A cuproptosis-related lncRNAs risk model to predict prognosis and guide immunotherapy for lung adenocarcinoma
Highlight box
Key findings
• A novel cuproptosis-related long noncoding RNAs risk model may be a potential biomarker for lung adenocarcinoma (LUAD).
What is known and what is new?
• Cuproptosis is one of the newest forms of cell death induction, which is considered as a novel target for tumor treatment. LncRNAs has been noted for its diagnostic ability and survival prediction.
• We established and verified a novel cuproptosis-related risk model to forecast prognosis and present new insights for personalized treatment of LUAD.
What is the implication, and what should change now?
• Compared with other clinically conventional markers, cuproptosis-related lncRNAs risk model has better prediction accuracy and closely association with immune response and immunotherapy. However, more experiments and more LUAD samples are required to check the stability and reliability of the risk signature.
Introduction
As the leading cause of cancer death, lung cancer has one of the highest incidence rates among cancers, second only to breast cancer (1,2). As one of the main pathological types of lung cancer, lung adenocarcinoma (LUAD) has significantly increased in incidence over the last 10 years, yet that of lung squamous cell carcinoma has decreased (3,4). Due to the application of targeted therapy and immunotherapy in LUAD, there has been significant progress in cancer therapy (5). However, the overall mortality of LUAD remains high (6).
Long noncoding RNA (lncRNA) is a type of nonprotein-coding RNA of at least 200 nucleotides in length (7). In the recent five years, an increasing amount of research has focused on the role of lncRNAs in different cancers including pancreatic, hepatocellular, colon, and lung (8-12). As a novel cancer biomarker, lncRNAs has been noted for its diagnostic ability and survival prediction. For example, LINC00472 has been shown to inhibit the invasion and migration of LUAD cells by binding to YBX1 (13). Similarly, lncRNA UPLA1 can be a prognostic marker of the progression of LUAD (14). A close relationship has been found between lncRNA and immunotherapy in LUAD. By enhancing the activity of c-Myc, programmed death ligand-1 (PD-L1) can promote the progression of LUAD by generating lncRNAs (15). Cuproptosis is a recently discovered cell death pathway, which is caused by the direct reaction of copper with the fatty acylated components of the tricarboxylic acid (TCA) cycle (16). LUAD is closely related to the TCA cycle (17). When the TCA cycle downregulates energy production, tumor cells can survive despite nutrient deficiency and hypoxia and escape the immune system (18). Utilizing the pathophysiological role of copper, cuproptosis provides a new pathway for anticancer treatments (19). Since cuproptosis has only recently been discovered as a form of cell death, insufficient information is currently available about its effect in LUAD. Therefore, screening of cuproptosis-related lncRNAs in clinical samples may be useful to forecast prognosis and enable direct treatment of LUAD. Diagnosis at an advanced stage, which may lead to the failure in treatment, is one of the main reasons for the poor prognosis of LUAD patients. Since genetic alterations may precede obvious histopathological alterations in cancer detection, the construction of a novel biomarker which can stratify patients with LUAD at an early stage, and direct treatment for LUAD is urgently needed. In recent years, for LUAD and non-small cell lung cancer, constructing new tumor biomarkers is a trend and has achieved significant results (20-23).
Based on The Cancer Genome Atlas (TCGA) database, this study aimed to construct a cuproptosis-related lncRNA (CRL) prognostic model to forecast prognosis of patients with LUAD. The CRL model was analyzed to investigate its latent predictive value and immune-related functions. In addition, we also preliminarily verified the expression of three CRLs in LUAD cell lines and tissues using quantitative polymerase chain reaction (qPCR).
Our findings are helpful to forecasting the prognosis of patients with LUAD and direct clinical chemotherapy and immunotherapy, which may facilitate early diagnosis and increase the overall survival (OS) of patients with LUAD. We present the following article in accordance with the TRIPOD reporting checklist (available at https://atm.amegroups.com/article/view/10.21037/atm-22-3195/rc).
Methods
Data capture and preprocessing
RNA sequence data and mutation data were obtained from TCGA-LUAD data set. Clinical information, including age, gender, stage, and tumor (T), node (N), and metastasis (M) classification, were also downloaded from TCGA-LUAD data set. Unqualified data were deleted. All data were processed and log2 converted uniformly. A total of 16,876 lncRNAs were identified from the TCGA-LUAD datebase. Using the ‘limma’ R package, lncRNAs, which had a significant correlation with cuproptosis-related genes, were identified (|corFilter| >0.4, P<0.001). Data analysis was performed with R software version 4.2.0 (The R Foundation for Statistical Computing, Indianapolis, IN, USA) was used for data analysis.
Construction of the cuproptosis-related prognostic risk model
Combining the survival information of patients with LUAD in the TCGA data set, we used univariate Cox regression analysis of screened cuproptosis-related difflncRNAs. A cuproptosis-related lncRNA model was constructed based on the screened prognostic CRLs. A total of 471 patients with LUAD were randomly and equally divided into the test and training groups. Then, using the R package ‘glmnet’, least absolute shrinkage and selection operator (LASSO) Cox regression analysis was applied, and 60 cuproptosis-related lncRNAs were found to be significantly associated with LUAD OS. Applying multivariate Cox regression analysis, we assessed factors independently related to prognosis and identified 16 cuproptosis-related lncRNAs as prognostic factors. Samples were separated into low- and high-risk groups based on the median risk score.
The risk score was calculated as follows:
where coef is the coefficient and expr is the expression level of lncRNA.
Clinical information analysis and model validation
Using the ‘survminer’ R package, Kaplan-Meier (KM) curves were generated with log rank tests to analyze differences in OS between the high- and low-risk groups. Receiver operating characteristic (ROC) curves (24) were drawn to assess the forecast accuracy of the risk model via the ‘timeROC’ R package and to validate the variables for each group. According to the TCGA database, univariate and multivariate Cox regression analyses were applied using the survival package in R, and risk score, age, gender, stage, and T, N, and M classification were included.
Application of gene set variation analysis (GSVA)
GSVA is a method which has been applied to evaluate transcriptome gene set enrichment (25). With the aim of analyzing the differences in gene set enrichment between high- and low-risk groups, we used GSVA to identify related pathways.
Functional enrichment analysis
Using the ‘edgeR’ R package (26), genes differentially expressed between the high- and low-risk groups were identified (|logFCfilter| >1 and fdrFilter <0.05). Using the ‘clusterProfiler’ package in R (27), Gene Ontology (GO) and the Kyoto Encyclopedia of Genes and Genomes (KEGG) were used based on the identified genes (P value <0.05).
Analysis of cuproptosis-related prognostic signature in immunity
Single sample gene set enrichment analysis (ssGSEA) was used to identify immunological differences in the infiltration of immune cells in tumors between the high- and low-risk groups. The R package ‘corrplot’ was employed to assess the correlation of risk score and immune cells. Then, using potential immune checkpoints analysis, PD-L1 and LAG-3 were found to have statistical significance (P<0.05).
Drug sensitivity prediction
Using the R package ‘pRRophetic’, we explored potential clinical drugs for the treatment of LUAD by predicting the half-maximal inhibitory concentration (IC50) values of the drugs according to data in the TCGA-LUAD database.
LUAD cell culture and tissue sample collection
Human LUAD cell lines A549, HCC827, and H1650 were purchased from the Shanghai Cell Bank of the Chinese Academy of Medical Sciences (CAMS; Beijing, China). Normal human pulmonary epithelial cell lines BEAS-2B were purchased from the American Type Culture Collection (ATCC; Rockville, MD, USA). All cell lines were cultured in a medium consisting of Roswell Park Memorial Institute (RPMI)-1640 (Gibco, Waltham, MA, USA), 10% fetal bovine serum (Gibco), 0.1 mg/mL streptomycin (Gibco), and 100 U/mL penicillin (Gibco) and were maintained at 37 °C at 5% CO2 atmosphere. Some 20 pairs of LUAD tissue samples were obtained from the Affiliated Hospital of Nantong University between January 2021 and April 2022. The study was conducted in accordance with the Declaration of Helsinki (as revised in 2013). The study was approved by the Ethics Committee of the Affiliated Hospital of Nantong University (No. 2022-L165) and informed consent was taken from all the patients.
RNA extraction and quantitative real-time PCR
Total RNA was extracted from tissue and LUAD cells using TRIzol Reagent (Invitrogen, Waltham, MA, USA). Utilizing the real-time (RT) Reagent kit (Thermo Fisher, Waltham, MA, USA), complementary DNA (cDNA) of tissue and LUAD cells were obtained according to the manufacture’s protocol. Glyceraldehyde 3-phosphate dehydrogenase (GAPDH) was chosen as the internal reference for normalization. The primer sequences were as follows: GADPH: 5'-GAC CTG ACC TGC CGT CTA-3' (forward) and 5'-AGG AGT GGG TGT CGC TGT-3' (reverse). LINC01230: 5'-CAGTCCCTCTTCCCCTCAAT-3' (forward) and 5'-GGGTGGTGGTAAGGAGATGA-3' (reverse). LINC00592: 5'-GCCCTCAGAAAGACTTGTCG-3' (forward) and 5'-GGAACCCATTTCTTCCCATT-3' (reverse). GLIS2-AS1: 5'-GCTCAGGGGAGTGAAGTGAC-3' (forward) and 5'-AGCACTGCATTGGTTTCTCC-3' (reverse).
The procedures of quantitative real-time PCR (qRT-PCR) were: 95 °C for 5 minutes and the subsequent step comprised 40 cycles, during which qRT-PCR was applied in SYBR Premix Ex Taq II on a Lightcycler 96 system (Roche, Basel, Switzerland). Data were analyzed using the 2−△△CT method.
Statistical analysis
The Wilcoxon rank sum test was used to compare the groups. We used the KM method and log-rank tests to conduct survival analysis. P<0.05 was considered to indicate statistical significance. R software version 4.1.2 was used to analyze the data.
Results
Identification of prognostic cuproptosis-related lncRNAs in LUAD and validation using qPCR
The research process of this study is shown in Figure 1. In all, 522 LUAD samples were obtained from the TCGA-LUAD cohort. A total of 16,876 lncRNAs were identified, and 19 cuproptosis-related genes were selected based on previous research. Using the ‘limma’ R package, the lncRNAs which had a significant correlation with cuproptosis-related genes were identified, and 2,244 cuproptosis-related lncRNAs were found (Figure 2A). To research the prognostic potential of these cuproptosis-related lncRNAs, univariate Cox analysis was employed to assess prognostic potential using the clinical data of the TCGA-LUAD database. Finally, 50 prognostic cuproptosis-related lncRNAs in LUAD were ascertained. Among them, 31 lncRNAs were identified as low-risk factors [hazard ratio (HR) <1], and 19 lncRNAs were identified as high-risk factors (HR >1) (Figure 2B). Then, 16 lncRNAs were identified from the above 50 prognostic cuproptosis-related lncRNAs using multivariate Cox analysis. The 16 lncRNAs details are shown in the Table 1. To further assess the relationship between the 16 lncRNAs and 19 cuproptosis-related genes, a gene-lncRNA coexpression network was mapped (Figure 2C). To verify the screened 16 lncRNAs, three prognostic cuproptosis-related lncRNAs, namely GLIS2-AS1, LINC01230, and LINC00592, were selected using a significance level of P<0.01, and these were considered to be closely related to LUAD prognosis. Using the qPCR experiment, the expression levels of these three prognostic CRLs in LUAD cell lines were detected. As shown in Figure 2D-2F, consistent with the result of the above analysis, LINC01230 and LINC00592 had significant higher levels of expression in A549, HCC827, and H1650 cell lines. By contrast, as a protective factor, GLIS2-AS1 had a lower expression in the three cell lines. Subsequently, we validated messenger RNA (mRNA) expression level of the three lncRNAs in 20 sample pairs of patients with LUAD. Similar to the cell expression trend, significantly higher mRNA expression levels in tumor tissues than in normal tissues were found in LINC01230 and LINC00592, and GLIS2-AS1 exhibited the opposite trend (Figure 2G-2I).
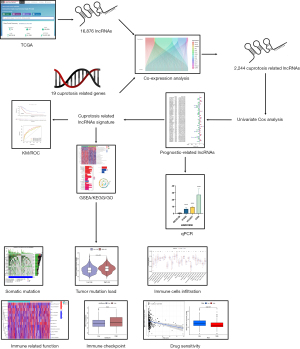
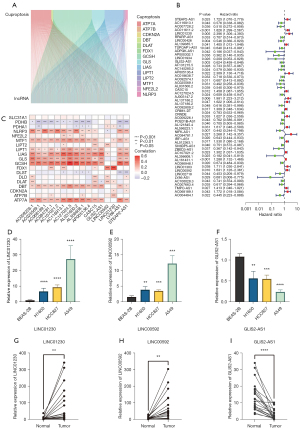
Table 1
ID | Coef | HR | HR.95L | HR.95H | P value | Risk |
---|---|---|---|---|---|---|
LY86-AS1 | −1.97914 | 0.080645 | 0.008394 | 0.774822 | 0.029186 | Low |
AL031600.2 | −1.49133 | 0.539729 | 0.300403 | 0.969721 | 0.039131 | Low |
AL356608.1 | −1.43573 | 0.182268 | 0.041011 | 0.81006 | 0.025303 | Low |
AC116913.1 | −1.00351 | 0.575818 | 0.337518 | 0.982364 | 0.042842 | Low |
AC025569.1 | −0.68591 | 0.491753 | 0.258425 | 0.935751 | 0.030598 | Low |
AC127024.5 | −0.61134 | 0.658718 | 0.458231 | 0.946922 | 0.024165 | Low |
GLIS2-AS1 | −0.26849 | 0.624009 | 0.459168 | 0.848027 | 0.002585 | Low |
LINC00592 | 0.336788 | 1.630534 | 1.125842 | 2.361469 | 0.009676 | High |
CASC15 | 0.425696 | 1.458256 | 1.087476 | 1.955453 | 0.011729 | High |
AC107021.2 | 0.566379 | 1.301997 | 1.037005 | 1.634703 | 0.023031 | High |
STEAP2-AS1 | 0.642635 | 1.724756 | 1.070362 | 2.779231 | 0.025136 | High |
LINC01230 | 0.662998 | 2.395525 | 1.306313 | 4.392928 | 0.004748 | High |
AC009226.1 | 0.712196 | 1.6271 | 1.035613 | 2.556411 | 0.034706 | High |
AC068189.1 | 0.828096 | 1.772334 | 1.018411 | 3.084379 | 0.042919 | High |
AP005136.4 | 2.395904 | 2.308989 | 1.130121 | 4.717574 | 0.021703 | High |
AC084781.1 | 3.132229 | 3.268461 | 1.141748 | 9.356565 | 0.027315 | High |
CRLs, cuproptosis-related long noncoding RNAs; HR, hazard ratio; coef, coefficient; HR.95L, hazard ratio 95% low; HR.95H, hazard ratio 95% high.
Construction of a cuproptosis-related prognostic model
To further assess the latent prognostic capability of these cuproptosis-related lncRNAs, patients with LUAD were divided randomly into the test and training groups. Table 2 shows the clinical information of the LUAD samples in the test and training groups.
Table 2
Covariates | Total, n (%) | Test, n (%) | Train, n (%) | P value |
---|---|---|---|---|
Age | ||||
≤65 years | 225 (47.77) | 111 (47.23) | 114 (48.31) | 0.888 |
>65 years | 236 (50.11) | 119 (50.64) | 117 (49.58) | |
Unknown | 10 (2.12) | 5 (2.13) | 5 (2.12) | |
Gender | ||||
Female | 256 (54.35) | 140 (59.57) | 116 (49.15) | 0.0294 |
Male | 215 (45.65) | 95 (40.43) | 120 (50.85) | |
Stage | ||||
Stage I | 255 (54.14) | 127 (54.04) | 128 (54.24) | 0.8644 |
Stage II | 108 (22.93) | 58 (24.68) | 50 (21.19) | |
Stage III | 75 (15.92) | 36 (15.32) | 39 (16.53) | |
Stage IV | 25 (5.31) | 12 (5.11) | 13 (5.51) | |
Unknown | 8 (1.70) | 2 (0.85) | 6 (2.54) | |
T | ||||
T1 | 160 (33.97) | 78 (33.19) | 82 (34.75) | 0.0219 |
T2 | 250 (53.08) | 127 (54.04) | 123 (52.12) | |
T3 | 39 (8.28) | 25 (10.64) | 14 (5.93) | |
T4 | 19 (4.03) | 4 (1.70) | 15 (6.36) | |
Unknown | 3 (0.64) | 1 (0.43) | 2 (0.85) | |
M | ||||
M0 | 318 (67.52) | 155 (65.96) | 163 (69.07) | 1 |
M1 | 24 (5.10) | 12 (5.11) | 12 (5.08) | |
Unknown | 129 (27.39) | 68 (28.94) | 61 (25.85) | |
N | ||||
N0 | 304 (64.54) | 146 (62.13) | 158 (66.95) | 0.6015 |
N1 | 87 (18.47) | 49 (20.85) | 38 (16.10) | |
N2 | 66 (14.01) | 33 (14.04) | 33 (13.98) | |
N3 | 2 (0.42) | 1 (0.43) | 1 (0.42) | |
Unknown | 12 (2.55) | 6 (2.55) | 6 (2.54) |
The percentage in brackets indicated the proportion of the data in the total, test or train group. LUAD, lung adenocarcinoma; T, tumor; M, metastasis; N, node.
Applying the optimal penalty parameter for the LASSO model, a prognostic model was constructed utilizing the above 16 prognostic cuproptosis-related lncRNAs. The cvfit and lambda curves were drawn (Figure 3A,3B). For each LUAD sample the following formula was used to calculate a risk score:
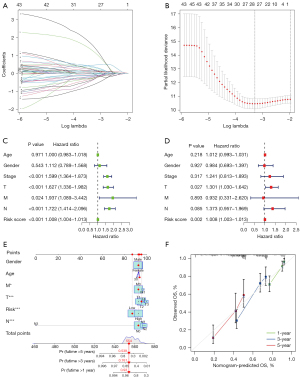
Using Cox univariate and multivariate regression analyses, we assessed the independent predictive value of this prognostic model. Cox univariate regression analysis indicated that the risk score was associated with the OS rates of patients with LUAD in the TCGA database (Figure 3C). Moreover, using multivariate Cox regression analysis, we found that risk score and T stage can be considered as an independent prognostic factor to forecast OS rate of the sample (Figure 3D). By adding the scores of many related factors, the predictive nomogram calculates the survival probability of these patients with LUAD. Compared with the ideal prediction model, 1-, 3-, and 5-year OS rates were accurately predicted (Figure 3E,3F).
Validation of the cuproptosis-related prognostic risk model
With the aim of verifying the reliability of this model, the LUAD samples were separated into high- and low-risk groups based on median risk score. Figure 4A-4C visualize the distribution of risk scores and survival status in the sample. Heatmaps were drawn to exhibit the distribution of 16 prognostic cuproptosis-related lncRNAs in two risk groups (Figure 4D-4F). Using KM survival analysis, we found that LUAD samples in the high-risk group had a worse survival prognosis compared with those in the low-risk group (Figure 4G-4I). Time-dependent ROC curves were also drawn in the different groups (Figure 4J-4L). The ROC curves were depicted to assess the accuracy of the prognostic model compared with common clinicopathological characteristics (Figure 4M-4O). The area under the curve (AUC) result of this risk score model was 0.792 in the overall group, which was the highest AUC value compared with other clinicopathological characteristics. The result indicated that the compliance index of the risk score was greater than those of age, gender, stage, T, M, and N, which illustrates that the risk score provided a more accurate prediction of LUAD prognosis. We validated the total distribution map, heatmap, KM analysis, and time-dependent ROC analysis in the overall training and test groups. The LUAD samples of high- and low-risk scores were found to be reasonably evenly distributed in the above three groups. The above figure fully verifies the reliability of risk score associated with the prognosis of patients with LUAD.
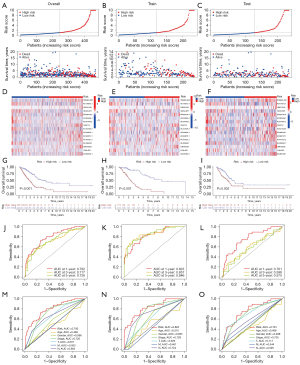
Cuproptosis-related risk score associated clinicopathological characteristics in patients with LUAD and cancer-related gene mutations
In the next study, using KM survival analysis, we further analyzed clinicopathological characteristics in the two risk score groups. In terms of age, samples were divided into an older group (over 65 years old) and a younger group (less than or equal to 65 years old). The low-risk score group had a better survival with statistically significance compared with the high-risk score group (Figure S1A,S1B). Figure S1C,S1D indicate that both male and female groups exhibited significant associations with the risk score. Similarly, regarding tumor staging, T, N, and stage are compared separately in Figure S1E-S1J. Samples in the high-risk score group showed worse survival in the above tumor stage. In summary, stratified analysis confirmed that the cuproptosis-related risk score signature had prognostic value for age, gender, T, N, and stage.
Subsequently, to analyze differences of tumor-related gene mutations between the high- and low-risk groups, waterfall plots were prepared to compare tumor somatic mutations in the two risk score groups separately, and the top 20 highest mutation frequency genes are shown in Figure 5A,5B. According to the somatic mutation data in TCGA, the tumor mutational burden (TMB) score was calculated. This TMB score in high-risk score group was significantly higher than that in the low-risk score group (P<0.001) (Figure 5C). Finally, the impact of TMB on OS was assessed. As shown in Figure 5D, patients with low TMB had a worse prognosis. Next, we combined TMB and cuproptosis-related risk score groups for analysis. Interestingly, patients with a low TMB in the high- and low-risk groups had worse OS than patients with a high TMB in different risk groups. Samples with low TMB in the high-risk group had the worst prognosis among compared with the other three groups (Figure 5E). Overall, compared with TMB, the risk model was a more important factor in predicting prognosis.
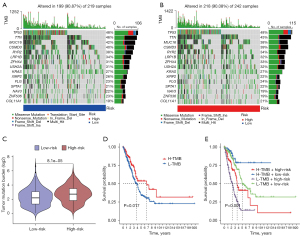
Principal component analysis (PCA) of the cuproptosis-related risk model
Four scatterplots were performed to reveal PCA of the two risk groups according to genome expression profiles from the TCGA (Figure S2A), cuproptosis-related encoding genes (Figure S2B), 16 prognostic cuproptosis-related lncRNAs (Figure S2C), and cuproptosis-related lncRNAs (Figure S2D), respectively. The PCA results showed that the cuproptosis-related risk model can divide patients with LUAD into high- and low-risk groups.
GSEA, GO, and KEGG analysis
To better understand signal pathways and biological functions of different risk groups, GSEA was performed (Figure 6A). As shown in the heatmap, several antitumor mechanism pathways were found enriching in the low-risk group, including taurine and hypotaurine metabolism (28) and alpha linolenic acid metabolism (29). However, for the high-risk group, several cellular metabolisms were enriched including cell cycle, DNA replication, amino sugar and nucleotide sugar metabolism, and pyrimidine metabolism. To further explore biological metabolism and functions of differentially expressed genes (DEGs) between the two risk groups, log2| FC| >1 and false discovery rate (FDR) <0.05 were set as conditions to screening DEGs. Using the filtered DEGs, GO enrichment analysis was performed and revealed enrichment of biological process (BP), molecular function (MF), and cell component (CC) (Figure 6B,6C). The results of KEGG analysis are shown in Figure 6D.
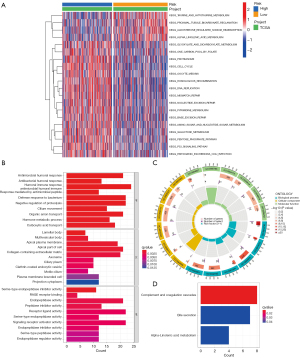
Immune-related analysis of the cuproptosis-related risk model
To further assess the correlation between the cuproptosis-related risk model and antitumour immunity, the infiltration of 23 kinds of immune cells was analyzed, and 12 kinds of immune cells were found to exhibit statistically significant differences in the two groups (Figure 7A). Compared with the high-risk group, eosinophil, immature dendritic cell, mast cell, plasmacytoid dendritic cell, and T follicular helper cells had a higher degree of infiltration in the low-risk group. By contrast, activated CD4 T cell, CD56bright natural killer cell, CD56dim natural killer cell, gamma delta T cell, natural killer T cell, neutrophil, and type 2 T helper cell were more numerous in the high-risk group. The connection of the cuproptosis-related risk score and tumor microenvironment (TME) infiltrating immune cells in LUAD was analyzed to investigate the immune characteristics of the risk score (Figure 7B). Applying the ‘GSVA’ R package, a heatmap of immune functions was performed (Figure 7C). Targeted therapy has attracted increased research attention in recent years. Therefore, we analyzed the therapeutic targets of the two risk groups. Interestingly, LAG-3 and PD-L1 were found to be significantly different in the expression of immune checkpoint between the two risk groups (Figure 7D,7E). In conclusion, our findings showed that the cuproptosis-related risk model was closely associated with immune response and immunotherapy, which have advantages compared with other clinically conventional markers.
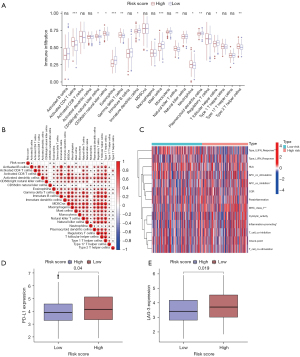
Potential drugs analysis by targeting the cuproptosis-related risk model
With the help of drug sensitivity analysis, we screened potential drugs, which target the cuproptosis metabolism to treat patients with LUAD. Using the pRRophetic algorithm, we assessed treatment outcome according to the half-maximal inhibitory concentration (IC50) of each patient with LUAD. A total of 15 types of drugs were screened comparing the IC50 levels of the two different risk groups. Among these, except for nine types of drugs, which have been shown to have an effect on lung cancer including paclitaxel, saracatinib, and cyclopamine (Figure S2E-2M), we also found A-770041, BMS-509744, CGP-60474, VX-680, WH-4-023, and WZ-1-84 had better drug sensitivity in the low-risk group similar to the above nine types of drugs (Figure 8A-8F). Overall, through drug sensitivity analysis, the low-risk group was revealed to have better drug sensitivity than the high-risk group, which further demonstrates that the low-risk group has a better prognosis, and that cuproptosis-related lncRNA models are reliable as a biological marker. In addition, six newly discovered drugs can be used as latent drugs for the treatment of LUAD.
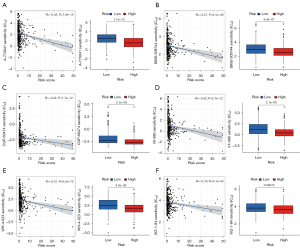
Discussion
With the development of immunotherapy and targeted therapy, the 5-year survival rate of LUAD has improved significantly (30). However, owing to the high heterogeneity, LUAD is still the main cause of cancer-related deaths amongst all cancer types (31). Therefore, a new classification for LUAD is urgently needed. As a novel cell death pathway, cuproptosis is based on the metabolism of copper in mitochondria (32). The discovery of cuproptosis provides a novel approach to anticancer treatment and is a potential candidate for clinical application (19). However, research about cuproptosis in LUAD remains limited. Because gene changes may precede obvious histopathological changes, the establishment of biomarkers is helpful for early diagnosis and treatment of cancer and plays an important role in improving the survival rate of cancer. Therefore, based on cuproptosis-related lncRNAs, we constructed a risk score model for the forecasts of prognosis in LUAD. Moreover, we focused on the role of this cuproptosis-related risk model in immune characteristics and immunotherapy.
In this study, we searched for research related to cuproptosis, identified 19 genes related to cuproptosis, and screened CRLs using coexpression analysis. Subsequently, based on the analysis of the prognosis of each sample and the expression data of these CRLs, 50 prognostic CRLs in LUAD were screened. Using multivariate Cox analysis, 16 lncRNA were selected to construct the cuproptosis-related risk signature. Previous studies have shown that some of the 16 lncRNAs are associated with the prognosis and progression of LUAD: CASC15 has a high expression in non-small cell lung cancer and boosts cell proliferation and metastasis (33); LY86-AS1 and STEAP2-AS1 correlate with prognosis of LUAD (34,35); GLIS2-AS1, LINC01230, and LINC00592 were identified as having a close relationship with the prognosis of LUAD in the above analysis (P<0.01). To verify the accuracy of the above analysis, qPCR was applied to analyze the expression level of GLIS2-AS1, LINC01230, and LINC00592 in LUAD cells and tissues. As a low-risk factor, GLIS2-AS1 was confirmed to be underexpressed in LUAD. However, as high-risk factors, LINC01230 and LINC00592 were highly expressed in LUAD. These results validate the accuracy of the above analysis.
Both ROC curves and nomograms have been used extensively to detect and predict the prognosis of cancer (36-38). In our analysis, we found that the risk model was significant in forecasting the survival and prognosis of patients. Compared with other routinely used markers, the risk model has the best prognostic accuracy. Moreover, the cuproptosis-related risk model had prognostic value in relation to age, gender, T, N, and stage. In summary, the cuproptosis-related risk signature was found to have the potential to become a marker to forecast the prognosis of patients with LUAD.
The TMB is considered the number of somatic mutations in the interrogated genome sequence per megagram of matrix, and it can be used as a predictive biomarker for immunotherapy of many kinds of tumors (39). Recent research has shown that TMB is consistent with the expression of PD-L1 (40). Compared with the low-risk group, significant high expression of TMB was found in the high-risk group. Similarly, patients with LUAD in the TCGA with high TMB had a better OS. In view of the close association between TMB and tumor immunity, immunity-related analysis was performed to investigate characteristics of the cuproptosis-related risk model in immunity. First, the correlation of two risk groups and TME cell infiltration was assessed. According to previous research, different types of immune cells have different functions in tumor immunity. For examples, CD4 T cells have been shown to play a negative effect in tumor immunity (41,42). Eosinophil infiltration in tumors was associated with a good prognosis in most cases (43). Neutrophils have been found to play a role in tumorigenesis, growth, proliferation, and metastasis (44,45). The T follicular helper cells have been demonstrated to participate in the antitumor immunity of patients with non-small cell lung cancer (46). In this study, activated CD4 T cells, CD56bright/dim natural killer cells, gamma delta T cells, natural killer T cells, neutrophils, and type 2 T helper cells showed higher immune infiltration in the high-risk group. Most of these indicate a promotion of tumor progression and inhibition of antitumor immunity, which lead to poor prognosis. Eosinophils, immature dendritic cells, mast cells, plasmacytoid dendritic cells, and T follicular helper cells, which represent immune activation, had a higher degree of infiltration in the low-risk group. Lastly, we found a close connection between the cuproptosis-related risk model and immunotherapy. We found that the cuproptosis-related risk score was negatively correlated with immune cell infiltration and expression of immune checkpoint, which indicates that the risk score has potential in predicting the results of immunotherapy. Moreover, patients with LUAD can benefit from immune checkpoint action, which may improve the prognosis of high-risk patients by enhancing immune response or inducing cuproptosis. Finally, based on IC50, we screened out six new drugs targeting the cuproptosis-related risk signature, which may a provide a reference for clinical drug treatment in the future. To sum up, compared with other clinically conventional markers, cuproptosis-related lncRNAs risk model has better prediction accuracy and closely association with immune response and immunotherapy.
This study also had some shortcomings. First, more LUAD samples are required to check the stability and reliability of the risk signature. Second, although three prognostic cuproptosis-related lncRNAs were detected in 20 pairs of clinical LUAD tissues and three types of LUAD cells, more samples and cell lines are needed to assess which could make the results more dependable. Third, we analyzed only 471 patients with LUAD from the TCGA database but did not incorporate samples from healthy patients. Finally, because research on cuproptosis is limited, further research on the correlation between lncRNAs and cuproptosis-related genes (CRGs) as prognostic indicators is significant.
Conclusions
This research constructed a novel prognostic predictive model based on 16 cuproptosis-related lncRNAs, which demonstrated accurate predictive ability for patients with LUAD. The correlation between the risk model and immune environment was analyzed. The results of this study provide the basis for a novel approach to predicting prognosis of patients with LUAD, including reference data for clinical drug treatment of patients with LUAD.
Acknowledgments
We would like to thank B. Meiser and J. Jones from AME Editing Service (http://editing.amegroups.cn/#editing) for their help in polishing our paper.
Funding: This work was supported by the “Six-One” Project for High-Level Health Talents (No. LGY2016037), Nantong Key Laboratory of Translational Medicine in Cardiothoracic Diseases, Nantong Clinical Medical Research Center of Cardiothoracic Disease, and Institution of Translational Medicine in Cardiothoracic Diseases in Affiliated Hospital of Nantong University.
Footnote
Reporting Checklist: The authors have completed the TRIPOD reporting checklist. Available at https://atm.amegroups.com/article/view/10.21037/atm-22-3195/rc
Data Sharing Statement: Available at https://atm.amegroups.com/article/view/10.21037/atm-22-3195/dss
Conflicts of Interest: All authors have completed the ICMJE uniform disclosure form (available at https://atm.amegroups.com/article/view/10.21037/atm-22-3195/coif). The authors have no conflicts of interests to declare.
Ethical Statement: The authors are accountable for all aspects of the work in ensuring that questions related to the accuracy or integrity of any part of the work are appropriately investigated and resolved. The study was conducted in accordance with the Declaration of Helsinki (as revised in 2013). The study was approved by the Ethics Committee of the Affiliated Hospital of Nantong University (No. 2022-L165) and informed consent was taken from all the patients.
Open Access Statement: This is an Open Access article distributed in accordance with the Creative Commons Attribution-NonCommercial-NoDerivs 4.0 International License (CC BY-NC-ND 4.0), which permits the non-commercial replication and distribution of the article with the strict proviso that no changes or edits are made and the original work is properly cited (including links to both the formal publication through the relevant DOI and the license). See: https://creativecommons.org/licenses/by-nc-nd/4.0/.
References
- Sung H, Ferlay J, Siegel RL, et al. Global Cancer Statistics 2020: GLOBOCAN Estimates of Incidence and Mortality Worldwide for 36 Cancers in 185 Countries. CA Cancer J Clin 2021;71:209-49. [Crossref] [PubMed]
- Edbrooke L, Aranda S, Granger CL, et al. Multidisciplinary home-based rehabilitation in inoperable lung cancer: a randomised controlled trial. Thorax 2019;74:787-96. [Crossref] [PubMed]
- Barta JA, Powell CA, Wisnivesky JP. Global Epidemiology of Lung Cancer. Ann Glob Health 2019;85:8. [Crossref] [PubMed]
- Zang R, Shi JF, Lerut TE, et al. Ten-Year Trends of Clinicopathologic Features and Surgical Treatment of Lung Cancer in China. Ann Thorac Surg 2020;109:389-95. [Crossref] [PubMed]
- Siegel RL, Miller KD, Fuchs HE, et al. Cancer statistics, 2022. CA Cancer J Clin 2022;72:7-33. [Crossref] [PubMed]
- Song J, Sun Y, Cao H, et al. A novel pyroptosis-related lncRNA signature for prognostic prediction in patients with lung adenocarcinoma. Bioengineered 2021;12:5932-49. [Crossref] [PubMed]
- Ponting CP, Oliver PL, Reik W. Evolution and functions of long noncoding RNAs. Cell 2009;136:629-41. [Crossref] [PubMed]
- Liu M, Zhong J, Zeng Z, et al. Hypoxia-induced feedback of HIF-1α and lncRNA-CF129 contributes to pancreatic cancer progression through stabilization of p53 protein. Theranostics 2019;9:4795-810. [Crossref] [PubMed]
- Pan W, Li W, Zhao J, et al. lncRNA-PDPK2P promotes hepatocellular carcinoma progression through the PDK1/AKT/Caspase 3 pathway. Mol Oncol 2019;13:2246-58. [Crossref] [PubMed]
- Cheng B, Rong A, Zhou Q, et al. LncRNA LINC00662 promotes colon cancer tumor growth and metastasis by competitively binding with miR-340-5p to regulate CLDN8/IL22 co-expression and activating ERK signaling pathway. J Exp Clin Cancer Res 2020;39:5. [Crossref] [PubMed]
- Zhang YX, Yuan J, Gao ZM, et al. LncRNA TUC338 promotes invasion of lung cancer by activating MAPK pathway. Eur Rev Med Pharmacol Sci 2018;22:443-9. [PubMed]
- Zhao M, Xin XF, Zhang JY, et al. LncRNA GMDS-AS1 inhibits lung adenocarcinoma development by regulating miR-96-5p/CYLD signaling. Cancer Med 2020;9:1196-208. [Crossref] [PubMed]
- Deng X, Xiong W, Jiang X, et al. LncRNA LINC00472 regulates cell stiffness and inhibits the migration and invasion of lung adenocarcinoma by binding to YBX1. Cell Death Dis 2020;11:945. [Crossref] [PubMed]
- Han X, Jiang H, Qi J, et al. Novel lncRNA UPLA1 mediates tumorigenesis and prognosis in lung adenocarcinoma. Cell Death Dis 2020;11:999. [Crossref] [PubMed]
- Qu S, Jiao Z, Lu G, et al. PD-L1 lncRNA splice isoform promotes lung adenocarcinoma progression via enhancing c-Myc activity. Genome Biol 2021;22:104. [Crossref] [PubMed]
- Tsvetkov P, Coy S, Petrova B, et al. Copper induces cell death by targeting lipoylated TCA cycle proteins. Science 2022;375:1254-61. [Crossref] [PubMed]
- Faubert B, Li KY, Cai L, et al. Lactate Metabolism in Human Lung Tumors. Cell 2017;171:358-371.e9. [Crossref] [PubMed]
- Xie M, Fu XG, Jiang K. Notch1/TAZ axis promotes aerobic glycolysis and immune escape in lung cancer. Cell Death Dis 2021;12:832. [Crossref] [PubMed]
- Li SR, Bu LL, Cai L. Cuproptosis: lipoylated TCA cycle proteins-mediated novel cell death pathway. Signal Transduct Target Ther 2022;7:158. [Crossref] [PubMed]
- Yu Y, Tian X. Analysis of genes associated with prognosis of lung adenocarcinoma based on GEO and TCGA databases. Medicine (Baltimore) 2020;99:e20183. [Crossref] [PubMed]
- Sayeeram D, Katte TV, Bhatia S, et al. Identification of potential biomarkers for lung adenocarcinoma. Heliyon 2020;6:e05452. [Crossref] [PubMed]
- Giannos P, Kechagias KS, Gal A. Identification of Prognostic Gene Biomarkers in Non-Small Cell Lung Cancer Progression by Integrated Bioinformatics Analysis. Biology (Basel) 2021;10:1200. [Crossref] [PubMed]
- Chen Y, Jin L, Jiang Z, et al. Identifying and Validating Potential Biomarkers of Early Stage Lung Adenocarcinoma Diagnosis and Prognosis. Front Oncol 2021;11:644426. [Crossref] [PubMed]
- Robin X, Turck N, Hainard A, et al. pROC: an open-source package for R and S+ to analyze and compare ROC curves. BMC Bioinformatics 2011;12:77. [Crossref] [PubMed]
- Hänzelmann S, Castelo R, Guinney J. GSVA: gene set variation analysis for microarray and RNA-seq data. BMC Bioinformatics 2013;14:7. [Crossref] [PubMed]
- Robinson MD, McCarthy DJ, Smyth GK. edgeR: a Bioconductor package for differential expression analysis of digital gene expression data. Bioinformatics 2010;26:139-40. [Crossref] [PubMed]
- Wu T, Hu E, Xu S, et al. clusterProfiler 4.0: A universal enrichment tool for interpreting omics data. Innovation (Camb) 2021;2:100141. [Crossref] [PubMed]
- Wang JJ, Wang Y, Hou L, et al. Immunomodulatory Protein from Nectria haematococca Induces Apoptosis in Lung Cancer Cells via the P53 Pathway. Int J Mol Sci 2019;20:5348. [Crossref] [PubMed]
- Vecchini A, Ceccarelli V, Susta F, et al. Dietary alpha-linolenic acid reduces COX-2 expression and induces apoptosis of hepatoma cells. J Lipid Res 2004;45:308-16. [Crossref] [PubMed]
- Islami F, Ward EM, Sung H, et al. Annual Report to the Nation on the Status of Cancer, Part 1: National Cancer Statistics. J Natl Cancer Inst 2021;113:1648-69. [Crossref] [PubMed]
- Yang D, Liu Y, Bai C, et al. Epidemiology of lung cancer and lung cancer screening programs in China and the United States. Cancer Lett 2020;468:82-7. [Crossref] [PubMed]
- Cobine PA, Moore SA, Leary SC. Getting out what you put in: Copper in mitochondria and its impacts on human disease. Biochim Biophys Acta Mol Cell Res 2021;1868:118867. [Crossref] [PubMed]
- Yu DJ, Zhong M, Wang WL. Long noncoding RNA CASC15 is upregulated in non-small cell lung cancer and facilitates cell proliferation and metastasis via targeting miR-130b-3p. Eur Rev Med Pharmacol Sci 2021;25:1765. [PubMed]
- Tao Y, Li Y, Liang B. Comprehensive analysis of microenvironment-related genes in lung adenocarcinoma. Future Oncol 2020;16:1825-37. [PubMed]
- Wang XW, Guo QQ, Wei Y, et al. Construction of a competing endogenous RNA network using differentially expressed lncRNAs, miRNAs and mRNAs in non-small cell lung cancer. Oncol Rep 2019;42:2402-15. [Crossref] [PubMed]
- Mandrekar JN. Receiver operating characteristic curve in diagnostic test assessment. J Thorac Oncol 2010;5:1315-6. [Crossref] [PubMed]
- Rose PG, Java J, Whitney CW, et al. Nomograms Predicting Progression-Free Survival, Overall Survival, and Pelvic Recurrence in Locally Advanced Cervical Cancer Developed From an Analysis of Identifiable Prognostic Factors in Patients From NRG Oncology/Gynecologic Oncology Group Randomized Trials of Chemoradiotherapy. J Clin Oncol 2015;33:2136-42. [Crossref] [PubMed]
- Balachandran VP, Gonen M, Smith JJ, et al. Nomograms in oncology: more than meets the eye. Lancet Oncol 2015;16:e173-80. [Crossref] [PubMed]
- Sha D, Jin Z, Budczies J, et al. Tumor Mutational Burden as a Predictive Biomarker in Solid Tumors. Cancer Discov 2020;10:1808-25. [Crossref] [PubMed]
- Chan TA, Yarchoan M, Jaffee E, et al. Development of tumor mutation burden as an immunotherapy biomarker: utility for the oncology clinic. Ann Oncol 2019;30:44-56. [Crossref] [PubMed]
- Saito T, Nishikawa H, Wada H, et al. Two FOXP3(+)CD4(+) T cell subpopulations distinctly control the prognosis of colorectal cancers. Nat Med 2016;22:679-84. [Crossref] [PubMed]
- Nishikawa H. Regulatory T cells in cancer immunotherapy. Rinsho Ketsueki 2014;55:2183-9. [PubMed]
- Davis BP, Rothenberg ME. Eosinophils and cancer. Cancer Immunol Res 2014;2:1-8. [Crossref] [PubMed]
- Coffelt SB, Wellenstein MD, de Visser KE. Neutrophils in cancer: neutral no more. Nat Rev Cancer 2016;16:431-46. [Crossref] [PubMed]
- Ocana A, Nieto-Jiménez C, Pandiella A, et al. Neutrophils in cancer: prognostic role and therapeutic strategies. Mol Cancer 2017;16:137. [Crossref] [PubMed]
- Ma QY, Huang DY, Zhang HJ, et al. Function of follicular helper T cell is impaired and correlates with survival time in non-small cell lung cancer. Int Immunopharmacol 2016;41:1-7. [Crossref] [PubMed]