Identification of ferroptosis-related genes in ulcerative colitis: a diagnostic model with machine learning
Highlight box
Key findings
• This study found 5 hub genes (LCN2, MUC1, PARP8, PLIN2, and TIMP1) that were positively correlated with ferroptosis in UC and constructed a diagnostic model with machine learning to identify UC patients from control participants.
What is known and what is new?
• Ferroptosis, a programmed cell death, links to an iron-dependent manner. An increasing amount of research has shown a connection between ferroptosis and the etiology of UC.
• The present work served as a referential basis to determine ferroptosis as a potential target against UC and elucidated whether there was a connection between ferroptosis-associated genes and UC.
What is the implication, and what should change now?
• This study found 5 hub genes that were correlated with ferroptosis in UC, allowing us to identify UC patients. However, more experiments are needed to show how these central genes affect the development of UC.
Introduction
Ulcerative colitis (UC) is an idiopathic, chronic disorder characterized by inflammation, injury, and disruption of the colonic mucosa (1). This condition typically occurs in the rectum and develops proximally in a continuous manner throughout a section or the whole colon (2). The prevalence of UC is on the rise, and it negatively impacts financial status and morbidity (3). The pathogenesis of UC is not fully understood; however, it might be associated with the imbalance of intestinal microbe, immunological response, lifestyle, as well as genetic susceptibility (4). As a result, in-depth recognition of UC contributes to improving its diagnosis, management, and prognosis (5). Patients with UC typically undergo 2 periods during the disease progression: an active state and the presence of more prominent symptoms, and a remission state, in which symptoms are absent and the disease is said to be in remission (6). UC is a progressive condition that may cause diverse disorders in the intestine, namely colon cancer, and patients will live in a poor quality of life (7). Early remission and maintenance are the goals of the treatment to prevent another episode of the disease (8). However, there are still many difficulties in the diagnosis and differential diagnosis of UC. The existing machine learning diagnosis model has low efficiency and single algorithm (9,10).
Ferroptosis, a programmed cell death lately discovered, links to the consumption of polyunsaturated fatty acids in the plasma membrane and additional accumulation of lipid reactive oxygen species (ROS) in an iron-dependent manner (11,12). An increasing number of researches have shown a connection between ferroptosis and the etiology of UC (13-15). Some previous research teams have reported that iron chelator administration can considerably decrease ROS in the colonic tissues, relieve clinical symptoms, and enhance endoscopic presentations for UC patients (16,17). In contrast, iron supplementation with a high iron content has worsened the disease conditions in patients and murine models of UC (18,19). Anecdotally, oral iron supplements aggravate inflammatory bowel disease and increase iron levels in the irritated mucosa. Desferrioxamine, an iron chelator, is allegedly useful in Crohn’s disease (16). Current research on ferroptosis in UC is in its infancy, and the significance of ferroptosis in the pathophysiology of UC is not entirely known. Many diagnostic models have been developed using ferroptosis-related genes and machine learning algorithms, and they have good diagnostic performance (20-22).
In addition to previous research, the present work served as a referential basis to determine ferroptosis as a potential target against UC. It aimed to elucidate whether there was a connection between ferroptosis-associated genes and UC. This research demonstrated the potential for hub genes to be biomarkers for the diagnosis and treatment monitoring of ferroptosis-related illness. We present the following article in accordance with the TRIPOD reporting checklist (available at https://atm.amegroups.com/article/view/10.21037/atm-23-276/rc).
Methods
Gene expression profiles
The GEO database contains 2 microarray datasets of UC (GSE87466 and GSE75214). GSE87466 was based on the GPL13158 platform (23), and GSE75214 was based on the GPL6244 platform (24). This work reviewed data from 87 UC participants and 21 control samples in GSE87466 and 97 UC cases and 22 samples for control in GSE75214. The study was conducted in accordance with the Declaration of Helsinki (as revised in 2013). The flowchart is presented in Figure 1.
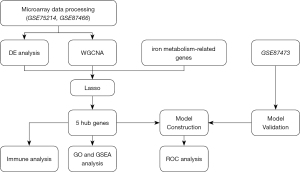
Differential expression analysis
The R package “limma” was employed for differential expression analysis and comparison of UC samples and control samples. Genes with abs (logFC) >1.00 and P_adj <0.01 were considered differentially expressed genes (DEGs). The “pheatmap” and “ggplot2” programs were used to generate DEG heat maps and volcano plots.ff.
Weighted correlation network analysis (WGCNA)
First, a batch effect was eliminated from the expression profiles of both datasets prior to further analysis. Network diagrams of gene co-expression were created via WGCNA (25). A weighted correlation coefficient was used to build an adjacency matrix. Subsequently, based on the data obtained, a topological matrix was created. After module identification using hierarchical clustering, eigengene was determined. Pearson correlation analysis was then carried out to determine where there existed a link between phenotype and the modules to identify UC-related modules.
Identification of hub genes by intersection and lasso regression
Genes associated with ferroptosis were retrieved from FerrDb, known as the world’s first manually curated database for ferroptosis regulators and ferroptosis-disease associations from published journal articles (26). To identify the hub genes of UC, the “VennDiagram” package in R software was used to intersect genes from DEGs, WGCNA, and ferroptosis-related genes. Then, these hub genes were further screened using the lasso regression algorithm. As a penalized regression method, lasso regression modifies the regression coefficient by using the L1 penalty to bring the weight of the vast majority of prospective indicators down to zero, reducing many potential indicators that have final weights other than zero (27).
Enrichment analysis
Functional enrichment was analyzed to understand possible molecular processes of hub genes impacting UC. We initially investigated the associated genes at the biological processes level through gene ontology (GO). Tthe findings were shown using the “GOplot” package of the R programming language. Gene set enrichment analysis (GSEA) was subsequently used to determine the specific roles of each gene. In light of the expression of hub gene median values, samples were divided into low and high expression groups. The “enrichplot” R software tool was used to visualize the findings. All analyses employed “clusterProfiler” package of the R program, with P adj 0.05 serving as the screening criterion.
Immune-related factors and immune infiltration
To examine the infiltration of immune cells into the microenvironment, CIBERSORT was used, which contains 22 human immune cells and 547 biomarkers, including T cells, myeloid cell subpopulations, plasma, and B cells. The approach of linear support vector regression is used for immune cell expression matrix deconvolution analysis, which serves as the foundation of this instrument. This research determined the proportional proportions of 22 immune cells in each sample.
Model construction and validation with machine learning
To effectively discriminate UC patients from controls, machine learning was developed that also analyzed the effectiveness of several algorithms for predicting the development of UC. Using the NaiveBeyas, Logistic, IBk, and RandomForest algorithms, we analyzed receiver operator characteristic (ROC) curves to develop a disease diagnostic model based on 5 hub genes: LCN2, MUC1, PARP8, PLIN, and TIMP1. In view of the imbalance in the sample size between UC and normal controls, we divided 184 UC patients (from GSE87466 and GSE75214) into 4 groups, with 46 patients in each group (corresponding to 43 patients in the normal control group), trained 4 sub-models respectively, and performed aggregation analysis on the results. The parameters of the classifiers were adjusted using five-fold cross-validation while the training operation was carried out. This was beneficial for the creation of the most accurate diagnostic model. The generated model was then validated using the microarray dataset GSE87473 (106 UC participants and 21 control samples). The accuracy of the diagnostic performance of the model was evaluated utilizing the ROC curves as well as the area under the ROC curve.
Statistical analysis
Differences between two groups were assessed using the Student’s t-test. The dimensionality of the selected genes was reduced by LASSO regression. Logistic regression, RandomForest, NaiveBayes and Ibk algorithms were used to construct and validate the diagnosis model. R version 4.2.0 was used for all data analysis and visualization.
Results
Gene expression profiles
The gene expression matrices GSE87466 and GSE75214 were acquired from the GEO database, available for gene level data of 184 UC patients and 43 control samples. Using a screening condition “P adj 0.05 and abs(logFC) >1.00,” 699 genes with differential expression were identified. Such DEGs were visualized using a volcano map (Figure 2A) showing the top 50 DEGs in the heatmap (Figure 2B).
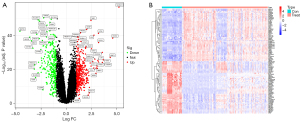
Weighted correlation network analysis (WGCNA)
Following the removal of unqualified samples, all genes were screened, and the extraction of relevant profiles was performed for 10,000 genes from gene expression matrices. A network diagram of weighted gene co-expression was established after filtering gene expression profiles. When the value of a soft threshold was adjusted to 16, scale independence was 0.9222, and the average value of connection was 26.2 (Figure 3A,3B). Cutting down trees dynamically produced 5 distinct co-expression modules (Figure 3C). Subsequently, a correlation study was carried out between each module and the clinical features. The results presented in Figure 3D indicate that the MEturquoise module was the most positively associated with UC (r=0.56; P=3e26), whereas the MEblue module presented a strong negative association with UC (r=0.77; P=1e58). With a total of 1,513 genes, the MEblue module was selected for additional research because it presented the greatest value for the absolute correlation coefficient. Additionally, a correlation study between MM and GS uncovered that both the module and the phenotype were considerably related to these genes (cor=0.74; P=1e200; Figure 3E).
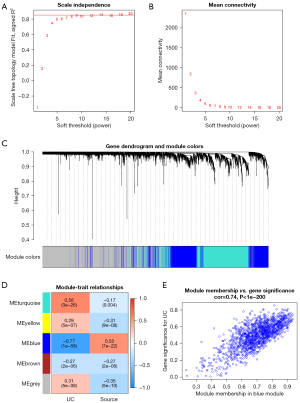
Identification of hub genes associated by intersection and lasso regression
From the FerrDB database, 565 genes relevant to iron metabolism were extracted. Using the DEGs’ shared genes, the WGCNA-retrieved genes, and the ferroptosis-related genes, 17 genes were obtained (Figure 4A). Five hub genes (LCN2, MUC1, PARP8, PLIN2, and TIMP1) were identified by further screening using the Lasso regression algorithm (Figure 4B).
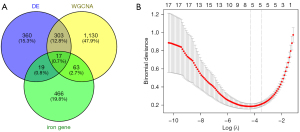
GO and GSEA analysis for the hub genes
We carried out enrichment analyses to clarify certain likely activities of previously described genes. GO analysis indicated that the 5 hub genes were associated with negative regulation of transcription by competitive promoter binding, cellular response to UV-A, protein mono-ADP-ribosylation, protein auto-ADP-ribosylation, iron coordination entity transport, and negative regulation of metallopeptidase activity (Figure 5A). GSEA analysis suggested that such hub genes linked to cytosolic_dna_sensing pathway, citrate cycle_tca_cycle, beta-alanine metabolism, fatty_acid_metabolism, glycosaminoglycan degradation, and proteasome (Figure 5B-5F).
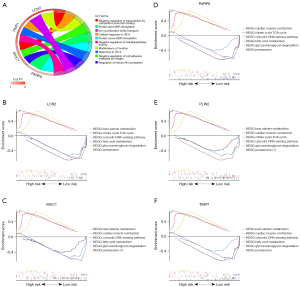
Infiltration of immune and immune-related factors
The microenvironment contains multiple extracellular matrixes, immune cells, inflammatory substances, and many growth factors, all of which substantially affect clinical treatment sensitivity and disease diagnosis. This investigation adopted a CIBERSORT method to estimate the percentage of 22 immune cells present in 184 UC samples and 43 control samples. The results of this investigation are presented in a histogram (Figure 6A). Immune cell infiltration in UC samples was compared with that of control using a vioplot (Figure 6B). A markedly higher proportion was revealed in the UC group for T cell CD4 memory activated, NK cell resting, macrophages M0, macrophages M1, dendritic cells activated, mast cell activated, and neutrophils (all P<0.001) as well as T cell follicular helper (P=0.0047). Meanwhile, a lower proportion was found in T cell CD8, T cell regulatory (Tregs), NK cells activated, macrophages M2, Mast cell resting, and dendritic cell resting (all P<0.001), as well as eosinophils (P=0.0439) than the control group. Following that, an investigation was carried out to figure out the underlying correlation of 5 obtained genes with immune infiltration. It was shown using lollipop graphics that there was a substantial association between them (Figure 7A-7E). Such findings indicated the vital role of each hub gene in the immune microenvironment.
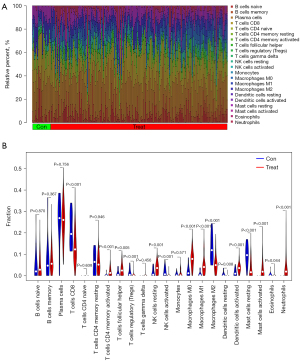
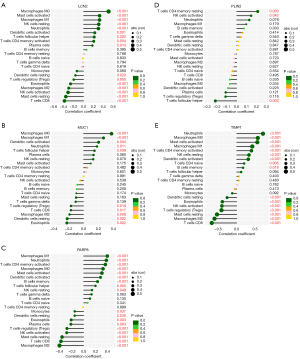
Construction and validation of a diagnostic model with machine learning
The findings indicated that the AUC of the diagnostic model constructed using the logistic algorithm was relatively high compared to other methods. Based on the logistic algorithm, the AUC values were 1.000 and 0.995 for the training and validation cohorts, respectively (Figure 8A,8B) and sensitivity is 0.962, specificity is 1.000 (Table 1). The findings of a sufficient sample indicated that the current model had some referential value for diagnosing UC in clinical practice.
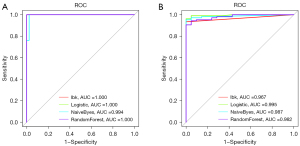
Table 1
Model | AUC | Sensitivity | Specificity |
---|---|---|---|
Ibk | 0.967 | 0.934 | 1.000 |
Logisitic | 0.995 | 0.962 | 1.000 |
NaiveBayes | 0.987 | 0.953 | 1.000 |
RandomForest | 0.982 | 0.906 | 1.000 |
AUC, area under the receiver operating curve.
Discussion
As a chronic inflammatory illness of the colon, UC is characterized by continuous mucosal inflammation of the rectum infiltrating varying intestinal sections. UC symptoms include diarrhea with blood, fecal urgency, and crampy stomach (28). The etiology of UC is currently believed to be connected with intestinal microbe imbalance, immunological response, lifestyle, and genetic vulnerability (29,30). Normal intestinal barrier conditions allow only a limited number of luminal antigens and microorganisms to pass into the lamina propria. Once there are compromised barrier functions or the occurrence of tolerance mechanism failures, numerous immunocytes can cause over-infiltration and chemokine and cytokine generation, leading to worsened inflammation. Such cells infiltrated in are NK T cells (31), macrophages (32), neutrophils (33), T cells (34), dendritic cells (35), and innate lymphoid cells (36). The activated immunocytes can interact through contact by secreting cytokines TNF, IFNg, IL-1b, 6, and 23, among others. An huge class of inflammatory chemicals, chemokines regulate the movement of leukocytes and their activation (37). Ferroptosis represents a kind of programmed cell death associated with iron due to a fatal buildup of lipid hydroperoxides. Ferroptotic damage has been linked to several common immunological disorders, including neuroinflammation, diabetes, asthma, and additional diseases; however, the precise role of ferroptosis in such pathology has not been fully defined (38,39). Immune cells are affected by ferroptosis in 2 distinct ways. One is that it impacts the quantity and function of immune cells. In contrast, immune cells may detect ferroptotic cells and subsequently elicit various inflammatory or specialized responses (40). This work is expected to identify genes shared by immunity and ferroptosis that explain and control this intricate metabolic cycle. Infiltration of immune cells in 184 UC samples with inherent individual proportional variable characteristics. The change in immune cell infiltration rate in 184 UC samples presented intrinsic individual heterogeneity. The infiltration ratios of 15 immunocyte types (T cells CD8, CD4 memory activated, Tregs, and follicular helper, NK cell resting and activated, dendritic cells resting and activated, macrophages M0, M1, and M2, Mast cells resting, and activated, neutrophils, and eosinophils) differed, implying that immunological infiltration is positively linked to the beginning of UC.
The 5 hub genes (LCN2, MUC1, PARP8, PLIN2, and TIMP1) were identified in this research using several analyses, including differential analysis, WGCNA, and the lasso algorithm. The current study indicated that the identified genes might have a tight relationship with UC. It provides a foundation for understanding the etiology of UC and discovering possible new therapies. MUC1 is a membrane-bound mucus layer glycoprotein released via absorptive cells and intestinal goblets. The expression of the MUC1 protein elevates in the inflamed intestine, which helps to maintain an integral mucosal barrier in the intestine and is a vital factor of UC pathogenesis (24). This gene is capable of regulating cell signal transduction and immunological control. The expression of MUC1 is increased dramatically during the disease development from colitis to cancer. Unfortunately, the exact action mechanism remains unknown (41). Recent research indicates that MUC1 is associated with ferroptosis (42,43). The expression of MUC1 has also indicated an increase in UC, which may be associated with ferroptosis (44). However, further study is required since no increase in MUC1 expression has been reported in animal trials. TIMP1 has been identified as a key contender in colorectal cancer promotion associated with UC (45). TIMP1, an inducible form, is a member of a four-member glycoprotein family that mediates extracellular matrix turnover (46). TIMP1 plays a role in inhibiting the angiogenic activity of macrophages by creating a particular complex with MMP9 (47). TIMP1 and MMP9 interactions are of great significance in colorectal cancer occurrence and progression associated with UC (48,49). Lipocalin-2 (LCN2) is a secreted glycoprotein and belongs to the lipocalin superfamily, containing multiple kinds of cells, myeloid cells, and intestinal epithelial cells. This is relevant to inflammatory bowel disease (50). LCN2 can be triggered by diverse proinflammatory stimuli, IL-1 and IL-22, and the activation of Toll-like receptors (51), and a large amount can be released into the gut lumen (52). When bacterial siderophores loaded with iron are blocked, the 25 kD protein works as an antimicrobial peptide, and luminal LCN2 can effectively control gut inflammation and microbial composition in humans (53). Serum LCN2, which is present in conjunction with MMP-9, coincides with endoscopic activity in both Crohn’s disease and UC, as revealed by De Bruyn (54). This is the case for both conditions. LCN2 is a potential candidate for molecular inflammation as it has been shown to have chronic mucosal overexpression, even though endoscopic and histological repair has been performed (55).
The ineffectiveness of UC diagnosis may be attributed partly to the absence of accurate markers and feasible prediction models (56,57). Machine learning is relevant to clinical prediction and beneficial for the clinical diagnosis of UC patients (10). In study of UC, logistic regression is acknowledged as an extensively applied machine learning technique (58). The use of machine learning can realize gene grouping of the entire blood sample, which helps identify UC prognostic indicators (43). The mathematical model established based on the data of histology, and endoscopy is employed to enhance the UC diagnostic effectiveness. Utilizing NaiveBeyas, Logistic, Ibk, and RandomForest algorithms, we analyzed ROC curves to develop a disease diagnostic model on the basis of previously stated key genes. After analysis, the AUC value for the training cohort was 1.000 and 0.995 for the validation cohorts compared to different algorithms. The results indicated that the currently established model had some referential value for the clinical diagnosis of UC. This study lacks further cell experiments and animal experiments. The putative ferroptosis-related genes in this study need further verification with larger samples, and the support of previous studies also needs to be verified by laboratory experiments. The genes associated with ferroptosis were obtained from FerrDb, which is updated regularly, and more genes are yet to be discovered.
Conclusions
We found 5 hub genes (LCN2, MUC1, PARP8, PLIN2, and TIMP1) that were positively correlated with ferroptosis in UC, allowing us to distinguish UC patients from control participants. By detecting the expression of some genes, the current model can be used to direct the diagnostic process for UC individuals. These hub genes could also help us understand how UC develops and how drugs can be applied to treat this disease.
Acknowledgments
We appreciate the work of the researchers and the study participants.
Funding: None.
Footnote
Reporting Checklist: The authors have completed the TRIPOD reporting checklist. Available at https://atm.amegroups.com/article/view/10.21037/atm-23-276/rc
Peer Review File: Available at https://atm.amegroups.com/article/view/10.21037/atm-23-276/prf
Conflicts of Interest: All authors have completed the ICMJE uniform disclosure form (available at https://atm.amegroups.com/article/view/10.21037/atm-23-276/coif). The authors have no conflicts of interest to declare.
Ethical Statement: The authors are accountable for all aspects of the work in ensuring that questions related to the accuracy or integrity of any part of the work are appropriately investigated and resolved. The study was conducted in accordance with the Declaration of Helsinki (as revised in 2013).
Open Access Statement: This is an Open Access article distributed in accordance with the Creative Commons Attribution-NonCommercial-NoDerivs 4.0 International License (CC BY-NC-ND 4.0), which permits the non-commercial replication and distribution of the article with the strict proviso that no changes or edits are made and the original work is properly cited (including links to both the formal publication through the relevant DOI and the license). See: https://creativecommons.org/licenses/by-nc-nd/4.0/.
References
- Adams SM, Close ED, Shreenath AP. Ulcerative Colitis: Rapid Evidence Review. Am Fam Physician 2022;105:406-11.
- Ordás I, Eckmann L, Talamini M, et al. Ulcerative colitis. Lancet 2012;380:1606-19. [Crossref] [PubMed]
- Park J, Cheon JH. Incidence and Prevalence of Inflammatory Bowel Disease across Asia. Yonsei Med J 2021;62:99-108. [Crossref] [PubMed]
- Du L, Ha C. Epidemiology and Pathogenesis of Ulcerative Colitis. Gastroenterol Clin North Am 2020;49:643-54. [Crossref] [PubMed]
- Spinelli A, Bonovas S, Burisch J, et al. ECCO Guidelines on Therapeutics in Ulcerative Colitis: Surgical Treatment. J Crohns Colitis 2022;16:179-89. [Crossref] [PubMed]
- Nakase H, Uchino M, Shinzaki S, et al. Evidence-based clinical practice guidelines for inflammatory bowel disease 2020. J Gastroenterol 2021;56:489-526. [Crossref] [PubMed]
- Lamb CA, Kennedy NA, Raine T, et al. British Society of Gastroenterology consensus guidelines on the management of inflammatory bowel disease in adults. Gut 2019;68:s1-s106. [Crossref] [PubMed]
- Pravda J. Can ulcerative colitis be cured? Discov Med 2019;27:197-200.
- Xu M, Kong Y, Chen N, et al. Identification of Immune-Related Gene Signature and Prediction of CeRNA Network in Active Ulcerative Colitis. Front Immunol 2022;13:855645. [Crossref] [PubMed]
- Lu J, Wang Z, Maimaiti M, et al. Identification of diagnostic signatures in ulcerative colitis patients via bioinformatic analysis integrated with machine learning. Hum Cell 2022;35:179-88. [Crossref] [PubMed]
- Mou Y, Wang J, Wu J, et al. Ferroptosis, a new form of cell death: opportunities and challenges in cancer. J Hematol Oncol 2019;12:34. [Crossref] [PubMed]
- Lei G, Zhuang L, Gan B. Targeting ferroptosis as a vulnerability in cancer. Nat Rev Cancer 2022;22:381-96. [Crossref] [PubMed]
- Dixon SJ, Lemberg KM, Lamprecht MR, et al. Ferroptosis: an iron-dependent form of nonapoptotic cell death. Cell 2012;149:1060-72. [Crossref] [PubMed]
- Xu M, Tao J, Yang Y, et al. Ferroptosis involves in intestinal epithelial cell death in ulcerative colitis. Cell Death Dis 2020;11:86. [Crossref] [PubMed]
- Huang F, Zhang S, Li X, et al. STAT3-mediated ferroptosis is involved in ulcerative colitis. Free Radic Biol Med 2022;188:375-85. [Crossref] [PubMed]
- Millar AD, Rampton DS, Blake DR. Effects of iron and iron chelation in vitro on mucosal oxidant activity in ulcerative colitis. Aliment Pharmacol Ther 2000;14:1163-8. [Crossref] [PubMed]
- Minaiyan M, Mostaghel E, Mahzouni P. Preventive Therapy of Experimental Colitis with Selected iron Chelators and Anti-oxidants. Int J Prev Med 2012;3:S162-9.
- Seril DN, Liao J, Ho KL, et al. Dietary iron supplementation enhances DSS-induced colitis and associated colorectal carcinoma development in mice. Dig Dis Sci 2002;47:1266-78. [Crossref] [PubMed]
- Kobayashi Y, Ohfuji S, Kondo K, et al. Association between dietary iron and zinc intake and development of ulcerative colitis: A case-control study in Japan. J Gastroenterol Hepatol 2019;34:1703-10. [Crossref] [PubMed]
- Yang BY, Zhao MS, Shi MJ, et al. Establishment of a novel prognostic prediction model through bioinformatics analysis for prostate cancer based on ferroptosis-related genes and its application in immune cell infiltration. Transl Androl Urol 2022;11:1130-47. [Crossref] [PubMed]
- Wang L, Chen Y, Zhao J, et al. Analysis and prediction model of ferroptosis related genes in breast cancer. Transl Cancer Res 2022;11:1970-76. [Crossref] [PubMed]
- Yang X, Yin F, Liu Q, et al. Ferroptosis-related genes identify tumor immune microenvironment characterization for the prediction of prognosis in cervical cancer. Ann Transl Med 2022;10:123. [Crossref] [PubMed]
- Li K, Strauss R, Ouahed J, et al. Molecular Comparison of Adult and Pediatric Ulcerative Colitis Indicates Broad Similarity of Molecular Pathways in Disease Tissue. J Pediatr Gastroenterol Nutr 2018;67:45-52. [Crossref] [PubMed]
- Vancamelbeke M, Vanuytsel T, Farré R, et al. Genetic and Transcriptomic Bases of Intestinal Epithelial Barrier Dysfunction in Inflammatory Bowel Disease. Inflamm Bowel Dis 2017;23:1718-29. [Crossref] [PubMed]
- Langfelder P, Horvath S. WGCNA: an R package for weighted correlation network analysis. BMC Bioinformatics 2008;9:559. [Crossref] [PubMed]
- Zhou N, Bao J. FerrDb: a manually curated resource for regulators and markers of ferroptosis and ferroptosis-disease associations. Database (Oxford) 2020;2020:baaa021. [Crossref] [PubMed]
- Mallick H, Yi N. A New Bayesian Lasso. Stat Interface 2014;7:571-82. [Crossref] [PubMed]
- Azam TU, Shadid HR, Blakely P, et al. Soluble Urokinase Receptor (SuPAR) in COVID-19-Related AKI. J Am Soc Nephrol 2020;31:2725-35. [Crossref] [PubMed]
- Gao X, Li J, Pang X, et al. Animal Models and Pathogenesis of Ulcerative Colitis. Comput Math Methods Med 2022;2022:5927384. [Crossref] [PubMed]
- Bullard BM, VanderVeen BN, McDonald SJ, et al. Cross talk between the gut microbiome and host immune response in ulcerative colitis: nonpharmacological strategies to improve homeostasis. Am J Physiol Gastrointest Liver Physiol 2022;323:G554-61. [Crossref] [PubMed]
- Fuss IJ, Heller F, Boirivant M, et al. Nonclassical CD1d-restricted NK T cells that produce IL-13 characterize an atypical Th2 response in ulcerative colitis. J Clin Invest 2004;113:1490-7. [Crossref] [PubMed]
- Lissner D, Schumann M, Batra A, et al. Monocyte and M1 Macrophage-induced Barrier Defect Contributes to Chronic Intestinal Inflammation in IBD. Inflamm Bowel Dis 2015;21:1297-305. [Crossref] [PubMed]
- Hanai H, Takeuchi K, Iida T, et al. Relationship between fecal calprotectin, intestinal inflammation, and peripheral blood neutrophils in patients with active ulcerative colitis. Dig Dis Sci 2004;49:1438-43. [Crossref] [PubMed]
- Heller F, Florian P, Bojarski C, et al. Interleukin-13 is the key effector Th2 cytokine in ulcerative colitis that affects epithelial tight junctions, apoptosis, and cell restitution. Gastroenterology 2005;129:550-64. [Crossref] [PubMed]
- Hart AL, Al-Hassi HO, Rigby RJ, et al. Characteristics of intestinal dendritic cells in inflammatory bowel diseases. Gastroenterology 2005;129:50-65. [Crossref] [PubMed]
- Buonocore S, Ahern PP, Uhlig HH, et al. Innate lymphoid cells drive interleukin-23-dependent innate intestinal pathology. Nature 2010;464:1371-5. [Crossref] [PubMed]
- Torres J, Danese S, Colombel JF. New therapeutic avenues in ulcerative colitis: thinking out of the box. Gut 2013;62:1642-52. [Crossref] [PubMed]
- Tang D, Chen X, Kang R, et al. Ferroptosis: molecular mechanisms and health implications. Cell Res 2021;31:107-25. [Crossref] [PubMed]
- Jiang X, Stockwell BR, Conrad M. Ferroptosis: mechanisms, biology and role in disease. Nat Rev Mol Cell Biol 2021;22:266-82. [Crossref] [PubMed]
- Luo X, Gong HB, Gao HY, et al. Oxygenated phosphatidylethanolamine navigates phagocytosis of ferroptotic cells by interacting with TLR2. Cell Death Differ 2021;28:1971-89. [Crossref] [PubMed]
- Sun Y, Fan L, Mian W, et al. Modified apple polysaccharide influences MUC-1 expression to prevent ICR mice from colitis-associated carcinogenesis. Int J Biol Macromol 2018;120:1387-95. [Crossref] [PubMed]
- Hasegawa M, Takahashi H, Rajabi H, et al. Functional interactions of the cystine/glutamate antiporter, CD44v and MUC1-C oncoprotein in triple-negative breast cancer cells. Oncotarget 2016;7:11756-69. [Crossref] [PubMed]
- Cui DJ, Chen C, Yuan WQ, et al. Integrative analysis of ferroptosis-related genes in ulcerative colitis. J Int Med Res 2021;49:3000605211042975. [Crossref] [PubMed]
- Qing L, Li Q, Dong Z. MUC1: An emerging target in cancer treatment and diagnosis. Bull Cancer 2022;109:1202-16. [Crossref] [PubMed]
- Ma B, Ueda H, Okamoto K, et al. TIMP1 promotes cell proliferation and invasion capability of right-sided colon cancers via the FAK/Akt signaling pathway. Cancer Sci 2022;113:4244-57. [Crossref] [PubMed]
- Gardner J, Ghorpade A. Tissue inhibitor of metalloproteinase (TIMP)-1: the TIMPed balance of matrix metalloproteinases in the central nervous system. J Neurosci Res 2003;74:801-6. [Crossref] [PubMed]
- Mook OR, Frederiks WM, Van Noorden CJ. The role of gelatinases in colorectal cancer progression and metastasis. Biochim Biophys Acta 2004;1705:69-89.
- Huang R, Wang K, Gao L, et al. TIMP1 Is A Potential Key Gene Associated With The Pathogenesis And Prognosis Of Ulcerative Colitis-Associated Colorectal Cancer. Onco Targets Ther 2019;12:8895-904. [Crossref] [PubMed]
- Eiro N, Barreiro-Alonso E, Fraile M, et al. Expression of MMP-2, MMP-7, MMP-9, and TIMP-1 by Inflamed Mucosa in the Initial Diagnosis of Ulcerative Colitis as a Response Marker for Conventional Medical Treatment. Pathobiology 2022; Epub ahead of print. [Crossref]
- Kou F, Cheng Y, Shi L, et al. LCN2 as a Potential Diagnostic Biomarker for Ulcerative Colitis-Associated Carcinogenesis Related to Disease Duration. Front Oncol 2021;11:793760. [Crossref] [PubMed]
- Behnsen J, Jellbauer S, Wong CP, et al. The cytokine IL-22 promotes pathogen colonization by suppressing related commensal bacteria. Immunity 2014;40:262-73. [Crossref] [PubMed]
- Nielsen BS, Borregaard N, Bundgaard JR, et al. Induction of NGAL synthesis in epithelial cells of human colorectal neoplasia and inflammatory bowel diseases. Gut 1996;38:414-20. [Crossref] [PubMed]
- Xiao X, Yeoh BS, Vijay-Kumar M. Lipocalin 2: An Emerging Player in Iron Homeostasis and Inflammation. Annu Rev Nutr 2017;37:103-30. [Crossref] [PubMed]
- de Bruyn M, Arijs I, Wollants WJ, et al. Neutrophil gelatinase B-associated lipocalin and matrix metalloproteinase-9 complex as a surrogate serum marker of mucosal healing in ulcerative colitis. Inflamm Bowel Dis 2014;20:1198-207. [Crossref] [PubMed]
- Zollner A, Schmiderer A, Reider SJ, et al. Faecal Biomarkers in Inflammatory Bowel Diseases: Calprotectin Versus Lipocalin-2-a Comparative Study. J Crohns Colitis 2021;15:43-54. [Crossref] [PubMed]
- Kaenkumchorn T, Wahbeh G. Ulcerative Colitis: Making the Diagnosis. Gastroenterol Clin North Am 2020;49:655-69. [Crossref] [PubMed]
- Kucharzik T, Koletzko S, Kannengiesser K, et al. Ulcerative Colitis-Diagnostic and Therapeutic Algorithms. Dtsch Arztebl Int 2020;117:564-74. [Crossref] [PubMed]
- Zhang J, Wang X, Xu L, et al. Investigation of Potential Genetic Biomarkers and Molecular Mechanism of Ulcerative Colitis Utilizing Bioinformatics Analysis. Biomed Res Int 2020;2020:4921387. [Crossref] [PubMed]
(English Language Editor: C. Mullens)