). Concomitant incontinence surgery may reduce the occurrence of postoperative SUI and improve quality of life (1,2). However, no unified standard exists for predicting postoperative SUI occurrence. Therefore, the decision to perform concomitant incontinence surgery remains a dilemma faced by clinicians due to its uncertain necessity, potential complications, and expenses (2). The decision should be made based on adequate preoperative evaluation findings to avoid overtreatment. Therefore, accurate individual risk prediction is key to preoperative decision-making.
Promising progress has been made because 3 prediction models have been established for postoperative SUI. Jelovsek et al. (3) developed the first model using data from a clinical trial (the 2014 model). Its predictive performance was significantly better than that of preoperative urinary stress testing [area under the receiver operating characteristic curve (AUC) 0.72 vs. 0.54; P<0.001] (3,4). However, this model can only be applied to women without preoperative SUI symptoms. Furthermore, this model did not have adequate predictive performance in external validation (AUC 0.58–0.63) (4). Subsequently, van der Ploeg et al. (5) constructed a second prediction model using data from other trials, which performed well (the 2019 model: AUC 0.74). Compared to the 2014 model, the 2019 model was suitable for women with or without preoperative SUI (5). Nevertheless, this model does not consider patients undergoing abdominal prolapse surgery or colpocleisis (6). Oh et al. (7) recently developed a new model based on data collected from 2 tertiary hospitals in South Korea (the 2022 model). The 2022 model included women undergoing colpocleisis, native tissue repair, and sacrocolpopexy with mesh. It was similarly efficient compared to the 2014 and 2019 models (AUC 0.74); however, transvaginal mesh (TVM) procedures were not considered, but these remain a major surgical option in East Asia (7-9).
Whether these existing models are suitable for Chinese patients remains questionable. Moreover, existing models cannot be applied to patients undergoing TVM surgery or colpocleisis or those with preoperative subject urinary incontinence. This study attempted to externally validate existing models for postoperative SUI in a Chinese population. In addition, we aimed to fill these gaps in previous models by developing a new prediction model for postoperative bothersome SUI that would suit women undergoing surgeries, including colpocleisis or TVM, regardless of preoperative SUI. We present the following article in accordance with the TRIPOD reporting checklist (available at https://atm.amegroups.com/article/view/10.21037/atm-22-3648/rc).
Methods
Patient selection
The medical records of 731 patients who underwent prolapse surgeries between January 1, 2015, and December 31, 2019, at Peking Union Medical College Hospital, China, were collected in this retrospective cohort study. Patients were included if they (I) were aged over 18 years, (II) had pelvic organ prolapse quantification (POP-Q) stage 2–4 anterior or apical prolapse, (III) were with or without preoperative urinary incontinence, and (IV) were with or without concomitant urinary incontinence surgery. Patients were excluded if they (I) had a history of any prolapse surgery or urinary incontinence surgery or (II) lacked 1-year follow-up results. This study was conducted in accordance with the Declaration of Helsinki (revised in 2013), and the Institutional Review Board of the Peking Union Medical College Hospital approved it (No. JS-2265). The requirement for individual consent for this retrospective analysis was waived. Information was anonymized prior to the analysis.
Term definition
According to previous studies, the following variables were selected as candidate predictors: age, body mass index (BMI), vaginal parity, menstrual status, smoking, alcohol use, chronic constipation, chronic cough, hypertension, diabetes mellitus, Aa, Ba, C, maximum POP-Q stage, preoperative subjective urinary incontinence (preUI), residual urine volume, 1-hour pad test, prior hysterectomy, surgery method for vault suspension, anterior or posterior vaginal repair, and concomitant urinary incontinence surgery (3,5,7). BMI, vaginal parity, Aa, Ba, and C were the continuous and binary variables dichotomized by cutoff values set in previous studies and/or Youden indices (5,7,10). PreUI was defined as a positive answer to questions 16 or 17 in the Chinese version of the Pelvic Floor Distress Inventory-20 (PFDI-20) or the presence of similar descriptions in the medical records (11). Residual urine volume was estimated using an abdominal ultrasound. The results of the prolapse reduction stress test and ordinary 1-hour pad test were collected as the variable “pad test”. Surgical methods for vault suspension were categorized as LeFort/colpocleisis, sacrocolpopexy, native tissue repair (uterosacral ligament suspension, sacrospinous ligament fixation, and ischial spinous fascia fixation), and TVM. All surgeries were performed by experienced clinicians. TVM was performed using self-cut mesh or a mesh kit. Sacrocolpopexy was performed using a pre-cut Y-shaped mesh. The procedures have been described previously (12,13). Vault suspension and anterior or posterior prolapse repair were applied to some patients concomitantly. Anterior and posterior vaginal repairs were listed as independent variables rather than native tissue repair for vault suspension to clarify their individual influence. Urinary incontinence surgery included the Burch procedure, tension-free vaginal tape, and transobturator tape.
The outcome was any bothersome SUI symptom and/or subsequent treatment 1 year postoperatively. Bothersome SUI symptoms were considered if the patient reported “moderately” or “great” to question 17 in the PFDI-20 or if similar descriptions were documented in medical or telephone follow-up records. Outpatient postoperative observation was performed by pelvic floor disease experts, while the questionnaire investigation and documentation were completed by experienced residents.
Missing data
Missing data were imputed using the median for continuous variables and the most frequent values for categorical variables. Variables with greater than 10% missing data were excluded from further analyses (14).
Model validation, construction, and evaluation
First, validation of existing logistic models was performed using the entire dataset. Second, the dataset was randomly sampled into a development set and an external validation set at a 4:1 ratio. The smaller group was used solely for external validation. Logistic regression, random forest, and extreme gradient boosting (XGBoost) were used to construct prediction models. Random forest and XGBoost were performed by the “randomForest” and “xgboost” R packages (the R Foundation for Statistical Computing), respectively. Variables with P values less than 0.1 in the univariate analysis were used in the multivariate analysis. Forward selection was performed based on Akaike information criterion. Random forest and XGBoost are popular machine learning strategies that explore high-dimensional relationships between predictors and outcomes (15). Feature selection was performed using the random forest algorithm, and nested 5-fold cross-validation was subsequently performed with the “mlr” package. The nested 5-fold cross-validation had an inner loop nested in the outer loop. The outer loop was used for internal validation in similar fashion to the procedure for ordinary cross-validation. The differences resided in the inner loop, which tuned the hyperparameters of the model in each fold. The hyperparameters were tuned using random or grid searches.
The model performance was assessed using discrimination and calibration, with AUC representing the discrimination ability. An AUC value greater than 0.6 indicated acceptable discrimination, and an AUC value greater than 0.7 indicated good discrimination. Model calibration was tested using the Spiegelhalter z test and mean absolute error (MSE), and the calibration was visualized using calibration curves. A P value for the z test greater than 0.05 indicated good calibration. MSE was used to quantify the difference between the ideal and actual calibration curves. Smaller values indicated better model calibration. The ideal calibration curve had a slope of 1 and an intercept of 0. The machine learning model was interpreted using importance ranking. The importance of variables was indicated by the information gain or Gini index.
Statistical analysis
Continuous variables are presented as their mean and standard deviation. Categorical variables are presented as counts and percentages. The mean and median differences were evaluated using the t-test and the Wilcoxon signed-rank test, respectively (16). Group differences were evaluated using the chi-square or Fisher exact tests. Statistical significance was set at a P value less than 0.05. All statistical analyses were performed using the R 4.1.2 (RRID: SCR_001905).
Results
Participants
Overall, 555 patients were enrolled in the study, with 445 and 110 randomly assigned to the development and external validation sets, respectively. Detailed patient selection procedures are presented in Figure 1. The characteristics of both datasets are summarized in Table 1. All characteristics were balanced between the sets. Most patients experienced preoperative urinary incontinence and POP-Q stage 3 prolapse. TVM and native tissue repair were the most common surgical methods in our population. Pad test results were missing in 10.6% of the patients; thus, this variable was excluded from further analyses. Notably, 15 patients who did not undergo vault surgery also underwent anterior vaginal repair. Only 1 patient who had previously received tension-free vaginal tape and sacrospinous ligament fixation underwent reoperation treatment within 1 year. A total of 116 (20.9%) patients reported bothersome postoperative SUI, 93 of whom were from the development set.
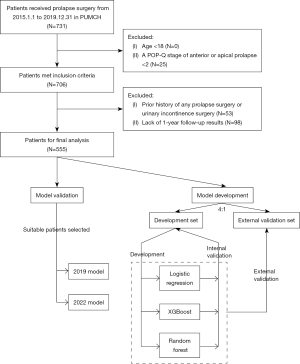
Table 1
Variables | Total (n=555), n (%) | Development set (n=445), n (%) | External validation set (n=110), n (%) | P value | Missing, % |
---|---|---|---|---|---|
Age (years), mean (SD) | 59.6 (10.8) | 59.5 (10.6) | 59.7 (11.4) | 0.919 | 0.0 |
Vaginal parity, mean (SD) | 1.7 (1.0) | 1.7 (1.1) | 1.7 (0.9) | 0.748 | 0.0 |
Menopause | 410 (73.9) | 329 (73.9) | 81 (73.6) | 1.000 | 0.0 |
BMI (kg/m2), mean (SD) | 24.5 (2.8) | 24.5 (2.8) | 24.3 (2.9) | 0.404 | 0.0 |
Smoker | 3 (0.5) | 1 (0.2) | 2 (1.8) | 0.189 | 0.0 |
Alcohol | 14 (2.5) | 11 (2.5) | 3 (2.7) | 1.000 | 0.0 |
Chronic constipation | 24 (4.3) | 16 (3.6) | 8 (7.3) | 0.151 | 0.2 |
Chronic cough | 10 (1.8) | 7 (1.6) | 3 (2.7) | 0.678 | 0.4 |
HTN | 196 (35.3) | 155 (34.8) | 41 (37.3) | 0.713 | 0.2 |
DM | 63 (11.4) | 52 (11.7) | 11 (10.0) | 0.741 | 0.2 |
PreUI | 334 (60.2) | 272 (61.1) | 62 (56.4) | 0.421 | 0.0 |
POP-Q stage | 0.758 | 0.0 | |||
2 | 33 (5.9) | 28 (6.3) | 5 (4.5) | ||
3 | 438 (78.9) | 349 (78.4) | 89 (80.9) | ||
4 | 84 (15.1) | 68 (15.3) | 16 (14.5) | ||
Aa (cm), mean (SD) | 0.9 (1.4) | 0.9 (1.4) | 1.0 (1.4) | 0.717 | 0.2 |
Ba (cm), mean (SD) | 2.7 (2.5) | 2.7 (2.5) | 2.7 (2.5) | 0.832 | 0.2 |
C (cm), mean (SD) | 2.4 (2.8) | 2.5 (2.8) | 2.1 (2.8) | 0.218 | 0.2 |
Residual urine volume (mL), mean (SD) | 20.4 (56.0) | 20.7 (58.0) | 19.5 (47.2) | 0.848 | 5.9 |
Prior hysterectomy | 61 (11.0) | 43 (9.7) | 18 (16.4) | 0.066 | 0.2 |
Positive pad test | 350 (63.1) | 285 (64.0) | 65 (59.1) | 0.393 | 10.6 |
Vault surgery | 0.654 | 0.0 | |||
None | 15 (2.7) | 12 (2.7) | 3 (2.7) | ||
LeFort/colpocleisis | 87 (15.7) | 65 (14.6) | 22 (20.0) | ||
Sacrocolpopexy | 74 (13.3) | 60 (13.5) | 14 (12.7) | ||
TVM | 196 (35.3) | 162 (36.4) | 34 (30.9) | ||
Native tissue repair | 183 (33.0) | 146 (32.8) | 37 (33.6) | ||
AVR | 52 (9.4) | 42 (9.4) | 10 (9.1) | 1.000 | 0.0 |
PVR | 99 (17.8) | 82 (18.4) | 17 (15.5) | 0.555 | 0.0 |
UI surgery | 48 (8.6) | 41 (9.2) | 7 (6.4) | 0.446 | 0.0 |
Bothersome SUI | 116 (20.9) | 93 (20.9) | 23 (20.9) | 1.000 | 0.0 |
SD, standard deviation; HTN, hypertension; DM, diabetes mellitus; BMI, body mass index; PreUI, preoperative subjective urinary incontinence; POP-Q, pelvic organ prolapse quantification; TVM, transvaginal mesh; AVR, anterior vaginal repair; PVR, posterior vaginal repair; UI, urinary incontinence; SUI, stress urinary incontinence.
Model validation
Detailed equations of previous models are summarized in the supplementary (Appendix 1). The stress test was mandatory in the 2014 model; however, it was not routinely performed in clinical centers, including our center, a fact also reported by Oh et al. (3,7). Therefore, only the 2019 and 2022 models were validated (3). Notably, the 2019 and 2022 models excluded patients who underwent colpocleisis or TVM. This resulted in the 2019 and 2022 model validation consisting of 468 and 359 patients, respectively (6,7). Comparisons of baseline characteristics are presented in Table 2. Distinct baseline discrepancies were observed in the different populations. As presented in Figure 2, the AUC for the 2019 and 2022 logistic models was 0.544 and 0.586, respectively, demonstrating a frustratingly dismal degree of discrimination for our population. Their calibration abilities were also poor.
Table 2
Variables | 2019 model | Ours 2019 | P value | 2022 model | Ours 2022 | P value |
---|---|---|---|---|---|---|
Number | 356 | 468 | – | 915 | 359 | – |
Age (years), mean (SD) | 60 (10.0) | 57 (10.0) | <0.001 | 67 [61–72]† | 55 [49–68]† | <0.001 |
Ba (cm), mean (SD) | 1.2 (1.8) | 2.5 (2.6) | <0.001 | – | – | – |
Parity, mean (SD) | 2.4 (1.2) | 1.6 (0.9) | <0.001 | – | – | – |
PreUI, n (%) | 227 (64.0) | 278 (60.0) | 0.230 | 617 (67.0) | 200 (56.0) | 0.003 |
UI surgery, n (%) | 103 (29.0) | 44 (9.4) | <0.001 | 466 (51.0) | 37 (10.0) | <0.001 |
Diabetes mellitus, n (%) | – | – | – | 153 (17.0) | 34 (10.0) | 0.002 |
Sacrocolpopexy, n (%) | – | – | – | 365 (40.0) | 74 (21.0) | <0.001 |
†, data are presented as median (interquartile range). SD, standard deviation; PreUI, preoperative subjective urinary incontinence; UI, urinary incontinence.
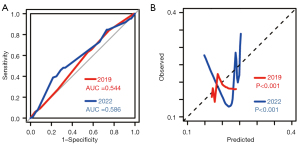
Logistic regression model
Univariate analysis was used for all variables. Youden age (age dichotomized by its Youden index), LeFort/colpocleisis, and TVM had P values of less than 0.1. However, none of the variables remained significant in the multivariate analysis. The results of univariate and multivariate analyses are presented in Table 3. After Akaike information criterion selection, Youden age, LeFort/colpocleisis, and TVM were used to construct the model. The model exhibited adequate calibration (P value for the z test >0.05); however, its mean AUC was 0.631 in the 5-fold cross-validation (Table 4).
Table 3
Variables | Univariate | Multivariate | |||
---|---|---|---|---|---|
OR (95% CI) | P value | OR (95% CI) | P value | ||
YoudenAge | 1.676 (1.043–2.679) | 0.031 | 1.456 (0.780–2.668) | 0.229 | |
LeFort/colpocleisis | 2.227 (1.238–3.926) | 0.006 | 1.447 (0.658–3.186) | 0.357 | |
TVM | 0.616 (0.368–1.007) | 0.058 | 0.665 (0.374–1.156) | 0.154 |
OR, odds ratio; CI, confidence interval; YoudenAge, age dichotomized by its Youden index; TVM, transvaginal mesh.
Table 4
Terms | Logistic regression | Random forest | XGBoost |
---|---|---|---|
Development | |||
AUC (95% CI) | 0.595 (0.532–0.657) | 0.842 (0.798–0.887) | 0.714 (0.658–0.770) |
MSE | 0.020 | 0.030 | 0.029 |
z test | 0.989 | <0.001 | 0.321 |
Internal validation | |||
Mean AUC | 0.631 | 0.648 | 0.721 |
External validation | |||
AUC (95% CI) | 0.593 (0.472–0.715) | 0.603 (0.485–0.721) | 0.704 (0.588–0.820) |
MSE | 0.045 | 0.046 | 0.042 |
z test | 0.855 | <0.001 | 0.688 |
Accuracy | 0.727 | 0.400 | 0.636 |
AUC, the area under the receiver operating characteristic curve; CI, confidence interval; MSE, mean absolute error; XGBoost, extreme gradient boosting.
Machine learning model
Feature selection was performed using the random forest algorithm (Table 5). The random forest algorithm showed that BMI, age, C, Ba, Aa, and parity had greater importance as continuous variables than as categorical variables. Therefore, they were input in a continuous form.
Table 5
Variable | Mean decrease Gini |
---|---|
BMI | 21.7638677 |
Age | 16.9901682 |
C | 13.1484941 |
Ba | 10.0808777 |
Aa | 9.06516993 |
Residual urine volume | 7.12244053 |
Parity | 6.26720427 |
Preoperative subjective urinary incontinence | 4.48973485 |
Hypertension | 3.56909667 |
LeFort/colpocleisis | 3.04690016 |
BMI (dichotomized by the cutoff value used in previous studies) | 2.94123521 |
Parity (dichotomized by its Youden index) | 2.93749152 |
Maximum POP-Q stage | 2.83415798 |
Transvaginal mesh surgery | 2.48664602 |
Posterior vaginal repair | 2.4817805 |
Age (dichotomized by its Youden index) | 2.27182237 |
BMI (dichotomized by its Youden index) | 2.14462723 |
Native tissue repair | 1.98841133 |
Diabetes mellitus | 1.88297761 |
Age (dichotomized by the cutoff value used in previous studies) | 1.80000975 |
Menopause | 1.75891459 |
Sacrocolpopexy | 1.66321171 |
Prior hysterectomy | 1.60104766 |
C (dichotomized by the cutoff value used in previous studies) | 1.48065575 |
Anterior vaginal repair | 1.44897331 |
Urinary incontinence surgery | 1.33539588 |
Parity (dichotomized by the cutoff value used in previous studies) | 0.8831204 |
Alcohol | 0.86679148 |
Chronic constipation | 0.78995965 |
Aa (dichotomized by the cutoff value used in previous studies) | 0.59923558 |
Ba (dichotomized by the cutoff value used in previous studies) | 0.47033086 |
Chronic cough | 0.22500867 |
Smoker | 0.03003707 |
BMI, body mass index; POP-Q, pelvic organ prolapse quantification.
The tuned hyperparameters of the random forest model were ntree =300, mtry =2, nodesize =24, and maxnode =19. The random forest model had excellent discrimination ability in the development set (AUC 0.842; 95% CI: 0.798–0.887); however, the AUC dropped to 0.648 and 0.603 for the internal and external validation, respectively. Moreover, the calibration ability was poor (P<0.001 for the z test). As for the XGBoost model, the hyperparameters were set as booster = “gbtree,” max_depth =12, eta =0.286, min_child_weight =14.9, subsample =0.877, colsample_bytree =0.823, gamma =2, objective = “binary:logistic,” nround =25, and eval_metric = “auc.” The XGBoost model maintained good AUC (AUC >0.7) regardless of the development set or internal and external validation. In addition, it had an acceptable calibration ability (Table 4; Figure 3). In the external validation set, its sensitivity, specificity, and accuracy at a Youden index of 0.207 were 0.783, 0.598, and 0.636, respectively. The feature importance is plotted in Figure 4. The top 5 variables for the XGBoost model were BMI, C, age, Aa, and TVM, whereas the top 5 variables for the random forest model were BMI, age, C, residual urine volume, and Ba.
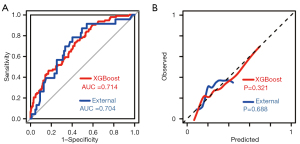
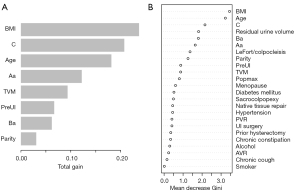
Discussion
Data collected from 555 patients who underwent POP surgery were included in the final analysis. Existing prediction models were evaluated in this population; however, none exhibited adequate performance. Subsequently, 3 new prediction models were developed via machine learning. The XGBoost model exhibited the best discrimination and calibration abilities of the 3 new models irrespective of preUI and surgical methods.
By pooling data from 555 patients enrolled in a retrospective cohort study of prolapse surgery, our study included an adequate amount of information comparable to that of previous studies (3,5,7). From a methodological point of view, this is the first comprehensive machine learning–based study to establish a prediction model for postoperative bothersome SUI. Its performance was validated internally and externally. Unlike previous studies, we did not exclude patients receiving colpocleisis or TVM or those with preUI, which allowed for greater generalizability of the study findings. A long-term multi-institutional study of Chinese prolapse patients revealed that the rate of synthetic mesh procedures was 46% and that TVM was still a common surgical choice (9). Accordingly, our model may have better local adaptability than that of existing models.
The 2019 and 2022 logistic models did not perform satisfactorily in this population. The decreased prediction efficacy may be due to several factors. An important reason for this is the discrepancies between the development and the validation populations, especially in the validation of data from other countries with differences in race, culture, and health systems. According to Table 2, most characteristics significantly differed between Chinese patients and the populations used in the existing models.
However, the newly developed logistic regression model was not satisfactory. Collecting more specific variables or performing more efficient modeling should be considered to improve the model’s performance. We collected the variables based on existing models or correlated postoperative SUI, ensuring their predictive ability. Targeting a population with more significant heterogeneity might affect the efficacy of these variables, which is a common phenomenon. Additionally, conventional logistic regression may be limited by distribution normality, non-informative or random censoring, and hazard risk linearity (17). The hidden nonlinear relationship between the variables and outcomes was difficult to determine using logistic regression, and it may be challenging to develop a more generalized model.
To better capture high-dimensional nonlinear relationships, we resorted to other machine learning methods. Machine learning classification tools are commonly used to estimate health outcome risks with relatively high and stable performances in diverse clinical situations (15). Random forest and XGBoost are mainstream variable selection tools based on different mathematical theories. Random forest is commonly used to perform feature selection and handle complex datasets owing to its high cost-effectiveness and interpretation ability (18-21). The XGBoost algorithm was selected due to its advanced ability to handle various inputs, its interpretability, and its internal optimization (22). We used random forest and XGBoost to screen variables and construct prediction models for postoperative bothersome SUI. As previously stated, the AUC values of the XGBoost model remained greater than 0.7 in different sets, and its calibration was good, while the random forest model exhibited poor discrimination and calibration. Therefore, the XGBoost model was used because of its outstanding performance.
Appropriately interpreting risk factors for individuals is also a challenge for clinicians. The interpretation is further complicated by the numerous clinical variables related to the occurrence of postoperative SUI. No independent risk factor was identified via the multivariate analyse; however, BMI, C, age, Aa, and TVM were the 5 most important predictors in the XGBoost model. BMI and age were predictors in previous models, and a higher BMI was correlated with a high risk of postoperative SUI (3,5,7,23-25). However, opinions on the influence of age vary across studies. Older age was a protective factor in the 2014 and 2019 models; nonetheless, it acted as a risk factor in the 2022 model and in several studies (3,5,7,25-27). The Aa point reflects the severity of anterior prolapse, and numerous reports demonstrated advanced anterior prolapse to be associated with postoperative SUI (24,28-30). Previous observations suggest that TVM might cause postoperative SUI due to overcorrection of the bladder neck, urethral supportive defects, and neural denervation (31-33). To our knowledge, there are no studies on the definite impact of point C on postoperative bothersome SUI, and further investigation is needed. Clinicians should be made aware of POP-Q inaccuracy. Ostrzenski (34) suggested that the length of the genital hiatus and perineal body may be enlarged because of muscle detachment. Similar problems may exist when measuring Aa, Ba, and C points. In general, the essential variables observed in our study correspond with clinical practice and previous studies. As indicated by the XGBoost model, BMI was the only intervenable risk factor; therefore, losing weight before surgery might help lower the risk of postoperative SUI.
A few variables generally considered important, such as the prolapse reduction stress test and urodynamic testing, were not included in our prediction model (1,35). Prolapse reduction stress tests performed among patients with heavy pelvic prolapse can reveal the presence of urinary incontinence (1). However, the results of the prolapse reduction stress tests and ordinary 1-hour pad tests were missing in more than 10% of patients. The pad test and prolapse reduction stress test were excluded in our analysis for additional reasons. First, the pad test is not recommended as a routine assessment of urinary incontinence (36). Some remote medical centers do not perform the pad test under some circumstances. Second, the results of an effective pad test rely on standardized procedures because duration and body movement affect the results. The quality of the pad test is difficult to guarantee at different medical centers. Regarding urodynamic testing, it is not recommended for patients with uncomplicated SUI but is proposed for patients experiencing prolapse with urinary incontinence when applicable (37-39). Based on these recommendations, only a few patients underwent urodynamic testing. Moreover, urodynamic testing would induce additional expenses, impairing its potential for extensive use.
Our study had some limitations. This was a single-center retrospective study, which may have inherent selection bias and may be limited in its generalizability. We tried to thoroughly validate the model performance via nested 5-fold cross-validation and external validation. In addition, there were some variables that we could not capture, such as strenuous physical activity, which a group of experts, including Jelovsek et al., have identified as a potential predictor of postoperative SUI (3). However, this variable also did not exhibit significance in the multivariate analysis of the 2014 model (3). New techniques, such as the urethral stabilization procedure, that do not use slings, meshes, or absorbable sutures were not included in our analysis because few patients underwent these surgeries in our country (40). The 95% confidence interval of the XGBoost model was relatively wide, suggesting a potential risk of suboptimal sample size. Therefore, our results should be interpreted with caution.
Conclusions
The existing models did not reach satisfactory discrimination and calibration in this population. Hence, we constructed and validated an XGBoost model to predict bothersome postoperative SUI irrespective of surgical methods and preUI. The XGBoost model simultaneously exhibited good discrimination and calibration, and the most important variables were BMI, C, age, Aa, and TVM. Its efficacy needs to be extensively verified under various scenarios. Nevertheless, we are optimistic that it will gain attention in clinical counseling among doctors and patients and support them in facilitating tailored surgical decisions.
Acknowledgments
Funding: This research was funded by the Beijing Natural Science Foundation (No. Z190021), the National Natural Science Foundation of China (No. 81971366), the CAMS Innovation Fund for Medical Sciences (No. CIFMS 2020-I2M-C&T-B-043), and the National High Level Hospital Clinical Research Funding (No. 2022-PUMCH-B-087).
Footnote
Reporting Checklist: The authors have completed the TRIPOD reporting checklist. Available at https://atm.amegroups.com/article/view/10.21037/atm-22-3648/rc
Data Sharing Statement: Available at https://atm.amegroups.com/article/view/10.21037/atm-22-3648/dss
Conflicts of Interest: All authors have completed the ICMJE uniform disclosure form (available at https://atm.amegroups.com/article/view/10.21037/atm-22-3648/coif). The authors have no conflicts of interest to declare.
Ethical Statement: The authors are accountable for all aspects of the work in ensuring that questions related to the accuracy or integrity of any part of the work are appropriately investigated and resolved. The study was conducted in accordance with the Declaration of Helsinki (as revised in 2013). The study was approved by the Institutional Review Board of Peking Union Medical College Hospital (No. JS-2265), and individual consent for this retrospective analysis was waived.
Open Access Statement: This is an Open Access article distributed in accordance with the Creative Commons Attribution-NonCommercial-NoDerivs 4.0 International License (CC BY-NC-ND 4.0), which permits the non-commercial replication and distribution of the article with the strict proviso that no changes or edits are made and the original work is properly cited (including links to both the formal publication through the relevant DOI and the license). See: https://creativecommons.org/licenses/by-nc-nd/4.0/.
References
- Baessler K, Christmann-Schmid C, Maher C, et al. Surgery for women with pelvic organ prolapse with or without stress urinary incontinence. Cochrane Database Syst Rev 2018;8:CD013108. [Crossref] [PubMed]
- van der Ploeg JM, van der Steen A, Zwolsman S, et al. Prolapse surgery with or without incontinence procedure: a systematic review and meta-analysis. BJOG 2018;125:289-97. [Crossref] [PubMed]
- Jelovsek JE, Chagin K, Brubaker L, et al. A model for predicting the risk of de novo stress urinary incontinence in women undergoing pelvic organ prolapse surgery. Obstet Gynecol 2014;123:279-87. [Crossref] [PubMed]
- Jelovsek JE, van der Ploeg JM, Roovers JP, et al. Validation of a Model Predicting De Novo Stress Urinary Incontinence in Women Undergoing Pelvic Organ Prolapse Surgery. Obstet Gynecol 2019;133:683-90. [Crossref] [PubMed]
- van der Ploeg JM, Steyerberg EW, Zwolsman SE, et al. Stress urinary incontinence after vaginal prolapse repair: development and internal validation of a prediction model with and without the stress test. Neurourol Urodyn 2019;38:1086-92. [Crossref] [PubMed]
- van der Steen A, van der Ploeg M, Dijkgraaf MG, et al. Protocol for the CUPIDO trials; multicenter randomized controlled trials to assess the value of combining prolapse surgery and incontinence surgery in patients with genital prolapse and evident stress incontinence (CUPIDO I) and in patients with genital prolapse and occult stress incontinence (CUPIDO II). BMC Womens Health 2010;10:16. [Crossref] [PubMed]
- Oh S, Lee S, Hwang WY, et al. Development and validation of a prediction model for bothersome stress urinary incontinence after prolapse surgery: A retrospective cohort study. BJOG 2022;129:1158-64. [Crossref] [PubMed]
- Kato K, Gotoh M, Takahashi S, et al. Techniques of transvaginal mesh prolapse surgery in Japan, and the comparison of complication rates by surgeons' specialty and experience. Int J Urol 2020;27:996-1000. [Crossref] [PubMed]
- Sun ZJ, Wang XQ, Lang JH, et al. A 14-year multi-institutional collaborative study of Chinese pelvic floor surgical procedures related to pelvic organ prolapse. Chin Med J (Engl) 2021;134:200-5. [Crossref] [PubMed]
- Youden WJ. Index for rating diagnostic tests. Cancer 1950;3:32-5. [Crossref] [PubMed]
- Ma Y, Xu T, Zhang Y, et al. Validation of the Chinese version of the Pelvic Floor Distress Inventory-20 (PFDI-20) according to the COSMIN checklist. Int Urogynecol J 2019;30:1127-39. [Crossref] [PubMed]
- Chen J, Yu J, Morse A, et al. Self-cut titanium-coated polypropylene mesh versus pre-cut mesh-kit for transvaginal treatment of severe pelvic organ prolapse: study protocol for a multicenter non-inferiority trial. Trials 2020;21:226. [Crossref] [PubMed]
- Liang S, Zhu L, Song X, et al. Long-term outcomes of modified laparoscopic sacrocolpopexy for advanced pelvic organ prolapse: a 3-year prospective study. Menopause 2016;23:765-70. [Crossref] [PubMed]
- Brunelli A, Salati M, Rocco G, et al. European risk models for morbidity (EuroLung1) and mortality (EuroLung2) to predict outcome following anatomic lung resections: an analysis from the European Society of Thoracic Surgeons database. Eur J Cardiothorac Surg 2017;51:490-7. [PubMed]
- Schwalbe N, Wahl B. Artificial intelligence and the future of global health. Lancet 2020;395:1579-86. [Crossref] [PubMed]
- Li H, Johnson T. Wilcoxon's signed-rank statistic: what null hypothesis and why it matters. Pharm Stat 2014;13:281-5. [Crossref] [PubMed]
- Zhou N, Ji Z, Li F, et al. Machine Learning-Based Personalized Risk Prediction Model for Mortality of Patients Undergoing Mitral Valve Surgery: The PRIME Score. Front Cardiovasc Med 2022;9:866257. [Crossref] [PubMed]
- Lebedev AV, Westman E, Van Westen GJ, et al. Random Forest ensembles for detection and prediction of Alzheimer's disease with a good between-cohort robustness. Neuroimage Clin 2014;6:115-25. [Crossref] [PubMed]
- Speiser JL, Miller ME, Tooze J, et al. A Comparison of Random Forest Variable Selection Methods for Classification Prediction Modeling. Expert Syst Appl 2019;134:93-101. [Crossref] [PubMed]
- Li W, Hong T, Liu W, et al. Development of a Machine Learning-Based Predictive Model for Lung Metastasis in Patients With Ewing Sarcoma. Front Med (Lausanne) 2022;9:807382. [Crossref] [PubMed]
- Wang J, Wang Z, Liu N, et al. Random Forest Model in the Diagnosis of Dementia Patients with Normal Mini-Mental State Examination Scores. J Pers Med 2022;12:37. [Crossref] [PubMed]
- Al'Aref SJ, Maliakal G, Singh G, et al. Machine learning of clinical variables and coronary artery calcium scoring for the prediction of obstructive coronary artery disease on coronary computed tomography angiography: analysis from the CONFIRM registry. Eur Heart J 2020;41:359-67. [Crossref] [PubMed]
- Khayyami Y, Elmelund M, Lose G, et al. De novo urinary incontinence after pelvic organ prolapse surgery-a national database study. Int Urogynecol J 2020;31:305-8. [Crossref] [PubMed]
- Cruz RA, Faria CA, Gomes SS Jr. Predictors for de novo stress urinary incontinence following pelvic reconstructive surgery with mesh. Eur J Obstet Gynecol Reprod Biol 2020;253:15-20. [Crossref] [PubMed]
- Hu P, Lei L, Wei L, et al. Investigation of risk factors of de novo urinary stress incontinence after cystocele repair: A retrospective cohort study. Int J Gynaecol Obstet 2022;158:213-5. [Crossref] [PubMed]
- Lo TS, Bt Karim N, Nawawi EA, et al. Predictors for de novo stress urinary incontinence following extensive pelvic reconstructive surgery. Int Urogynecol J 2015;26:1313-9. [Crossref] [PubMed]
- Wu PC, Wu CH, Lin KL, et al. Predictors for de novo stress urinary incontinence following pelvic reconstruction surgery with transvaginal single-incisional mesh. Sci Rep 2019;9:19166. [Crossref] [PubMed]
- Bump RC, Mattiasson A, Bø K, et al. The standardization of terminology of female pelvic organ prolapse and pelvic floor dysfunction. Am J Obstet Gynecol 1996;175:10-7. [Crossref] [PubMed]
- Sato H, Abe H, Ikeda A, et al. Severity of Cystocele and Risk Factors of Postoperative Stress Urinary Incontinence after Laparoscopic Sacrocolpopexy for Pelvic Organ Prolapse. Gynecol Minim Invasive Ther 2022;11:28-35. [Crossref] [PubMed]
- Leruth J, Fillet M, Waltregny D. Incidence and risk factors of postoperative stress urinary incontinence following laparoscopic sacrocolpopexy in patients with negative preoperative prolapse reduction stress testing. Int Urogynecol J 2013;24:485-91. [Crossref] [PubMed]
- Liang CC, Lin YH, Chang YL, et al. Urodynamic and clinical effects of transvaginal mesh repair for severe cystocele with and without urinary incontinence. Int J Gynaecol Obstet 2011;112:182-6. [Crossref] [PubMed]
- Lo TS, Bt Karim N, Cortes EF, et al. Comparison between Elevate anterior/apical system and Perigee system in pelvic organ prolapse surgery: clinical and sonographic outcomes. Int Urogynecol J 2015;26:391-400. [Crossref] [PubMed]
- Oride A, Kanasaki H, Hara T, et al. Postoperative Outcomes Following Tension-Free Vaginal Mesh Surgery for Pelvic Organ Prolapse: A Retrospective Study. Urol J 2019;16:581-5. [PubMed]
- Ostrzenski A. Pelvic Organ Prolapse Quantification (POP-Q) system needs revision or abandonment: The anatomy study. Eur J Obstet Gynecol Reprod Biol 2021;267:42-8. [Crossref] [PubMed]
- Pecchio S, Novara L, Sgro LG, et al. Concomitant stress urinary incontinence and pelvic organ prolapse surgery: Opportunity or overtreatment? Eur J Obstet Gynecol Reprod Biol 2020;250:36-40. [Crossref] [PubMed]
- NICE Guidance - Urinary incontinence and pelvic organ prolapse in women: management: (c) NICE (2019) Urinary incontinence and pelvic organ prolapse in women: management. BJU Int 2019;123:777-803. [Crossref] [PubMed]
- Urogynocology Subgroup, Chinese Society of Obstetrics and Gynocology, Chinese Medical Association. Update of guideline on the diagnosis and treatment of female stress urinary incontinence (2017). Zhonghua Fu Chan Ke Za Zhi 2017;52:289-93. [PubMed]
- Urogynecology Subgroup, Chinese Society of Obstetrics and Gynecology, Chinese Medical Association. Chinese guideline for the diagnosis and management of pelvic orang prolapse (2020 version). Zhonghua Fu Chan Ke Za Zhi 2020;55:300-6. [PubMed]
- Nambiar AK, Arlandis S, Bø K, et al. European Association of Urology Guidelines on the Diagnosis and Management of Female Non-neurogenic Lower Urinary Tract Symptoms. Part 1: Diagnostics, Overactive Bladder, Stress Urinary Incontinence, and Mixed Urinary Incontinence. Eur Urol 2022;82:49-59. [Crossref] [PubMed]
- Ostrzenski A. The new etiology and surgical therapy of stress urinary incontinence in women. Eur J Obstet Gynecol Reprod Biol 2020;245:26-34. [Crossref] [PubMed]
(English Language Editors: C. Mullens and J. Gray)