Investigation of hub ferroptosis-related genes and the immune landscape in recurrent pregnancy loss and unexplained infertility using bioinformatics analysis
Highlight box
Key findings
• Ferroptosis-related genes may disrupt endometrial functions and signaling pathways and lead to the occurrence of RPL and UI.
What is known and what is new?
• Ferroptosis and immunity are closely related to the normal physiological function of the endometrium and may play a role in the pathogenesis of RPL and UI.
• This research provides novel potential ferroptosis-related biomarkers for the diagnosis and treatment of RPL and UI.
What is the implication, and what should change now?
• Ferroptosis and immunity are closely related to the occurrence of RPL and UI. On this basis, we seek novel measures for the treatment and diagnosis of RPL and UI.
Introduction
The endometrium plays an important role in the reproduction and continuation of human species. Estrogen and progesterone levels regulate the endometrium, which undergoes dynamic functional changes throughout the menstrual cycle (1,2). However, abnormal endometrial function leads to miscarriage or infertility. For example, a thin endometrium is a critical cause of unexplained infertility (UI), recurrent pregnancy loss (RPL), and placental abnormalities (3). RPL is defined as two or more clinically confirmed pregnancy failures before 20–24 weeks of pregnancy, including embryo and fetal loss, and the incidence rate among women suitable for pregnancy is approximately 2.5% (4,5). UI is another pregnancy disorder and is defined as women with normal follicle-stimulating hormone (FSH), luteinizing hormone (LH), estradiol (E2), prolactin (PRL), and thyroid stimulating hormone (TSH) levels, normal uterine cavity shape and size, and bilateral tubal patency on a hysterosalpingogram, and their partners have normal spermiogram results (6). UI represents up to 30% of all cases of infertility (7). RPL and UI are diagnosed when current diagnostic methods do provide an explanation, and there is no clear cause. These diseases have a significant impact on women’s physical and mental health and the social economy.
Due to an insufficient understanding of the pathogenesis of RPL and UI, treatment is challenging. The pathogenesis of RPL and UI is multifactorial, and recurring fetal chromosomal abnormalities, the presence of antiphospholipid antibodies, and clinical thyroid disorders are considered risk factors for RPL and UI (8,9). However, the endometrium is a critical factor in RPL and UI because it receives embryos (10,11). Pirtea et al. reviewed the endometrial causes of recurrent pregnancy losses, including endometriosis, adenomyosis, and chronic endometritis (12). Therefore, abnormalities in the physiological function or anatomy of the endometrium may lead to pregnancy disorders. The endometrium has a physiological mechanism, programmed cell death. The mechanism of endometrial programmed cell death is not clear, but it is related to periodic changes in ovarian nest hormones (13). Programmed cell death is the orderly death of cells autonomously controlled by gene programming and includes ferroptosis, cuproptosis, autophagy, pyroptosis and apoptosis (14-17). Few studies investigated the relationship between ferroptosis and the endometrium. Therefore, we focused on the relationship between ferroptosis and endometrium-related infertility and miscarriage.
Ferroptosis is a new type of programmed cell death, and its main mechanism is under the action of divalent iron or ester oxygenase, which catalyze the high expression of unsaturated fatty acids on the cell membrane and results in lipid peroxidation and cell death (18-20). Many studies have shown that ferroptosis is closely related to the prognosis of many kinds of tumors. For example, Ma et al. created an ferroptosis-related prognostic signature that can accurately predict the prognosis of patients with lung cancer (21); He et al. comprehensively analyzed the relationship between sideroptosis and tumor immune infiltration and cell mutation in breast cancer, and divided breast cancer into two different types, which provided a new idea for immunotherapy of breast cancer patients (22). Recent findings suggest that ferroptosis is closely related to endometrial-related infertility and miscarriage. Ni et al. revealed that iron-overloaded follicular fluid increased the risk of endometriosis-related infertility (23). Hu et al. indicated that an increase in uterine and placental iron prolapse was related to oxidative stress-induced fetal loss (24). Li et al. demonstrated that iron overload in endometriosis disrupted blastocyst formation, decreased glutathione peroxidase 4 (GPX4) expression and induced lipid peroxidation in mice, which suggests that iron overload causes embryotoxicity and induces ferroptosis (25). This evidence suggests that ferroptosis is associated with abnormalities in endometrial function and embryos, which lead to infertility or miscarriage.
Gene microarray sequencing technology has introduced great resource sharing and convenience to many scientific researchers. We can use the Gene Expression Omnibus (GEO) database to mine a large amount of data for preliminary screening and analyses to prepare for subsequent research. The present study downloaded GSE165004 from the GEO database to compare the differentially expressed ferroptosis-related genes (DE-FRGs) in healthy, RPL and UI endometrial samples and identify hub DE-FRGs to provide potential biomarkers for the diagnosis and treatment of RPL and UI. We present the following article in accordance with the STREGA reporting checklist (available at https://atm.amegroups.com/article/view/10.21037/atm-23-97/rc).
Methods
Raw data download
We downloaded the GSE165004 dataset from the Gene Expression Omnibus (GEO) database, which contained the transcriptome data of 72 endometrial samples, including 24 healthy controls. There were 24 samples of recurrent pregnancy loss (women under 35 years of age who lost at least two consecutive pregnancies in a regular menstrual cycle of 20 weeks or less) and 24 samples of unexplained infertility (women under 35 years of age in a regular menstrual cycle with normal levels of FSH, LH, E2, PRL and TSH on the second to third days, normal shape and size of the uterine cavity, and unobstructed bilateral fallopian tubes on hysterosalpingography). We downloaded a list of ferroptosis-related genes from the Zhounan website (http://www.zhounan.org/ferrdb/current/). The study was performed in accordance with the Declaration of Helsinki (as revised in 2013).
Extraction of FRGs and analysis of differentially expressed ferroptosis-related genes (DE-FRGs)
The GSE165004 dataset was standardized using the normalizeBetweenArrays function of R statistical analysis software (https://cran.r-project.org/), and 409 FRGs were extracted. The differential expression of FRGs in healthy controls and RPL and UI was analyzed using the limma package, and the log2-fold change (logFC) >0 and a false discovery rate (FDR) <0.05 were regarded as DE-FRGs. A heatmap and volcano map of DEGs were created in the ggplot2 package.
Screening of disease characteristic genes in DE-FRGs
We used the least absolute shrinkage and selection operator (LASSO) algorithm and support vector machine-recursive feature elimination (SVM-RFE) machine learning algorithm in the glmnet package to screen for disease (RPL and UI) characteristic genes.
Construction of the protein-protein interaction network
We constructed a PPI network of DE-FRGs using the STRING (https://cn.string-db.org/) online website, and the cytoHubba plug-in in Cytoscape software was used to identify the top 10 maximum margin criterion (MMC) proteins.
Screening, expression and ROC curve construction of hub DE-FRGs
We obtained the hub DE-FRGs from the intersection of the top 10 genes in the PPI network and the disease characteristic genes identified using LASSO regression and SVM-RFE. The expression of the hub DE-FRGs between the healthy control group and the patients with RPL and UI is displayed using a box plot. The pROC package was used to draw the ROC curve for the diagnosis of RPL and UI via single genes and multiple genes of hub DE-FRGs.
GO function enrichment analysis and GSEA function enrichment analysis of DEGs
GO is a database set up by the Gene Ontology Consortium, which aims to define and describe the functions of genes and proteins for a variety of species. We used the clusterProfiler package for GO functional enrichment analysis of DE-FRGs. GSEA is a computational method used to assess whether there is a statistically significant and consistent difference between two biological data sets in a preset gene set. We used the clusterProfiler package to analyze GSEA gene functional enrichment in hub DE-FRGs (grouped by median expression level).
Analysis of immune cell infiltration in the endometrium of RPL and UI
The CIBERSORT algorithm was used to calculate the relative content of 22 types of immune cells in each of the 72 samples and analyze the correlation between the expression of hub DE-FRGs and the content of immune cells. Differences in the expression of immune cell content between healthy controls and RPL and UI were also analyzed.
Statistical analysis
All statistical methods were performed in R software. The limma package was used for differential gene expression analysis. LogFC >0 and a FDR <0.05 were regarded as statistically significant differences. The wilcox.test function was used for comparisons between groups. Correlations were analyzed using Spearman’s correlation. P<0.05 was considered a significant difference.
Results
DE-FRGs in RPL and UI
Integrated analysis of the list of ferroptosis-related genes and the GSE165004 dataset extracted 409 FRGs. The results of the difference analysis showed 36 up-regulated DE-FRGs and 32 down-regulated DE-FRGs in the RPL and UI groups compared to the healthy group (Figure 1A,1B).
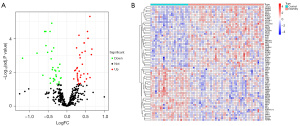
Screening of hub DE-FRGs in RPL and UI
Twenty-one genes were screened using the LASSO regression algorithm (Figure 2A,2B), and 17 genes were screened using the SVM-RFE algorithm (Figure 2C,2D). The LASSO genes and SVM-RFE genes were intersected with the top 10 MMC proteins of the PPI network to obtain 5 hub DE-FRGs, SRC, KRAS, RELA, MAPK1, and STK11 (Figure 3A,3B).
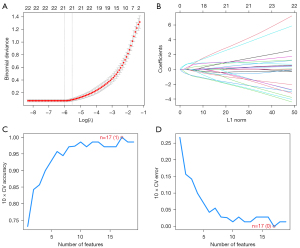
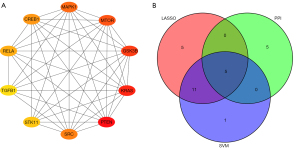
The diagnostic value of hub DE-FRGs in RPL and UI patients (ROC curve)
At the single-gene expression level, the AUC values of the ROC curve for RPL and UI patient diagnosis were KRAS =0.908, MAPK1 =0.811, RELA =0.760, SRC =0.849, and STK11 =0.709 (Figure 4A).
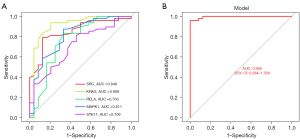
For the multigene expression model, the AUC value of the ROC curve for RPL and UI patient diagnosis was 0.996 (95% CI: 0.984–1.000) (Figure 4B).
The expression levels of 5 hub DE-FRGs in healthy controls and RPL and UI patients
Among the 5 hub DE-FRGs, KRAS, RELA and SRC were expressed at low levels in RPL and UI patients, and MAPK1 and STK11 were expressed at high levels in RPL and UI patients (Figure 5A-5E).
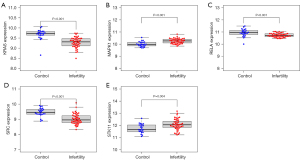
GO and GSEA functional enrichment analysis of DE-FRGs
The GO functional enrichment analysis results of DE-FRGs indicated that striated muscle tissue development, muscle tissue development, positive regulation of catabolic process, cardiac muscle tissue development, regulation of cardiac muscle tissue growth, regulation of heart growth, cardiac muscle tissue growth, heart growth, regulation of organ growth and response to fluid shear stress were the top 10 biological processes (BPs). Transcription regulator complex, focal adhesion, cell-substrate junction, RNA polymerase II transcription regulator complex, cytoplasmic side of membrane, mitochondrial outer membrane, organelle outer membrane, outer membrane, PML nuclear body scaffold (PML body) and CREB regulated transcription coactivator 2 (TORC2) complex were the top 10 cell components (CC). DNA-binding transcription factor binding, protein serine/threonine kinase activity, RNA polymerase II-specific DNA-binding transcription factor binding, phosphoprotein binding, activating transcription factor binding, transforming growth factor beta receptor binding, transcription coactivator binding, wide pore channel activity, disordered domain specific binding and NADP binding were the top 10 molecular functions (MFs) (Figure 6A,6B).
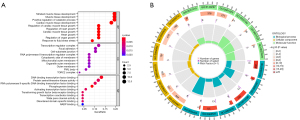
GSEA functional enrichment analysis results indicated that the cytokine-cytokine receptor interaction signaling pathway was the common pathway for hub DE-FRGs (Figure 7A-7E).
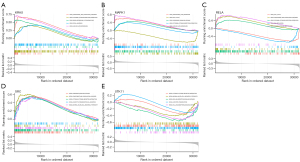
Immune cell infiltration in healthy controls and RPL and UI patients
Immune cell infiltration analysis showed that T follicular helper cells had high infiltration in RPL and UI patients compared to healthy samples, and M1 and M2 macrophages had low infiltration in RPL and UI patients (Figure 8A).
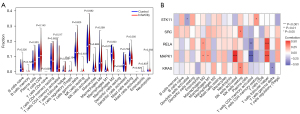
Correlation analysis of DE-FRGs and immune cells showed that the MAPK1 expression level positively correlated with T follicular helper cell and activated NK cell infiltration but negatively correlated with CD4 T memory activated cells. The expression of RELA positively correlated with T follicular helper cells (Figure 8B).
Discussion
Miscarriage and infertility are two major problems that continue to affect the physical and mental health of young women. An estimated 23 million miscarriages occur annually worldwide, which translates to 44 pregnancy losses each minute (5). Two of the more intractable problems in miscarriage and infertility are recurrent pregnancy loss and unexplained infertility, and endometrial factors are one cause of these pregnancy disorders (26). To understand the differences between the endometrial transcripts in RPL and UI and healthy controls, we downloaded the GSE165004 dataset from the GEO database and investigated differences in ferroptosis-related genes between RPL, UI and healthy controls to identify key biomarkers.
The present study screened 68 DE-FRGs between the RPL and UI groups and the healthy group. Five hub DE-FRGs were identified via the intersection of the LASSO algorithm, the SVM-RFE algorithm and the PPI network. We performed GO functional enrichment analysis on 68 DE-FRGs, and the results indicated that these DE-FRGs were primarily enriched in the following biological processes: striated muscle tissue development, muscle tissue development, positive regulation of catabolic process, cardiac muscle tissue development, regulation of cardiac muscle tissue growth, regulation of heart growth, cardiac muscle tissue growth, heart growth, regulation of organ growth and response to fluid shear stress. DE-FRGs were primarily concentrated in the growth and development of muscle tissue, the heart and organs. Perez-Garcia et al. showed that defects in heart development led to fetal miscarriage in mice, which is consistent with our findings (27). A study from the University of Leeds showed that the main structure of the fetal heart formed in just four days of pregnancy in many cases of miscarriage and abnormal fetal heart development. One in 10 miscarriages is likely caused by abnormal fetal heart development (28). This new discovery provides a better understanding of the causes of miscarriage. Our GSEA functional enrichment analysis found that the cytokine-cytokine receptor interaction signaling pathway was the common pathway of the hub DE-FRGs. Several studies showed that the cytokine-cytokine receptor interaction signaling pathway was a common pathogenic mechanism of miscarriage, spontaneous preterm delivery and infertility (29-33). Therefore, the dysregulation of gene expression leads to the dysfunction of biological processes and signaling pathway functions, which lead to the abnormal execution of normal physiological functions of the endometrium. These alterations may lead to pregnancy disorders, such as miscarriage or infertility.
The journal Cell confirmed that ferroptosis was an iron-dependent form of nonapoptotic cell death in 2012 (34). Since 2019, some studies showed that endometriosis was closely related to ferroptosis (35-37). We identified 5 hub DE-FRGs in the present study. Among the 5 hub biomarkers, KRAS, RELA and SRC were expressed at low levels in RPL and UI patients, and MAPK1 and STK11 were expressed at high levels in RPL and UI patients. Inoue et al. showed that mutation of the ferroptosis gene KRAS produced an approximately 11% higher incidence of miscarriage than wild type (38). Skliutė suggested that RELA was highly expressed in the infertile endometrium, which is consistent with our study (39). Wieser et al. suggested that SRC played a role in the regulation of human endometrial remodeling (40). Kaczynski et al. indicated that prostaglandins stimulated the MAPK1/3 pathway in endometrial luminal epithelial cells and participated in the embryo-maternal implantation process (41). However, there is no relevant study on STK11 and infertility and miscarriage. One study showed that the ferroptosis-related NLR family pyrin domain containing 1 (NLRP1) inflammasome led to adverse pregnancy outcomes (42). Ni et al. revealed that iron-overloaded follicular fluid increased the risk of endometriosis-related infertility (23). Hu et al. indicated that an increase in uterine and placental iron prolapse was related to oxidative stress-induced fetal loss (24). Li et al. showed that iron overload in endometriosis disrupted blastocyst formation, decreased glutathione peroxidase 4 (GPX4) expression and induced lipid peroxidation in mice, which suggest that iron overload causes embryotoxicity and induces ferroptosis (25). The results of a study show that the microenvironment of hyperandrogenemia and insulin resistance can regulate the iron prolapse of pregnancy uterus and placenta (43). However, there are no studies on the role of ferroptosis-related genes in predicting the therapeutic effect and drug sensitivity of RPL and UI patients. Therefore, the five hub DE-FRGs we screened were likely to cause recurrent pregnancy loss and unexplained infertility due to dysregulation and mediation of endometrial dysfunction. These biomarkers may be used as molecular markers for the diagnosis and treatment of RPL and UI in the future.
We also investigated differences in endometrial immune cell infiltration between healthy controls and RPL and UI patients and the relationship between hub biomarkers and immune cell infiltration. Our results indicated that T follicular helper cells had high infiltration in RPL and UI patients, and M1 and M2 macrophages had low infiltration in RPL and UI patients. The MAPK1 expression level positively correlated with T follicular helper cell and activated NK cell infiltration but negatively correlated with CD4 T memory activated cells. The expression of RELA positively correlated with T follicular helper cells. Recent findings suggest that embryo implantation is an inflammatory response (44-46). Many types of immune cells play a crucial role in embryo implantation and placenta formation, including macrophages, T cells, natural killer cells, monocytes, mast cells, dendritic cells, B cells and neutrophils. Many cytokines have been identified in the implant site (39,47,48). During pregnancy, various immune effectors and molecules involved in the immune microenvironment establish specific maternal tolerance to the semiallogeneic fetus (49). For example, Th1 immunity favors invading trophoblasts rather than harming it, and Treg and Th9 cells regulate local inflammatory immune responses that may be harmful to the fetus (49). Therefore, the dysregulation of endometrial immune cell infiltration caused by inflammation will affect the implantation and growth of the embryo and lead to infertility, miscarriage and other pregnancy disorders. Some studies showed that people with recurrent implantation failure and recurrent pregnancy loss may be treated with immune cells (e.g., peripheral blood mononuclear cells, platelet-rich plasma and subcutaneous granulocyte colony-stimulating factor) or immunomodulators (e.g., paternal leukocyte immunity, intravenous immunoglobulin (IVIg), lipolactone and fagastine) (50,51). These effective immunotherapies demonstrated that miscarriage and infertility were closely related to endometrial immune function. Our results provide novel ideas for immunotherapy of RPL and UI. However, our research still lacks the cellular function of iron death or animal experiments in vivo and in vitro, and then we will start to carry out related experimental studies.
Conclusions
The present study identified five hub ferroptosis-related genes using bioinformatics analysis that may cause dysfunction of endometrial functions and signaling pathways and lead to the occurrence of RPL and UI. This research provides potential biomarkers for the diagnosis and treatment of RPL and UI.
Acknowledgments
Funding: This work was supported by the following funding: Improving the Scientific Research Basic Ability of Young and Middle-Aged Teachers in Guangxi Universities in 2022 (No. 2022KY0551); Young and Middle-Aged Backbone Talent Project of Affiliated Hospital of Youjiang Medical College for Ethnic Minorities (No. Y20212605); the Third Batch of Self-Funded Projects of Baise Scientific Research and Technology Development Plan in 2022, Encyclopedia (No. 20222931).
Footnote
Reporting Checklist: The authors have completed the STREGA reporting checklist. Available at https://atm.amegroups.com/article/view/10.21037/atm-23-97/rc
Peer Review File: Available at https://atm.amegroups.com/article/view/10.21037/atm-23-97/prf
Conflicts of Interest: All authors have completed the ICMJE uniform disclosure form (available at https://atm.amegroups.com/article/view/10.21037/atm-23-97/coif). The authors have no conflicts of interest to declare.
Ethical Statement: The authors are accountable for all aspects of the work in ensuring that questions related to the accuracy or integrity of any part of the work are appropriately investigated and resolved. The study was performed in accordance with the Declaration of Helsinki (as revised in 2013).
Open Access Statement: This is an Open Access article distributed in accordance with the Creative Commons Attribution-NonCommercial-NoDerivs 4.0 International License (CC BY-NC-ND 4.0), which permits the non-commercial replication and distribution of the article with the strict proviso that no changes or edits are made and the original work is properly cited (including links to both the formal publication through the relevant DOI and the license). See: https://creativecommons.org/licenses/by-nc-nd/4.0/.
References
- Critchley HOD, Maybin JA, Armstrong GM, et al. Physiology of the Endometrium and Regulation of Menstruation. Physiol Rev 2020;100:1149-79. [Crossref] [PubMed]
- Nikolakopoulou K, Turco MY. Investigation of infertility using endometrial organoids. Reproduction 2021;161:R113-27. [Crossref] [PubMed]
- Lv H, Zhao G, Jiang P, et al. Deciphering the endometrial niche of human thin endometrium at single-cell resolution. Proc Natl Acad Sci U S A 2022;119:e2115912119. [Crossref] [PubMed]
- Dimitriadis E, Menkhorst E, Saito S, et al. Recurrent pregnancy loss. Nat Rev Dis Primers 2020;6:98. [Crossref] [PubMed]
- Quenby S, Gallos ID, Dhillon-Smith RK, et al. Miscarriage matters: the epidemiological, physical, psychological, and economic costs of early pregnancy loss. Lancet 2021;397:1658-67. [Crossref] [PubMed]
- Carson SA, Kallen AN. Diagnosis and Management of Infertility: A Review. JAMA 2021;326:65-76. [Crossref] [PubMed]
- Abdallah KS, Hunt S, Abdullah SA, et al. How and Why to Define Unexplained Infertility? Semin Reprod Med 2020;38:55-60. [Crossref] [PubMed]
- Christiansen OB. Special Issue Recurrent Pregnancy Loss: Etiology, Diagnosis, and Therapy. J Clin Med 2021;10:5040. [Crossref] [PubMed]
- O'Mathuna D, Berghella V, Bellussi F, et al. Cochrane Review Summaries-October 2020. Obstet Gynecol 2020;136:844-6. [Crossref] [PubMed]
- Shi Y, Yamada H, Sasagawa Y, et al. Uterine endometrium microbiota and pregnancy outcome in women with recurrent pregnancy loss. J Reprod Immunol 2022;152:103653. [Crossref] [PubMed]
- Carbonnel M, Pirtea P, de Ziegler D, et al. Uterine factors in recurrent pregnancy losses. Fertil Steril 2021;115:538-45. [Crossref] [PubMed]
- Pirtea P, Cicinelli E, De Nola R, et al. Endometrial causes of recurrent pregnancy losses: endometriosis, adenomyosis, and chronic endometritis. Fertil Steril 2021;115:546-60. [Crossref] [PubMed]
- Hung SW, Zhang R, Tan Z, et al. Pharmaceuticals targeting signaling pathways of endometriosis as potential new medical treatment: A review. Med Res Rev 2021;41:2489-564. [Crossref] [PubMed]
- Bertheloot D, Latz E, Franklin BS. Necroptosis, pyroptosis and apoptosis: an intricate game of cell death. Cell Mol Immunol 2021;18:1106-21. [Crossref] [PubMed]
- Tang D, Chen X, Kroemer G. Cuproptosis: a copper-triggered modality of mitochondrial cell death. Cell Res 2022;32:417-8. [Crossref] [PubMed]
- Fang Y, Tian S, Pan Y, et al. Pyroptosis: A new frontier in cancer. Biomed Pharmacother 2020;121:109595. [Crossref] [PubMed]
- Mizushima N, Komatsu M. Autophagy: renovation of cells and tissues. Cell 2011;147:728-41. [Crossref] [PubMed]
- Yang WS, Stockwell BR. Ferroptosis: Death by Lipid Peroxidation. Trends Cell Biol 2016;26:165-76. [Crossref] [PubMed]
- Gao M, Monian P, Quadri N, et al. Glutaminolysis and Transferrin Regulate Ferroptosis. Mol Cell 2015;59:298-308. [Crossref] [PubMed]
- Chen X, Kang R, Kroemer G, et al. Ferroptosis in infection, inflammation, and immunity. J Exp Med 2021;218:e20210518. [Crossref] [PubMed]
- Ma C, Li F, Luo H. Prognostic and immune implications of a novel ferroptosis-related ten-gene signature in lung adenocarcinoma. Ann Transl Med 2021;9:1058. [Crossref] [PubMed]
- He Z, Zhou Z, Wang F, et al. Comprehensive analysis of the relationship between the ferroptosis and tumor-infiltrating immune cells, mutation, and immunotherapy in breast cancer. Ann Transl Med 2022;10:833. [Crossref] [PubMed]
- Ni Z, Li Y, Song D, et al. Iron-overloaded follicular fluid increases the risk of endometriosis-related infertility by triggering granulosa cell ferroptosis and oocyte dysmaturity. Cell Death Dis 2022;13:579. [Crossref] [PubMed]
- Hu M, Zhang Y, Ma S, et al. Suppression of uterine and placental ferroptosis by N-acetylcysteine in a rat model of polycystic ovary syndrome. Mol Hum Reprod 2021;27:gaab067. [Crossref] [PubMed]
- Li S, Zhou Y, Huang Q, et al. Iron overload in endometriosis peritoneal fluid induces early embryo ferroptosis mediated by HMOX1. Cell Death Discov 2021;7:355. [Crossref] [PubMed]
- Agarwal A, Aponte-Mellado A, Premkumar BJ, et al. The effects of oxidative stress on female reproduction: a review. Reprod Biol Endocrinol 2012;10:49. [Crossref] [PubMed]
- Perez-Garcia V, Fineberg E, Wilson R, et al. Placentation defects are highly prevalent in embryonic lethal mouse mutants. Nature 2018;555:463-8. [Crossref] [PubMed]
- Pervolaraki E, Dachtler J, Anderson RA, et al. Ventricular myocardium development and the role of connexins in the human fetal heart. Sci Rep 2017;7:12272. [Crossref] [PubMed]
- Wang P, Pan J, Tian X, et al. Transcriptomics-determined chemokine-cytokine pathway presents a common pathogenic mechanism in pregnancy loss and spontaneous preterm birth. Am J Reprod Immunol 2021;86:e13398. [Crossref] [PubMed]
- Mu Y, Yuan Y, Han W, et al. Exploring the Molecular Mechanism and Biomarker of Recurrent Spontaneous Abortion Based on RNA Sequencing Analysis. Clin Lab 2020; [Crossref] [PubMed]
- Li T, Li X, Guo Y, et al. Distinct mRNA and long non-coding RNA expression profiles of decidual natural killer cells in patients with early missed abortion. FASEB J 2020;34:14264-86. [Crossref] [PubMed]
- Chen L, Ni Z, Cai Z, et al. The Mechanism Exploration of Follicular Fluids on Granulose Cell Apoptosis in Endometriosis-Associated Infertility. Biomed Res Int 2021;2021:6464686. [Crossref] [PubMed]
- Zhang X, Hou J, Wang G, et al. Gonadal Transcriptome Analysis in Sterile Double Haploid Japanese Flounder. PLoS One 2015;10:e0143204. [Crossref] [PubMed]
- Dixon SJ, Lemberg KM, Lamprecht MR, et al. Ferroptosis: an iron-dependent form of nonapoptotic cell death. Cell 2012;149:1060-72. [Crossref] [PubMed]
- Bielfeld AP, Pour SJ, Poschmann G, et al. A Proteome Approach Reveals Differences between Fertile Women and Patients with Repeated Implantation Failure on Endometrial Level-Does hCG Render the Endometrium of RIF Patients?. Int J Mol Sci 2019;20:425. [Crossref] [PubMed]
- Li Y, Zeng X, Lu D, et al. Erastin induces ferroptosis via ferroportin-mediated iron accumulation in endometriosis. Hum Reprod 2021;36:951-64. [Crossref] [PubMed]
- Li G, Lin Y, Zhang Y, et al. Endometrial stromal cell ferroptosis promotes angiogenesis in endometriosis. Cell Death Discov 2022;8:29. [Crossref] [PubMed]
- Inoue S, Yoshida E, Fukui Y, et al. KRAS mutations in uterine endometrium are associated with gravidity and parity. Cell Death Dis 2020;11:347. [Crossref] [PubMed]
- Skliutė G, Baušytė R, Ramašauskaitė D, et al. Characterization of Epigenetic and Molecular Factors in Endometrium of Females with Infertility. Biomedicines 2022;10:1324. [Crossref] [PubMed]
- Wieser F, Schneeberger C, Hudelist G, et al. Endometrial nuclear receptor co-factors SRC-1 and N-CoR are increased in human endometrium during menstruation. Mol Hum Reprod 2002;8:644-50. [Crossref] [PubMed]
- Kaczynski P, Kowalewski MP, Waclawik A. Prostaglandin F2α promotes angiogenesis and embryo-maternal interactions during implantation. Reproduction 2016;151:539-52. [Crossref] [PubMed]
- Meihe L, Shan G, Minchao K, et al. The Ferroptosis-NLRP1 Inflammasome: The Vicious Cycle of an Adverse Pregnancy. Front Cell Dev Biol 2021;9:707959. [Crossref] [PubMed]
- Zhang Y, Hu M, Jia W, et al. Hyperandrogenism and insulin resistance modulate gravid uterine and placental ferroptosis in PCOS-like rats. J Endocrinol 2020;246:247-63. [Crossref] [PubMed]
- Chen P, Zhou L, Chen J, et al. The Immune Atlas of Human Deciduas With Unexplained Recurrent Pregnancy Loss. Front Immunol 2021;12:689019. [Crossref] [PubMed]
- Franasiak JM, Alecsandru D, Forman EJ, et al. A review of the pathophysiology of recurrent implantation failure. Fertil Steril 2021;116:1436-48. [Crossref] [PubMed]
- Li Y, Yu S, Huang C, et al. Evaluation of peripheral and uterine immune status of chronic endometritis in patients with recurrent reproductive failure. Fertil Steril 2020;113:187-196.e1. [Crossref] [PubMed]
- Schumacher A, Zenclussen AC. Human Chorionic Gonadotropin-Mediated Immune Responses That Facilitate Embryo Implantation and Placentation. Front Immunol 2019;10:2896. [Crossref] [PubMed]
- Geisert R, Fazleabas A, Lucy M, et al. Interaction of the conceptus and endometrium to establish pregnancy in mammals: role of interleukin 1β. Cell Tissue Res 2012;349:825-38. [Crossref] [PubMed]
- Wang W, Sung N, Gilman-Sachs A, et al. T Helper (Th) Cell Profiles in Pregnancy and Recurrent Pregnancy Losses: Th1/Th2/Th9/Th17/Th22/Tfh Cells. Front Immunol 2020;11:2025. [Crossref] [PubMed]
- Turocy J, Williams Z. Novel therapeutic options for treatment of recurrent implantation failure. Fertil Steril 2021;116:1449-54. [Crossref] [PubMed]
- Carp H. Immunotherapy for recurrent pregnancy loss. Best Pract Res Clin Obstet Gynaecol 2019;60:77-86. [Crossref] [PubMed]