Identification of candidate biomarkers and mechanisms in foam cell formation from heterogeneous cellular origins via integrated transcriptome analysis
Highlight box
Key findings
• We identified several multicellular biomarkers and candidate pathways that might participate in initial atherogenic events.
What is known and what is new?
• Foam cells play a crucial role in atherosclerotic lesion development, and macrophages and vascular smooth muscle cells (VSMCs) appear to contribute to the formation of the majority of atheromatous foam cells via oxidized low-density lipoprotein (ox-LDL) uptake.
• We elucidated the comprehensive landscape of the transcriptional regulations in macrophages and VSMCs under ox-LDL treatment.
What is the implication, and what should change now?
• Our findings show considerable promise for the emerging capability to ascertain corresponding mechanisms and offer projections on developing new approaches for potential therapeutic modulation in cardiological practice.
Introduction
Atherosclerotic cardiovascular disease (ASCVD) is the leading cause of mortality and morbidity worldwide, accounting for approximately 17.6 million deaths annually (1). The role of low-density lipoprotein cholesterol (LDL-C) in the development of ASCVD is at the forefront of current research, and both observational and epidemiological studies consistently demonstrate that individuals with low plasma levels of LDL-C have lower rates of ASCVD throughout the course of life (2-4). Thus, LDL-C is the key target of therapy for primary and secondary prevention, which is in accordance with the recommendations of international guidelines (5,6). However, despite appropriate lifestyle modification and the administration of lipid-lowering drugs, patients remain at a substantial residual risk for ASCVD recurrence (7,8), and much work is still required if we are to reach the aspirational vision of ASCVD prevention at the initial phase of disease.
Evidence from many decades of research supports the concept of oxidized-LDL (ox-LDL) accumulation as one of the essential processes responsible for atherogenesis and foam cell formation within the lipid-rich subendothelial space of the affected artery (9). Additionally, recent studies suggest that monocyte-derived macrophages and vascular smooth muscle cells (VSMCs) contribute to the formation of the majority of atheromatous foam cells via ox-LDL uptake (10,11). Both cell types express scavenger receptors responsible for taking up different forms of modified lipoprotein as well as regulating several inflammatory responses (12,13). Thus, studying the potential mechanisms of foam cell formation during the initial stage of atherogenesis may uncover the significant cellular processes at play and help to discern the relative contributions of heterogeneous foam cell populations with shared functions. In turn, these findings may contribute extensively to realizing the future development of foam cell-specific treatment.
Since its rapid development and adoption, transcriptome analysis has become a productive approach to unravelling the dynamic expression of genes and holistically elucidating the relevant cell physiology and molecular mechanisms (14,15). In this study, we conducted a series of microarray data bioinformatics reanalyses and used a machine-learning algorithm to investigate the distinctive and shared gene expression variations in human macrophages and VSMCs treated with ox-LDL. The results were validated using an external dataset, and they may contribute to a better understanding of the pathophysiological mechanisms of foam cell formation and drive new therapeutics in reducing ASCVD. We present the following article in accordance with the STREGA reporting checklist (available at https://atm.amegroups.com/article/view/10.21037/atm-22-3761/rc).
Methods
Microarray datasets collection
In the discovery phase, the gene expression datasets GSE54666 and GSE68021 were downloaded from the Gene Expression Omnibus (GEO) database (https://www.ncbi.nlm.nih.gov/geo/), which serves as a public data archive providing access to high-throughput gene expression and genomics datasets submitted by the research community (16). The series GSE54666, tested on the Illumina HumanHT-12 v. 4.0 Expression BeadChip platform (San Diego, CA, USA), contains gene expression profiles of human monocyte-derived macrophages treated with ox-LDL (50 µg/mL ox-LDL for 48 h) or control buffer (17). The series GSE68021, tested on the Affymetrix Human Gene 1.0 ST Array platform (Santa Clara, CA, USA), consists of expression data of human VSMCs in response to ox-LDL treatment (7.5 µg/mL ox-LDL for 1, 5, and 24 h) (18). The normalized data were acquired and reanalyzed with a systematic bioinformatics approach to identifying the differentially expressed genes (DEGs) and the downstream functional annotations. The overview of the analytic procedure is shown in Figure 1. The study was conducted in accordance with the Declaration of Helsinki (as revised in 2013).
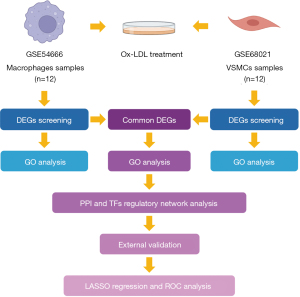
Data preprocessing and DEG screening
The reference matrix files were downloaded from the platforms, and the official gene symbols were matched with the gene probes. For a situation in which multiple probes matched a single gene symbol, the average gene expression value was retained. The following procedures were analyzed according to the processed matrix file. The limma package (linear models for microarray data; version 3.40.6) in R (The R Foundation for Statistical Computing) was used to screen DEGs between early and advanced phases of atherosclerosis (9). The fold change (FC) of logarithmic operations with 2 as the base number was calculated for the expression of each DEG in the ox-LDL–treated and control samples, and the statistical significance was defined by an adjusted P value <0.05 corrected by the Benjamini-Hochberg method. The results of probe-matching and DEG identification were also verified via GEO2R (19). For GSE68021, the human VSMCs used in this study were not terminally differentiated and, therefore, were sufficiently plastic to present a contractile phenotype after physiological stimulation (20). To retain as many samples as possible, the samples of VSMCs under ox-LDL treatment at each time point (1, 5, 24 h) were taken as a whole group in order to compare them with the negative control. The overlap of DEGs in the 2 datasets were identified and visualized by Venn diagrams. The top 5 upregulated and downregulated overlapped DEGs were selected according to the sum values of FC in GSE54666 and GSE68021.
Gene Ontology enrichment analysis
The Database for Annotation, Visualization and Integrated Discovery (DAVID; https://david.ncifcrf.gov) was used to investigate Gene Ontology (GO) enrichment, including the categories of biological process (BP), cellular component (CC), molecular function (MF), and the Kyoto Encyclopedia of Genes and Genomes (KEGG) based on the top 20 upregulated and downregulated DEGs in each dataset (21). The GO annotation for the overlapped DEGs of the 2 datasets was conducted and visualized with the ClueGO v. 2.5.8 and CluePedia v. 1.5.8 tool kits (22,23), which can interpret functionally grouped GO annotation networks and extract representative terms using a hypergeometric test via Cytoscape software (version 3.9.1). A P value <0.05 was considered statistically significant.
Protein interactions and the transcriptional factor network of overlapped DEGs
The Search Tool for the Retrieval of Interacting Genes v. 11.5 (STRING; http://string-db.org) online database was used to construct the protein-protein interaction (PPI) network of the overlapped DEGs (24), and interactions with a combined score of more than 0.15 were included. Then, the PPI network was displayed using Cytoscape software. The Molecular Complex Detection (MCODE) Cytoscape plugin (v. 2.0.0), an automated kit that can find densely connected regions or molecular complexes based on the topology of the PPI network, was used to screen the gene clusters according to the following parameters: degree cutoff, 2; node score cutoff of 0.2; max depth, 100; and k-score, 2. The Transcriptional Regulatory Relationships Unraveled by Sentence-based Text-mining (TRRUST) v. 2 database (www.grnpedia.org/trrust) was applied to predict the key regulators in transcriptional factor (TF)-gene interactions in order to assess the effect of the TFs on the expression and functional pathways of the top overlapped DEGs (25). The visualization of the regulatory network was presented via Cytoscape.
External validation of the overlapped DEGs
For the validation of the top 5 upregulated and downregulated overlapped DEGs, the series GSE9874 dataset was downloaded from the GEO database. It contains 15 samples of baseline macrophages and foam cells from individuals with atherosclerosis tested on the Affymetrix Human Genome U133A Array (26).
Selection of candidate biomarkers via least absolute shrinkage and selection operator Cox regression
The least absolute shrinkage and selection operator (LASSO) Cox regression analysis was conducted via the glmnet package in R software to calculate and select the linear models and preserve valuable DEGs (27). The expression levels of the overlapped DEGs and the cell types of the 15 samples were obtained from the probe-matched matrix file, and the 1 standard error of the minimum criterion (the 1-SE criterion) lambda value was applied to build the classification model with good performance but the least number of variables. The pROC package in R software was used for the calculation of the receiver operating characteristic (ROC) analysis, and the discriminatory power of the selected biomarkers correlated to foam cell formation was identified by the area under the curve (AUC) (28).
Statistical analysis
All calculations were performed via the R v. 4.1.2 software. The Euclidean genetic distance combining complete distance hierarchical cluster method was used for the cluster analysis based on Z score transformation. In external validation, the expressions of the DEGs were extracted from the microarray dataset and analyzed by paired-samples t-test. A P value <0.05 was defined as statistically significant.
Results
Identification of DEGs in response to ox-LDL treatment
To investigate the variations of gene expression in foam cell formation from different cellular origins, we conducted limma analysis of the dataset series, GSE54666 and GSE68021 (Figure 2; see https://cdn.amegroups.cn/static/public/atm-22-3761-1.xlsx for details). For monocyte-derived macrophages under the treatment of ox-LDL, a total of 504 DEGs were identified. The top 5 upregulated DEGs were AKR1C3, PLIN2, PDK4, TMEM158, and FABP4, while the top 5 downregulated DEGs were LDLR, F13A1, SQLE, INSIG1, and CD93 (Figure 2A). For VSMCs, a total of 4400 DEGs were identified. The top 5 upregulated DEGs were RGS7BP, RFTN2, CALCRL, OCLN, and HSD17B6, and the top 5 downregulated DEGs were ACTG2, BEX1, IFI30, TGM2, and TFPI2 in response to ox-LDL treatment (Figure 2C). These results indicated that at the transcriptomic level, both human macrophages and VSMCs could respond to ox-LDL exposure via altering gene expressions, and the different cell types show their own characteristic patterns of variation.
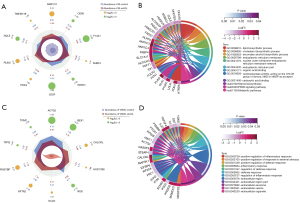
GO functional annotations in foam cell formation
Based on the previous step, the top 20 upregulated and downregulated DEGs identified in each dataset were subjected to GO enrichment analysis using the DAVID database (see https://cdn.amegroups.cn/static/public/atm-22-3761-1.xlsx and Tables S1 for details). As shown in Figure 2B, for macrophages, the predominant changes in the functional pathways of DEGs were significant enrichment of the lipid biosynthetic process (GO:0008610; P=1.15E-13), cholesterol biosynthetic process (GO:0006695; P=1.15E-13), and secondary alcohol biosynthetic process (GO:1902653; P=1.15E-13). Additionally, approximately half of the selected DEGs were annotated to participate in the lipid metabolic process (GO:0006629; gene count =19; P=2.45E-11; available online: https://cdn.amegroups.cn/static/public/atm-22-3761-1.xlsx). In the category of cellular component, the endoplasmic reticulum membrane (GO:0005789; P=9.82E-5) was annotated as the leading term, and 17 DEGs were matched to the extracellular region (GO:0005576; P=2.21E-2). For the annotation of molecular function, the following were found to be significant and potentially involved in the biosynthesis of esters and fatty acids: organic acid binding (GO:0043177; P=7.15E-6); oxidoreductase activity (GO:0016628) acting on the CH-CH group of donors, NAD, or NADP as the acceptor (P=2.65E-5); and carboxylic acid binding (GO:0031406; P=8.45E-5). Moreover, the KEGG pathways were also annotated as follows: steroid biosynthesis (hsa00100; P=2.85E-5), peroxisome proliferator-activated receptor (PPAR) signaling pathway (hsa03320; P=1.51E-3), and metabolic pathways (hsa01100; P=3.351E-2), which further highlighted the relevance of lipid-related metabolic pathways in the conversion of macrophages to foam cells.
In contrast to macrophages, VSMCs exhibited quite different functional pathway variations in response to ox-LDL treatment. As shown in Figure 2D, positive regulation of the inflammatory response (GO:0050729; P=2.62E-4), positive regulation of the response to external stimulus (GO:0032103; P=6.12E-4), and positive regulation of the defense response (GO:0031349; P=6.44E-4) were identified as dominant terms of the biological process, and all the significantly enriched terms of the cellular component were found to be related to the extracellular region, including the exosome, vesicle, and organelle. These results indicated that the initial responses of VSMCs under ox-LDL stimulus could be considered a series of functional defenses against atherogenesis, suggesting the immunoinflammatory nature of atherogenic pathophysiology.
The common DEGs in heterogeneous cellular origins related to foam cell formation
To investigate whether there were common transcriptional and functional variations in the conversion of foam cells from different cell types incubated with ox-LDL, we identified the overlapped DEGs in GSE54666 and GSE68021. Of all the DEGs, there were 34 upregulated (Figure 3A) and 26 downregulated (Figure 3B) DEGs overlapped in the 2 datasets (Table S2). Next, we investigated the functional enrichment of these overlapped DEGs, and despite the differences in the most significant pathways of heterogeneous cellular origins, as shown in Figure 3C, there were still several representative and common functional pathways, including cellular response to reactive oxygen species (GO:0034614; P=2.33E-3), regulation of lipid storage (GO:0010883; P=7.79E-3), long-chain fatty acid transport (GO:0015909; P=1.37E-2), integral component of the luminal side of the endoplasmic reticulum membrane (GO:0071556; P=2.26E-3), caveola (GO:0005901; P=1.69E-2), prostanoid metabolic process (GO:0006692; P=7.95E-3), and positive regulation of lymphocyte proliferation (GO:0050671; P=1.95E-3), according to the hypergeometric test conducted via ClueGo (see Figure S1 and Table S3 for details). To further narrow down the target DEGs, the top 5 upregulated and downregulated DEGs were selected as candidate biomarkers based on their FC in both datasets; the detailed descriptions are summarized in Table 1. The heatmaps of the selected DEGs in these 2 datasets showed accurate hierarchical clustering of altered transcriptions in various groups (Figure 3D,3E), facilitating the classification of cell status by collecting similar expression patterns. All these analyses revealed the characteristics and functions of common DEGs between the 2 cell types, which may become potential targets for interventions in the initial phase of the atherosclerotic process via both macrophages and VSMCs simultaneously.
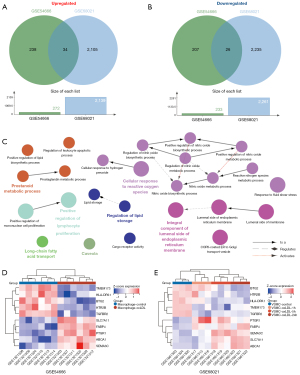
Table 1
Gene name | Description | GSE68021 | GSE54666 | |||
---|---|---|---|---|---|---|
Log2FC | Adjusted P value | Log2FC | Adjusted P value | |||
Upregulated | ||||||
SLC7A11 | Solute carrier family 7-member 11 | 0.9580 | 3.28E-2 | 1.4540 | 3.98E-3 | |
ABCA1 | ATP binding cassette subfamily A member 1 | 1.2683 | 4.00E-2 | 0.8465 | 5.51E-3 | |
FABP4 | Fatty acid binding protein 4 | 0.4687 | 2.39E-2 | 1.4704 | 2.03E-5 | |
PTGR1 | Prostaglandin reductase 1 | 0.4400 | 1.71E-3 | 1.2743 | 6.20E-6 | |
SEMA3C | Semaphorin 3C | 0.6273 | 2.78E-3 | 1.0839 | 1.44E-2 | |
Downregulated | ||||||
HTR2B | 5-hydroxytryptamine (serotonin) receptor 2B | −1.3784 | 4.79E-3 | −1.4981 | 9.80E-3 | |
TMEM173 | Transmembrane protein 173 | −0.6550 | 1.18E-3 | −1.3948 | 7.03E-4 | |
HLA-DPA1 | Major histocompatibility complex, class II, DP alpha 1 | −0.8343 | 1.60E-3 | −0.8223 | 3.10E-2 | |
TGFBR2 | Transforming growth factor beta receptor 2 | −0.9837 | 1.68E-5 | −0.3800 | 5.24E-3 | |
BTG2 | B-cell translocation gene 2, anti-proliferation gene family | −0.6687 | 5.63E-3 | −0.6412 | 2.61E-2 |
Description of the genes were obtained from Human Genome Resources at NCBI. The log2FC and adjusted P value were calculated via a comparison of the ox-LDL treatment group with controls in the 2 datasets. DEG, differentially expressed gene; NCBI, National Center for Biotechnology Information; ox-LDL, oxidized low-density lipoprotein; FC, fold change.
PPI and TF regulatory network analysis
To comprehensively analyze the molecular mechanisms and functional versatility of the common DEGs in foam cell formation, we constructed the PPI network based on the topological features acquired from the STRING database, and a total of 55 nodes and 107 edges were filtered into the PPI network (Figure 4A). According to the criterion of node degree >5, the following 12 DEGs were identified to have strong connectivity: ABCA1 (degree =12), CD74 (degree =11), ANXA1 (degree =10), TGFBR2 (degree =9), SGK1 (degree =9), KLF2 (degree =8), FABP4 (degree =8), BTG2 (degree =8), SLC7A11 (degree =6), UTRN (degree =6), IGBP1 (degree =6), and CTSC (degree =6). Five of these were also the candidate biomarkers selected in the previous step with distinct FC expression. The plug-in kit, MCODE, was used to analyze the significant clusters. As shown in Figure 4A, cluster 1 (shown in green) had 4 nodes and 5 edges, and both cluster 2 (shown in yellow) and cluster 3 (shown in purple) had 3 nodes and 3 edges, representing the prominent subnetworks of closely connected DEGs.
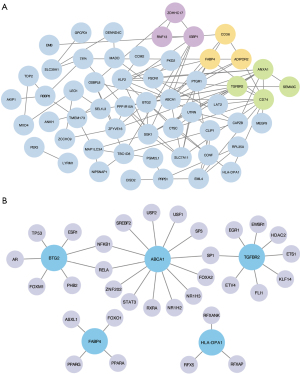
For the 10 candidate biomarkers we identified, a gene-TF regulatory network was constructed, including 5 genes and 32 TFs (Figure 4B). Specifically, 3 key TFs, nuclear factor kappa B subunit 1 (NFKB1), RELA proto-oncogene, NF-KB subunit (RELA), and Sp1 transcription factor (SP1) were identified with close interactions, having more than 1 DEG from the gene-TF regulatory network. NFKB1 and RELA, which could regulate BTG2 and ABCA1, and SP1, which could regulate ABCA1 and TGFBR2, could potentially be underlying targets for the prevention of atherosclerosis via gene transcription regulation.
External validation of the candidate biomarkers in foam cell formation
The above-mentioned series of bioinformatics analyses screened for potential biomarkers and the associated molecular mechanisms used in the conversion of foam cells from human macrophages and VSMCs under an ox-LDL stimulus. To verify the reliability of these biomarkers in atherogenesis, an external cohort, the GSE9874, which contains 15 samples of baseline macrophages and foam cells from patients with atherosclerosis, was included. The expression values of the candidate biomarkers were extracted and analyzed independently. As shown in Figure 5A, 8 (n=8) out of the 10 (n=10) selected biomarkers were detected and matched in GSE9874. Consistent with the previous results, these biomarkers all exhibited a similar trend of variation, particularly SLC7A11 (P<0.0001), ABCA1 (P<0.0001), FABP4 (P<0.05), and SEMA3C (P<0.05), which were significantly upregulated, while BTG2 (P<0.0001) was significantly downregulated in foam cell formation under the stimulus of ox-LDL.
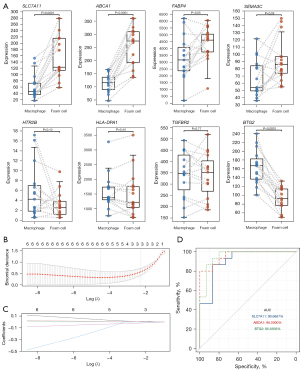
To further filter for the optimal biomarkers, the LASSO regression model for the 8 matched biomarkers from GSE9874 was conducted to investigate an optimal linear combination in the prediction of foam cell formation (Figure 5B,5C). With coefficients −0.0192, 0.0086, and 0.0076 for BTG2, ABCA1, and SLC7A11, respectively, the model could perfectly discriminate the samples from macrophages and foam cells. We further conducted an ROC analysis to investigate the feasibility of BTG2, ABCA1, and SLC7A11 as independent variables, and the results showed good predictive accuracy (Figure 5D). For SLC7A11, the AUC was 90.67% with a 95% confidence interval (CI) of 1.00–0.80; for ABCA1, the AUC was 96.00%, with a 95% CI of 1.00–0.90; and for BTG2, the AUC was 95.56%, with a 95% CI of 1.00–0.89.
Together, the expression levels of SLC7A11, ABCA1, FABP4, SEMA3C, and BTG2 were validated in an external cohort which further demonstrated their importance in foam cell formation, suggesting their feasibility as potential biomarkers for exploring molecular mechanisms and targets for intervention.
Discussion
It has long been demonstrated that atherogenesis is a complicated process involving numerous cellular mechanisms and pathways in which foam cells are pivotal in the formation of atherosclerosis and the lethal clinical consequences, including myocardial infarction or stroke (9). A previous misconception considered that all foam cells of atherosclerotic plaques are derived exclusively from macrophages, while recent studies have clearly indicated that VSMCs and macrophages are prominent sources of foam cells and resident in the arterial wall (29,30). Correspondingly, the pathophysiology of atherosclerosis remains a promising scientific topic, and the development of novel antiatherosclerosis therapies continues. The discovery of new genomic paradigms in cardiovascular diseases has been accelerated by the application of recent advances in high-throughput sequencing and computational bioinformatics. These technologies are having a tremendous impact on state-of-the-art diagnostics, biomarkers, and medications in the era of precision medicine, aiming to comprehensively measure and quantify such holistic biological information in a fast and cost-effective manner (31,32). Microarray is one of the transcriptome measurements based on the specific hybridization of RNA transcripts to DNA probes, and it can broadly reflect the functional state of a cell or tissue via screening of its gene expression pattern, providing valuable insights into the molecular targets and pathways controlled by transcriptional regulators (33). In the present study, we comprehensively performed a series of bioinformatics analyses to investigate and compare the DEGs and their related biological pathways in both human macrophages and VSMCs under the treatment of ox-LDL. We also applied machine learning to statistically evaluate and select the candidate biomarkers, which we further validated in an external cohort. The results may provide relevant transcriptional signatures and potential therapeutic targets to inhibit the development of atherosclerosis.
In order to capture the variations in cellular transcript levels in response to ox-LDL treatment, from a holistic perspective, we first analyzed the DEGs in VSMC-derived and macrophage-derived foam cells separately. As expected, the transcriptome exerted significant differences in different cell types (Figure 2) as apparent from the fact that expression responses to exogenous exposure were often highly cell-specific, with an examples being the differences in expression of molecular targets (34). Furthermore, the annotated functional pathways in the 2 cell types based on leading DEGs also showed distinct discrepancies. For macrophages, the predominant variations in functional pathways were mainly enriched in terms related to lipid metabolism. Macrophages are capable of recognizing and binding to ox-LDL for uptake, esterification, and storage in the cell, and excessive intracellular lipid accumulation results in the formation of foam cells, thus exerting proatherogenic functions, leading to a greater likelihood of plaque rupture and, consequently, cardiovascular accidents (11,35). In addition, the cell component annotation showed terms associated with the endoplasmic reticulum membrane, where cytoplasmic lipid droplets containing cholesterol esters can be generated after the re-esterification of free cholesterol by the enzyme acyl cholesterol transferase 1 (ACAT1) (36). Notably, the classic PPAR signaling pathway was also annotated in our results. The activation of PPAR pathways was found to be an important regulator of lipid uptake and efflux in macrophages, potentially promoting cholesterol efflux and reducing foam cell formation (37). Moreover, PPAR can also regulate immunoinflammatory processes, with several animal atherosclerosis models and human clinical trials suggesting the suppression of proinflammatory cytokines via the application of PPAR agonists (38,39), making it a highly promising antiatherogenic target. Interestingly, we found that several annotated pathways were related to human 3α-hydroxysteroid dehydrogenase (3α-HSD) isoforms, members of the aldo-keto reductase (AKR) enzyme superfamily, especially the type 1 3α-HSD (AKR1C4) and the type 2 3α(17β)-HSD (AKR1C3). A previous study based on chromatin immunoprecipitation (ChIP)/microarray technology identified that AKR1C4 was involved in cholesterol degradation modulated by oxysterols (40). Our study further suggested the putative roles of these DEGs in the formation of foam cells from macrophages incubated with ox-LDL.
In contrast to that in macrophages, the annotated biological process based on the DEGs in VSMCs showed tight regulation of immunoinflammatory responses, with significantly overexpressed characteristic cytokines, such as interleukin-33 (IL-33) and tumor necrosis factor superfamily member 4 (TNFSF4), as well as complement C3, the central component of the complement system. A growing body of evidence supports the conclusion that IL-33 triggers proinflammatory, proatherogenic, and proangiogenic effects, all processes known to be involved in cardiovascular diseases (41,42). In humans, increased expression of IL-33 has been observed in VSMCs of atherosclerotic coronary artery sections compared to arteries without lesions (43). Importantly, IL-33 may also serve a protective role in cell preservation and repair in response to mechanical stress (44). The expression of TNFSF4 has been shown to be susceptible risk factor for the pathogenesis of atherosclerosis, with several genetic studies indicating its variation and polymorphisms to be associated with atherothrombosis incidence (45,46). One study demonstrated that interruption of the TNFSF4-related pathway could attenuate atherogenesis and increase the levels of anti–ox-LDL immunoglobulin M in LDL receptor-deficient mice (47). The complement system is a crucial component of innate immunity that participates in the regulation of inflammation. In our results, complement C3 dominated the GO annotation; however, its expression level decreased in response to ox-LDL treatment. This was in line with a published study in mice models of atherosclerosis (ApoE−/− and Ldlr−/− background) and knock-out C3 expression (C3−/−) which indicated that the C3-deficient mice had a more proatherogenic lipoprotein profile and a larger lesion size of atherosclerotic plaque (48). Accordingly, from our results, it is reasonable to propose that these versatile transcriptomic responses triggered by the presence of ox-LDL could generate a series of inflammatory processes through communication with the immune system, suggesting that the initial response of human VSMCs towards a proatherogenic stimulus could be considered a functional defense process (49).
Based on the previous results, we further attempted to identify the crosstalk and the compensatory mechanisms between the macrophages and VSMCs responsible for regulating ox-LDL metabolism, highlighting the potentiality of targeting more than one process at a time to show greater efficacy at slowing down the rate of foam cell formation. We investigated the covariant DEGs in macrophages and VSMCs, and due to the heterogeneity of cellular origins, only a minority of the DEGs were overlapped. Although, as mentioned above, there were notable divergences in the main functional pathways of the 2 cell types, we nonetheless found some co-occurring biological processes in response to ox-LDL stimulation. The most representative pathways were cellular responses to reactive oxygen species (ROS), which accounted for about 40% of the annotations, with upregulation of the membrane receptor cluster of differentiation 36 (CD36), which was also one of the cluster genes in the PPI network. The presence of ox-LDL is capable of inducing ROS production, and the oxidative stress triggered by ROS further stimulates CD36 expression on the surface of various cells, which in turn recognizes ox-LDL and mediates its uptake into cells, thus forming a feedback loop that figures centrally in atherogenesis (50,51). Additionally, the ox-LDL-CD36 signaling axis has been found to be necessary for macrophage foam cell formation (52). In our results, the co-upregulation of adenosine triphosphate (ATP) binding cassette transporter A1 (ABCA1) and annexin A1 (ANXA1) was found to be significant, as were the pathways related to lipid storage and transport. ABCA1 promotes cholesterol efflux and reduces cholesterol accumulation in cells (53), and recent studies have also suggested that it could reduce inflammatory responses via the removal of ROS (54). Interestingly, ABCA1 mediates ANXA1 release, the effect of which could also exert anti-inflammatory actions to inhibit the development of atherosclerosis (55). However, the direct mechanisms of ANXA1 and the ABCA1-ANXA1 interaction in atheroprogression have not been investigated in any detail. We also noticed an association between ANXA1 expression and prostanoid metabolic process pathways. The biosynthesis of prostaglandins was strongly induced in macrophages stimulated with ox-LDL, indicating an anti-inflammatory response to ox-LDL-induced injury for alleviating cytotoxicity (56).
We then targeted the screening of statistically significant covariants in macrophages and VSMCs based on the FCs in both datasets. To further validate the reliability of these candidate biomarkers, we examined their expression levels in an external dataset, applied a machine-learning algorithm of LASSO regression to select the biomarkers with decent performances in discriminating the samples with different statuses, and finally filtered the cystine/glutamate antiporter solute carrier family 7 member 11 (SLC7A11), ABCA1, and B-cell translocation gene 2 (BTG2) as candidate biomarkers. SLC7A11 encodes a multipass transmembrane protein that mediates cystine/glutamate antiporter activity, which was recently discovered to be associated with ferroptosis in cancer cells (57). Ferroptosis is a newly discovered form of iron-dependent cell death induced by the accumulation of lipid peroxides and the production of ROS that overwhelm the balance between free radical formation and depletion in the cellular membrane (58). An increasing amount of research supports the notion that iron-induced oxidative stress is involved in various pathological conditions of cardiovascular diseases (59-61), but the mechanisms involved have not been well characterized. BTG2 is a well-known tumor suppressor and has found to be downregulated in several types of cancer. Furthermore, the knockdown of BTG2 expression was shown to enhance lipid accumulation and upregulate the expression of adipogenic marker genes (62), yet there is no direct evidence of its association with atherosclerosis. Through the study and further in-depth exploration of the ferroptosis and BTG2-related cellular pathways involved in foam cell formation, future therapies could potentially target these pathways and provide an additional therapeutic route.
Our study had several limitations. First, the samples included in this study were from different datasets, which might have induced interexperiment variabilities, such as different experimental procedures and different array platforms from different laboratories. To minimize the impact of these confounding factors on the results, rather than simply merging the datasets, we analyzed them separately and independently after rigorous data testing before implementing the intersection and performing external validation. Additionally, the information acquired from the GO databases will need to be revised if the databases update the latest annotations. Moreover, the results of this study are preliminary, and further biological proof-of-concept studies are required to verify the potential mechanisms. Nevertheless, our study has the merit of providing a comprehensive summary of the landscape of transcriptional regulations in macrophages and VSMCs under ox-LDL treatment from a bioinformatics respective and of suggesting several multicellular biomarkers and candidate pathways that might participate in the initial atherogenic events. There is considerable promise then for the emerging capability to ascertain corresponding mechanisms and offer insights into developing new approaches for potential therapeutic modulation in cardiological practice.
Conclusions
Our study provides a comprehensive summary of the landscape of the transcriptional regulations in macrophages and VSMCs under ox-LDL treatment from a bioinformatics perspective, which may contribute to a better understanding of the pathophysiological mechanisms of foam cell formation.
Acknowledgments
We thank all the authors of the mentioned datasets for sharing the dataset on NCBI Gene Expression Omnibus.
Funding: This work was supported by the Chinese Academy of Medical Sciences (CAMS) Innovation Fund for Medical Sciences (CIFMS, No. 2016-I2M-1-009).
Footnote
Reporting Checklist: The authors have completed the STREGA reporting checklist. Available at https://atm.amegroups.com/article/view/10.21037/atm-22-3761/rc
Peer Review File: Available at https://atm.amegroups.com/article/view/10.21037/atm-22-3761/prf
Conflicts of Interest: Both authors have completed the ICMJE uniform disclosure form (available at https://atm.amegroups.com/article/view/10.21037/atm-22-3761/coif). The authors have no conflicts of interest to declare.
Ethical Statement: The authors are accountable for all aspects of the work in ensuring that questions related to the accuracy or integrity of any part of the work are appropriately investigated and resolved. The study was conducted in accordance with the Declaration of Helsinki (as revised in 2013).
Open Access Statement: This is an Open Access article distributed in accordance with the Creative Commons Attribution-NonCommercial-NoDerivs 4.0 International License (CC BY-NC-ND 4.0), which permits the non-commercial replication and distribution of the article with the strict proviso that no changes or edits are made and the original work is properly cited (including links to both the formal publication through the relevant DOI and the license). See: https://creativecommons.org/licenses/by-nc-nd/4.0/.
References
- Virani SS, Alonso A, Benjamin EJ, et al. Heart Disease and Stroke Statistics-2020 Update: A Report From the American Heart Association. Circulation 2020;141:e139-596. [Crossref] [PubMed]
- Prospective Studies Collaboration. Blood cholesterol and vascular mortality by age, sex, and blood pressure: a meta-analysis of individual data from 61 prospective studies with 55,000 vascular deaths. Lancet 2007;370:1829-39. [Crossref] [PubMed]
- Ference BA, Yoo W, Alesh I, et al. Effect of long-term exposure to lower low-density lipoprotein cholesterol beginning early in life on the risk of coronary heart disease: a Mendelian randomization analysis. J Am Coll Cardiol 2012;60:2631-9. [Crossref] [PubMed]
- Holmes MV, Asselbergs FW, Palmer TM, et al. Mendelian randomization of blood lipids for coronary heart disease. Eur Heart J 2015;36:539-50. [Crossref] [PubMed]
- Grundy SM, Stone NJ, Bailey AL, et al. 2018 AHA/ACC/AACVPR/AAPA/ABC/ACPM/ADA/AGS/APhA/ASPC/NLA/PCNA Guideline on the Management of Blood Cholesterol: A Report of the American College of Cardiology/American Heart Association Task Force on Clinical Practice Guidelines. Circulation 2019;139:e1082-143. [PubMed]
- Mach F, Baigent C, Catapano AL, et al. 2019 ESC/EAS Guidelines for the management of dyslipidaemias: lipid modification to reduce cardiovascular risk. Eur Heart J 2020;41:111-88. [Crossref] [PubMed]
- Sandesara PB, Virani SS, Fazio S, et al. The Forgotten Lipids: Triglycerides, Remnant Cholesterol, and Atherosclerotic Cardiovascular Disease Risk. Endocr Rev 2019;40:537-57. [Crossref] [PubMed]
- Farnier M, Zeller M, Masson D, et al. Triglycerides and risk of atherosclerotic cardiovascular disease: An update. Arch Cardiovasc Dis 2021;114:132-9. [Crossref] [PubMed]
- Libby P, Buring JE, Badimon L, et al. Atherosclerosis. Nat Rev Dis Primers 2019;5:56. [Crossref] [PubMed]
- Allahverdian S, Chehroudi AC, McManus BM, et al. Contribution of intimal smooth muscle cells to cholesterol accumulation and macrophage-like cells in human atherosclerosis. Circulation 2014;129:1551-9. [Crossref] [PubMed]
- Maguire EM, Pearce SWA, Xiao Q. Foam cell formation: A new target for fighting atherosclerosis and cardiovascular disease. Vascul Pharmacol 2019;112:54-71. [Crossref] [PubMed]
- Beyea MM, Reaume S, Sawyez CG, et al. The oxysterol 24(s),25-epoxycholesterol attenuates human smooth muscle-derived foam cell formation via reduced low-density lipoprotein uptake and enhanced cholesterol efflux. J Am Heart Assoc 2012;1:e000810. [Crossref] [PubMed]
- Dai XY, Cai Y, Mao DD, et al. Increased stability of phosphatase and tensin homolog by intermedin leading to scavenger receptor A inhibition of macrophages reduces atherosclerosis in apolipoprotein E-deficient mice. J Mol Cell Cardiol 2012;53:509-20. [Crossref] [PubMed]
- de Jong TV, Moshkin YM, Guryev V. Gene expression variability: the other dimension in transcriptome analysis. Physiol Genomics 2019;51:145-58. [Crossref] [PubMed]
- Segundo-Val IS, Sanz-Lozano CS. Introduction to the Gene Expression Analysis. Methods Mol Biol 2016;1434:29-43. [Crossref] [PubMed]
- Clough E, Barrett T. The Gene Expression Omnibus Database. Methods Mol Biol 2016;1418:93-110. [Crossref] [PubMed]
- Reschen ME, Gaulton KJ, Lin D, et al. Lipid-induced epigenomic changes in human macrophages identify a coronary artery disease-associated variant that regulates PPAP2B Expression through Altered C/EBP-beta binding. PLoS Genet 2015;11:e1005061. [Crossref] [PubMed]
- Damián-Zamacona S, Toledo-Ibelles P, Ibarra-Abundis MZ, et al. Early Transcriptomic Response to LDL and oxLDL in Human Vascular Smooth Muscle Cells. PLoS One 2016;11:e0163924. [Crossref] [PubMed]
- Barrett T, Wilhite SE, Ledoux P, et al. NCBI GEO: archive for functional genomics data sets--update. Nucleic Acids Res 2013;41:D991-5. [Crossref] [PubMed]
- Chistiakov DA, Orekhov AN, Bobryshev YV. Vascular smooth muscle cell in atherosclerosis. Acta Physiol (Oxf) 2015;214:33-50. [Crossref] [PubMed]
- Sherman BT, Hao M, Qiu J, et al. DAVID: a web server for functional enrichment analysis and functional annotation of gene lists (2021 update). Nucleic Acids Res 2022; Epub ahead of print. [Crossref] [PubMed]
- Bindea G, Galon J, Mlecnik B. CluePedia Cytoscape plugin: pathway insights using integrated experimental and in silico data. Bioinformatics 2013;29:661-3. [Crossref] [PubMed]
- Bindea G, Mlecnik B, Hackl H, et al. ClueGO: a Cytoscape plug-in to decipher functionally grouped gene ontology and pathway annotation networks. Bioinformatics 2009;25:1091-3. [Crossref] [PubMed]
- Szklarczyk D, Gable AL, Nastou KC, et al. The STRING database in 2021: customizable protein-protein networks, and functional characterization of user-uploaded gene/measurement sets. Nucleic Acids Res 2021;49:D605-12. [Crossref] [PubMed]
- Han H, Cho JW, Lee S, et al. TRRUST v2: an expanded reference database of human and mouse transcriptional regulatory interactions. Nucleic Acids Res 2018;46:D380-6. [Crossref] [PubMed]
- Hägg DA, Jernås M, Wiklund O, et al. Expression profiling of macrophages from subjects with atherosclerosis to identify novel susceptibility genes. Int J Mol Med 2008;21:697-704. [Crossref] [PubMed]
- Engebretsen S, Bohlin J. Statistical predictions with glmnet. Clin Epigenetics 2019;11:123. [Crossref] [PubMed]
- Robin X, Turck N, Hainard A, et al. pROC: an open-source package for R and S+ to analyze and compare ROC curves. BMC Bioinformatics 2011;12:77. [Crossref] [PubMed]
- Owsiany KM, Alencar GF, Owens GK. Revealing the Origins of Foam Cells in Atherosclerotic Lesions. Arterioscler Thromb Vasc Biol 2019;39:836-8. [Crossref] [PubMed]
- Allahverdian S, Chaabane C, Boukais K, et al. Smooth muscle cell fate and plasticity in atherosclerosis. Cardiovasc Res 2018;114:540-50. [Crossref] [PubMed]
- Dai H, Younis A, Kong JD, et al. Big Data in Cardiology: State-of-Art and Future Prospects. Front Cardiovasc Med 2022;9:844296. [Crossref] [PubMed]
- Oulas A, Minadakis G, Zachariou M, et al. Systems Bioinformatics: increasing precision of computational diagnostics and therapeutics through network-based approaches. Brief Bioinform 2019;20:806-24. [Crossref] [PubMed]
- Tebani A, Afonso C, Marret S, et al. Omics-Based Strategies in Precision Medicine: Toward a Paradigm Shift in Inborn Errors of Metabolism Investigations. Int J Mol Sci 2016;17:1555. [Crossref] [PubMed]
- Hodos R, Zhang P, Lee HC, et al. Cell-specific prediction and application of drug-induced gene expression profiles. Pac Symp Biocomput 2018;23:32-43. [Crossref] [PubMed]
- McLaren JE, Michael DR, Ashlin TG, et al. Cytokines, macrophage lipid metabolism and foam cells: implications for cardiovascular disease therapy. Prog Lipid Res 2011;50:331-47. [Crossref] [PubMed]
- Olzmann JA, Carvalho P. Dynamics and functions of lipid droplets. Nat Rev Mol Cell Biol 2019;20:137-55. [Crossref] [PubMed]
- Li AC, Binder CJ, Gutierrez A, et al. Differential inhibition of macrophage foam-cell formation and atherosclerosis in mice by PPARalpha, beta/delta, and gamma. J Clin Invest 2004;114:1564-76. [Crossref] [PubMed]
- Christofides A, Konstantinidou E, Jani C, et al. The role of peroxisome proliferator-activated receptors (PPAR) in immune responses. Metabolism 2021;114:154338. [Crossref] [PubMed]
- Barbier O, Torra IP, Duguay Y, et al. Pleiotropic actions of peroxisome proliferator-activated receptors in lipid metabolism and atherosclerosis. Arterioscler Thromb Vasc Biol 2002;22:717-26. [Crossref] [PubMed]
- Stayrook KR, Rogers PM, Savkur RS, et al. Regulation of human 3 alpha-hydroxysteroid dehydrogenase (AKR1C4) expression by the liver X receptor alpha. Mol Pharmacol 2008;73:607-12. [Crossref] [PubMed]
- Demyanets S, Stojkovic S, Huber K, et al. The Paradigm Change of IL-33 in Vascular Biology. Int J Mol Sci 2021;22:13288. [Crossref] [PubMed]
- Mantovani A, Dinarello CA, Molgora M, et al. Interleukin-1 and Related Cytokines in the Regulation of Inflammation and Immunity. Immunity 2019;50:778-95. [Crossref] [PubMed]
- Govatati S, Pichavaram P, Janjanam J, et al. NFATc1-E2F1-LMCD1-Mediated IL-33 Expression by Thrombin Is Required for Injury-Induced Neointima Formation. Arterioscler Thromb Vasc Biol 2019;39:1212-26. [Crossref] [PubMed]
- Altara R, Ghali R, Mallat Z, et al. Conflicting vascular and metabolic impact of the IL-33/sST2 axis. Cardiovasc Res 2018;114:1578-94. [Crossref] [PubMed]
- Wang X, Ria M, Kelmenson PM, et al. Positional identification of TNFSF4, encoding OX40 ligand, as a gene that influences atherosclerosis susceptibility. Nat Genet 2005;37:365-72. [Crossref] [PubMed]
- Mälarstig A, Eriksson P, Rose L, et al. Genetic variants of tumor necrosis factor superfamily, member 4 (TNFSF4), and risk of incident atherothrombosis and venous thromboembolism. Clin Chem 2008;54:833-40. [Crossref] [PubMed]
- van Wanrooij EJ, van Puijvelde GH, de Vos P, et al. Interruption of the Tnfrsf4/Tnfsf4 (OX40/OX40L) pathway attenuates atherogenesis in low-density lipoprotein receptor-deficient mice. Arterioscler Thromb Vasc Biol 2007;27:204-10. [Crossref] [PubMed]
- Persson L, Borén J, Robertson AK, et al. Lack of complement factor C3, but not factor B, increases hyperlipidemia and atherosclerosis in apolipoprotein E-/- low-density lipoprotein receptor-/- mice. Arterioscler Thromb Vasc Biol 2004;24:1062-7. [Crossref] [PubMed]
- Libby P. Inflammation during the life cycle of the atherosclerotic plaque. Cardiovasc Res 2021;117:2525-36. [Crossref] [PubMed]
- Kattoor AJ, Pothineni NVK, Palagiri D, et al. Oxidative Stress in Atherosclerosis. Curr Atheroscler Rep 2017;19:42. [Crossref] [PubMed]
- Cho K, Choi SH. ASK1 Mediates Apoptosis and Autophagy during oxLDL-CD36 Signaling in Senescent Endothelial Cells. Oxid Med Cell Longev 2019;2019:2840437. [Crossref] [PubMed]
- Rahaman SO, Lennon DJ, Febbraio M, et al. A CD36-dependent signaling cascade is necessary for macrophage foam cell formation. Cell Metab 2006;4:211-21. [Crossref] [PubMed]
- Wang D, Hiebl V, Xu T, et al. Impact of natural products on the cholesterol transporter ABCA1. J Ethnopharmacol 2020;249:112444. [Crossref] [PubMed]
- Babashamsi MM, Koukhaloo SZ, Halalkhor S, et al. ABCA1 and metabolic syndrome; a review of the ABCA1 role in HDL-VLDL production, insulin-glucose homeostasis, inflammation and obesity. Diabetes Metab Syndr 2019;13:1529-34. [Crossref] [PubMed]
- Shen X, Zhang S, Guo Z, et al. The crosstalk of ABCA1 and ANXA1: a potential mechanism for protection against atherosclerosis. Mol Med 2020;26:84. [Crossref] [PubMed]
- Lara-Guzmán OJ, Gil-Izquierdo Á, Medina S, et al. Oxidized LDL triggers changes in oxidative stress and inflammatory biomarkers in human macrophages. Redox Biol 2018;15:1-11. [Crossref] [PubMed]
- Dixon SJ, Lemberg KM, Lamprecht MR, et al. Ferroptosis: an iron-dependent form of nonapoptotic cell death. Cell 2012;149:1060-72. [Crossref] [PubMed]
- Stockwell BR, Jiang X. The Chemistry and Biology of Ferroptosis. Cell Chem Biol 2020;27:365-75. [Crossref] [PubMed]
- Kobayashi M, Suhara T, Baba Y, et al. Pathological Roles of Iron in Cardiovascular Disease. Curr Drug Targets 2018;19:1068-76. [Crossref] [PubMed]
- Gammella E, Recalcati S, Rybinska I, et al. Iron-induced damage in cardiomyopathy: oxidative-dependent and independent mechanisms. Oxid Med Cell Longev 2015;2015:230182. [Crossref] [PubMed]
- Yan F, Li K, Xing W, et al. Role of Iron-Related Oxidative Stress and Mitochondrial Dysfunction in Cardiovascular Diseases. Oxid Med Cell Longev 2022;2022:5124553. [Crossref] [PubMed]
- Kim S, Hong JW, Park KW. B cell translocation gene 2 (Btg2) is regulated by Stat3 signaling and inhibits adipocyte differentiation. Mol Cell Biochem 2016;413:145-53. [Crossref] [PubMed]