Ferroptosis-related lncRNA model based on CFAP58-DT for predicting prognosis and immunocytes infiltration in endometrial cancer
Highlight box
Key findings
• A ferroptosis-related lncRNA model based on CFAP58-DT may help predict the prognosis and immunocyte infiltration in endometrial cancer.
What is known and what is new?
• Ferroptosis refers to iron-dependent programmed cell death and is involved in a series of cancer processes. LncRNA plays a crucial role in various tumors. The ability of ferroptosis-related lncRNAs to predict prognosis and guide treatment has not been exhaustively investigated.
• A prognostic ferroptosis-related lncRNA model based on CFAP58-DT for predicting the prognosis and immune-infiltrating conditions in EC. The potential oncogenic role of CFAP58-DT can further guide immunotherapy and chemotherapy.
What is the implication, and what should change now?
• The ferroptosis-related lncRNA model based on CFAP58-DT in endometrial cancer can be used to predict the prognosis and immune-infiltrating conditions. The potential oncogenic role of CFAP58-DT can further guide immunotherapy and chemotherapy.
Introduction
In 2021, there were 66,570 estimated new cases and 12,940 estimated new deaths recorded in the United States, making endometrial cancer (EC) one of the most common gynecological tumors (1). The incidence of EC has been increasing in recent years and is affecting younger patients (2,3). Geriatric patients, late-stage, or poorly differentiated patients have a worse prognosis. However, approximately 20% of patients exhibit low-risk pathological results (4). Currently, there are limitations in evaluating the risk of recurrence and metastasis and formulating treatment plans solely based on pathology, resulting in excessive or insufficient treatment. Therefore, further study on the markers related to the prognosis of EC is important for women worldwide.
Ferroptosis refers to iron-dependent programmed cell death, with morphological, biochemical, and genetic features (5-7), which leads to a decrease in mitochondria and cristae and an increase in membrane density. The nucleus exhibits no obvious morphological changes. Ferroptosis is characterized by increased lipid peroxidation and reactive oxidative species (ROS) in the cell. Some characteristic genes, including glutathione peroxidase 4 (GPX4), glutathione synthetase (GSS), and acyl-CoA synthetase long chain family member 4 (ACSL4), are also altered. Ferroptosis is involved in a series of cancer processes, including diagnosis, prognosis, carcinogenesis, cancer resistance, and treatment of hepatocellular carcinoma (8-10), renal cell carcinoma (11-13), glioma (14-16), ovarian cancer (17-19), and so on. Furthermore, drugs such as quercetin, simvastatin and amentoflavone, as well as genes including CircRAPGEF5, protein tyrosine phosphatase non-receptor type 18 (PTPN18) and tyrosine kinase (ELK1) Oncogene participated in regulation of ferroptosis and thus played a role in EC (20-26). What’s more, ferroptosis plays an important role in anti-tumor immunity. TLR2 on macrophages eliminates ferroptosis cells by recognizing phosphatidylethanolamine on ferroptosis cells, neutrophils maintain the inflammatory reaction after iron death in relevant tissues, and CD8+ T cells kill cancer cells by stimulating ferroptosis (27).
Long non-coding RNA (lncRNA) are non-coding RNAs that are over 200 nucleotides in length; they do not encode proteins but regulate gene expression (28,29). Compelling evidence has been presented that lncRNAs play a crucial role in various tumors (30,31). For instance, the lncRNA OIP5-AS1 exerts an inhibitory effect on the progression of EC via modulation of the PTEN/PI3K pathway (32). Additionally, certain lncRNAs may even serve as predictive factors that are strongly correlated with the prognosis of EC patients (33). Notably, lncRNAs have been established to play a pivotal role in the tumor microenvironment (TME) (34). For instance, the upregulation of the lncRNA MALAT1 contributes to survival, proliferation, and migration in the ovarian TME via cell apoptosis (35). The lncRNA KRT19P3 prevents breast cancer progression via immune pathways, thereby reducing programmed cell death 1 ligand 1 (PD-L1) expression and enhancing cluster of differentiation (CD) 8+ T cell expression (36). Moreover, as a crucial feature of the TME, hypoxia activates the lncRNA HABON and prolongs the survival of liver cancer tissues through the inhibition of necroptosis (37). Besides, there was a report saying that the signature composed of 11 ferroptosis-related lncRNAs accurately predicted the prognosis of ovarian cancer patients (38). However, the ability of ferroptosis-related lncRNAs to predict prognosis and guide treatment has not been investigated exhaustively.
Therefore, we utilized the machine learning method to analyze The Cancer Genome Atlas (TCGA) data and constructed a model of ferroptosis-related lncRNA (FerlncRNA) to predict the survival time of EC patients and determine the underlying connection with the TME. CFAP58-DT is an RNA Gene, and is affiliated with the lncRNA class. And there were few studies in roles of CFAP58-DT in cancers. We also employed in vitro cell experiments to verify the effects of CFAP58-DT, the model’s key lncRNA, on cell viability and migration. We present the following article in accordance with the TRIPOD and MDAR reporting checklists (available at https://atm.amegroups.com/article/view/10.21037/atm-22-6659/rc).
Methods
The entire data analysis process is depicted in Figure 1.
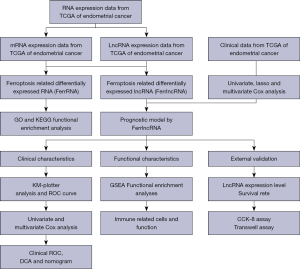
Data acquisition and tissue collection
Patients who were pathologically diagnosed with uterine corpus endometrial carcinoma (UCEC) up to October 24th, 2022 were enrolled and their messenger RNA (mRNA) expression and clinical data were obtained from TCGA database (https://portal.gdc.cancer.gov/repository). After removing cases with incomplete clinical information, 526 UCEC patients and 23 patients with normal endometrial tissues remained. Next, we downloaded the GTF files from Ensembl (http://asia.ensembl.org) to distinguish mRNAs and lncRNAs. A total of 382 ferroptosis-related genes were received from the FerrDb database (http://www.zhounan.org/ferrdb/) for the co-expression analysis of the ferroptosis-related lncRNAs (gene correlation coefficients >0.4 and P<0.001). The study was conducted in accordance with the Declaration of Helsinki (as revised in 2013).
Differentially expressed gene (DEG) analysis
The “limma” R package in R software (R 4.2.0, USA) was employed to search for differentially expressed ferroptosis-related genes and lncRNAs (DEFRGs and DEFerlncRNAs) between the tumor and normal tissues. The Wilcoxon signed-rank test was performed to screen the DEFRGs and DEFerlncRNAs [false discovery rate (FDR) <0.05].
Gene Ontology (GO) and Kyoto Encyclopedia of Genes and Genomes (KEGG) functional enrichment
The “clusterProfiler” R package was employed to perform GO and KEGG pathway analyses of the DEFRGs. The “ggplot2” R package was applied to visualize the the top enriched pathways (P<0.05).
Construction and confirmation of the prognostic model of DEFerlncRNA
Univariate Cox analysis was conducted to initially screen DEFerlncRNA related to survival after Benjamini & Hochberg (BH) correction (P<0.001). Least absolute shrinkage and selection operator (LASSO) analysis via the “glmnet” R package was applied to minimize the deviance with a 10-round cross-validation. Then, multivariate Cox analysis was performed to correct and obtain the corresponding coefficient of each gene. We then calculated the risk score (score = sum of products of expression level of each gene and corresponding coefficient) according to the references (39,40). and patients were divided into high- and low-risk groups according to the median risk score value. The “Rtsne” R package was used to calculate the expression spectrum and group the patients. Moreover, principal components analysis (PCA) was conducted using the “stats” package. Kaplan-Meier (KM) analysis was performed using the “survive” and “survminer” packages to illustrate the prognostic differences between the two groups. The “survivalROC” package was utilized to draw the receiver operating characteristic (ROC) curves at multiple time points.
Clinical evaluation of the prognostic model of DEFerlncRNA
The relationship between the risk score and clinical indicators was analyzed by univariate and multivariate Cox regression analyses to evaluate the model’s diagnostic performance. The “limma” and “ggupbr” packages were applied to draw forest maps. Using the “ComplexHeatmap” R software package, the Chi-square test was used to analyze the relationship between the model and clinical indicators. Patients aged ≤60 years old were grouped, and the remaining patients were placed in the other group. Moreover, patients with stages I, II, III, and IV were in four groups, while patients with grades 1, 2, and 3 were in three groups. The Wilcoxon signed-rank test was utilized to compare the group risk score differences of these clinical indicators. The ROC curves were applied to assess the sensitivity and specificity of the model. Decision curve analysis (DCA) was performed to integrate the preferences of patients or decision-makers into the analysis to meet the needs of clinical decision-making (41). A nomogram was constructed based on the model to predict the overall survival (OS) of UCEC patients at 1, 3, and 5 years.
GSEA of the prognostic model of DEFerlncRNA
Gene Set Enrichment Analysis (GSEA) software (GSEA_4.0.3, USA) was applied for the c2.cp.kegg.v.7.2.symbols.gmt gene sets to comprehend the enriched pathway differences with FDR <0.25 and nominal (NOM) P<0.05 after 1,000 permutations in the high-risk versus low-risk groups.
Evaluation of the immune-infiltrating condition of the prognostic model of DEFerlncRNA
Immune-related databases were used to analyze the immune-cell characteristics in patients with different risk scores by Spearman correlation analysis (P<0.05). The “gsva” R package compared the normalized gene expression data with the gene sets possessing common biological functions, chromosomal localization, and physiological regulation in single sample Gene Set Enrichment Analysis (ssGSEA) (42). The differences in 16 types of immune cells and 13 immune-related functions between the high- and low-risk groups were determined.
Cell culture and siRNA transfection
HEC-1A and Ishikawa cell lines were purchased from Procell (Wuhan, China). The cells were cultured in McCoy’s 5A and Roswell Park Memorial Institute (RPMI) 1640 medium (VivaCell Biosciences Ltd., Shanghai, China) supplemented with 10% Fetal Bovine Serum (FBS) (Procell, Wuhan, China) in a 37 ℃, 5% carbon dioxide (CO2) incubator. Small-interfering-RNA(SiRNA) targeted CFAP58-DT were ordered from GenePharma (Suzhou, China), and the following sequences were used: si-CFAP58-DT#1 (sense: GGUUGAUGAAUAAAUGCAATT; anti-sense: UUGCAUUUAUUCAUCAACCTT), si-CFAP58-DT#2 (sense: GGUGCAGAGCUUAAGGCAUTT; anti-sense: AUGCCUUAAGCUCUGCACCTT), negative control (NC) (sense: UUCUCCGAACGUGUCACGUTT; anti-sense: ACGUGACACGUUCGGAGAATT). Green fluorescent protein (GFP)-transfect-mate (GenePharma, Suzhou, China) was used for transfection.
RNA extraction and quantitative real-time PCR (qRT-PCR)
Total cell RNA was extracted by the Trizol (Takara Bio, Kusatsu, Japan) method followed by reverse transcription using the PrimeScript™ RT reagent Kit with a genomic DNA (gDNA) Eraser (Perfect Real Time; Takara Bio, Shiga, Japan). qRT-PCR was performed using the ABI 7500 Fast system with the ChamQ Universal SYBR qPCR Master Mix (Vazyme Biotech Co., Ltd., China). The primer sequences used were as follows: CFAP58-DT (forward: GGGCATCTACCACACTTCACTTCC; reverse: GGACCACGGACAAAGACGATCATG), glyceraldehyde-3-phosphate dehydrogenase (GAPDH) (forward: CAGGAGGCATTGCTGATGAT; reverse: GAAGGCTGGGGCTCATTT). LncRNA expression was calculated using the 2−∆∆Ct method relative to GAPDH.
Transwell assay
Eight-µm-pore Transwell chambers coated with or without Matrigel (BD, San Diego, USA) (Corning, NY, USA) were used for cell invasion and migration detection. 600 µL of complete medium was added into the lower chamber of the 24-well plate, and the chambers were then placed in the plate, 200 µL (1.0×104 cells/200 µL for migration and 3.0×104 cells for invasion) of cell suspension were taken into the upper chamber and finally placed into the incubator for 24 h. After 24 h, 4% paraformaldehyde was applied for 30 minutes for fixing and 0.1% crystal violet was used for 20 min for staining. Cells were observed in five visual fields under a 200× microscope (Nikon, USA) and counted.
Cell viability assay
The cell suspension [100 µL (3,000 cells)/hole] was inoculated and the culture plate was placed in the incubator for pre-culture (37 ℃, 5% CO2). Ten µL of cell counting kit (CCK) solution (Procell, Wuhan, China) was then added to each hole. The culture plate was incubated in the incubator for 2 h and the absorbance was measured at 450 nm using a microplate reader (TECAN, Switzerland).
Statistical analysis
Statistical analysis was conducted using the R package and GraphPad Prism 9 software (La Jolla, CA, USA). All experiments were repeated three times. Variance homogeneous and normal distributed continuous variables were analyzed using the unpaired student’s t-test, otherwise, the Mann-Whitney U-test or Kruskal-Wallis H-test was used. DEGs were screened by a Wilcoxon signed-rank test (false discovery rate, FDR <0.05), and BH corrections were used to adjust p values. The relationship between lncRNA and clinicopathological characteristics were evaluated using logistic regression analyses and a heatmap graph. P<0.05 was considered statistically significant.
Results
Patients included in TCGA database
Patients who were pathologically diagnosed with UCEC up to October 24th, 2022 were enrolled in this study, and their mRNA expression and clinical data were obtained from TCGA database (https://portal.gdc.cancer.Gov/repository). After removing cases with incomplete clinical information, 526 UCEC patients and 23 patients with normal endometrial tissues remained. The UCEC patients’ details are shown in https://cdn.amegroups.cn/static/public/atm-22-6659-1.xlsx.
Differentially expressed FRGs and FerlncRNA analysis
The expression data were obtained from TCGA database and annotated with Ensembl GTF files. Correlation analysis of the ferroptosis-related gene and lncRNA was performed, yielding 1,731 FerlncRNAs (available online: https://cdn.amegroups.cn/static/public/atm-22-6659-1.xlsx). A total of 1,404 DEFerlncRNAs (available online: https://cdn.amegroups.cn/static/public/atm-22-6659-1.xlsx) were identified, among which 608 were down-regulated and 796 were up-regulated. Also, 209 of 382 ferroptosis-related genes were found to be differently expressed in tumor and normal tissues (available online: https://cdn.amegroups.cn/static/public/atm-22-6659-1.xlsx).
GO and KEGG functional enrichment of DEFRGs
According to the GO analysis, DEFRGs were enriched in response to oxidative stress, chemical stress, starvation, external stimulus, autophagy, autophagosomes, and antioxidant activity (Figure 2A, available online: https://cdn.amegroups.cn/static/public/atm-22-6659-1.xlsx). Apoptosis, autophagy, ferroptosis, cellular senescence, forkhead box O (FoxO) signaling, central carbon metabolism in cancer, ROSs, hypoxia inducible factor 1 (HIF-1) signaling, PD-L1 expression, and programmed cell death protein 1 (PD-1) checkpoint, nucleotide-binding oligomerization domain (NOD)-like receptor signaling, and the mechanistic target of rapamycin kinase (mTOR) and epidermal growth factor receptor tyrosine kinase domain (ErbB) signaling pathways were among the many common pathways involved in carcinogenesis (Figure 2B, available online: https://cdn.amegroups.cn/static/public/atm-22-6659-1.xlsx).
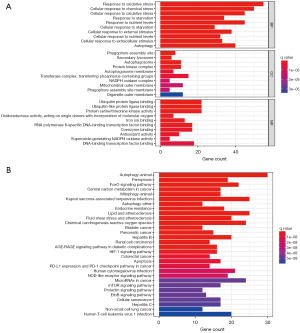
Construction of the prognostic model of DEFerlncRNA
The univariate Cox analysis with a P value <0.001 of OS screened 20 prognostic DEFerlncRNAs (Figure 3A). LASSO regression analysis indicated that 13 DEFerlncRNA were more significant (Figure 3B,3C), and multivariate Cox analysis finally confirmed nine DEFerlncRNAs for constructing the model. The lncRNA in the model was then investigated through univariate and multivariate Cox analyses (Figure 3D,3E). The patient’s risk score was calculated as follows: risk score = expression condition of AC103563.2 × (0.245115408) + expression condition of DM1-AS × (0.256214921) + expression condition of AC080013.4 × (0.08810844) + expression condition of LINC01629 × (0.140964966) + expression condition of AC009237.15 × (0.062121601) + expression condition of BOLA3-AS1 × (0.160408677) + expression condition of RAB11B-AS1 × (−0.149834647) + expression condition of AC244517.7 × (0.387741188) + expression condition of CFAP58-DT × (0.772955964). The correlation between this 9 lncRNAs model and FRGs is displayed in Figure 4.
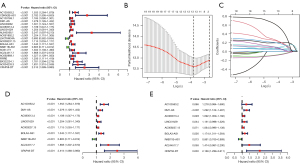
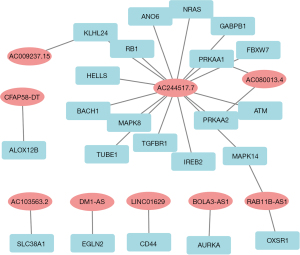
Confirmation of the prognostic model of DEFerlncRNA
The expression spectrum classified the patients as either high- or low-risk (Figure 5A, available online: https://cdn.amegroups.cn/static/public/atm-22-6659-1.xlsx). Meanwhile, we visualized the survival status with an increasing risk score (Figure 5B). Next, PCA and t-distributed stochastic neighbor embedding (t-SNE) analyses suggested that the grouping condition was good (Figure 5C,5D). Furthermore, KM analysis showed the patients in the low-risk group had a poor prognosis (Figure 5E). The area under the ROC curves (AUCs) were above 0.7, with the 1-year AUC reaching 0.758 (Figure 5F).
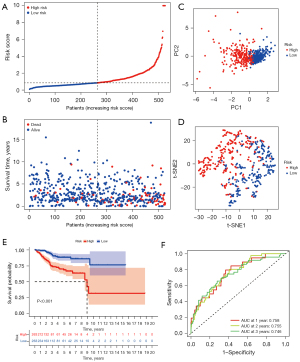
Clinical evaluation of the prognostic model of DEFerlncRNA
The univariate and multivariate Cox analysis including the clinicopathological characteristics showed that the DEFerlncRNA prognostic model could be an independent prognostic index (Figure 6A,6B). The clinicopathological characteristics were significantly correlated and had a positive relationship with the risk score (Figure 7A-7D). Thus, our model can predict the diagnosis to some degree. In addition, the AUCs of the ROC curves and DCA indicated that our model was the most efficient (Figure 8A,8B). The nomogram uniting the model and clinical features was utilized for survival prediction (Figure 8C).
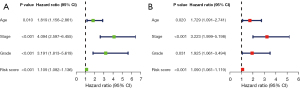
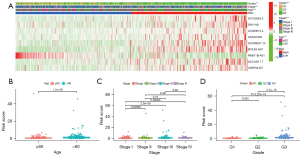
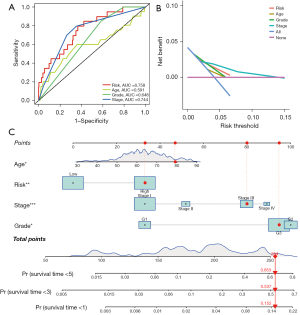
GSEA of the DEFerlncRNA prognostic model
DEGs were employed for GSEA to determine the enriched pathways among the two groups. DEGs in the low-risk group were enriched in antigen processing and presentation (Figure 9A), natural killer cell-mediated cytotoxicity (Figure 9B), cell adhesion molecules (CAMs) (Figure 9C), the Janus Kinase-Signal Transducer and Activator of Transcription (JAK-STAT) signaling pathway (Figure 9D), the cytosolic and sensing pathway (Figure 9E), and the apoptosis pathway (Figure 9F). DEGs enriched in extracellular cell matrix (ECM) receptor interaction (Figure 9G), focal adhesion (Figure 9H), and the hedgehog signaling pathway were found in the high-risk group (Figure 9I).
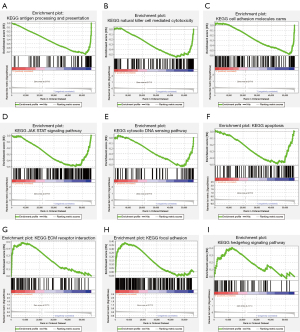
Evaluation of the immune-infiltrating condition of the DEFerlncRNA prognostic model
We found that patients with high-risk scores had higher infiltration of immune cells, such as neutrophils, macrophages, plasma cells, and T cells, using a conjoint analysis of many well-known immune-related databases (Figure 10A, available online: https://cdn.amegroups.cn/static/public/atm-22-6659-1.xlsx). The ssGSEA analysis indicated that most of the immune cell proportions and immune functions differed significantly between the two groups (Figure 10B,10C).
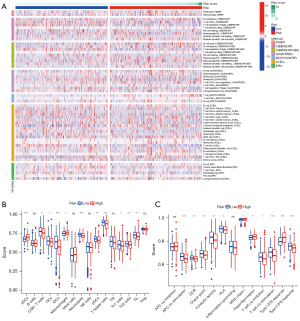
Knockdown of CFAP58-DT inhibits EC cells’ viability and migration
CFAP58-DT exhibited the largest coefficient in the model, so we further studied the expression of CFAP58-DT of EC as well as its effect on cell biological behavior. To explore the roles of CFAP58-DT on the viability, invasion, and migration of EC cells, we built a downregulated CFAP58-DT model in the HEC-1A and Ishikawa cell lines. Figure 11A displays the knockdown efficiency. The CCK-8 experiments indicated that cell viability decreased following CFAP58-DT knockdown (Figure 11B,11C). The Transwell assays demonstrated that the invasion and migration abilities of the two cell lines were attenuated following CFAP58-DT downregulation (Figure 11D,11E).
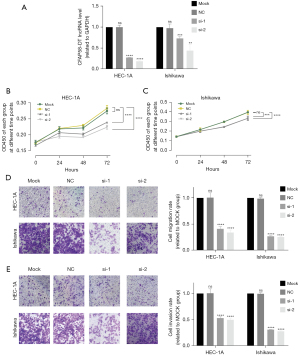
Discussion
Ferroptosis is associated with numerous human processes and diseases, including tumor inhibition, neuronal degeneration, the antiviral immune response, and ischemia-reperfusion injury. Therefore, ferroptosis could be promoted to eliminate harmful cancer cells and virus-infected cells, or inhibited to protect healthy cells. It has also been reported that ferroptosis plays an important role in EC (22,43). However, there are no reports on the DEFerlncRNA model in EC.
Transcriptome profiling in TCGA database and 382 FRGs were screened to differentiate ECs from normal tumors. Finally, we found that 1,731 FerlncRNA and 209 FRGs were significantly differentially expressed in EC. Further functional analysis of 209 DEFRGs using GO and KEGG indicated that these genes were enriched in a variety of carcinogenic processes. The response to oxidative stress, autophagy, PD-L1 expression, and PD-1 checkpoint, HIF, and mTOR signaling pathways are all well-known cancer-related pathways. Lysosomal stress, as previously stated, can lead to the inactivation of the mTOR autophagy-related pathway and the nuclear translocation of TFEB (triggered by transcription factor EB). TFEB dependently enhances lysosomal proteins and superoxide dismutase (SOD) expression and then inhibits ROS production and ferroptosis (44). In addition, a recent study has shown that tyrosine-protein kinase receptor TYRO3 (TYRO3) is highly expressed in tumors, inhibiting the ferroptosis of tumor cells caused by anti-PD-1/PD-L1 and promoting the formation of the tumor precursor microenvironment by reducing the M1/M2 macrophage ratio, resulting in drug resistance to PD-1/PD-L1 treatment (45). Miess et al. reported in 2018 that the expression level of von hippel-lindau tumor suppressor (VHL), a regulator of hypoxia-inducible factors, is related to ferroptosis induction in clear cell renal cell carcinoma (ccRCC) (46). According to Li et al., FG-4592, as an inhibitor of prolyl hydroxylase of HIF, activates nuclear factor erythroid 2-related factor 2 (Nrf2) and decreases ferroptosis in the initial process of folic acid-induced kidney injury (47). Overall, although ferroptosis is known to affect cancer cell progression, further exploration in EC is still needed.
Recently, the relationship between lncRNA and ferroptosis has been reported. For example, the lncRNAs MT1DP and LINC00618 accelerates ferroptosis, while the lncRNAs OIP-AS1, RP11-89, and LINC00336 induce ferroptosis resistance in cancers, highlighting the importance of FerlncRNA in carcinogenesis (48-52). Therefore, through Cox regression analysis, 9 DEFerlncRNAs were utilized for model construction in this study. Also, the conformation and clinical evaluation of the DEFerlncRNA prognostic model suggested that it could be an independent prognostic index with improved sensitivity, specificity, and efficiency in EC. The DEGs between the two groups were then found to be functionally enriched in the GSEA and immune characteristics. Using GSEA software, we identified that antigen processing and presentation, natural killer cell-mediated cytotoxicity, CAMs, the JAK-STAT signaling pathway, the cytosolic and sensing pathway, the apoptosis pathway, ECM receptor interaction, focal adhesion, and the hedgehog signaling pathway were all enriched.
The next step was to compare the immune-infiltrating conditions of the two groups. The results indicated that most of the immune cell proportions and immune functions were significantly different between the two groups. Dendritic cells and T cells were shown to be downregulated in the high-risk group. To initiate an immune response, antigen-presenting cells absorb, process, and transmit ferroptotic cells to T cells, activate T cells, and stimulate cytokine secretion (5). Although some previous studies have investigated ferroptosis and immune infiltrating conditions, further research is still needed to uncover the underlying mechanism.
Our model’s highest coefficient is CFAP58-DT, and high CFAP58-DT expression is related to poor EC outcomes. So, we explored the effects of CFAP58-DT on the viability, invasion, and migration of EC cells and discovered that CFAP58-DT knockdown significantly reduced cell viability, invasion, and migration abilities of HEC-1A and Ishikawa cells.
Although we employed machine learning methods to construct a 9-FerlncRNA model and conducted some experiments to discover the role of CFAP58-DT in vitro, our research has significant limitations that should be considered. Firstly, since there are no other open databases with EC-related clinical and survival information, further efforts in terms of the inclusion, detection, and follow-up of EC patients are needed to verify the constitution of this model, and EC needs to be further studied. Lastly, more experiments are needed to explore the immune conditions and risk score differences in EC.
Conclusions
In summary, we used machine learning methods to identify a 9-FerlncRNA (AC103563.2, DM1-AS, AC080013.4, LINC01629, AC009237.15, BOLA3-AS1, RAB11B-AS1, AC244517.7, CFAP58-DT) model including CFAP58-DT for the prediction of EC prognosis and immune-infiltrating conditions. We also inferred the potential oncogenic role of CFAP58-DT in EC. However, the model constructed in this study and the roles of lncRNAs in EC still require further clinical research. Moreover, the mechanism and relationship between lncRNAs should also be considered more closely.
Acknowledgments
Funding: None.
Footnote
Reporting Checklist: The authors have completed the TRIPOD and MDAR reporting checklists. Available at https://atm.amegroups.com/article/view/10.21037/atm-22-6659/rc
Data Sharing Statement: Available at https://atm.amegroups.com/article/view/10.21037/atm-22-6659/dss
Conflicts of Interest: All authors have completed the ICMJE uniform disclosure form (available at https://atm.amegroups.com/article/view/10.21037/atm-22-6659/coif). All authors are from Shanghai Huaota Biopharmaceutical Co., Ltd. The authors have no other conflicts of interest to declare.
Ethical Statement: The authors are accountable for all aspects of the work, including ensuring that any questions related to the accuracy or integrity of any part of the work have been appropriately investigated and resolved. The study was conducted in accordance with the Declaration of Helsinki (as revised in 2013).
Open Access Statement: This is an Open Access article distributed in accordance with the Creative Commons Attribution-NonCommercial-NoDerivs 4.0 International License (CC BY-NC-ND 4.0), which permits the non-commercial replication and distribution of the article with the strict proviso that no changes or edits are made and the original work is properly cited (including links to both the formal publication through the relevant DOI and the license). See: https://creativecommons.org/licenses/by-nc-nd/4.0/.
References
- Siegel RL, Miller KD, Fuchs HE, et al. Cancer Statistics, 2021. CA Cancer J Clin 2021;71:7-33. [Crossref] [PubMed]
- Amant F, Moerman P, Neven P, et al. Endometrial cancer. Lancet 2005;366:491-505. [Crossref] [PubMed]
- Moore K, Brewer MA. Endometrial Cancer: Is This a New Disease? Am Soc Clin Oncol Educ Book 2017;37:435-42. [Crossref] [PubMed]
- Smith D, Stewart CJR, Clarke EM, et al. ER and PR expression and survival after endometrial cancer. Gynecol Oncol 2018;148:258-66. [Crossref] [PubMed]
- Friedmann Angeli JP, Krysko DV, Conrad M. Ferroptosis at the crossroads of cancer-acquired drug resistance and immune evasion. Nat Rev Cancer 2019;19:405-14. [Crossref] [PubMed]
- Bebber CM, Müller F, Prieto Clemente L, et al. Ferroptosis in Cancer Cell Biology. Cancers (Basel) 2020;12:164. [Crossref] [PubMed]
- Xu T, Ding W, Ji X, et al. Molecular mechanisms of ferroptosis and its role in cancer therapy. J Cell Mol Med 2019;23:4900-12. [Crossref] [PubMed]
- Liang JY, Wang DS, Lin HC, et al. A Novel Ferroptosis-related Gene Signature for Overall Survival Prediction in Patients with Hepatocellular Carcinoma. Int J Biol Sci 2020;16:2430-41. [Crossref] [PubMed]
- Qi W, Li Z, Xia L, et al. LncRNA GABPB1-AS1 and GABPB1 regulate oxidative stress during erastin-induced ferroptosis in HepG2 hepatocellular carcinoma cells. Sci Rep 2019;9:16185. [Crossref] [PubMed]
- Shang Y, Luo M, Yao F, et al. Ceruloplasmin suppresses ferroptosis by regulating iron homeostasis in hepatocellular carcinoma cells. Cell Signal 2020;72:109633. [Crossref] [PubMed]
- Li D, Liu S, Xu J, et al. Ferroptosis-related gene CHAC1 is a valid indicator for the poor prognosis of kidney renal clear cell carcinoma. J Cell Mol Med 2021;25:3610-21. [Crossref] [PubMed]
- Markowitsch SD, Schupp P, Lauckner J, et al. Artesunate Inhibits Growth of Sunitinib-Resistant Renal Cell Carcinoma Cells through Cell Cycle Arrest and Induction of Ferroptosis. Cancers (Basel) 2020;12:3150. [Crossref] [PubMed]
- Yang WH, Ding CC, Sun T, et al. The Hippo Pathway Effector TAZ Regulates Ferroptosis in Renal Cell Carcinoma. Cell Rep 2019;28:2501-2508.e4. [Crossref] [PubMed]
- Wang Z, Ding Y, Wang X, et al. Pseudolaric acid B triggers ferroptosis in glioma cells via activation of Nox4 and inhibition of xCT. Cancer Lett 2018;428:21-33. [Crossref] [PubMed]
- Zhang HY, Zhang BW, Zhang ZB, et al. Circular RNA TTBK2 regulates cell proliferation, invasion and ferroptosis via miR-761/ITGB8 axis in glioma. Eur Rev Med Pharmacol Sci 2020;24:2585-600. [PubMed]
- Zhuo S, Chen Z, Yang Y, et al. Clinical and Biological Significances of a Ferroptosis-Related Gene Signature in Glioma. Front Oncol 2020;10:590861. [Crossref] [PubMed]
- Carbone M, Melino G. Stearoyl CoA Desaturase Regulates Ferroptosis in Ovarian Cancer Offering New Therapeutic Perspectives. Cancer Res 2019;79:5149-50. [Crossref] [PubMed]
- Tesfay L, Paul BT, Konstorum A, et al. Stearoyl-CoA Desaturase 1 Protects Ovarian Cancer Cells from Ferroptotic Cell Death. Cancer Res 2019;79:5355-66. [Crossref] [PubMed]
- Wang Y, Zhao G, Condello S, et al. Frizzled-7 Identifies Platinum-Tolerant Ovarian Cancer Cells Susceptible to Ferroptosis. Cancer Res 2021;81:384-99. [Crossref] [PubMed]
- Ma RH, Ni ZJ, Thakur K, et al. Transcriptome and proteomics conjoint analysis reveal metastasis inhibitory effect of 6-shogaol as ferroptosis activator through the PI3K/AKT pathway in human endometrial carcinoma in vitro and in vivo. Food Chem Toxicol 2022;170:113499. [Crossref] [PubMed]
- Zhou D, Wu Q, Qiu H, et al. Simvastatin Inhibits Endometrial Cancer Malignant Behaviors by Suppressing RAS/Mitogen-Activated Protein Kinase (MAPK) Pathway-Mediated Reactive Oxygen Species (ROS) and Ferroptosis. Evid Based Complement Alternat Med 2022;2022:6177477. [Crossref] [PubMed]
- Wang H, Peng S, Cai J, et al. Silencing of PTPN18 Induced Ferroptosis in Endometrial Cancer Cells Through p-P38-Mediated GPX4/xCT Down-Regulation. Cancer Manag Res 2021;13:1757-65. [Crossref] [PubMed]
- Li X, Zhu Q, Ma M, et al. Quercetin inhibits the progression of endometrial HEC-1-A cells by regulating ferroptosis-a preliminary study. Eur J Med Res 2022;27:292. [Crossref] [PubMed]
- Wei S, Yu Z, Shi R, et al. GPX4 suppresses ferroptosis to promote malignant progression of endometrial carcinoma via transcriptional activation by ELK1. BMC Cancer 2022;22:881. [Crossref] [PubMed]
- Zhang J, Chen S, Wei S, et al. CircRAPGEF5 interacts with RBFOX2 to confer ferroptosis resistance by modulating alternative splicing of TFRC in endometrial cancer. Redox Biol 2022; Epub ahead of print. [Crossref] [PubMed]
- Sun Q, Zhen P, Li D, et al. Amentoflavone promotes ferroptosis by regulating reactive oxygen species (ROS) /5'AMP-activated protein kinase (AMPK)/mammalian target of rapamycin (mTOR) to inhibit the malignant progression of endometrial carcinoma cells. Bioengineered 2022;13:13269-79. [Crossref] [PubMed]
- Zhong FM, Yao FY, Liu J, et al. Ferroptosis-related molecular patterns reveal immune escape, inflammatory development and lipid metabolism characteristics of the tumor microenvironment in acute myeloid leukemia. Front Oncol 2022;12:888570. [Crossref] [PubMed]
- Aljubran F, Nothnick WB. Long non-coding RNAs in endometrial physiology and pathophysiology. Mol Cell Endocrinol 2021;525:111190. [Crossref] [PubMed]
- Statello L, Guo CJ, Chen LL, et al. Gene regulation by long non-coding RNAs and its biological functions. Nat Rev Mol Cell Biol 2021;22:96-118. [Crossref] [PubMed]
- Liu H, Wan J, Chu J. Long non-coding RNAs and endometrial cancer. Biomed Pharmacother 2019;119:109396. [Crossref] [PubMed]
- Amelio I, Bernassola F, Candi E. Emerging roles of long non-coding RNAs in breast cancer biology and management. Semin Cancer Biol 2021;72:36-45. [Crossref] [PubMed]
- Liu Y, Cai X, Cai Y, et al. lncRNA OIP5-AS1 Suppresses Cell Proliferation and Invasion of Endometrial Cancer by Regulating PTEN/AKT via Sponging miR-200c-3p. J Immunol Res 2021;2021:4861749. [Crossref] [PubMed]
- Piergentili R, Zaami S, Cavaliere AF, et al. Non-Coding RNAs as Prognostic Markers for Endometrial Cancer. Int J Mol Sci 2021;22:3151. [Crossref] [PubMed]
- Park EG, Pyo SJ, Cui Y, et al. Tumor immune microenvironment lncRNAs. Brief Bioinform 2022;23:bbab504. [Crossref] [PubMed]
- Mao TL, Fan MH, Dlamini N, et al. LncRNA MALAT1 Facilitates Ovarian Cancer Progression through Promoting Chemoresistance and Invasiveness in the Tumor Microenvironment. Int J Mol Sci 2021;22:10201. [Crossref] [PubMed]
- Fan Y, Dong X, Li M, et al. LncRNA KRT19P3 Is Involved in Breast Cancer Cell Proliferation, Migration and Invasion. Front Oncol 2022;11:799082. [Crossref] [PubMed]
- Wo L, Zhang X, Ma C, et al. LncRNA HABON promoted liver cancer cells survival under hypoxia by inhibiting mPTP opening. Cell Death Discov 2022;8:171. [Crossref] [PubMed]
- Gu B, Shang X, Yan M, et al. Variations in incidence and mortality rates of endometrial cancer at the global, regional, and national levels, 1990-2019. Gynecol Oncol 2021;161:573-80. [Crossref] [PubMed]
- Zhang X, Yang Q. A Pyroptosis-Related Gene Panel in Prognosis Prediction and Immune Microenvironment of Human Endometrial Cancer. Front Cell Dev Biol 2021;9:705828. [Crossref] [PubMed]
- Zhang X, Yang Q. An Immune-Related lncRNA Pairing Model for Predicting the Prognosis and Immune-Infiltrating Cell Condition in Human Ovarian Cancer. Biomed Res Int 2022;2022:3168408. [Crossref] [PubMed]
- Vickers AJ, Elkin EB. Decision curve analysis: a novel method for evaluating prediction models. Med Decis Making 2006;26:565-74. [Crossref] [PubMed]
- Subramanian A, Tamayo P, Mootha VK, et al. Gene set enrichment analysis: a knowledge-based approach for interpreting genome-wide expression profiles. Proc Natl Acad Sci U S A 2005;102:15545-50. [Crossref] [PubMed]
- López-Janeiro Á, Ruz-Caracuel I, Ramón-Patino JL, et al. Proteomic Analysis of Low-Grade, Early-Stage Endometrial Carcinoma Reveals New Dysregulated Pathways Associated with Cell Death and Cell Signaling. Cancers (Basel) 2021;13:794. [Crossref] [PubMed]
- Li L, Sun S, Tan L, et al. Polystyrene Nanoparticles Reduced ROS and Inhibited Ferroptosis by Triggering Lysosome Stress and TFEB Nucleus Translocation in a Size-Dependent Manner. Nano Lett 2019;19:7781-92. [Crossref] [PubMed]
- Jiang Z, Lim SO, Yan M, et al. TYRO3 induces anti-PD-1/PD-L1 therapy resistance by limiting innate immunity and tumoral ferroptosis. J Clin Invest 2021;131:e139434. [Crossref] [PubMed]
- Miess H, Dankworth B, Gouw AM, et al. The glutathione redox system is essential to prevent ferroptosis caused by impaired lipid metabolism in clear cell renal cell carcinoma. Oncogene 2018;37:5435-50. [Crossref] [PubMed]
- Li X, Zou Y, Xing J, et al. Pretreatment with Roxadustat (FG-4592) Attenuates Folic Acid-Induced Kidney Injury through Antiferroptosis via Akt/GSK-3β/Nrf2 Pathway. Oxid Med Cell Longev 2020;2020:6286984. [Crossref] [PubMed]
- Zhang Y, Guo S, Wang S, et al. LncRNA OIP5-AS1 inhibits ferroptosis in prostate cancer with long-term cadmium exposure through miR-128-3p/SLC7A11 signaling. Ecotoxicol Environ Saf 2021;220:112376. [Crossref] [PubMed]
- Wang Z, Chen X, Liu N, et al. A Nuclear Long Non-Coding RNA LINC00618 Accelerates Ferroptosis in a Manner Dependent upon Apoptosis. Mol Ther 2021;29:263-74. [Crossref] [PubMed]
- Wang M, Mao C, Ouyang L, et al. Correction to: Long noncoding RNA LINC00336 inhibits ferroptosis in lung cancer by functioning as a competing endogenous RNA. Cell Death Differ 2020;27:1447. [Crossref] [PubMed]
- Luo W, Wang J, Xu W, et al. LncRNA RP11-89 facilitates tumorigenesis and ferroptosis resistance through PROM2-activated iron export by sponging miR-129-5p in bladder cancer. Cell Death Dis 2021;12:1043. [Crossref] [PubMed]
- Gai C, Liu C, Wu X, et al. MT1DP loaded by folate-modified liposomes sensitizes erastin-induced ferroptosis via regulating miR-365a-3p/NRF2 axis in non-small cell lung cancer cells. Cell Death Dis 2020;11:751. [Crossref] [PubMed]
(English Language Editor: A. Kassem)