Current status and trends of artificial intelligence research on the four traditional Chinese medicine diagnostic methods: a scientometric study
Highlight box
Key findings
• AI research on the four TCM diagnostic methods has been developed since 2007; China has the highest number of authors, articles, and institutions in this field, but its influence needs to be strengthened. Tongue diagnosis and classification based on deep neural networks will be a research hotspot in this field for a long time.
What is known and what is new?
• Analysis of large amounts of complicated clinical data using AI methods has been widely applied in the field of tongue and pulse research.
• Through bibliometric methods, we assessed and visualized the current status of AI-based research on the four TCM diagnostic methods according to the country, journal, organization, author, keywords, etc.
What is the implication, and what should change now?
• There is a need for more interdisciplinary, cross-country, and cross-institutional collaboration in AI research on the four TCM diagnostic methods.
Introduction
The four traditional Chinese medicine (TCM) diagnoses are a non-intrusive approach for doctors to diagnose diseases and involve inspection, auscultation, inquiring, and palpation. These diagnostic methods have been widely used in China for the past 3,000 years (1). Tongue, pulse, and facial diagnoses are recognized diagnostic methods in TCM, which are based on the overall evaluation of the heart, liver, spleen, lungs, and kidneys inside the human body. With the occurrence of disease, the changes in the functions of blood vessels and organs will be reflected in the tongue, pulse, and face manifestations. Thus, the color, thickness, frequency, and smell of these areas can be used to understand the severity and cause of disease. With the rapid development of TCM research, the four TCM diagnoses have advanced accordingly with modern science and technology (2), including artificial intelligence (AI). AI was proposed by McCarthy et al. in the 50s of the 20th century (3). Since then, AI has been rapidly developed and widely used in different fields such as finance and medicine. In recent years, due to the development of big data in TCM, the field of image analysis of TCM has become a hot spot in artificial intelligence research. With predictive analytics on AI-based medical images, doctors can make better diagnosis and treatment decisions. Many meaningful tongue and pulse features for the diagnosis of various diseases have been discovered using large amounts of complicated clinical data via AI-based data mining methods. For instance, the multi-step approach (4), Genetic Algorithm_Extreme Gradient Boosting (GA_XGBT) model (5), random forest (6), and convolutional neural network (MIMT-CNN) (7) have been widely applied in diabetic tongue research, while AdaBoost (8), Support Vector Machine (SVM) (9), and logistic regression (10) have been applied in pulse research. The use of AI to understand the clinical data of diseases can help to objectively and efficiently improve the accuracy and precision of diagnosis (2).
Up to now, AI-directed four TCM diagnoses have achieved significant progress in several fields. However, there are still difficulties in the development of AI-directed four TCM diagnostic methods, including model interpretation, unstable and sufficient clinical data, external model validation, explaining the relationship between the machine learning model and ancient Chinese philosophy, the quantification of smelling, the standardization of tools for collecting data, and well-designed storage databases or cloud database creation. Scientometric study is a quantitative method of studying the impact of a society and comparing its impact at different national levels (11). It can objectively show the contribution of a research field and analyze the hot spots and trends in that research field. In this study, we applied scientometric approaches and visualization tools to integrate information.
This review summarizes the current status and trends of AI research to discover novel information about the four TCM diagnostic methods and develop and investigate this TCM approach and its challenges.
Methods
Search strategy and selection criteria
The Science Citation Index Expanded (SCI-Expanded, 1999–present) of Clarivate Analytics Web of Science Core Collection (WoSCC) is one of the most authoritative and comprehensive databases in various disciplines, comprising a wide range of academic journals and literature, and is widely used as the data source for bibliometric study (12). Literature on the AI studies of the four TCM diagnoses from the WoSCC was collected. Two researchers conducted an independent search to ensure accuracy and consistency, and the search period was from the inception of the database [1980] to October 1, 2022.
The following search terms/parameters were applied: TS = (“tongue” OR “face” OR “pulse*” OR “symptom” OR “syndrome” OR “voice” OR “lip” OR “sublingual vein”) AND (“TCM” OR “Traditional Chinese medicine” OR “Chinese medicine”) AND (“deep learning” OR “machine learning” OR “artificial intelligence”) AND Language = (English) (13). Data including titles, authors, keywords, citations, journals, institutions, and references of the publications were saved, and a total of 136 effective studies were obtained from 2007 to 2022.
Data analysis
First, the documents were imported to Citespace (version 6.1.R3, Drexel University, United States) to remove duplicates. Next, Microsoft Excel 2019 (Redmond, WA, USA) was used to conduct data aggregation and analysis, and VOSviewer software (version 1.6.18, https://www.vosviewer.com/, accessed on 15 Sep 22) was used to create the bibliometric networks (14). In this study, the retrieval characteristics of the four TCM diagnoses for AI included the distribution of publication years, regions, organizations, journals, core authors, keywords, and key references. For the co-authorship map, the authors, keywords, etc. were introduced into the software as a unit and linked to each other based on the number of joint articles and keywords. The results were displayed using density visualizations.
In the network visualization map, nodes formed clusters, which were characterized by colors, circles, lines, and the number of clusters. Different clusters have different colors; large circles indicated that terms were more important and closer clusters represented strong relationships. The line between the terms represented the relationship, with thicker lines denoting stronger correlations between the two terms (15). Citespace (version 6.1.R3) software was utilized to accomplish the timeline of co-citation analysis of the keywords and burst keywords. A free online platform (https://www.mapchart.net/) was used to conduct a map of the countries.
Results
Annual publications and trends
The annual number of papers published on AI in the four TCM diagnoses is shown in Figure 1. From 2007–2017, the number of papers published was fewer than 10, indicating a slow start to the study of the four TCM diagnostic methods by AI. From 2018, the number of papers published exceeded 10, with an overall upward trend. In particular, the highest number of articles was 32, which was achieved in 2020. Recently, AI studies on the four TCM diagnoses have received increasing attention.
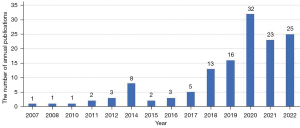
Analysis of countries
We analyzed the national and regional characteristics of AI studies of the four TCM diagnostic methods. The results showed that among 15 countries that had published articles in this field, the majority of papers were from China (n=126), indicating a significant central tendency. Also, the United States and Canada were identified in more than five articles. Table 1 summarizes the top 10 countries with the highest frequency. China, Canada, and the United States were the highest-ranked countries and had been cited more than 50 times. Despite this, Japan accounted for the highest number of citations per paper. Intelligence studies of the four TCM diagnoses were mainly distributed in Eastern Asia, North America, and Europe, as shown in Figure 2.
Table 1
Rank | Countries | Number of articles | Total citations | Average citations |
---|---|---|---|---|
1 | China | 125 | 863 | 6.90 |
2 | Canada | 7 | 81 | 11.57 |
3 | USA | 7 | 86 | 12.29 |
4 | India | 5 | 15 | 3.00 |
5 | Australia | 4 | 19 | 4.75 |
6 | South Korea | 4 | 21 | 5.25 |
7 | England | 2 | 14 | 7.00 |
8 | Germany | 2 | 9 | 4.50 |
9 | Italy | 2 | 1 | 0.50 |
10 | Japan | 2 | 25 | 12.50 |
TCM, traditional Chinese medicine.
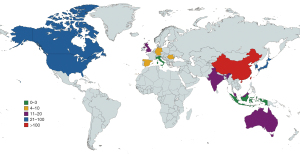
Analysis of journals and conferences
The journals and conferences published on the study of the four TCM diagnostic methods were analyzed, and the characteristics of the journals and conferences, such as article numbers, total citation status, and average citation status, were recorded and quantified. Based on the data analysis, documents related to AI in the four TCM diagnoses published from 2007 to 2022 were distributed in 81 different journals and conferences. Evidence-Based Complementary and Alternative Medicine and IEEE Access published the most papers in this field, with more than 10 publications per journal. It is worth noting that the 2020 IEEE International Conference on Bioinformatics and Biomedicine published four articles in this field (Table 2).
Table 2
Rank | Journal title | Number of articles | Total citations | Average citations |
---|---|---|---|---|
1 | Evidence-Based Complementary and Alternative Medicine | 10 | 70 | 7.00 |
2 | IEEE Access | 10 | 66 | 6.60 |
3 | JMIR Medical Informatics | 6 | 27 | 4.50 |
4 | Biomed Research International | 5 | 54 | 10.80 |
5 | Concurrency and Computation-Practice & Experience | 5 | 41 | 8.20 |
6 | 2020 IEEE International Conference on Bioinformatics and Biomedicine | 4 | 5 | 1.25 |
7 | Computational and Mathematical Methods in Medicine | 4 | 35 | 8.75 |
8 | Journal of Healthcare Engineering | 4 | 2 | 0.50 |
9 | Artificial Intelligence in Medicine | 3 | 82 | 27.33 |
10 | European Journal of Integrative Medicine | 3 | 32 | 10.67 |
11 | Multimedia Tools and Applications | 3 | 12 | 4.00 |
TCM, traditional Chinese medicine.
The VOSviewer visualization map of the most commonly cited journals related to AI in the four TCM diagnoses showed that of the 1,919 cited journals, 45 met the threshold (the minimum number of citations of a source was 15). As shown in Figure 3, the co-citation network of the journal consisted of three clusters: red, blue, and green. The IEEE Conference on Computer Vision and Pattern Recognition (CVPR IEEE) had a total of 133 citations, which was higher than that of any other conference or journal. Notably, the Evidence-Based Complementary and Alternative Medicine journal had the highest number of citations among the journals (126 times). In the three clusters, the red cluster journals were mainly in the field of TCM, focusing on the application of AI in TCM, and articles mainly cited these journals to analyze and review existing research and provide empirical support for their own research. Green was mainly comprised of journals in the IEEE field, and these are often technical journals. Moreover, the blue cluster journals were mainly general and neurological journals, and the purpose of citing these journals was mainly to provide technical support. Each point in the density visualization has a color that indicates the density of the journal; the larger the number of journals in the neighborhood of a point and the higher the weights of the neighboring journal, the closer the color of the point is to yellow (Figure 4).
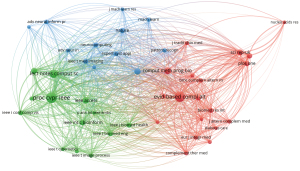
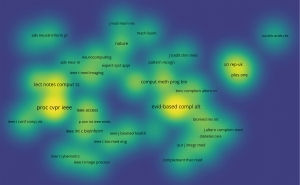
Analysis of authors
A total of 652 authors participated in the publication of papers on AI in the four TCM diagnostic methods. Table 3 lists the authors who published more than four articles. The most productive authors were mostly from China. Xiaojuan Hu, Jiatuo Xu, and Liping Tu were the top-three ranked authors, with 9, 9, and 8 papers respectively. Meanwhile, although Jinhong Guo and Weihong Li published fewer papers, their average citations were as high as 23.75 and 22.75, respectively. Figure 5 displays the analysis results of the cooperative network among authors who had published at least one paper. The size of the circle in the figure indicates the extent of the author’s cooperation, the width of the line connecting the authors indicates the strength of cooperation, and the color indicates the cluster network of the subgroups. The collaborative network of authors in AI studies of the four TCM diagnoses presents the trend of centralized characteristics and regional concentration.
Table 3
Rank | Author | Documents | Citations | Average citations |
---|---|---|---|---|
1 | Xiaojuan Hu | 9 | 85 | 9.44 |
2 | Jiatuo Xu | 9 | 84 | 9.33 |
3 | Liping Tu | 8 | 82 | 10.25 |
4 | Ji Cui | 6 | 59 | 9.83 |
5 | Guihua Wen | 6 | 33 | 5.50 |
6 | Zhikui Chen | 5 | 61 | 12.20 |
7 | Jun Li | 5 | 27 | 5.40 |
8 | Changchuan Bai | 4 | 71 | 17.75 |
9 | Longtao Cui | 4 | 26 | 6.50 |
10 | Jinhong Guo | 4 | 95 | 23.75 |
11 | Jingbin Huang | 4 | 26 | 6.50 |
12 | Tao Jiang | 4 | 33 | 8.25 |
13 | Peng Li | 4 | 61 | 15.25 |
14 | Weihong Li | 4 | 91 | 22.75 |
15 | Qingchen Zhang | 4 | 71 | 17.75 |
16 | Change Zhou | 4 | 26 | 6.50 |
TCM, traditional Chinese medicine.
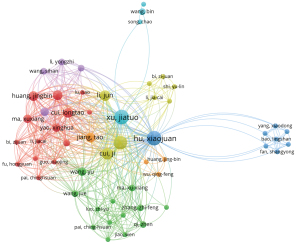
Moreover, cluster analysis identified seven cluster cooperative teams. Among them, Longtao Cui, Zhifeng Zhang, and Jiatuo Xu were considered high-yielding scholars with greater influence. A co-citation (cited authors) visualization map was also generated using VOSviewer software. A total of 39 authors who met the threshold (the minimum number of citations of an author was set to 10) were identified among 2,864 authors. Through analysis of co-citation (cited authors), we classified the main authors in the field of AI for the four TCM diagnostic methods according to this “distance”. We observed that Zhang B, He KM, and Wang XZ had made significant contributions to AI for the four TCM diagnoses (Figure 6).
Analysis of the institutions and funding agencies
A total of 200 institutions participated in publishing research papers on AI for the four TCM diagnoses. The most productive institutions among the top 10 were the Shanghai University of Traditional Chinese Medicine, followed by Xiamen University and the China Academy of Chinese Medical Sciences. The top 10 ranked institutions were all Chinese. There were five TCM institutions in the top 10 but the total number of citations of the TCM institutions (n=343) was lower than that of non-TCM institutions (n=372), as shown in Table 4.
Table 4
Rank | Organizations | Documents | Citations | Average citations |
---|---|---|---|---|
1 | Shanghai University of Traditional Chinese Medicine | 18 | 135 | 7.50 |
2 | Xiamen University | 11 | 65 | 5.91 |
3 | China Academy of Chinese Medical Sciences | 9 | 40 | 4.44 |
4 | University of Science and Technology of China | 7 | 133 | 19 |
5 | Tsinghua University | 7 | 80 | 11.43 |
6 | Chengdu University of Traditional Chinese Medicine | 6 | 121 | 20.17 |
7 | Beijing University of Traditional Chinese Medicine | 6 | 11 | 1.83 |
8 | South China University of Technology | 6 | 33 | 5.5 |
9 | Fujian University of Traditional Chinese Medicine | 5 | 36 | 7.2 |
10 | Dalian University of Technology | 5 | 61 | 12.2 |
TCM, traditional Chinese medicine.
Figure 7 illustrates the cooperation network analysis of institutions that published at least two articles. The size of the circle indicates the degree of institutional cooperation, the width of the line between the connecting mechanism indicates the cooperation strength, and the color indicates the cluster network of subgroups. Eight key cooperation clusters were identified. In addition, the organizations with the most citations included the Shanghai University of Traditional Chinese Medicine, Xiamen University, and the University of Science and Technology of China. Furthermore, the node in light purple indicated that the Shanghai University of Traditional Chinese Medicine had a higher number of citations than any other organization, suggesting that it might be a very important institution for the study of AI in the four TCM diagnoses (Figure 8).
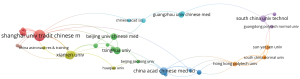
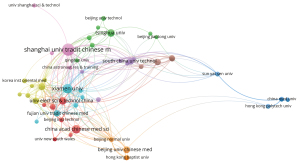
Funding often plays a role in reflecting the attention that certain areas of research have received and represents the development of available scientific resources in that field of research. Figure 9 summarizes the top 10 funding agencies by publication. We found that all of the funding agencies were from China. The National Natural Science Foundation of China is the most funded agency (n=40). From a regional perspective, Guangzhou (n=10) and Shanghai (n=6) were the most funded regions in China (n=10).
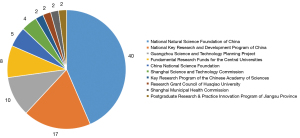
Analysis of the keywords
A total of 43 keywords with a frequency of at least three times were retrieved from among 503 keywords. Six clusters were generated based on their association using VOSviewer software. The top keywords in each cluster are listed in Table 5 and the visualization map of the keywords is shown in Figure 10. Cluster 1 consisted mainly of “traditional Chinese medicine”, “machine learning”, “diagnosis”, “syndrome differentiation”, and “natural language processing”. This cluster mainly expressed the research progress of AI in TCM diagnosis and symptom classification (red). Cluster 2 focused on “deep learning”, “tongue segmentation”, and “feature extraction”; it mainly introduced the application of deep learning in tongue image processing (green). Cluster 3 focused on “algorithm”, “diseases”, “smart Chinese medicine”, and “model”. This cluster mainly constructed models of TCM-related diseases (blue). Cluster 4 focused on “neural network” and “tongue image classification”; studies in this cluster focused on tongue image classification based on the neural network (yellow). Cluster 5 focused on “deep transfer learning”, “representation learning”, and “tongue image”; it primarily researched the application of different AI methods in TCM diagnosis (purple). Cluster 6 focused on “tooth-marked tongue”, “image-analysis”, and “tongue diagnosis”. This cluster primarily researched the application of different AI methods in TCM diagnosis (purple), as well as the association between the tooth-marked tongue and TCM diagnosis (sky blue).
Table 5
Clustering number | Color | Number of articles | Hotspot trend |
---|---|---|---|
Cluster 1 | Red | Artificial intelligence, classification, data mining, diagnosis, feature selection, machine learning, medicine, natural language processing, prediction, syndrome differentiation, system, traditional Chinese medicine | AI in TCM diagnosis and TCM symptom classification |
Cluster 2 | Green | Deep learning, feature extraction, image, segmentation, task analysis, tongue segmentation | Application of deep learning in tongue image processing |
Cluster 3 | Blue | Algorithm, diseases, hypertension, model, smart Chinese medicine | Models of TCM-related diseases |
Cluster 4 | Yellow | Color, convolutional neural network, image classification, tongue image classification, tongue images | Neural networks are classified with tongue images |
Cluster 5 | Purple | Covid-19, deep transfer learning, representation learning, tongue image | Deep transfer learning and tongue diagnosis |
Cluster 6 | Sky blue | Image-analysis, tongue diagnosis, tooth-marked tongue | Tooth-marked tongue |
TCM, traditional Chinese medicine; AI, artificial intelligence.
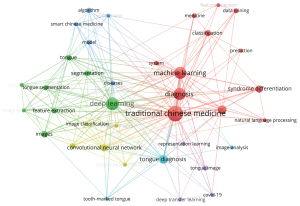
The keyword timeline graph was developed to depict the relationships and occurrences of keywords related to the search topics. An overlay visualization timeline graph of the keywords was generated using Citespace software to explore the occurrence in AI for the four TCM diagnoses over a period of time (Figure 11). All keywords were marked according to the sequence of keyword appearance. Notably, in 2007, “tongue diagnosis”, “deep learning”, and “machine learning” appeared in the visualization map. Compared with the AI-related keywords, such as “representation learning”, “extreme machine learning”, etc., TCM keywords including “pulse signal”, etc. appear later, indicating that the research on AI for the four TCM diagnoses has increased in recent years. We believe that the study of AI-based TCM diagnosis has research value and will become a hotspot in TCM research in the future.
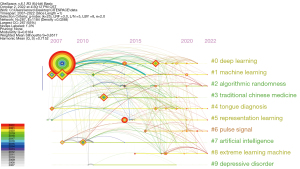
The top 10 keywords with the strongest citation bursts were listed in Figure 12. Among these, “machine learning”, the earliest keyword burst, was detected in 2007. Subsequently, research related to AI, such as “information fusion”, became hot topics. A hot topic related to TCM diagnosis, “syndrome differentiation”, first appeared in 2008, followed by “tongue shape classification” in 2012. The most recent keywords burst (“neural network” and “image segmentation”) occurred in 2019, suggesting that AI-related neural networks are attracting increased attention in the study of tongue images and might become new research hotspots in the next few years.
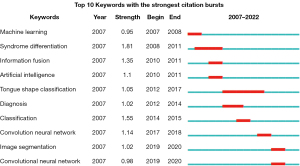
Discussion
The four TCM diagnostic methods mainly include looking, smelling, asking, and touching. In this study, we used bibliometrics to analyze the literature related to AI for these four TCM diagnoses. The results showed that relevant literature only began to appear in 2007. Over the past 15 years, the literature on AI for the four TCM diagnoses increased every year (Figure 1).
The number of papers published is an important indicator of the level of relevant scientific research of countries or institutions. Country analysis showed that China published the highest number of AI papers on the four TCM diagnoses (Table 1 and Figure 2), indicating that China is highly influential in this field. The number of citations is often used to measure impact (16); the average citation per paper in China was unsatisfactory, with a lower number of average citations than in Japan, the USA, and Canada. China also lacked highly cited articles, which may lead to insufficient international influence in the future. As for countries/regions cooperation, China was the center of research. However, most research were limited to Europe and a few Asian countries, such as Japan, South Korea. Therefore, cross-country and regional cooperation was essential in the future, especially with South America and Africa. This phenomenon may be influenced by culture, but also closely related to the level of the economy. It was therefore necessary to strengthen national and regional cooperation, particularly with those developing countries.
Determining the importance of a journal provides researchers with reliable reference information and identifies target journals for their literature searches and submissions (17). According to the results, among the 81 journals/conferences involved in this study, most journals are biological, engineering, and computer journals, as shown in Table 2. Our results showed that the Evidence-Based Complementary and Alternative Medicine and IEEE Access journals published the highest number of papers, indicating that most articles related to AI for the four TCM diagnoses would be considered for publication in this journal. In terms of conference documents, the 2020 IEEE International Conference on Bioinformatics and Biomedicine published the most papers, illustrating that this field was a particular focus in the engineering field.
In the author/co-authorship analysis, Xiaojuan Hu and Jiatuo Xu have the most published literature among the 652 authors who published papers related to AI for the four TCM diagnostic methods (Table 3). Furthermore, Xiaojuan Hu, Jiatuo Xu, Liping Tu, and Ji Cui maintained a very close relationship and published a large amount of literature on group cooperation (Figure 5). It is worth noting that although Jinhong Guo and Weihong Li published fewer than five papers, their average number of citations exceeded 20, highlighting the importance of their articles in this field. The visualization map of co-citation showed that no representative authors or experts have yet emerged in this research area. Therefore, we believe that these top teams will publish representative papers in this field by strengthening cooperation with each other.
The top 10 most productive institutions were all from China, of which five belong to TCM institutions, two were comprehensive institutions, and three were science and technology institutions (Table 4). In addition, the Shanghai University of Traditional Chinese Medicine published the most AI-related papers on the four TCM diagnoses [the red node represents the Shanghai University of Traditional Chinese Medicine, and the purple node denotes institutions that were cited more often (Figure 7)]. From the distance of the points, we observed that research institutes in this field are scattered. Although the top 10 institutions in terms of production were all in China, they did not have close cooperation with each other, and it is more noteworthy that they had little cooperation with foreign institutions. Therefore, China’s academic institutions should strengthen cooperation to achieve the purpose of improving academic influence. Through the analysis of funding, it was concluded that the National Natural Science Foundation of China sponsors the most research in this field (Figure 9). The other regions with more sponsorship were first-tier cities and regions in China, which showed that research in this field has not attracted attention in other regions of China.
Keywords can provide up-to-date information about the themes in a particular study. In this analysis, we highlighted the network of keywords connected by co-occurrence (18), and six research clusters were generated based on their association. As shown in Figure 10, in the center of the network map were high-frequency keywords, mainly including “traditional Chinese medicine”, “deep learning”, and “tongue diagnosis”, etc. The keywords of these centers reflect that deep learning research related to tongue diagnosis in AI for the four TCM diagnoses was an interesting research hotspot.
In this paper, the timeline map on how keywords change over time was also analyzed. At the top of the figure, we observed that research in this area first appeared in 2007, five different machine learning techniques were used to classify the tongue texture and tongue coating (19), and the SVM machine learning method had the best performance. The nodes on the left abscissa represent the migration behavior of the research hotspots, and the lines between them denote citing. During the middle stage, articles on AI for the four TCM diagnoses mainly focused on the updating and evolution of methodology. For example, they proposed a supervised machine learning technique based on the shape of a tongue image to recognize diseases (20), explored new methods for the clinical characterization of ZHENG using various machine learning algorithms, ZHENG is equivalent to syndrome, which is the basic unit and foundation of TCM treatment, and there were also some studies on tongue color and facial features, etc. (21,22). In the most recent stage. In addition to the previous research on the tongue, there were also more studies on the pulse and TCM symptoms (8,23-28).
Burst detection is an algorithm developed to determine the sharp increase in citation or keyword popularity within a limited period of time, and can be used as an effective method of identifying hot spots (12). Our research showed that the first detected keyword was “machine learning” in 2007. Since 2008, the keyword “syndrome differentiation” related to TCM began to appear. From 2010 to 2020, keywords related to machine learning, such as “information fusion”, “classification”, etc., began to appear. It is worth noting that the keyword “tongue shape classification” lasted for 6 years from 2012 to 2017.
TCM adheres to a “people-oriented” principle and emphasizes prevention. While inheriting and further developing its characteristics, TCM comprehensively combines modern technology and technology to maximize its advantages, so as to promote the objectification, standardization, and accuracy of TCM according to the “holistic concept” and “treatment based on syndrome differentiation”. The four TCM diagnostic methods are the primary means of disease differentiation and treatment in TCM. In recent years, with the rapid development of AI technology, the four diagnoses of TCM have also achieved fruitful results. At present, the problems of intelligent research on the four diagnostic methods of TCM include the lack of accurately labeled datasets, the limited generalizability of feature extraction, and the lack of intelligent research on the fusion of the four diagnostic approaches (29).
Currently, research on monitoring intelligence is relatively mature and ideal. The intelligent process can be divided into several steps, including image acquisition, image preprocessing, image segmentation, feature extraction/selection and classification, and recognition. Research on AI in TCM is mainly concentrated in the fields of eye, tongue, and face diagnoses. To solve the problem of prescription sample imbalance, Xi et al. (30) proposed algorithm that combines machine learning to obtain more accurate and stable results. Based on computer vision and AI technology, body mass identification and disease diagnosis are carried out by respectively observing the images of the eyes, tongue, and face. Numerous scholars have also proposed various intelligent solutions based on fundus image segmentation and disease diagnosis and prediction (31).
In addition, many scholars have focused on the algorithm research of tongue and face segmentation and classification. There are intelligent face diagnosis machines that can classify signs by objectification and intelligent complexion, facial gloss, and lip color. Also, intelligent tongue instruments can diagnose TCM syndromes according to the characteristics of tongue color, shape, and coating, and intelligent eye diagnostic instruments for analysis and health assessment according to the characteristics of scleral spots and blood vessels (32,33). Olfactory diagnosis is divided into two categories: acoustic diagnosis and olfactory diagnosis. It is usually studied through the collection, preprocessing, feature extraction, and pathological diagnosis of sound or odor signals, and relies on the development of intelligent auscultation and olfactory diagnostic instruments. At present, the intelligent research of olfaction is still mainly in the academic theory exploration stage. Existing smart sniffing technology can perform gas sampling, oral exhalation analysis, gas composition analysis, and disease diagnosis. Scholars have mainly utilized odor identification intelligent technology for the diagnosis of asthma, cancer, tuberculosis, and other diseases (34).
The AI consultation system can provide efficient and convenient TCM consultation services, which usually involve two aspects: input/output equipment and an intelligent diagnostic model, and can be presented on a smartphone, computer web page, etc. Here, technical implementation is mainly divided into two steps: quantification of the patient’s symptoms and intelligent diagnosis. Human-computer interactive voice question answering refers to the user describing their body condition through speech, obtaining semantic information through natural language processing, and then performing intelligent analysis and disease diagnosis. The intellectualization of TCM emphasizes the intellectualization of research under the guidance of TCM theory and uses AI technology to achieve clinical diagnostic evaluation and daily health care with TCM. In recent years, emerging deep learning algorithms can process unstructured data such as images, audio signals, texts, etc., in a method that is consistent with the principle of the four TCM diagnoses. In the field of TCM research, the four diagnostic parameters based on AI can be regarded as a multi-modal fusion method. At present, there are some common problems in TCM diagnostic methods, such as the use of collection instruments are not uniform, the format and size of the pictures are different, so the collection process and collection instruments of TCM tongue image and pulse information need to be stipulated by relevant national or industry standards, laying the foundation for the processing of big data of the four diagnoses of TCM in the future.
AI-assisted pulse diagnosis can be used to distinguish pregnancy or diagnose various diseases, including gastritis, cholecystitis, pancreatitis, duodenum, bulbar ulcer, acute appendicitis, hypertension, coronary heart disease, diabetes mellitus, acute appendicitis, arteriosclerosis, and cirrhosis, and can also be employed to quantitatively assess the risk of certain diseases (8,35). However, the reliability of AI-assisted pulse diagnosis needs to be improved, and thus, it is more suitable for assisting doctors in diagnosis rather than independently identifying the syndromes or diseases of patients (36). AI-assisted consultation is mainly based on data analytical methods, including SVM, neural networks, graph models, multi-label learning, and so on. The multi-label learning method can be used to mine data and construct an intelligent consultation model, and its classification accuracy of coronary heart disease is up to 78% (37). Some scholars have utilized the complex system and probability graph model to model and divide the consultation data and determine its average value for chronic gastritis, with an accuracy of up to 82.5% (38). On the other hand, there are problems associated with the application of AI technology in the field of TCM diagnosis. Firstly, the superficial form of TCM AI cannot enable technology empowerment, nor does it conform to the TCM theory, which makes it difficult to be used in clinical practice. Thus, it is necessary to adjust the direction of technology integration and TCM to promote deep integration. Secondly, the TCM diagnostic process simulated by AI is overly complex and comprehensive, which requires further theoretical decomposition and interpretation before it can be recognized and measured by modern technology. A unified glossary is needed to improve the objectification and standardization of TCM diagnosis.
Conclusions
Through the analysis of keywords and timeline maps, the diagnosis and classification of related diseases based on deep learning algorithm and neural network of tongue images will still be a hotspot in future research, but in addition to these studies, AI research on clinical ZHENG and symptoms of TCM will rise in the future and become research trends. The advantages of introducing AI technology into TCM diagnosis include its objectified, quantified, and precise data output and diagnostic results, as well as the fact that it can use scientific and standard “universal” language to diagnose and predict diseases and spread TCM. Finally, we firmly believe that through further technical decomposition and elucidating the needs of TCM AI-assisted diagnosis, the cross-integration of TCM and AI technology can be promoted.
Acknowledgments
Funding: This project was supported by the National Key Research and Development Program of China (No. 2017YFC1703305).
Footnote
Conflicts of Interest: All authors have completed the ICMJE uniform disclosure form (available at https://atm.amegroups.com/article/view/10.21037/atm-22-6431/coif). The authors have no conflicts of interest to declare.
Ethical Statement: The authors are accountable for all aspects of the work in ensuring that questions related to the accuracy or integrity of any part of the work are appropriately investigated and resolved.
Open Access Statement: This is an Open Access article distributed in accordance with the Creative Commons Attribution-NonCommercial-NoDerivs 4.0 International License (CC BY-NC-ND 4.0), which permits the non-commercial replication and distribution of the article with the strict proviso that no changes or edits are made and the original work is properly cited (including links to both the formal publication through the relevant DOI and the license). See: https://creativecommons.org/licenses/by-nc-nd/4.0/.
References
- Velik R. An objective review of the technological developments for radial pulse diagnosis in Traditional Chinese Medicine. European Journal of Integrative Medicine 2015;7:321-31. [Crossref]
- Shi Y, Yao X, Xu J, et al. A New Approach of Fatigue Classification Based on Data of Tongue and Pulse With Machine Learning. Front Physiol 2022;12:708742. [Crossref] [PubMed]
- McCarthy J, Minsky ML, Rochester N, et al. A proposal for the dartmouth summer research project on artificial intelligence, august 31, 1955. AI magazine 2006;27:12-4.
- Li J, Huang J, Jiang T, et al. A multi-step approach for tongue image classification in patients with diabetes. Comput Biol Med 2022;149:105935. [Crossref] [PubMed]
- Li J, Yuan P, Hu X, et al. A tongue features fusion approach to predicting prediabetes and diabetes with machine learning. J Biomed Inform 2021;115:103693. [Crossref] [PubMed]
- Xiang Y, Shujin L, Hongfang C, et al. Artificial Intelligence-Based Diagnosis of Diabetes Mellitus: Combining Fundus Photography with Traditional Chinese Medicine Diagnostic Methodology. Biomed Res Int 2021;2021:5556057. [Crossref] [PubMed]
- Wang Z, Sun S, Poon J, et al. editors. Cnn based multi-instance multi-task learning for syndrome differentiation of diabetic patients. 2018 IEEE International Conference on Bioinformatics and Biomedicine (BIBM). 2018: IEEE.
- Luo ZY, Cui J, Hu XJ, et al. A Study of Machine-Learning Classifiers for Hypertension Based on Radial Pulse Wave. Biomed Res Int 2018;2018:2964816. [Crossref] [PubMed]
- Yan J, Cai X, Chen S, et al. Ensemble Learning-Based Pulse Signal Recognition: Classification Model Development Study. JMIR Med Inform 2021;9:e28039. [Crossref] [PubMed]
- Chen H-C, Damarjati C, Putra KT, et al. Pulse-line intersection method with unboxed artificial intelligence for hesitant pulse wave classification. 2022;59:102855.
- Theodore DD, Shree S, Reddy AA, et al. Synergy: Information technology and health sciences. 2016;4:58.
- Shen Z, Hu J, Wu H, et al. Global research trends and foci of artificial intelligence-based tumor pathology: a scientometric study. J Transl Med 2022;20:409. [Crossref] [PubMed]
- Shen Z, Wu H, Chen Z, et al. The Global Research of Artificial Intelligence on Prostate Cancer: A 22-Year Bibliometric Analysis. Front Oncol 2022;12:843735. [Crossref] [PubMed]
- Van Eck NJ, Waltman L. VOSviewer manual. Manual for VOSviewer version 2011;1.
- Eck NJV, Waltman L. Visualizing bibliometric networks. Measuring scholarly impact. Springer; 2014:285-320.
- Kulkarni AV, Aziz B, Shams I, et al. Comparisons of citations in Web of Science, Scopus, and Google Scholar for articles published in general medical journals. JAMA 2009;302:1092-6. [Crossref] [PubMed]
- Zhuang Y, Jiang N, Wu Z, et al. Efficient and robust large medical image retrieval in mobile cloud computing environment. Information Sciences 2014;263:60-86. [Crossref]
- Tong Y, Cheng N, Jiang X, et al. The Trends and Hotspots in Premature Ovarian Insufficiency Therapy from 2000 to 2022. Int J Environ Res Public Health 2022;19:11728. [Crossref] [PubMed]
- Hui SC, He Y, Thach DTC. editors. Machine learning for tongue diagnosis. 2007 6th International Conference on Information, Communications & Signal Processing; 2007: IEEE.
- Devi GU, Anita EAM. editors. An analysis of tongue shape to identify diseases by using supervised learning techniques. 2017 International Conference on Information Communication and Embedded Systems (ICICES); 2017: IEEE.
- Sun C, Zhang H, Qiu X, et al. editors. Automatic facial spirit classification for traditional Chinese medicine based on multiple facial features. 2014 IEEE International Conference on Bioinformatics and Biomedicine (BIBM); 2014: IEEE.
- Hou J, Su HY, Yan B, et al. editors. Classification of tongue color based on CNN. 2017 IEEE 2nd international conference on big data analysis (ICBDA); 2017: IEEE.
- Shi YL, Liu JY, Hu XJ, et al. A New Method for Syndrome Classification of Non-Small-Cell Lung Cancer Based on Data of Tongue and Pulse with Machine Learning. Biomed Res Int 2021;2021:1337558. [Crossref] [PubMed]
- Wang Y, Wu Q, Zhu J, et al. editors. A TCM syndrome differentiation algorithm based on deep belief network. 2020 35th Youth Academic Annual Conference of Chinese Association of Automation (YAC); 2020: IEEE.
- Huang Z, Miao J, Chen J, et al. A Traditional Chinese Medicine Syndrome Classification Model Based on Cross-Feature Generation by Convolution Neural Network: Model Development and Validation. JMIR Med Inform 2022;10:e29290. [Crossref] [PubMed]
- Liu Z, Bai C, Yu H, et al. An adaptive deep learning model to differentiate syndromes of infectious fever in smart medicine. Future Generation Computer Systems 2020;111:853-8. [Crossref]
- Xia SJ, Gao BZ, Wang SH, et al. Modeling of diagnosis for metabolic syndrome by integrating symptoms into physiochemical indexes. Biomed Pharmacother 2021;137:111367. [Crossref] [PubMed]
- Kumar S, Veer K, Kumar S. A spider tool-based qualitative analysis of machine learning for wrist pulse analysis. Network Modeling Analysis in Health Informatics and Bioinformatics 2022;11:1-17. [Crossref]
- Li H, Li C, Lang X, et al. The Current Status and Key Techniques of Intelligentization of the Four Examinations in Traditional Chinese Medicine. Journal of Traditional Chinese Medicine 2022;63:1101-8.
- Xi C, Zhang J, Xiao Y, et al. Research on Application Paradigm of Random Sampling Method in Data Analysis of Traditional Chinese Medicine—Taking Insomnia Prescriptions of Famous Old Tcm Physicians as an Example. May 2022. Available online: https://ssrn.com/abstract=4120086
10.2139/ssrn.4120086 10.2139/ssrn.4120086 - Naveed K, Abdullah F, Madni HA, et al. Towards Automated Eye Diagnosis: An Improved Retinal Vessel Segmentation Framework Using Ensemble Block Matching 3D Filter. Diagnostics (Basel) 2021;11:114. [Crossref] [PubMed]
- Ma J, Wen G, Wang C, et al. Complexity perception classification method for tongue constitution recognition. Artif Intell Med 2019;96:123-33. [Crossref] [PubMed]
- Huan EY, Wen GH. Transfer learning with deep convolutional neural network for constitution classification with face image. Multimedia Tools Applications 2020;79:11905-19. [Crossref]
- Karakaya D, Ulucan O, Turkan M. Electronic nose and its applications: A survey. I Int J Autom Comput 2020;17:179-209. [Crossref]
- Hu X, Zhu H, Xu J, et al. editors. Wrist pulse signals analysis based on deep convolutional neural networks. 2014 IEEE conference on computational intelligence in bioinformatics and computational biology; 2014: IEEE.
- Zhang SR, Sun QF, editors. Human pulse recognition based on convolutional neural networks. 2016 International Symposium on Computer, Consumer and Control (IS3C); 2016: IEEE.
- Chu X, Sun B, Huang Q, et al. Quantitative knowledge presentation models of traditional Chinese medicine (TCM): A review. Artif Intell Med 2020;103:101810. [Crossref] [PubMed]
- Liu GP, Li GZ, Wang YL, et al. Modelling of inquiry diagnosis for coronary heart disease in Traditional Chinese Medicine by using multi-label learning. BMC Complement Altern Med 2010;10:37. [Crossref] [PubMed]
(English Language Editor: A. Kassem)