Predicting specific mortality from laryngeal cancer based on competing risk model: a retrospective analysis based on the SEER database
Highlight box
Key findings
• Laryngeal cancer patients whose primary site is the posterior pharyngeal wall and the piriform crypt have high short-term mortality rates.
What is known and what is new?
• The mortality of laryngeal cancer patients with keratinized squamous cell carcinoma (SCC) was higher than that of those with non-keratinized SCC.
• Patients with laryngeal cancer whose primary site was the posterior pharyngeal wall and the piriform crypt had high short-term mortality rates.
What is the implication, and what should change now?
• We used data from the SEER database to establish a competitive risk model to predict the short-term survival rates of laryngeal cancer patients. Compared to traditional risk prediction models, this model of specific death outcomes is more suitable for predicting disease-specific deaths.
Introduction
Head and neck tumors, which are mainly located in the mouth, pharynx, larynx, sinuses, nasal cavity, and salivary glands, are currently among the top 10 tumors in the world (1). Laryngeal carcinoma is one of the most common types of head and neck tumors (2). The main histological manifestation of laryngeal carcinoma is squamous cell carcinoma (SCC), which accounts for >95% of laryngeal cancers (3,4). The most common manifestations of laryngeal cancer are a sore throat, hoarseness, dysphagia, a laryngeal mass, and dyspnea (5). The pathogenesis of laryngeal cancer is relatively complex and related to many risk factors. Previous studies have shown that smoking and drinking are the main risk factors for laryngeal cancer, and the coordinated effect of the 2 factors is more than the risk of any single factor (3,6-8). For example, in South America, >60% of throat cancer patients are associated with smoking and drinking habits (6,9,10). In addition, recent studies have detected human papillomavirus (HPV) infection in approximately 20–30% of laryngeal cancer patients and may be associated with survival in laryngeal cancer patients (11-13).
Studies have also shown that laryngeal cancer is more common in men than women (7). One study estimated that by 2016, the incidence of laryngeal cancer would be 5.8 per 100,000 in males, and 1.2 cases per 100,000 in females (14). In addition, racial differences in laryngeal cancer are also very obvious; for example, African Americans appear to be of a younger age and have higher morbidity and mortality than whites (7). In recent decades, studies have shown that the mortality rate of laryngeal cancer has not decreased significantly worldwide, but the 5-year survival rate has decreased from 66% to 63% (7). However, the reasons for the decline in the survival rate remain unclear. In a retrospective study by Fujii et al. (15), it was found that the death rate of laryngeal cancer was correlated with the number of metastatic lymph nodes, but not with the primary tumor. In Dr. Roberto Padier’s study (16), a median human univariate analysis showed that the extent of laryngeal cancer, lymph node involvement, stage, and previous organostomy were associated with mortality in laryngeal cancer patients. Thus, more evidence is needed to evaluate both the short-term prognosis and survival rate of patients and to improve the long-term quality of life of laryngeal cancer patients, human life and health, social value and scientific developments.
At present, most tumor prognostic studies use the traditional Cox proportional hazards model. This model selects the survival events related to the study objectives through the analysis of univariate and multivariate Cox proportional models, and designs and constructs confidence intervals (CIs). However, when competing risks exist, traditional Cox regression models are not quite appropriate, as in such models, the competing events are deleted (17), which leads to the mortality rate for a given cancer being overestimated. For disease mortality prediction, cancer events and non-cancer events that lead to a patient’s eventual death should be distinguished. Competing hazards models (e.g., the Fine-Gray proportional hazards model) handles the relationship between cancer outcomes and competing events well. However, presently, there is no short-term competing risk model for laryngeal cancer patients.
Additionally, at each stage of disease progression or treatment, the quality of life of each patient is affected differently, including each patient’s psychology, happiness index, family harmony, and social identity. At present, there is still a lack of evidence on the short-term survival prediction of laryngeal cancer. This study screened univariate and multivariate factors to evaluate the risk factors related to the short-term death of laryngeal cancer patients to establish a competitive risk model for the death risk of laryngeal cancer patients that can be used to better evaluate the specific death risk and prognosis of patients. We present the following article in accordance with the TRIPOD reporting checklist (available at https://atm.amegroups.com/article/view/10.21037/atm-23-400/rc).
Methods
Data sources and study population
The data for this study were obtained from the Surveillance, Epidemiology and Final Results (SEER) database (https://seer.cancer.gov/). This public database, which was established in 1974, includes data on patients’ race, age, treatment method, final cause of death, and survival time (18). The study was conducted in accordance with the Declaration of Helsinki (as revised in 2013). In this study, we extracted data using SEER*stat (Version 4.6.1) software. We selected a dataset submitted in 2021 from the SEER database: “Incidence - SEER Research Plus Data, 17 Registries, Noy 2021 Sub (2000-2019)”. Later, we screened out patients whose primary tumor site was in the larynx by the variable—“Primary Site” in the SEER database. Patients were excluded if they had missing data for 1 or more variants, and patients with follow-up times of less than one month were excluded. The data collected for the laryngeal cancer patients included data on race, the laryngeal cancer primary site, the tumor histological type, the tumor size, the tumor number, the clinical T, N, M neoplasm staging classification for the tumor, lymph node involvement, treatment, including surgery, chemotherapy, and radiation therapy, the laryngeal cancer metastasis organ, survival time, the final cause of death, and family income.
After screening 3,805 laryngeal cancer patients, the patients were divided into groups based on a number of indicators. The patients were divided into the following 3 groups based on age: the <60-year-old group, the 60–80-year-old group, and the >80-year-old group. The patients were also divided into the following groups based on the histological type: the laryngeal carcinoma group, the SCC group, the keratinized SCC group, the non-keratinized SCC group, and the other histological types group. The patients were further divided into the following groups according to the primary site of laryngeal cancer: the piriform vaginal fossa group, the posterior hypopharyngeal wall group, the Aryepiglottic fold group, and the other primary sites group. In addition, the patients were divided into the following 3 groups according to the size of the tumor tissue: the >6-cm group, the 3–6-cm group, and the <3-cm group. These groups were established to explore the effect of each subtype of variable on the short-term survival rates of patients with laryngeal cancer.
Statistical analyses (competitive survival risk regression model)
The entire cohort was randomly split into a training cohort and a validation cohort at a 7:3 ratio. The training cohort was used in building the model while the validation cohort was used to validate the model. The demographic and clinical characteristics of the patients were depicted descriptively for the whole group, and separately for the training cohort and validation cohort. Chi-square tests were used to compare the categorical variables, and t-tests or rank-sum tests were used to compare the continuous variables. The follow-up time was defined as the time from laryngeal cancer diagnosis to death or the follow-up deadline. Consider non-laryngeal cancer death as a competing risk event. Within the training cohort, to evaluate the effect of various factors on the specific survival rates of patients with laryngeal cancer, we conducted univariate and multivariate Fine&Grey analyses for each variable using the risk Regression package in R software and determined the HR values and 95% CIs. The statistically significant variables were selected and included in the final competitive risk model, and the nomogram was drawn with the rms package. Receiver operating characteristic (ROC) and calibration curves were drawn to validate the Fine&Grey competing risk model. All the statistical analyses were performed using R 4.2.1. A 2-sided P value <0.05 was considered statistically significant.
Results
We first included 10,564 laryngeal cancer patients from the SEER database, excluded laryngeal cancer patients with missing data (n=6,163), and laryngeal cancer patients with follow-up times of less than one month (n=596). ultimately, 3,805 patients (2,264 in the training set and 1,141 in the validation set) were included in the analysis. Among all the patients, a total of 411 died of laryngeal cancer, among whom 288 were in the training set and 123 were in the validation set. In total, 1,290 patients survived, and 2,104 patients died of non-laryngeal cancer-specific deaths. More specifically, 903 and 387 patients survived, and 1,473 and 631 died of non-laryngeal cancer-specific deaths in the training and validation sets, respectively. The median survival time of all laryngeal cancer patients included in the study was 16 months, the median survival time of the laryngeal cancer patients in the training set was 16 months, and the median survival time of the laryngeal cancer patients in the validation set was 18 months.
By analyzing the data of the laryngeal cancer patients included in the study, we found that among the total number of patients, the majority of patients with laryngeal cancer were aged 60–80 years. We also found that the primary tumor location for 47% of the total number of patients was Wneoplasm staging. In the training set and validation set, 47% and 47.2% of the patients had a primary site of pyriform sinus (PS), respectively. In terms of the histological type, 70.4% of the total number of patients had SCC, among whom, 16.8% had keratinized SCC and 5.4% had non-keratinized SCC. More specifically, in the training set 16.5% of the patients had keratinized SCC and 5.5% had non-keratinized SCC, while in the validation set, 17.6% had keratinized SCC and 5.3% had non-keratinized SCC. In relation to tumor tissue size, we found that the >6-cm group comprised the most patients, and the <3-cm group comprised the fewest patients. In the total population, 2,770 patients had tumor tissue >6 cm in size, 554 patients had tumor tissue between 3–6 cm in size, and 481 patients had tumor tissue <3 cm in size. More specifically, the tumor tissue size of 1,934 patients in the training set and 836 patients in the validation set was >6 cm. The tumor size of 396 patients in the training set and 158 patients in the verification set was between 3-6 cm, and the tumor size of 334 patients in the training set and 147 patients in the verification set was less than 3 cm. Somewhat surprisingly, 50 percent of throat cancer patients earned more than $60,000. See Table 1 for details for the other indicators.
Table 1
Characteristics | Overall (n=3,805) | Testing data set (n=1,141) | Training data set (n=2,664) | P |
---|---|---|---|---|
Status (%) | 1 | |||
0 | 1,290 (33.9) | 387 (33.9) | 903 (33.9) | |
1 | 411 (10.8) | 123 (10.8) | 288 (10.8) | |
2 | 2,104 (55.3) | 631 (55.3) | 1,473 (55.3) | |
Time (months), [median (IQR)] | 16.00 (6.00, 42.00) | 18.00 (7.00, 42.00) | 16.00 (6.00, 42.00) | 0.033 |
Sex, male (%) | 3,079 (80.9) | 917 (80.4) | 2,162 (81.2) | 0.602 |
Race (%) | 0.822 | |||
Asian | 274 (7.2) | 84 (7.4) | 190 (7.1) | |
Black | 542 (14.2) | 163 (14.3) | 379 (14.2) | |
Other | 50 (1.3) | 12 (1.1) | 38 (1.4) | |
White | 2,939 (77.2) | 882 (77.3) | 2,057 (77.2) | |
Primary site (%) | 0.44 | |||
AF | 286 (7.5) | 80 (7.0) | 206 (7.7) | |
Other | 1,444 (38.0) | 447 (39.2) | 997 (37.4) | |
PS | 1,790 (47.0) | 538 (47.2) | 1,252 (47.0) | |
PWH | 285 (7.5) | 76 (6.7) | 209 (7.8) | |
Grade (%) | 0.36 | |||
Grade I | 148 (3.9) | 48 (4.2) | 100 (3.8) | |
Grade II | 1,498 (39.4) | 460 (40.3) | 1,038 (39.0) | |
Grade III | 1,147 (30.1) | 355 (31.1) | 792 (29.7) | |
Grade IV | 32 (0.8) | 9 (0.8) | 23 (0.9) | |
Unknown | 980 (25.8) | 269 (23.6) | 711 (26.7) | |
Behavior (%) | 0.866 | |||
Other | 278 (7.3) | 84 (7.4) | 194 (7.3) | |
SCC | 2,679 (70.4) | 795 (69.7) | 1,884 (70.7) | |
SCC, keratinizing | 641 (16.8) | 201 (17.6) | 440 (16.5) | |
SCC, non-keratinizing | 207 (5.4) | 61 (5.3) | 146 (5.5) | |
Summary stage (%) | 0.236 | |||
Distant | 1,329 (34.9) | 376 (33.0) | 953 (35.8) | |
Localized | 667 (17.5) | 203 (17.8) | 464 (17.4) | |
Regional | 1,809 (47.5) | 562 (49.3) | 1,247 (46.8) | |
Stage group (%) | 0.229 | |||
I | 143 (3.8) | 48 (4.2) | 95 (3.6) | |
II | 330 (8.7) | 83 (7.3) | 247 (9.3) | |
III | 548 (14.4) | 175 (15.3) | 373 (14.0) | |
IV | 1,998 (52.5) | 596 (52.2) | 1,402 (52.6) | |
Unknown | 786 (20.7) | 239 (20.9) | 547 (20.5) | |
T* staging (%) | 0.122 | |||
T0/T1 | 308 (8.1) | 100 (8.8) | 208 (7.8) | |
T2 | 1,021 (26.8) | 283 (24.8) | 738 (27.7) | |
T3 | 735 (19.3) | 242 (21.2) | 493 (18.5) | |
T4 | 867 (22.8) | 249 (21.8) | 618 (23.2) | |
TX | 257 (6.8) | 86 (7.5) | 171 (6.4) | |
Unknown | 617 (16.2) | 181 (15.9) | 436 (16.4) | |
N staging (%) | 0.963 | |||
N0 | 988 (26.0) | 304 (26.6) | 684 (25.7) | |
N1 | 566 (14.9) | 164 (14.4) | 402 (15.1) | |
N2 | 1,430 (37.6) | 429 (37.6) | 1,001 (37.6) | |
N3 | 156 (4.1) | 46 (4.0) | 110 (4.1) | |
NX | 665 (17.5) | 198 (17.4) | 467 (17.5) | |
Nodes, removed, yes (%) | 640 (16.8) | 206 (18.1) | 434 (16.3) | 0.199 |
Surg rad seq (%) | 0.375 | |||
1 | 61 (1.6) | 23 (2.0) | 38 (1.4) | |
2 | 830 (21.8) | 242 (21.2) | 588 (22.1) | |
3 | 2,900 (76.2) | 870 (76.2) | 2,030 (76.2) | |
Other | 14 (0.4) | 6 (0.5) | 8 (0.3) | |
Surgery, yes (%) | 854 (22.4) | 268 (23.5) | 586 (22.0) | 0.333 |
Radiation, yes (%) | 2,871 (75.5) | 863 (75.6) | 2,008 (75.4) | 0.897 |
Chemotherapy, yes (%) | 2,311 (60.7) | 716 (62.8) | 1,595 (59.9) | 0.103 |
Systemic, therapy, yes (%) | 775 (20.4) | 234 (20.5) | 541 (20.3) | 0.923 |
Months, treatment (%) | 0.145 | |||
<3 | 3,108 (81.7) | 952 (83.4) | 2,156 (80.9) | |
>3 | 316 (8.3) | 90 (7.9) | 226 (8.5) | |
Unknown | 381 (10.0) | 99 (8.7) | 282 (10.6) | |
Nodes, positive, yes (%) | 2,686 (70.6) | 798 (69.9) | 1,888 (70.9) | 0.59 |
Size (%) | 0.707 | |||
<3 | 481 (12.6) | 147 (12.9) | 334 (12.5) | |
>6 | 2,770 (72.8) | 836 (73.3) | 1,934 (72.6) | |
3–6 | 554 (14.6) | 158 (13.8) | 396 (14.9) | |
Bone metastasis, yes (%) | 75 (2.0) | 19 (1.7) | 56 (2.1) | 0.447 |
Liver metastasis (%) | 0.159 | |||
No | 3,755 (98.7) | 1,129 (98.9) | 2,626 (98.6) | |
Yes | 49 (1.3) | 11 (1.0) | 38 (1.4) | |
Unknown | 1 (0.0) | 1 (0.1) | 0 (0.0) | |
Lung metastasis (%) | 0.918 | |||
No | 3,613 (95.0) | 1,085 (95.1) | 2,528 (94.9) | |
Unknown | 12 (0.3) | 3 (0.3) | 9 (0.3) | |
Yes | 180 (4.7) | 53 (4.6) | 127 (4.8) | |
Node metastasis (%) | 0.933 | |||
No | 1,161 (30.5) | 344 (30.1) | 817 (30.7) | |
Unknown | 2,606 (68.5) | 785 (68.8) | 1,821 (68.4) | |
Yes | 38 (1.0) | 12 (1.1) | 26 (1.0) | |
Primary, yes (%) | 2,725 (71.6) | 809 (70.9) | 1,916 (71.9) | 0.549 |
Number of malignant, >3 (%) | 127 (3.3) | 50 (4.4) | 77 (2.9) | 0.025 |
Age, years (%) | 0.839 | |||
<60 | 1,152 (30.3) | 353 (30.9) | 799 (30.0) | |
>80 | 429 (11.3) | 130 (11.4) | 299 (11.2) | |
60–80 | 2,223 (58.4) | 658 (57.7) | 1,565 (58.7) | |
Unknown | 1 (0.0) | 0 (0.0) | 1 (0.0) | |
Marital status (%) | 0.752 | |||
Divorced | 518 (13.6) | 142 (12.4) | 376 (14.1) | |
Married | 1,710 (44.9) | 529 (46.4) | 1,181 (44.3) | |
Other | 219 (5.8) | 63 (5.5) | 156 (5.9) | |
Separated | 56 (1.5) | 16 (1.4) | 40 (1.5) | |
Single | 915 (24.0) | 277 (24.3) | 638 (23.9) | |
Widowed | 387 (10.2) | 114 (10.0) | 273 (10.2) | |
Income (%) | 0.707 | |||
<$40,000 | 252 (6.6) | 75 (6.6) | 177 (6.6) | |
>$60,000 | 2,428 (63.8) | 739 (64.8) | 1,689 (63.4) | |
$40,000–$60,000 | 1,125 (29.6) | 327 (28.7) | 798 (30.0) |
*, TNM staging, TNM groups by 7th edition of the American Joint Committee on Cancer Classifications. AF, aryepiglottic fold; PS, pyriform sinus; PWH, posterior wall of hypopharynx; SCC, sequamous-cell carcinoma.
Before constructing the nomogram, we determined the HR value of each variable and its 95% CI. The multivariate analysis revealed that patients aged >80 years had the highest risk of exposure (HR =1.86, CI: 1.28–2.7). Compared to non-keratinized SCC (HR =0.92, CI: 0.4–2.16), keratinized SCC (HR =1.8, CI: 0.98–3.3) had a higher risk of exposure in terms of laryngeal cancer histological types. Patients with a primary tumor site in the piriform fossa (HR =1.79, CI: 0.96–3.33) and posterior pharyngeal wall (HR =1.8, CI: 0.87–3.75) had a slightly higher risk of death than other primary tumor site, but the difference was not significant. Patients with a tumor size of 3–6 cm (HR =2.3, CI: 1.37–3.87) had a significantly increased risk of death than patients with a tumor size >6 cm (HR =1.54, CI: 0.96–2.45). In addition, the univariate analysis showed that in patients with laryngeal cancer, the risk coefficient of patients with liver metastasis (HR =1.89, CI: 1.89–4.03) was higher than that of patients with lung metastasis (HR =1.59, CI: 1.59–2.52). N stage N2 (HR =1.84, CI: 1.84–2.52), and Grade III (HR =0.89, CI: 0.89–1.58) had the highest mortality risk coefficients in other stages and grades see Table 2 for details for the other indicators.
Table 2
Levels | Factor | Univariate analysis | Multivariate analysis | |||
---|---|---|---|---|---|---|
HR (95% CI) | P | HR (95% CI) | P | |||
Age >80 years | Age | 1.79 (1.79–2.6) | 0.0020 | 1.86 (1.28–2.7) | 0.0012 | |
Age 60–80 years | Age | 1.22 (1.22–1.61) | 0.1400 | 1.18 (0.9–1.55) | 0.2400 | |
Age unknown | Age | 24.59 (24.59–31.57) | 0.0000 | 20.31 (12.78–32.26) | 0.0000 | |
Behavior SCC | Behavior | 1.77 (1.77–3.09) | 0.0440 | 1.76 (1.01–3.07) | 0.0470 | |
Behavior SCC, keratinizing | Behavior | 1.75 (1.75–3.21) | 0.0730 | 1.8 (0.98–3.3) | 0.0570 | |
Behavior SCC, non-keratinizing | Behavior | 0.93 (0.93–2.16) | 0.8600 | 0.92 (0.4–2.16) | 0.8600 | |
Bone metastasis: yes | Bone metastasis | 3.26 (3.26–5.52) | 0.0000 | 3.35 (1.94–5.79) | 0.0000 | |
Chemotherapy: yes | Chemotherapy | 1 (1–1.26) | 0.9700 | |||
Grade: Grade II | Grade | 0.88 (0.88–1.55) | 0.6500 | |||
Grade: Grade III | Grade | 0.89 (0.89–1.58) | 0.6800 | |||
Grade: Grade IV | Grade | 0.31 (0.31–2.43) | 0.2700 | |||
Grade: unknown | Grade | 0.72 (0.72–1.31) | 0.2900 | |||
Income > $60,000 | Income | 1.01 (1.01–1.6) | 0.9700 | |||
Income $40,000–$60,000 | Income | 0.92 (0.92–1.5) | 0.7300 | |||
Liver metastasis: yes | Liver metastasis | 1.89 (1.89–4.03) | 0.0980 | |||
Lung metastasis: unknown | Lung metastasis | 1.15 (1.15–8.46) | 0.8900 | |||
Lung metastasis: yes | Lung metastasis | 1.59 (1.59–2.52) | 0.0510 | |||
Marital status: married | Marital status | 0.93 (0.93–1.31) | 0.6800 | |||
Marital status: other | Marital status | 0.54 (0.54–1.08) | 0.0810 | |||
Marital status: separated | Marital status | 0.65 (0.65–2.14) | 0.4800 | |||
Marital status: single | Marital status | 0.96 (0.96–1.41) | 0.8500 | |||
Marital status: widowed | Marital status | 1.3 (1.3–2.01) | 0.2400 | |||
Months treatment: >3 | Months treatment | 0.83 (0.83–1.3) | 0.4100 | |||
Months treatment: unknown | Months treatment | 1.1 (1.1–1.59) | 0.6200 | |||
Nodes metastasis: unknown | Nodes metastasis | 0.77 (0.77–0.99) | 0.0440 | |||
Nodes metastasis: yes | Nodes metastasis | 0.77 (0.77–3.18) | 0.7200 | |||
Nodes positive: yes | Nodes positive | 1.57 (1.57–2.07) | 0.0017 | |||
Nodes removed: yes | Nodes removed | 0.79 (0.79–1.11) | 0.1700 | |||
N* staging: N1 | N stage | 1.57 (1.57–2.32) | 0.0220 | |||
N* staging: N2 | N stage | 1.84 (1.84–2.52) | 0.0001 | |||
N* staging: N3 | N stage | 1.61 (1.61–2.96) | 0.1200 | |||
N* staging: NX | N stage | 1.68 (1.68–2.56) | 0.0150 | |||
Number of malignant: >3 | Number of malignant | 0.23 (0.23–0.93) | 0.0390 | |||
Primary site: other | Primary site | 2.54 (2.54–4.71) | 0.0030 | 2.34 (1.25–4.38) | 0.0078 | |
Primary site: PS | Primary site | 1.97 (1.97–3.64) | 0.0310 | 1.79 (0.96–3.33) | 0.0680 | |
Primary site: PWH | Primary site | 1.96 (1.96–4.06) | 0.0710 | 1.8 (0.87–3.75) | 0.1200 | |
Primary: yes | Primary | 1.27 (1.27–1.66) | 0.0910 | |||
Race: black | Race | 0.5 (0.5–0.83) | 0.0080 | 0.56 (0.33–0.95) | 0.0330 | |
Race: other | Race | 0.71 (0.71–2.08) | 0.5400 | 0.87 (0.29–2.59) | 0.8000 | |
Race: white | Race | 0.71 (0.71–1.04) | 0.0780 | 0.78 (0.52–1.17) | 0.2200 | |
Radiation: yes | Radiation | 0.9 (0.9–1.18) | 0.4500 | |||
Sex: male | Sex | 0.93 (0.93–1.24) | 0.6200 | |||
Size: >6 cm | Size | 1.51 (1.51–2.42) | 0.0820 | 1.54 (0.96–2.45) | 0.0720 | |
Size: 3–6 cm | Size | 2.39 (2.39–4.03) | 0.0011 | 2.3 (1.37–3.87) | 0.0017 | |
Stage group II | Stage group II | 0.91 (0.91–2.35) | 0.8500 | |||
Stage group III | Stage group III | 1.64 (1.64–3.84) | 0.2500 | |||
Stage group IV | Stage group IV | 2.31 (2.31–5.14) | 0.0400 | |||
Stage group II: unknown | Stage group II | 1.89 (1.89–4.38) | 0.1400 | |||
Summary stage: localized | Summary stage | 0.41 (0.41–0.63) | 0.0000 | |||
Summary stage: regional | Summary stage | 0.79 (0.79–1.01) | 0.0600 | |||
Surgery radiation sequence: 2 | Radiation after surgery | 0.83 (0.83–2.02) | 0.6900 | |||
Surgery radiation sequence: 3 | No radiation | 0.79 (0.79–1.88) | 0.6000 | |||
Surgery radiation sequence: other | Other | 0.85 (0.85–6.89) | 0.8800 | |||
Surgery: yes | Surgery | 0.82 (0.82–1.1) | 0.1900 | |||
Systemic therapy: yes | Systemic therapy | 1.12 (1.12–1.48) | 0.4000 | |||
T* staging: T2 | T stage | 1.57 (1.57–2.76) | 0.1200 | |||
T* staging: T3 | T stage | 1.92 (1.92–3.41) | 0.0270 | |||
T* staging: T4 | T stage | 2.36 (2.36–4.12) | 0.0025 | |||
T* staging: TX | T stage | 1.14 (1.14–2.42) | 0.7300 | |||
T* staging: unknown | T stage | 2.02 (2.02–3.74) | 0.0250 |
*, TNM staging, TNM groups by 7th edition of the American Joint Committee on Cancer Classifications. SCC, sequamous-cell carcinoma; PS, pyriform sinus; PWH, posterior wall of hypopharynx.
The areas under the ROC curve (AUC) were 0.702 and 0.695 for the training cohort and 0.737 and 0.644 for the validation cohort at 6 and 12 months, respectively. The AUC of the ROC model was > or around 0.7, indicating good predictive performance in this study, and the calibration curve showed good homogeneity without overestimation and underestimation risks. The ROC model and calibration curve showed that there was no significant difference between the variables in the training set and the validation set, and thus the sets were comparable. See Figures 1,2 for further details.
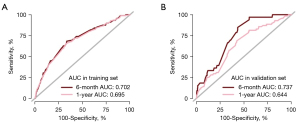
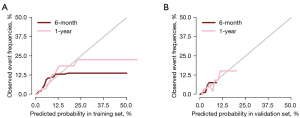
In the Fine&Gray analysis, according to the established nomogram, we found that patients aged >80 years, patients with keratinized SCC, patients with primary tumor in PS, and patients with a tumor size of 3–6 cm were at a higher risk of death in the short-term, which is consistent with the above description. Using the nomogram, we could roughly predict the short-term specific mortality of patients with laryngeal cancer based on an accumulation of the risk factors. See Figure 3 for details.
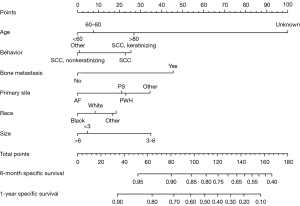
Discussion
Pathological types and short-term survival of laryngeal carcinoma patients
Laryngeal carcinoma is a common head and neck tumor, and SCC, including traditional keratinized SCC, non-keratinized SCC, laryngeal basal SCC, verrucous SCC, papillary SCC, and spinous SCC, accounts for about 95% of all laryngeal carcinomas (the histological classification of SCC is based on the World Health Organization’s classification of laryngeal carcinoma) (19,20).
In our nomogram, the short-term survival rate of non- keratinized SCC was higher than that of keratinized SCC, which is consistent with previous studies that have shown that the mortality rate of keratinized SCC was higher than that of non-keratinized SCC. Previous studies and the continuous improvement of research techniques have confirmed that the laryngeal carcinoma is mainly associated with smoking and alcohol consumption over a long period (21). The study found that excessive smoking and drinking was closely related to keratinized SCC, such as verrucous squamous cell carcinoma (VSCC) (22).
In the past few decades, research has indicated that HPV is a risk factor of laryngeal cancer and is mainly related to keratinized SCC (23,24). Non-keratinized SCC has not been found to be associated with smoking and alcohol abuse (25). The cytological morphology of these non-keratinized SCC is usually blurred cell (oval and spindle-shaped basal cell) boundaries, and the tumor cells are mitotically active with numerous mitosis and apoptosis (26,27). This type of non-keratinized SCC is mainly associated with high-risk HPV16, 18 (21).
High-risk HPV oncoproteins may result in the hydrolysis of transcription factor p53 (P53) (28), which in turn may interfere with the function of P53 and Retinoblastoma gene (RB), a transcriptional cofactor. P53 and RB control many transcription-related genes mainly through p53-021-Rb. The impaired function of P53 and RB is a core step in the occurrence of cancer, and their impaired function will affect the normal life cycle of cells, inhibit cell apoptosis, promote cell immortalization, and cause cell malignant transformation (29-32). This type of HPV-associated laryngeal cancer is present in both non-keratinized SCC, and less common SCC types. Kasperbauer et al. (33) suggested that verrucous SCC might be associated with HPV, and Crissman et al. (34) suggested that papillary SCC was associated with HPV (21). It is thought that this HPV-associated non-keratinized SCC of the throat and the rarer HPV-associated SCC are more sensitive to chemotherapy and radiotherapy, and the prognosis of these types is significantly better than that of traditional, more typical keratinized SCC (35). There was no detailed classification of diagonalized SCC and non-keratinized SCC in our nomogram, which may be the reason why the short-term survival rate of the non-keratinized SCC patients was higher than that of the keratinized SCC patients in our nomogram.
Primary location, metastasis, and short-term survival of laryngeal carcinoma patients
Laryngeal cancer is divided into supraglottic laryngeal cancer, glottic laryngeal cancer, and sub-hilar laryngeal cancer according to the location of onset. The laryngeal cancer glottis area is relatively frequent. Subglottic and supraglottic laryngeal carcinoma have local invasion and distant metastasis due to the abundant lymphatic system and blood source system in their anatomical locations. Supraglotic laryngeal carcinoma and Subglottic laryngeal carcinoma are often detected late because the initial symptom is dysphagia. Previous study has reported that the recurrence rate of glottic type in laryngeal cancer after 2 years is 4%, the Supraglottic recurrence rate is 16%, and the subglottic recurrence rate is 11% (36). The first symptoms from the above and the chance of recurrence.
We note that the prognosis of supraglottic and subglottic laryngeal cancer is worse than that of glottic laryngeal cancer. In the SEER-based data used in this study, most laryngeal cancers occurred in the subglottic area.
We used our nomogram to predict the short-term survival risks for the main types of laryngeal cancer. In our analysis, the stage of laryngeal cancer was not significant and thus was not included in the competing risk model. However, in relation to the type, we found that the short-term mortality risk of laryngeal cancer originating in the posterior pharyngeal wall was higher than that of laryngeal cancer originating in the piriform crypt, but the difference between the 2 was not significant. In addition, the occurrence of multiple organ metastases, such as liver, bone, lung, and brain metastases, in laryngeal cancer patients was closely related to the prognosis and survival rates of patients. Among them, liver (45%) and bone metastases (25%) have been reported to be common (37).
Incidence of laryngeal cancer and economic income
In the survival competing risk model, we found that the risk of laryngeal cancer was correlated with the income of patients, and most laryngeal cancers occurred in the high-income population. This may be related to current social factors. For example, because of the increase of work pressure and the improvement and enrichment of material conditions, more of these patients choose to smoke and drink to relieve stress (38-40). However, the data reported up to 2016 revealed that there has been a significant decrease in the prevalence of laryngeal cancer in high-risk countries in Northern Europe (1) due to a decline in the smoking rates and amendments to national tobacco control policies. Additionally, a diet high in red meat has been linked to throat cancer (7), which may also be related to economics.
This study had a number of strengths. First of all, the sample size of previous studies on the mortality rate of patients with laryngeal cancer was relatively small. For example, in the study on the survival rate of laryngeal cancer conducted by Fujii et al. (15), only 88 patients with laryngeal cancer were included, and all underwent laryngeal cancer surgery. However, Francis et al.’s study only analyzed the survival rate of patients with laryngeal cancer at T4 stage (16,41). Compared with previous studies, our study included more people with a larger sample size and included laryngeal cancer patients of different races, ages and genders.
Next, common machine learning models such as random forest, neural network and support vector machine have a “black box” effect, while the graph model is intuitive and clear, which is easier to gain the trust of clinicians. In addition, we filled a gap in the research on short-term specific mortality risks in patients with laryngeal cancer. In contrast to traditional Cox models, we developed a Fine&Gray model with a specific mortality outcome of death from laryngeal cancer, as patients with laryngeal cancer have a very high risk of dying of non-laryngeal cancer. We excluded death from other causes, such as car accidents, other social factors such as cardiovascular disease or non-laryngeal cancer, which are more specific for predicting short-term survival in patients with laryngeal cancer. In addition, our nomogram was better able to predict the survival rate of an individual patient with multiple risk factors. However, our study still had some limitations. First, due to limitations of the data available in the SEER database, we did not provide a more detailed classification of SCC, and the nomogram did not include different treatment methods (e.g., surgery, radiotherapy, chemotherapy, and targeted drug therapy). Second, we did not examine the combination of tumor grade, stage, and different treatments in our short-term survival risk predictions and assessments, and we did not examine the relationship between the short-term survival rate and vocational education, family environment and patient suffering. In the future, we intend to develop Fine&Gray models to predict laryngeal cancer survival rates in both the short and long term.
Conclusions
We developed a competitive risk model based on the SEER database for laryngeal cancer patients to predict specific mortality. In our competitive risk model, we intuitively showed that age, tumor histological type, and primary location were all associated with laryngeal cancer mortality. Moreover, this competitive risk model may help doctors predict the specific mortality of laryngeal cancer patients more intuitively.
Acknowledgments
Funding: This work was supported by the Beijing Hospitals Authority's Ascent Plan (No. DFL20221102); and the Beijing Hospitals Authority Clinical Medicine Development of Special Funding (No. XLMX202110).
Footnote
Reporting Checklist: The authors have completed the TRIPOD reporting checklist. Available at https://atm.amegroups.com/article/view/10.21037/atm-23-400/rc
Peer Review File: Available at https://atm.amegroups.com/article/view/10.21037/atm-23-400/prf
Conflicts of Interest: All authors have completed the ICMJE uniform disclosure form (available at https://atm.amegroups.com/article/view/10.21037/atm-23-400/coif). The authors have no conflicts of interest to declare.
Ethical Statement: The authors are accountable for all aspects of the work in ensuring that questions related to the accuracy or integrity of any part of the work are appropriately investigated and resolved. The study was conducted in accordance with the Declaration of Helsinki (as revised in 2013).
Open Access Statement: This is an Open Access article distributed in accordance with the Creative Commons Attribution-NonCommercial-NoDerivs 4.0 International License (CC BY-NC-ND 4.0), which permits the non-commercial replication and distribution of the article with the strict proviso that no changes or edits are made and the original work is properly cited (including links to both the formal publication through the relevant DOI and the license). See: https://creativecommons.org/licenses/by-nc-nd/4.0/.
References
- Mannelli G, Lazio MS, Luparello P, et al. Conservative treatment for advanced T3-T4 laryngeal cancer: meta-analysis of key oncological outcomes. Eur Arch Otorhinolaryngol 2018;275:27-38. [Crossref] [PubMed]
- Huang A, Wu XL, Song J, et al. Global trend and risk factors of the disease burden for pharynx and larynx cancers between 1990 and 2019: a systematic analysis of the global burden of disease study 2019. BMC Public Health 2022;22:2192. [Crossref] [PubMed]
- Tuyns AJ, Estève J, Raymond L, et al. Cancer of the larynx/hypopharynx, tobacco and alcohol: IARC international case-control study in Turin and Varese (Italy), Zaragoza and Navarra (Spain), Geneva (Switzerland) and Calvados (France). Int J Cancer 1988;41:483-91. [Crossref] [PubMed]
- Cui W, Meng W, Zhao L, et al. TGF-β-induced long non-coding RNA MIR155HG promotes the progression and EMT of laryngeal squamous cell carcinoma by regulating the miR-155-5p/SOX10 axis. Int J Oncol 2019;54:2005-18. [Crossref] [PubMed]
- Kolator M, Kolator P, Zatoński T. Assessment of quality of life in patients with laryngeal cancer: A review of articles. Adv Clin Exp Med 2018;27:711-5. [Crossref] [PubMed]
- Bradley PJ, Piazza C, Paderno A. A roadmap of six different pathways to improve survival in laryngeal cancer patients. Curr Opin Otolaryngol Head Neck Surg 2021;29:65-78. [Crossref] [PubMed]
- Steuer CE, El-Deiry M, Parks JR, et al. An update on larynx cancer. CA Cancer J Clin 2017;67:31-50. [Crossref] [PubMed]
- Bosetti C, Gallus S, Franceschi S, et al. Cancer of the larynx in non-smoking alcohol drinkers and in non-drinking tobacco smokers. Br J Cancer 2002;87:516-8. [Crossref] [PubMed]
- Szymańska K, Hung RJ, Wünsch-Filho V, et al. Alcohol and tobacco, and the risk of cancers of the upper aerodigestive tract in Latin America: a case-control study. Cancer Causes Control 2011;22:1037-46. [Crossref] [PubMed]
- Dantas de Oliveira NP, Barbosa IR, Vieria Paulino JN, et al. Regional and gender differences in laryngeal cancer mortality: trends and predictions until 2030 in Brazil. Oral Surg Oral Med Oral Pathol Oral Radiol 2016;122:547-54. [Crossref] [PubMed]
- Gama RR, Carvalho AL, Longatto Filho A, et al. Detection of human papillomavirus in laryngeal squamous cell carcinoma: Systematic review and meta-analysis. Laryngoscope 2016;126:885-93. [Crossref] [PubMed]
- Mylly M, Nissi L, Huusko T, et al. Epidemiological Study of p16 Incidence in Head and Neck Squamous Cell Carcinoma 2005-2015 in a Representative Northern European Population. Cancers (Basel) 2022;14. [Crossref] [PubMed]
- Lee HI, Lee J, Lee JH, et al. Evaluation of Optimal Assessment Schedules for Surveillance After Definitive Locoregional Treatment of Locally Advanced Head and Neck Cancer: A Retrospective Cohort Study With Parametric Modeling of Event-Free Survival. JAMA Otolaryngol Head Neck Surg 2022;148:1059-67. [Crossref] [PubMed]
- Baselga J. Why the epidermal growth factor receptor? The rationale for cancer therapy. Oncologist 2002;7:2-8. [Crossref] [PubMed]
- Fujii T, Miyabe J, Yoshii T, et al. Metabolic tumor volume of metastatic lymph nodes and survival after total laryngectomy in laryngeal and hypopharyngeal cancer. Oral Oncol 2019;93:107-13. [Crossref] [PubMed]
- Pradier R, Gonzalez A, Matos E, et al. Prognostic factors in laryngeal carcinoma. Experience in 296 male patients. Cancer 1993;71:2472-6. [Crossref] [PubMed]
- Xu YB, Liu H, Cao QH, et al. Evaluating overall survival and competing risks of survival in patients with early-stage breast cancer using a comprehensive nomogram. Cancer Med 2020;9:4095-106. [Crossref] [PubMed]
- Doll KM, Rademaker A, Sosa JA. Practical Guide to Surgical Data Sets: Surveillance, Epidemiology, and End Results (SEER) Database. JAMA Surg 2018;153:588-9. [Crossref] [PubMed]
- Licitra L, Bernier J, Grandi C, et al. Cancer of the larynx. Crit Rev Oncol Hematol 2003;47:65-80. [Crossref] [PubMed]
- Yan L, Song X, Yang G, et al. Identification and Validation of Immune Infiltration Phenotypes in Laryngeal Squamous Cell Carcinoma by Integrative Multi-Omics Analysis. Front Immunol 2022;13:843467. [Crossref] [PubMed]
- López F, Williams MD, Cardesa A, et al. How phenotype guides management of non-conventional squamous cell carcinomas of the larynx? Eur Arch Otorhinolaryngol 2017;274:2709-26. [Crossref] [PubMed]
- Echanique KA, Desai SV, Marchiano E, et al. Laryngeal Verrucous Carcinoma. Otolaryngol Head Neck Surg 2017;156:38-45. [Crossref] [PubMed]
- Prabhu SR, Wilson DF. Human papillomavirus and oral disease - emerging evidence: a review. Aust Dent J 2013;58:2-10; quiz 125. [Crossref] [PubMed]
- Wu X, Xiao Y, Zhou S, et al. Transcriptomic Landscape of Gene Expression Profiles and Pathways in Juvenile-Onset Recurrent Respiratory Papillomatosis Tumor Tissues and Human Papillomavirus 6 and 11 E6- and E7-Overexpressing Head and Neck Squamous Cell Carcinoma Cell Lines. J Virol 2022;96:e0134221. [Crossref] [PubMed]
- El-Mofty SK. Human papillomavirus-related head and neck squamous cell carcinoma variants. Semin Diagn Pathol 2015;32:23-31. [Crossref] [PubMed]
- El-Mofty SK, Lu DW. Prevalence of human papillomavirus type 16 DNA in squamous cell carcinoma of the palatine tonsil, and not the oral cavity, in young patients: a distinct clinicopathologic and molecular disease entity. Am J Surg Pathol 2003;27:1463-70. [Crossref] [PubMed]
- Chernock RD, El-Mofty SK, Thorstad WL, et al. HPV-related nonkeratinizing squamous cell carcinoma of the oropharynx: utility of microscopic features in predicting patient outcome. Head Neck Pathol 2009;3:186-94. [Crossref] [PubMed]
- Hernández Borrero LJ, El-Deiry WS. Tumor suppressor p53: Biology, signaling pathways, and therapeutic targeting. Biochim Biophys Acta Rev Cancer 2021;1876:188556. [Crossref] [PubMed]
- Dyson NJ. RB1: a prototype tumor suppressor and an enigma. Genes Dev 2016;30:1492-502. [Crossref] [PubMed]
- Engeland K. Cell cycle regulation: p53-p21-RB signaling. Cell Death Differ 2022;29:946-60. [Crossref] [PubMed]
- Chen J, Guanizo A, Luong Q, et al. Lineage-restricted neoplasia driven by Myc defaults to small cell lung cancer when combined with loss of p53 and Rb in the airway epithelium. Oncogene 2022;41:138-45. [Crossref] [PubMed]
- Mori T. Involvement of the p53-p16/RB pathway control mechanism in early-stage carcinogenesis in head and neck squamous cell carcinoma. Pathol Int 2022;72:577-88. [Crossref] [PubMed]
- Kasperbauer JL, O'Halloran GL, Espy MJ, et al. Polymerase chain reaction (PCR) identification of human papillomavirus (HPV) DNA in verrucous carcinoma of the larynx. Laryngoscope 1993;103:416-20. [Crossref] [PubMed]
- Crissman JD, Kessis T, Shah KV, et al. Squamous papillary neoplasia of the adult upper aerodigestive tract. Hum Pathol 1988;19:1387-96. [Crossref] [PubMed]
- Cai C, Chernock RD, Pittman ME, et al. Keratinizing-type squamous cell carcinoma of the oropharynx: p16 overexpression is associated with positive high-risk HPV status and improved survival. Am J Surg Pathol 2014;38:809-15. [Crossref] [PubMed]
- Spector JG, Sessions DG, Haughey BH, et al. Delayed regional metastases, distant metastases, and second primary malignancies in squamous cell carcinomas of the larynx and hypopharynx. Laryngoscope 2001;111:1079-87. [Crossref] [PubMed]
- Berrino F, Gatta G. Variation in survival of patients with head and neck cancer in Europe by the site of origin of the tumours. EUROCARE Working Group. Eur J Cancer 1998;34:2154-61. [Crossref] [PubMed]
- Tomioka K, Kurumatani N, Saeki K. The Association Between Education and Smoking Prevalence, Independent of Occupation: A Nationally Representative Survey in Japan. J Epidemiol 2020;30:136-42. [Crossref] [PubMed]
- Skrzynski C, Creswell KG, Bachrach RL, et al. Social discomfort moderates the relationship between drinking in response to negative affect and solitary drinking in underage drinkers. Addict Behav 2018;78:124-30. [Crossref] [PubMed]
- Temmen CD, Crockett LJ. Relations of Stress and Drinking Motives to Young Adult Alcohol Misuse: Variations by Gender. J Youth Adolesc 2020;49:907-20. [Crossref] [PubMed]
- Francis E, Matar N, Khoueir N, et al. T4a laryngeal cancer survival: retrospective institutional analysis and systematic review. Laryngoscope 2014;124:1618-23. [Crossref] [PubMed]
(English Language Editor: L. Huleatt)