Nomogram for tumour response based on prospective cohorts of hepatocellular carcinoma patients receiving immunotherapy combined with targeted therapy: development and validation
Highlight box
Key findings
• This study constructed robust and pragmatic models for predicting the tumour response in hepatocellular carcinoma patients receiving immunotherapy combined with targeted therapy on the basis of synthesized analysis of tumour burden, pre-treatment laboratory indicators and immune target-related adverse events.
What is known and what is new?
• Although immunotherapy combined with targeted therapy can be effective for hepatocellular carcinoma, not all patients respond to this treatment, and models for predicting tumour response in these patients are lacking.
• This study aimed to explore how clinical characteristics and immune target-related adverse events affect tumour response in HCC patients and to establish a holistic predictive nomogram for tumour response based on the analysis of prospective cohorts.
What is the implication, and what should change now?
• The current study provides clinicians with new insights into selecting patients for immunotherapy combined with targeted therapy and contributes to the development of immunotherapy for HCC.
Introduction
Primary liver cancer is a common malignant cancer of the digestive tract, with morbidity and mortality ranked 6th and 3rd in the global cancer rankings in 2020 (1). Hepatocellular carcinoma (HCC) comprises 75 to 85% of primary liver cancers. Surgery is the only curative option for HCC patients, but only a small proportion of patients are considered ideal candidates for resection (2). In addition, even after R0 resection, the recurrence rate of HCC at 5 years is nearly 60% (3).
Although immunotherapies are revolutionizing the management of cancer (4-6) and immunotherapy combined with targeted therapy represents a paradigm shift in the first-line treatment of patients with advanced HCC (7-9), not all tumours respond to immunotherapy combined with targeted therapy; many tumours that initially respond eventually become resistant (10). Predicting the efficacy of immunotherapy and its combinations at an early stage of cancer could improve the tumour response (11). Unfortunately, no potent biomarkers for predicting the tumour response to immunotherapy combined with targeted therapy in HCC patients have been identified, and no available biomarkers promoting clinical decision-making have been validated (12). Previous studies have partially shed light on the mechanisms underpinning the tumour response in HCC patients (13,14); however, the clinical significance of the mechanisms for predicting the tumour response has not been validated. Several studies have established predictive models for the tumour response in HCC patients. Nevertheless, most of these studies (15-17) overlooked patients who received immunotherapy combined with targeted treatment, and the models were mainly constructed based on the signatures of gene pairs or long noncoding RNAs that were not commonly detected; thus, the existing models are both expensive and inconvenient.
Due to the increasing administration (18,19) of immunotherapy combined with targeted therapy in HCC patients and the lack of predictive models for the tumour response, it is of critical importance to investigate useful markers and construct novel and pragmatic models for the prediction of the tumour response in HCC patients receiving immunotherapy combined with targeted treatment. However, there is a lack of evidence on how immune-related adverse events (irAEs) affect tumour response in HCC patients. RESCUE (9) and HX008 were two prospective phase II trials focusing on immunotherapy combined with targeted treatment in advanced HCC patients. Based on these prospective cohorts, this study aimed to explore how clinical characteristics (e.g., tumour burden and pre-treatment laboratory indicators) and immune target-related adverse events (itrAEs) affect the tumour response in HCC patients and establish a holistic predictive nomogram for tumour response. The following article is presented in accordance with the TRIPOD reporting checklist (available at https://atm.amegroups.com/article/view/10.21037/atm-22-3045/rc).
Methods
Study population
Between March 2018 and August 2021, a total of 221 HCC patients from two prospective cohorts (RESCUE and HX008) were reviewed, and the patient sample size depended on the enrolment of those two trials. Patients were included in this study based on our inclusion and exclusion criteria. The study was conducted in accordance with the Declaration of Helsinki (as revised in 2013). This study was approved by the institutional review boards of the participating centres (approval No: 2017L04642; trial registration No. NCT03463876), and all patients provided informed consent. The inclusion criteria for this study were as follows: (I) patients with a pathologically confirmed diagnosis of unresectable or metastatic advanced HCC; (II) patients who received immunotherapy combined with targeted therapy; (III) patients with at least one assessable target lesion [according to Response Evaluation Criteria in Solid Tumours (RECIST) v1.1 guidelines] identified by imaging examination during the period of immunotherapy combined with targeted therapy; and (IV) patients with Barcelona Clinic Liver Cancer (BCLC) stage B or C disease, an Eastern Cooperative Oncology Group (ECOG) performance status of 0 or 1 and Child–Pugh class A status. The exclusion criteria were as follows: (I) patients who had previously received radiotherapy, chemotherapy, hormone therapy or surgery within 4 weeks of commencing immunotherapy combined with targeted therapy; (II) patients with any history of active autoimmune disease or concurrent medical use of immunosuppressive medications; (III) patients with other malignant tumours; or (IV) patients lacking follow-up data or clinical data. The patients were randomly divided into training (n=154) and validation cohorts (n=67) at a ratio of 7:3. The randomization process of our study was performed once with the createDataPartition function of the “caret” package of R studio. Among the patients, 109 of them received first-line treatment, and 112 patients received second-line treatment.
Study variables and treatment
Standard clinical data were collected for each patient, including age, sex, hepatitis B infection status, laboratory tests, alpha fetoprotein (AFP) level, Child-Pugh stage, Eastern Cooperative Oncology Group Performance Status (ECOG PS), and itrAEs. Tumour-related data were also collected, including BCLC stage, tumour size, number of tumours, macrovascular invasion, extrahepatic metastasis, and tumour response. A baseline laboratory test was performed per routine clinical practice, which included blood count, myocardial enzyme levels, thyroid function, kidney function and liver function. The pre-treatment neutrophil-to-lymphocyte ratio (NLR), platelet-to-lymphocyte ratio (PLR), prognostic nutritional index (PNI), aspartate aminotransferase (AST)-to-platelet ratio (APRI) and AST-to-neutrophil ratio index (ANRI) were evaluated. After the beginning of the treatment, clinical and laboratory tests were carried out as clinically indicated at least every 2 weeks in each patient. Tumour responses were evaluated using RECIST 1.1 guidelines. itrAEs were defined and graded based on the Common Terminology Criteria for Adverse Events version 4.0. Two small-molecule targeted drugs, lenvatinib and apatinib, were included in this study. Lenvatinib was administered once daily at 8 mg (patient weight less than 60 kg) or 12 mg (patient weight greater than or equal to 60 kg). Apatinib was administered once daily at 250 mg. This study included two anti-PD-1 agents, which were given at 200 mg every 3 weeks.
Outcomes
Following immunotherapy combined with targeted therapy, all patients were regularly followed and monitored for cancer progression via thoracic, abdominal, and pelvic computed tomography (CT) and magnetic resonance imaging (MRI) scans of the liver at least every 6 weeks. Tumour response was evaluated using RECIST 1.1 guidelines as progressive disease (PD), stable disease (SD), partial response (PR) or complete response (CR). In our study, objective response (OR) was defined as PR or CR, whereas disease control (DC) was defined as SD, PR or CR.
Statistical analysis
The chi-square test was performed to compare the categorical variables presented as percentages. Continuous variables were compared using t-tests or Mann-Whitney U tests. A receiver operating characteristic curve was constructed to estimate the predictive value of the pretreatment NLR, PLR, PNI, APRI and ANRI. The optimal cut-off values of these markers for tumour response were identified by the highest Youden index (sensitivity + specificity − 1). Since there were no verified biomarkers associated with the tumour response in HCC patients who received immunotherapy combined with targeted therapy, we included as many variables as possible in the univariate analysis, which could be classified as variables of tumour burden, pretreatment laboratory indicators and itrAEs. All predictors with P<0.10 in the univariate analysis were retained to construct the multivariate logistic regression model to estimate the association between the included variables and the tumour response. Then, all the predictors with P<0.05 were included in the multivariate logistic regression model. The multivariate logistic regression model included all the statistically significant risk factors to construct the nomogram in the training set. The nomogram constructed from the training set was evaluated in the validation, first-line and second-line treatment sets. The areas under the receiver operating characteristic curves (AUROCs) were used to determine the sensitivity and specificity of the nomogram. Calibration plots and Hosmer-Lemeshow chi-square tests were performed to assess the model calibration. Differences with two-sided P<0.05 were considered statistically significant in all statistical analyses. Statistical analyses were conducted using SPSS version 25 software (Armonk NY, USA) and R software (http://www.r-project.org).
Results
Patients and treatment (training set)
A total of 154 patients were enrolled in the training set. The median age of the study population was 53.0 [interquartile range (IQR), 44.8–59.0] years. A total of 138 (89.6%) patients were male, and 130 (84.4%) patients had a hepatitis B infection. All patients were Child-Pugh stage A. ECOG PS greater than or equal to 1 was observed in 71 (46.1%) patients. BCLC stages B and C were found in 35 and 119 patients, respectively. The median largest lesion size was 3.4 (IQR, 2.0–5.1) cm. Regarding targeted treatment, 29 (18.8%) patients received lenvatinib, and 125 (81.2%) received apatinib. The main baseline characteristics are shown in Table 1.
Table 1
Items | Training set (n=154) | Validation set (n=67) | P value |
---|---|---|---|
Age, years, median (IQR) | 53.0 (44.8–59.0) | 52.0 (45.0–62.0) | 0.818 |
Male, n (%) | 138 (89.6) | 59 (88.1) | 0.733 |
Hepatitis B infection, n (%) | 130 (84.4) | 60 (89.5) | 0.312 |
Child-Pugh stage A, n (%) | 154 (100.0) | 67 (100.0) | − |
ECOG PS ≥1, n (%) | 71 (46.1) | 32 (47.8) | 0.820 |
Pretreatment AFP ≥200 ng/mL, n (%) | 15 (9.7) | 4 (6.0) | 0.358 |
Tumour size, cm, median (IQR) | 3.4 (2.0–5.1) | 3.3 (2.1–5.9) | 0.958 |
Multiple tumours, n (%) | 119 (77.3) | 49 (73.1) | 0.508 |
Macrovascular invasion, n (%) | 41 (26.6) | 21 (31.3) | 0.929 |
Extrahepatic metastasis, n (%) | 110 (71.4) | 43 (64.2) | 0.283 |
BCLC stage, n (%) | 0.176 | ||
B | 35 (22.7) | 21 (31.3) | |
C | 119 (77.3) | 46 (68.7) | |
Targeted therapy, n (%) | 0.543 | ||
Lenvatinib | 29 (18.8) | 15 (22.4) | |
Apatinib | 125 (81.2) | 52 (77.6) | |
Platelet count, ×109/L, median (IQR) | 156.5 (124.75–186.25) | 155.0 (120.0–191.0) | 0.912 |
INR (× ULN), mean ± SD | 1.0±0.1 | 1.0±0.1 | 0.658 |
NLR ≥6.46 | 8 (5.2) | 2 (3.0) | 0.468 |
PLR ≥234.55 | 11 (7.1) | 5 (7.5) | 0.933 |
PNI ≥54.30 | 28 (18.2) | 11 (16.4) | 0.752 |
APRI ≥0.32 | 130 (84.4) | 55 (82.1) | 0.667 |
ANRI ≥3.53 | 149 (96.8) | 65 (97.0) | 0.919 |
Prior regional treatment, n (%) | |||
Surgery | 108 (70.1) | 46 (68.7) | 0.827 |
Interventional therapy | 62 (40.3) | 28 (41.2) | 0.831 |
Ablation | 38 (24.7) | 20 (29.9) | 0.178 |
Radiotherapy | 14 (9.1) | 7 (10.4) | 0.752 |
Other | 12 (7.8) | 6 (9.0) | 0.771 |
Treatment line, n (%) | 0.549 | ||
First-line treatment | 78 (50.6) | 31 (46.3) | |
Second-line treatment | 76 (49.4) | 36 (53.7) |
HCC, hepatocellular carcinoma; IQR, interquartile range; ECOG PS, Eastern Cooperative Oncology Group Performance Status; AFP, alpha fetoprotein; BCLC, Barcelona Clinic Liver Cancer; INR, international normalized ratio; ULN, upper limit of normal; NLR, neutrophil-to-lymphocyte ratio; PLR, platelet-to-lymphocyte ratio; PNI, prognostic nutritional index; APRI, aspartate aminotransferase-to-platelet ratio index; ANRI, aspartate aminotransferase-to-neutrophil ratio index.
Tumour response and immune target-related adverse events (training set)
Based on the RECIST v1.1 guidelines, CR was observed in one (0.6%) patient, PR in 43 (27.9%), SD in 74 (48.1%), and PD in 36 (23.4%). The OR and DC rates were 28.5% (44/154) and 76.6% (118/154), respectively. itrAEs were recorded at every visit. A total of 150 (97.4%) patients experienced itrAEs. itrAEs of grade 3 to 5 accounted for 72.0% of the AEs. The most common itrAEs were hepatotoxicity (n=119, 77.3%), followed by haematotoxicity (n=102, 66.2%) and hypertension (n=77, 50.0%). The most common haematotoxicity was thrombocytopenia (n=86, 55.8%), followed by neutropenia (n=56, 36.4%) and lymphopenia (n=20, 13.0%). Details are shown in Table 2.
Table 2
Items | Training set (n=154) |
---|---|
Adverse events grade, n (%) | |
0 | 4 (2.6) |
1 | 5 (3.3) |
2 | 34 (22.1) |
3 | 97 (63.0) |
4 | 11 (7.1) |
5 | 3 (1.9) |
Multisystem adverse events, n(%) | 145 (94.2) |
Neutropenia, n (%) | 56 (36.4) |
Lymphopenia, n (%) | 20 (13.0) |
Thrombocytopenia, n (%) | 86 (55.8) |
Elevated alanine aminotransferase, n (%) | 83 (53.9) |
Elevated aspartate aminotransferase, n (%) | 97 (63.0) |
Elevated bilirubin, n (%) | 86 (55.8) |
Thyroid toxicity, n (%) | 28 (18.2) |
Nephrotoxicity, n (%) | 14 (9.1) |
Dermatologic toxicity, n (%) | 72 (46.8) |
Elevated serum cardiac biomarkers, n (%) | 26 (16.9) |
Hypertension, n (%) | 77 (50.0) |
Diarrhoea, n (%) | 40 (26.0) |
Fatigue, n (%) | 49 (31.8) |
Tumour response, n (%) | |
PD | 36 (23.4) |
SD | 74 (48.1) |
PR | 43 (27.9) |
CR | 1 (0.6) |
ORR (PR + CR) | 44 (28.5) |
DCR (SD + PR + CR) | 118 (76.6) |
PD, progressive disease; SD, stable disease; PR, partial response; CR, complete response; ORR, objective response rate; DCR, disease control rate.
Independent predictors of the tumour response in the training set
In the univariate analysis, a single tumour (P=0.012), neutropenia (P=0.004), and hypertension (P=0.014) were associated with OR. These three predictors were included in the multivariate analysis. Multiple tumours (OR =0.298, 95% CI: 0.126–0.705, P=0.006), neutropenia (OR =3.213, 95% CI: 1.475–6.997, P=0.003) and hypertension (OR =2.215, 95% CI: 1.029–4.769, P=0.042) independently predicted ORs (Table 3).
Table 3
Items | Univariate analysis | Multivariate analysis | |||
---|---|---|---|---|---|
P | OR (95% CI) | P | OR (95% CI) | ||
Age ≥60 years | 0.069 | 2.143 (0.943–4.873) | |||
Male | 0.163 | 0.471 (0.164–1.356) | |||
Hepatitis B infection | 0.674 | 1.239 (0.457–3.362) | |||
ECOG PS ≥1 | 0.798 | 1.096 (0.544–2.208) | |||
Preoperative AFP ≥200 ng/mL | 0.443 | 0.598 (0.160–2.229) | |||
Tumour size ≥5 cm | 0.138 | 0.519 (0.218–1.235) | |||
Multiple tumours | 0.012 | 0.365 (0.166–0.804) | 0.006 | 0.298 (0.126–0.705) | |
Macrovascular invasion | 0.852 | 0.921 (0.390–2.180) | |||
Extrahepatic metastasis | 0.312 | 1.521 (0.675–3.429) | |||
BCLC stage C | 0.671 | 1.204 (0.512–2.829) | |||
NLR ≥6.46 | 0.819 | 0.825 (0.160–4.255) | |||
PLR ≥234.55 | 0.555 | 1.471 (0.408–5.301) | |||
PNI ≥54.30 | 1 | 1.000 (0.404–2.476) | |||
APRI ≥0.32 | 0.171 | 0.537 (0.220–1.308) | |||
ANRI ≥3.53 | 0.669 | 1.623 (0.176–14.937) | |||
Multisystem adverse events | 0.259 | 3.373 (0.409–27.795) | |||
itrAEs grade ≥3 | 0.195 | 1.740 (0.754–4.017) | |||
Neutropenia | 0.004 | 2.925 (1.421–6.022) | 0.003 | 3.213 (1.475–6.997) | |
Lymphopenia | 0.087 | 2.314 (0.885–6.054) | |||
Thrombocytopenia | 0.220 | 1.569 (0.764–3.221) | |||
Elevated alanine aminotransferase | 0.241 | 1.532 (0.751–3.124) | |||
Elevated aspartate aminotransferase | 0.116 | 1.846 (0.859–3.966) | |||
Elevated bilirubin | 0.220 | 1.569 (0.764–3.221) | |||
Thyroid toxicity | 0.169 | 1.824 (0.775–4.293) | |||
Nephrotoxicity | 1.000 | 1.000 (0.296–3.374) | |||
Dermatologic toxicity | 0.054 | 2.010 (0.988–4.089) | |||
Elevated serum cardiac biomarkers | 0.456 | 1.407 (0.574–3.448) | |||
Hypertension | 0.014 | 2.497 (1.205–5.147) | 0.042 | 2.215 (1.029–4.769) | |
Diarrhoea | 0.523 | 1.289 (0.591–2.811) | |||
Fatigue | 0.252 | 1.535 (0.737–3.195) |
OR, objective response; ECOG PS, Eastern Cooperative Oncology Group Performance Status; AFP, alpha fetoprotein; BCLC, Barcelona Clinic Liver Cancer; NLR, neutrophil-to-lymphocyte ratio; PLR, platelet-to-lymphocyte ratio; PNI, prognostic nutritional index; APRI, aspartate aminotransferase-to-platelet ratio; ANRI, aspartate aminotransferase-to-neutrophil ratio index; itrAEs, immune target-related adverse events.
In the univariate analysis, tumour size less than 5 cm (P=0.007), PNI greater than or equal to 54.3 (P=0.039), neutropenia (P=0.003), thrombocytopenia (P=0.021), elevated alanine aminotransferase level (P=0.041), and fatigue (P=0.030) were significantly associated with DC. A single tumour (P=0.066) and ANRI greater than or equal to 3.53 (P=0.075) tended to be associated with DC. In the multivariate analysis, tumour size greater than or equal to 5 cm (OR =0.269, 95% CI: 0.107–0.678, P=0.005), multiple tumours (OR =0.282, 95% CI: 0.086–0.928, P=0.037), PNI greater than or equal to 54.3 (OR =5.227, 95% CI: 1.109–24.630, P=0.037), neutropenia (OR =4.931, 95% CI: 1.689–14.396, P=0.004) and fatigue (OR =3.040, 95% CI: 1.048–8.812, P=0.041) independently predicted DC (Table 4).
Table 4
Items | Univariate analysis | Multivariate analysis | |||
---|---|---|---|---|---|
P | OR (95% CI) | P | OR (95% CI) | ||
Age ≥60 years | 0.555 | 1.344 (0.504–3.586) | |||
Male | 0.435 | 1.569 (0.507–4.858) | |||
Hepatitis B infection | 0.749 | 1.190 (0.410–3.450) | |||
ECOG PS ≥1 | 0.095 | 0.525 (0.246–1.119) | |||
Preoperative AFP ≥200 ng/mL | 0.342 | 0.574 (0.183–1.805) | |||
Tumour size ≥5 cm | 0.007 | 0.336 (0.152–0.742) | 0.005 | 0.269 (0.107–0.678) | |
Multiple tumours | 0.066 | 0.351 (0.115–1.072) | 0.037 | 0.282 (0.086–0.928) | |
Macrovascular invasion | 0.551 | 0.766 (0.318–1.842) | |||
Extrahepatic metastasis | 0.338 | 0.651 (0.270–1.566) | |||
BCLC stage C | 0.592 | 0.776 (0.307–1.962) | |||
NLR ≥6.46 | 0.466 | 2.207 (0.262–18.563) | |||
PLR ≥234.55 | 0.674 | 1.404 (0.289–6.813) | |||
PNI ≥54.30 | 0.039 | 4.804 (1.082–21.340) | 0.037 | 5.227 (1.109–24.630) | |
APRI ≥0.32 | 0.269 | 1.697 (0.664–4.340) | |||
ANRI ≥3.53 | 0.075 | 5.273 (0.845–32.886) | |||
Multisystem adverse events | 0.138 | 2.825 (0.716–11.141) | |||
itrAEs grade≥3 | 0.410 | 1.403 (0.627–3.139) | |||
Neutropenia | 0.003 | 4.719 (1.715–12.988) | 0.004 | 4.931 (1.689-14.396) | |
Lymphopenia | 0.703 | 1.255 (0.391–4.025) | |||
Thrombocytopenia | 0.021 | 2.460 (1.144–5.288) | |||
Elevated alanine aminotransferase | 0.041 | 2.213 (1.031–4.749) | |||
Elevated aspartate aminotransferase | 0.150 | 1.745 (0.818–3.721) | |||
Elevated bilirubin | 0.236 | 1.574 (0.744–3.331) | |||
Thyroid toxicity | 0.216 | 2.043 (0.659–6.335) | |||
Nephrotoxicity | 0.631 | 0.741 (0.218–2.521) | |||
Dermatologic toxicity | 0.485 | 1.308 (0.615–2.782) | |||
Elevated serum cardiac biomarkers | 0.297 | 1.833 (0.588–5.721) | |||
Hypertension | 0.255 | 1.550 (0.729–3.297) | |||
Diarrhoea | 0.151 | 2.024 (0.773–5.300) | |||
Fatigue | 0.030 | 2.867 (1.105–7.437) | 0.041 | 3.040 (1.048–8.812) |
DC, disease control; ECOG PS, Eastern Cooperative Oncology Group Performance Status; AFP, alpha fetoprotein; BCLC, Barcelona Clinic Liver Cancer; NLR, neutrophil-to-lymphocyte ratio; PLR, platelet-to-lymphocyte ratio; PNI, prognostic nutritional index; APRI, aspartate aminotransferase-to-platelet ratio; ANRI, aspartate aminotransferase-to-neutrophil ratio index; itrAEs, immune target-related adverse events.
Nomogram development in the training set and validation in the validation, first-line and second-line treatment sets
A nomogram with independent predictors of OR was constructed (Figure 1). The specific scores of independent factors were as follows: single tumour, 100; neutropenia, 97; and hypertension, 66. The total risk scores of each patient ranged from 0 to 263. The nomogram for the prediction of OR had AUROCs of 0.734 (95% CI: 0.653–0.814) (Figure 2A), 0.674 (95% CI: 0.523–0.819) (Figure 2B), 0.730 (95% CI: 0.6346–0.825) (Figure 2C), and 0.707 (95% CI: 0.595–0.820) (Figure 2D) in the training set, validation set, first-line treatment set and second-line treatment set, respectively. The Hosmer–Lemeshow tests and calibration curves showed acceptable model calibration in the training (P=0.879) (Figure 2E), validation (P=0.974) (Figure 2F) first-line treatment (P=0.470) (Figure 2G) and second-line treatment (P=0.747) sets (Figure 2H).
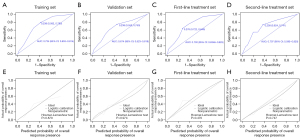
In addition, a nomogram with the independent predictors for DC was established (Figure 3). The specific scores of the independent factors were as follows: tumour size less than 5 cm, 79; single tumour, 77; PNI greater than or equal to 54.3, 100; neutropenia, 96; and fatigue, 67. The total risk scores of each patient ranged from 0 to 419. The nomogram for the prediction of DC had AUROCs of 0.804 (95% CI: 0.725–0.882) (Figure 4A), 0.667 (95% CI: 0.541–0.794) (Figure 4B), and 0.768 (95% CI: 0.671–0.866) (Figure 4C) in the training, first-line and second-line treatment sets, respectively. The Hosmer–Lemeshow tests and calibration curves showed acceptable model calibration in the training (P=0.678) (Figure 4D), first-line treatment (P=0.401) (Figure 4E) and in the second-line treatment (P=0.959) sets (Figure 4F).
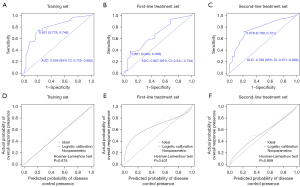
Discussion
This study is the first to collect and analyse information from prospective cohorts to construct predictive models for the tumour response in HCC patients receiving immunotherapy combined with targeted treatment. Notably, since we collected and analysed the information of the prospective cohorts, the reliability and accuracy of the data in our study are much higher than those in retrospective cohorts (15-17,20). Regarding targeted therapy, we only included patients treated with antiangiogenic small-molecule drugs to further eliminate the confounding effects of different treatments. In addition, the effects of tumour burden (tumour size and number), pre-treatment laboratory indicators and itrAEs were comprehensively considered in the predictive models; these models exhibited high specificity and accuracy in the training set and achieved good verification results in the validation, first-line and second-line treatment sets.
Overall, the HCC patients receiving immunotherapy combined with targeted therapy in this study demonstrated an objective response rate (ORR) of 28.5% (44/154), and the disease control rate (DCR) was 76.6% (118/154), consistent with previous clinical trial data (7,8,21) revealing an ORR between 27.3% and 36% and a DCR between 73.6% and 82.8%. However, approximately one-fifth of the HCC patients experienced PD, and over half did not reach OR in these clinical trials. If patients less likely to benefit from combined therapy could be identified early, clinicians could more precisely classify them and develop individualized treatment plans, thus improving the efficiency of medical resource allocation and reducing the time costs to these HCC patients.
No reliable biomarker can precisely predict the tumour response to combined therapy in HCC patients; thus, developing an applicable predictive approach would be of great clinical benefit. Intriguingly, it has been reported that cancer patients with certain types of adverse events have a favourable tumour response and better survival outcomes (22-24). Additionally, the hand-foot skin reaction related to targeted therapy (25) was reported as a beneficial factor in HCC patients, and Lu et al. (20) demonstrated that HCC patients who experienced immunotherapy-related adverse events had better PFS outcomes. However, evidence is lacking regarding whether the adverse events related to immunotherapy combined with targeted therapy affect the tumour response and outcomes in HCC patients. In this study, itrAEs (neutropenia, hypertension and fatigue) were associated with better tumour responses. The potential mechanisms underlying these responses and the associations between itrAEs and tumour responses are unclear. Regarding neutropenia, numerous studies have indicated that neutrophils, especially tumour-associated neutrophils that can inhibit the function of T cells and NK cells, play an important role in cancer initiation and progression (26). In addition, in a meta-analysis of expression signatures from approximately 18,000 human tumours, neutrophils were identified as the immune cell population associated with worse prognoses, and increased neutrophil counts were associated with adverse oncological outcomes (27). Additionally, studies (28,29) have revealed that targeted therapy against neutrophils is another reliable option to suppress tumour progression. Hence, it is reasonable to postulate that neutropenia may indicate that aforementioned adverse effects would be diminished and is thus suggestive of a better tumour response. The itrAEs of hypertension and fatigue could indicate an inflammatory activation of the immune system, which would cause damage to the cancer tissues and influence pressure natriuresis (30) or the peripheral and central nervous systems (31), respectively.
Previous studies have revealed that PNI is associated with the prognosis of oesophageal (32), gastric (33), and colorectal (34) cancer patients; PNI has also been used to predict oncological outcomes in HCC patients receiving immunotherapy (35) or hepatectomy (36). However, the predictive value of PNI in HCC patients receiving immunotherapy combined with targeted therapy remains to be elucidated. Interestingly, this study revealed that a higher PNI was significantly associated with favourable DC. The underlying mechanism could be as follows: (I) a higher PNI indicates a better nutritional status, enhancing T-cell activation by increasing available nutrients, and T cells could thus be released from metabolic competition with cancer cells and prevent the suppression effect (37,38); (II) a lower PNI could reflect a worse tumour-infiltrating lymphocyte status (32), potentially influencing the immune response (39); or (III) a lower PNI indicates malnutrition, which could lead to a hypercatabolic status and accelerate the degradation process of monoclonal antibodies (40). Regarding tumour burden, patients with multiple tumours and tumour sizes greater than or equal to 5 cm had a worse tumour response. Multiple tumours and a tumour size greater than or equal to 5 cm indicate a higher tumour burden, which has been reported (41) to increase the proportion of immunosuppressive cells in the tumour microenvironment and increase suppressive cytokine production in comparison with lower tumour burdens.
More importantly, based on the above analysis, this study was the first to establish prediction models for both OR and DC in HCC patients receiving immunotherapy combined with targeted therapy. In constructing the nomograms, we considered tumour burden, pretreatment laboratory indicators and treatment-related adverse events as essential biomarkers for curative effects. We further assessed the validity of the predictive models in the validation, first-line and second-line treatment sets and found that the sensitivity and specificity of the nomograms were good. Compared with a previous study (35), in which researchers mainly focused on the relationship between a certain type of biomarker and the curative effect in HCC patients, the present study further combined independent and different clinical characteristics to form an objective scoring system that calculated the probability of the objective curative effect for HCC patients in a personalized manner and could thus help clinicians accurately identify patients who would most benefit from immunotherapy combined with targeted therapy.
Our study had several limitations. Firstly, our findings must be interpreted cautiously due to the relatively small number of enrolled HCC patients. Secondly, we could not study the relationship between these characteristics and survival outcomes due to the bias between the two prospective cohorts, which could not be eliminated. Although we included as many variables as possible to predict the tumour response in the current study, there is still a possibility that some variables associated with the tumour response were potentially overlooked, which could influence the predictive ability of our models. In the future, we plan to consider more potential variables to improve the prognostic ability of the models and hope to expand the scale of our research and perform prospective studies to further verify our findings.
In conclusion, we successfully identified valuable markers based on the synthesized analysis of tumour burden, pre-treatment laboratory indicators and itrAEs and constructed robust and pragmatic models for predicting the tumour response in HCC patients receiving immunotherapy combined with targeted therapy. The nomograms had high specificity and accuracy and achieved good verification and can thus help to identify HCC patients who may benefit from immunotherapy combined with targeted therapy and provide guidance for treatment.
Acknowledgments
The relevant data of HX008 in this article were obtained from a clinical trial sponsored by Lepu Biopharma Co., Ltd.
Funding: This study was supported by the National Natural Science Foundation of China (Nos. 81972311, 82141127), the CAMS Innovation Fund for Medical Sciences (CIFMS) (No. 2021-I2M-1-066), the Non-profit Central Research Institution Fund of Chinese Academy of Medical Sciences (No. 2019PT310026), and the Sanming Project of Medicine in Shenzhen (No. SZSM202011010).
Footnote
Reporting Checklist: The authors have completed the TRIPOD reporting checklist. Available at https://atm.amegroups.com/article/view/10.21037/atm-22-3045/rc
Data Sharing Statement: Available at https://atm.amegroups.com/article/view/10.21037/atm-22-3045/dss
Conflicts of Interest: All authors have completed the ICMJE uniform disclosure form (available at https://atm.amegroups.com/article/view/10.21037/atm-22-3045/coif). All authors report that the relevant data of HX008 in this article were from a clinical trial sponsored by Lepu Biopharma Co., Ltd. The authors have no other conflicts of interest to declare.
Ethical Statement: The authors are accountable for all aspects of the work in ensuring that questions related to the accuracy or integrity of any part of the work are appropriately investigated and resolved. The study was conducted in accordance with the Declaration of Helsinki (as revised in 2013). This study was approved by the institutional review boards of the participating centres (No. NCT03463876; 2017L04642), and all patients provided informed consent.
Open Access Statement: This is an Open Access article distributed in accordance with the Creative Commons Attribution-NonCommercial-NoDerivs 4.0 International License (CC BY-NC-ND 4.0), which permits the non-commercial replication and distribution of the article with the strict proviso that no changes or edits are made and the original work is properly cited (including links to both the formal publication through the relevant DOI and the license). See: https://creativecommons.org/licenses/by-nc-nd/4.0/.
References
- Sung H, Ferlay J, Siegel RL, et al. Global Cancer Statistics 2020: GLOBOCAN Estimates of Incidence and Mortality Worldwide for 36 Cancers in 185 Countries. CA Cancer J Clin 2021;71:209-49. [Crossref] [PubMed]
- Roayaie S, Jibara G, Tabrizian P, et al. The role of hepatic resection in the treatment of hepatocellular cancer. Hepatology 2015;62:440-51. [Crossref] [PubMed]
- Tabrizian P, Jibara G, Shrager B, et al. Recurrence of hepatocellular cancer after resection: patterns, treatments, and prognosis. Ann Surg 2015;261:947-55. [Crossref] [PubMed]
- Motzer RJ, Escudier B, McDermott DF, et al. Nivolumab versus Everolimus in Advanced Renal-Cell Carcinoma. N Engl J Med 2015;373:1803-13. [Crossref] [PubMed]
- Sharma P, Retz M, Siefker-Radtke A, et al. Nivolumab in metastatic urothelial carcinoma after platinum therapy (CheckMate 275): a multicentre, single-arm, phase 2 trial. Lancet Oncol 2017;18:312-22. [Crossref] [PubMed]
- Topalian SL, Drake CG, Pardoll DM. Immune checkpoint blockade: a common denominator approach to cancer therapy. Cancer Cell 2015;27:450-61. [Crossref] [PubMed]
- Finn RS, Qin S, Ikeda M, et al. Atezolizumab plus Bevacizumab in Unresectable Hepatocellular Carcinoma. N Engl J Med 2020;382:1894-905. [Crossref] [PubMed]
- Finn RS, Ikeda M, Zhu AX, et al. Phase Ib Study of Lenvatinib Plus Pembrolizumab in Patients With Unresectable Hepatocellular Carcinoma. J Clin Oncol 2020;38:2960-70. [Crossref] [PubMed]
- Xu J, Shen J, Gu S, et al. Camrelizumab in Combination with Apatinib in Patients with Advanced Hepatocellular Carcinoma (RESCUE): A Nonrandomized, Open-label, Phase II Trial. Clin Cancer Res 2021;27:1003-11. [Crossref] [PubMed]
- Giannone G, Ghisoni E, Genta S, et al. Immuno-Metabolism and Microenvironment in Cancer: Key Players for Immunotherapy. Int J Mol Sci 2020;21:4414. [Crossref] [PubMed]
- Hegde PS, Chen DS. Top 10 Challenges in Cancer Immunotherapy. Immunity 2020;52:17-35. [Crossref] [PubMed]
- Llovet JM, Castet F, Heikenwalder M, et al. Immunotherapies for hepatocellular carcinoma. Nat Rev Clin Oncol 2022;19:151-72. [Crossref] [PubMed]
- Huinen ZR, Huijbers EJM, van Beijnum JR, et al. Anti-angiogenic agents - overcoming tumour endothelial cell anergy and improving immunotherapy outcomes. Nat Rev Clin Oncol 2021;18:527-40. [Crossref] [PubMed]
- Viallard C, Audiger C, Popovic N, et al. BMP9 signaling promotes the normalization of tumor blood vessels. Oncogene 2020;39:2996-3014. [Crossref] [PubMed]
- Dai Y, Qiang W, Lin K, et al. An immune-related gene signature for predicting survival and immunotherapy efficacy in hepatocellular carcinoma. Cancer Immunol Immunother 2021;70:967-79. [Crossref] [PubMed]
- Gu X, Guan J, Xu J, et al. Model based on five tumour immune microenvironment-related genes for predicting hepatocellular carcinoma immunotherapy outcomes. J Transl Med 2021;19:26. [Crossref] [PubMed]
- Wang Y, Ge F, Sharma A, et al. Immunoautophagy-Related Long Noncoding RNA (IAR-lncRNA) Signature Predicts Survival in Hepatocellular Carcinoma. Biology (Basel) 2021;10:1301. [Crossref] [PubMed]
- El-Khoueiry AB, Hanna DL, Llovet J, et al. Cabozantinib: An evolving therapy for hepatocellular carcinoma. Cancer Treat Rev 2021;98:102221. [Crossref] [PubMed]
- Hack SP, Spahn J, Chen M, et al. IMbrave 050: a Phase III trial of atezolizumab plus bevacizumab in high-risk hepatocellular carcinoma after curative resection or ablation. Future Oncol 2020;16:975-89. [Crossref] [PubMed]
- Lu L, Xing K, Wei W, et al. Immune-related adverse events predict responses to PD-1 blockade immunotherapy in hepatocellular carcinoma. Int J Cancer 2021; Epub ahead of print. [Crossref] [PubMed]
- Han C, Ye S, Hu C, et al. Clinical Activity and Safety of Penpulimab (Anti-PD-1) With Anlotinib as First-Line Therapy for Unresectable Hepatocellular Carcinoma: An Open-Label, Multicenter, Phase Ib/II Trial (AK105-203). Front Oncol 2021;11:684867. [Crossref] [PubMed]
- Shankar B, Zhang J, Naqash AR, et al. Multisystem Immune-Related Adverse Events Associated With Immune Checkpoint Inhibitors for Treatment of Non-Small Cell Lung Cancer. JAMA Oncol 2020;6:1952-6. [Crossref] [PubMed]
- Maher VE, Fernandes LL, Weinstock C, et al. Analysis of the Association Between Adverse Events and Outcome in Patients Receiving a Programmed Death Protein 1 or Programmed Death Ligand 1 Antibody. J Clin Oncol 2019;37:2730-7. [Crossref] [PubMed]
- Freeman-Keller M, Kim Y, Cronin H, et al. Nivolumab in Resected and Unresectable Metastatic Melanoma: Characteristics of Immune-Related Adverse Events and Association with Outcomes. Clin Cancer Res 2016;22:886-94. [Crossref] [PubMed]
- Wang P, Tan G, Zhu M, et al. Hand-foot skin reaction is a beneficial indicator of sorafenib therapy for patients with hepatocellular carcinoma: a systemic review and meta-analysis. Expert Rev Gastroenterol Hepatol 2018;12:1-8. [Crossref] [PubMed]
- Coffelt SB, Wellenstein MD, de Visser KE. Neutrophils in cancer: neutral no more. Nat Rev Cancer 2016;16:431-46. [Crossref] [PubMed]
- Teramukai S, Kitano T, Kishida Y, et al. Pretreatment neutrophil count as an independent prognostic factor in advanced non-small-cell lung cancer: an analysis of Japan Multinational Trial Organisation LC00-03. Eur J Cancer 2009;45:1950-8. [Crossref] [PubMed]
- Nielsen SR, Strøbech JE, Horton ER, et al. Suppression of tumor-associated neutrophils by lorlatinib attenuates pancreatic cancer growth and improves treatment with immune checkpoint blockade. Nat Commun 2021;12:3414. [Crossref] [PubMed]
- Zhang L, Yao J, Wei Y, et al. Blocking immunosuppressive neutrophils deters pY696-EZH2-driven brain metastases. Sci Transl Med 2020;12:eaaz5387. [Crossref] [PubMed]
- Rodriguez-Iturbe B, Pons H, Johnson RJ. Role of the Immune System in Hypertension. Physiol Rev 2017;97:1127-64. [Crossref] [PubMed]
- Lee CH, Giuliani F. The Role of Inflammation in Depression and Fatigue. Front Immunol 2019;10:1696. [Crossref] [PubMed]
- Okadome K, Baba Y, Yagi T, et al. Prognostic Nutritional Index, Tumor-infiltrating Lymphocytes, and Prognosis in Patients with Esophageal Cancer. Ann Surg 2020;271:693-700. [Crossref] [PubMed]
- Xishan Z, Ye Z, Feiyan M, et al. The role of prognostic nutritional index for clinical outcomes of gastric cancer after total gastrectomy. Sci Rep 2020;10:17373. [Crossref] [PubMed]
- Hu Z, Li Y, Mao W, et al. Impact of Nutritional Indices on the Survival Outcomes of Patients with Colorectal Cancer. Cancer Manag Res 2020;12:2279-89. [Crossref] [PubMed]
- Muhammed A, Fulgenzi CAM, Dharmapuri S, et al. The Systemic Inflammatory Response Identifies Patients with Adverse Clinical Outcome from Immunotherapy in Hepatocellular Carcinoma. Cancers (Basel) 2021;14:186. [Crossref] [PubMed]
- Ji F, Liang Y, Fu S, et al. Prognostic value of combined preoperative prognostic nutritional index and body mass index in HCC after hepatectomy. HPB (Oxford) 2017;19:695-705. [Crossref] [PubMed]
- Zhao S, Peralta RM, Avina-Ochoa N, et al. Metabolic regulation of T cells in the tumor microenvironment by nutrient availability and diet. Semin Immunol 2021;52:101485. [Crossref] [PubMed]
- Kedia-Mehta N, Finlay DK. Competition for nutrients and its role in controlling immune responses. Nat Commun 2019;10:2123. [Crossref] [PubMed]
- Kitahara H, Shoji F, Akamine T, et al. Preoperative prognostic nutritional index level is associated with tumour-infiltrating lymphocyte status in patients with surgically resected lung squamous cell carcinoma. Eur J Cardiothorac Surg 2021;60:393-401. [Crossref] [PubMed]
- Johannet P, Sawyers A, Qian Y, et al. Baseline prognostic nutritional index and changes in pretreatment body mass index associate with immunotherapy response in patients with advanced cancer. J Immunother Cancer 2020;8:e001674. [Crossref] [PubMed]
- Kim SI, Cassella CR, Byrne KT. Tumor Burden and Immunotherapy: Impact on Immune Infiltration and Therapeutic Outcomes. Front Immunol 2020;11:629722. [Crossref] [PubMed]