International publication trends in the application of artificial intelligence in ophthalmology research: an updated bibliometric analysis
Highlight box
Key findings
• Current hotspots in artificial intelligence (AI)-related ophthalmology research are focused on chronic ocular disease, particularly on retinopathy and glaucoma, and are gradually shifting to anterior segment diseases such as cataracts and Keratopathy.
What is known, and what is new?
• AI plays an important role in diagnosing, managing, and predicting ocular disorders.
• Telemedicine, real-world research, new AI algorithms, and the association between eye and systemic biomarkers are possible future research trends.
What is the implication, and what should change now?
• The transformation and clinical application of AI-based productions should be encouraged.
Introduction
Artificial intelligence (AI) is a new technology science that investigates and develops theories, methods, and technologies for mimicking and augmenting human intelligence (1,2). From its birth in the 1950s to the present, AI has been used to assist in the ancillary diagnosis of diseases, and it is a rapidly rising sector (3). The benefits of AI in medicine are immense. With its sophisticated algorithms and capacity for learning, AI is progressively and widely adopted in all facets of health care due to its overwhelming benefits (4). Currently, the real potential of AI comprises screening, diagnosis, classification, and therapy guidance, which are conducive to the development of precision medicine (5).
In recent years, ophthalmology has garnered considerable and widespread scientific and clinical attention. The number of ophthalmic disorders is increasing among the aging global population. In many instances, blindness can be prevented by the early identification and prompt action. Since the publication of several pioneering works on the use of deep learning to screen for diabetic retinopathy (DR) in 2016, AI research, particularly deep learning, has blossomed in the area of ophthalmology (6-8). Ophthalmic diagnosis largely depends on imaging examinations. With the widespread use of optical coherence tomography (OCT) and fundus photography, AI-based deep learning approaches can rapidly and noninvasively evaluate enormous image data sets and identify, locate, and quantify disease characteristics (9-11). Initially, the majority of ophthalmic AI studies concentrated on posterior segment disorders, such as DR, age-related macular degeneration (AMD), glaucoma, and retinopathy of prematurity (ROP) (7,12,13). In recent years, there has been a proliferation of studies using AI on anterior segment illnesses and imaging (14-16). The medical assistant diagnosis system based on image recognition is beneficial for conducting large-scale population illness screening, enhancing the efficiency of clinical work, and developing novel strategies to alleviate the shortage of medical resources. In addition, the integration of AI with telemedicine is currently emerging as another viable solution to the shortage of medical resources (17).
With the surge in interest in research into AI applications in ophthalmology and the publication of many relevant articles, researchers are finding it challenging to stay abreast of the most recent developments and research hotspots in the field. According to ongoing studies, AI is still evolving rapidly in the field of ophthalmology and is still in the early stages of development. In this bibliometric analysis, we comprehensively evaluated the AI literature in ophthalmology, described the global research trends and hotspots, and visualized publishing trends. This study is a valuable resource for researchers who wish to acquire a broad overview of the progression of AI use in ophthalmology.
Methods
Database and search strategy
The Science Citation Index-Expanded (SCI-E) of the Web of Science (WOS) is the best professional database for bibliometric analysis. Consequently, we used the WOS database to conduct a literature search that covered the period from January 1, 2000 to June 1, 2022. To avoid biases introduced by daily updates of the open database, all searches were concluded on June 1, 2022.
Following a preliminary literature review and consultation with a librarian, search terms related to (I) AI technologies and (II) ophthalmology were identified. After combining keywords with Boolean operators, we entered the following retrieval search string: (TS = (“artificial intelligence”) OR TS = (“machine learning”) OR TS = (“data learning”) OR TS = (“deep learning”) OR TS = (“neural network”) OR TS = (“intelligent learning”)) AND (TI = (“eye disease”) OR TI = (“Keratoconus”) OR TI = (“cornea”) OR TI = (“dry eye”) OR TI = (“Glaucoma”) OR TI = (“refractive errors”) OR TI = (“uvea”) OR TI = (“retinopathy”) OR TI = (“macula”) OR TI = (“optic disk”) OR TI = (“choroid”) OR TI = (“glaucoma) OR TI = (“cataract”) OR TI = (“myopia”) OR TI = (“ocular disease”) OR TI = (“fundus image”) OR TI = (“eyelid tumor”)) AND publication year = (2000–2022). The language was restricted to English, and we retrieved original articles and reviews.
Screening strategy
To ensure the data’s accuracy and the research’s repeatability, the data were downloaded and examined by 2 different researchers. The targeted files were assessed using Microsoft Excel 2019 (Redmond, Washington, USA) and GraphPad Prism version 9 (GraphPad Prism Software Inc., San Diego, CA, USA). The top-cited or most productive authors, countries/regions, publications, journals, and institutions were summarized in bar charts and tables. VOSviewer (Leiden University, Leiden, the Netherlands) and CiteSpace (5.8R3) were used for data processing and visualization. To obtain a total of 1,686 publications that matched the search parameters, we included all of the original articles and reviews in the main database. Figure 1 depicts our inclusion and exclusion techniques.
Bibliometric analysis
In this investigation, we approximated the growth of publications, research frontiers (countries and authors), patterns of publication (institutions and journals), and focuses of research activities (topics and keywords). As illustrated below, the growth rate of publications through time was calculated by multiplying the ratio of publications in 2021 divided by the publications in 2000 by a factor of 1/24 according to the following formula:
The ratio of publications in one field to publications in all other fields was used to calculate relative research interest (RRI). The H-index (18), introduced by Hirsch, is a mixed index. It was used as a major indicator to evaluate the quantity and quality of academic output of a scientific researcher, country, journal, or institution.
In order to examine time trends and potential publication patterns, Microsoft Excel 2019 was used to create the following cumulative publication–based prediction model: f(x) = ax3 + bx2 + cx + d based. In this equation, x stands for time (year), and f (x) represents the total number of publications created as of a particular year.
Statistical analysis
The amount and caliber of studies published in various locations were examined using GraphPad Prism version 9. A co-occurrence network of terms from titles and abstracts was created using VOSviewer and CiteSpace, which are programs that can examine the connections between highly cited literature and productive writers. Additionally, keywords were grouped into separate clusters and simultaneously colored according to their temporal progression. The novelty of a study topic was assessed using the average appearing year.
Results
The growth rate of publications
Based on the data searching strategy, we gathered 1,686 papers from WOS published over the past 23 years, including 1,539 original articles and 147 reviews (Figure 1). The national contributions and annual trends of publications about AI in ophthalmology are depicted in Figure 2. The average annual growth rate of AI-related academic articles from 2020 to 2021 was 22.47%. Between 2000 and 2009, there was a 9.62% growth rate, followed by a 23.21% growth rate between 2010 and 2016, and an 87.5% growth rate between 2017 and 2021. The number of publications expanded dramatically between 2008 and 2022, contributing to 82.91% (1,398/1,686) of the total number of included papers (Figure S1).
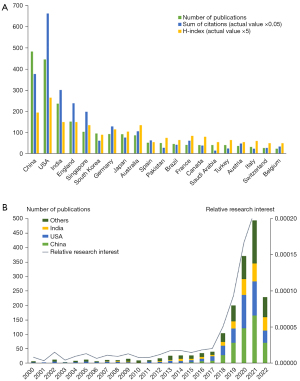
Although China ranked first among all countries, with 483 publications, the frequency of papers cited was significantly lower than that of the United States (446 papers; Figure 2). The citation frequency of the USA accounted for 43.3% of the total citations (14,367 citations and 13,256 without self-cited citations) and also obtained the highest paper H-index [54], which was almost twice that of China (7,535 citations and 6,548 without self-cited citations; H-index 40).
Research frontiers
Countries to global publications
China generated the most publications (483, 28.7%) from 2000 to 2022, followed by the United States (446, 26.5%) and India (237, 14.1%; Figure 2A). Most research was published in 2021 (494, 29.3%) in terms of the number of publications per year. Based on a comparison of the RRI value to the total number of works published in the field, our findings demonstrate that, before 2019, this field received about 0.009% of the world’s attention. By 2020, this percentage had climbed to 0.017% (Figure 2B).
Authors’ contribution
The top 10 authors published 234 papers, which accounted for 13.9% of the entire body of work in this field. Duke-National University School (NUS) of Medicine made a significant contribution. Ting DSW had the most publications (35 papers; Table 1). Despite having the most citations (1,800 times), Wong TY came in second (27 papers). Among the top 10 authors, 4 authors were American, 3 were Singaporean, and the remaining 3 were from China, England, and Japan. Notably, despite ranking fifth in terms of total publications with 22 papers, Lin HT from Sun Yat-sen University only had 299 citations. This is may be primarily due to the fact that the research was published more recently (18 papers were published in 2020–2022, 82%), suggesting Lin HT will make more remarkable contributions in the future.
Table 1
Author | Country | Affiliation | No. of publications | No. of citations | Main areas |
---|---|---|---|---|---|
Ting DSW | Singapore | Duke-NUS Medical School | 35 | 1,662 | Frontiers in various ophthalmic disorders; telemedicine |
Wong TY | Singapore | Duke-NUS Medical School | 27 | 1,800 | Association between retina and systemic biomarkers |
Weinreb RN | USA | University of California | 25 | 985 | Diagnosis and management of glaucoma |
Keane PA | England | UCL Institute of Ophthalmology | 23 | 1,489 | Application of new algorithms |
Lin HT | China | Sun Yat-sen University | 22 | 299 | Big data in medicine; management of cataract and myopia |
Acharya UR | Singapore | Singapore University of Social Sciences | 21 | 1,450 | New algorithms for glaucoma |
Bowd C | USA | University of California | 21 | 832 | Detection and classification of glaucoma |
Medeiros FA | USA | Duke University | 21 | 612 | Progression of glaucoma |
Zangwill LM | USA | University of California | 21 | 817 | Detection of glaucomatous optic neuropathy |
Asaoka R | Japan | The University of Tokyo | 18 | 415 | Prediction of glaucomatous optic neuropathy |
AI, artificial intelligence.
Publication patterns
Publication growth trend predictions
The model fitting curve predicted an increase in publications about AI in ophthalmology. The publishing trend for the next 5 years was estimated according to the total number of works published over the last 2 decades (Figure 3). Over the past 5 years, there has been a significant increase in the number of international publications. With the earliest start, the United States exhibited the trends of many large nations, including India (Figure 3C,3D). China entered this field later than did other countries, but its growth rate has since sharply increased (Figure 3B).
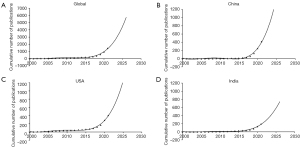
Journals and institutions
These papers were generally published in 377 different journals, with 20 of those journals accounting for nearly half of the publications (699, 41.46%; Figure 4A). IEEE Access and Scientific Reports published the most papers (66/1,686, 3.91%), followed by Translational Vision Science Technology (65/1,473, 3.8%), and Investigative Ophthalmology and Visual Science (2.31%, 39/1,473).
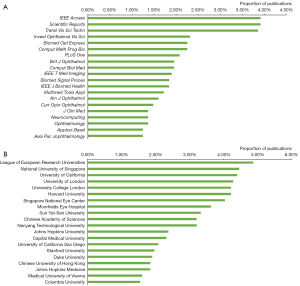
In terms of institutions, the League of European Research Universities (LERU) published the most studies (82, 4.86%), followed by the National University of Singapore (75, 4.46%; Figure 4B). Along with the LERU, the top 20 institutions for AI research included 8 in the United States, 4 in China, 3 in the United Kingdom, 3 in Singapore, and 1 in Austria. Institutional cooperation is depicted in Figure 5, which suggests that transnational cooperation has not been strong.
Focuses of research activities
A network visualization map and overlay visualization map for keywords were created using VOSviewer and CiteSpace (Figure S2). All author keywords were divided into 4 clusters, as shown in Figure 6A. In Figure 6A, each color in the network visualization map represents a cluster. The red cluster focuses on the use of AI-related technologies in the classification and diagnosis of glaucoma. Examples of keywords include “classification”, “glaucoma”, “diagnosis”, and others. We categorized it as #cluster 1, glaucoma biomedical imaging analysis and progress prediction. The green cluster is primarily concerned with segmentation and algorithms based on fundus images and was defined as #cluster 2, fundus image algorithm AI. “Optical coherence tomography (OCT)”, “retinal diseases”, and “macular degeneration” are the main keywords of the blue cluster, which was defined as #3 cluster, research on the automatic diagnosis of retinal and macular diseases based on OCT. The purple cluster is #4, study on the classification, diagnosis, and risk factors prediction of DR.
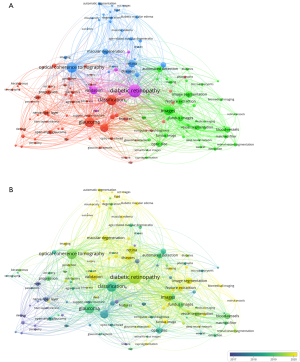
Figure 6B is a visual map of keywords superimposed over time, illustrating the evolution of keywords. The yellow nodes represent emerging keywords, indicating that these keywords have the potential to become the research focus in the future. From these results, “feature extraction”, “convolution neural network”, “image segmentation”, and “macular degeneration” are the keywords that appeared most frequently over the past 3 years, indicating that they will become future research hotspots.
Discussion
This study investigated AI research in ophthalmology by assessing the growth rate of publications, publishing patterns, features of research activities, and research hotspot trends using bibliometric data.
The growth rate of publications
Since the first publication on this topic was arose in 1994, the study of AI in ophthalmology has slowly expanded (19). However, after 2016, this field has begun to advance quickly. The publication growth rate over the last 5 years has risen to 69.8% from 2016 to 2021, which is more than 7 times the pace from 2000 to 2015. There are various causes for this recent increase. During this span, the technological achievements of AI facilitated the accelerated expansion of the use of AI in ophthalmic research (20). Since 2015, deep learning, neural networks and machine learning have created an opportunity to forecast, identify, cure, or manage diseases in ways that have never been possible (5,21). Our model-fitting time curve demonstrates that ophthalmology-related AI research is growing exponentially, and this trend is expected to continue as the number of ophthalmology-related AI publications continues to increase. By applying the growth rate of the previous 5 years to the coming 5 years, we estimate there will be twice as many publications on ophthalmic AI research in 5 years as there are now.
Research frontiers
AI-related research in ophthalmology has attracted individuals from all around the world. According to our observations, the USA, China, and India are the top 3 countries for total citations and H-index scores in the field of AI-related ophthalmology research. Undoubtedly, the USA has contributed the most and has consistently been at the forefront of research. The main driving force for AI research in ophthalmology has been high-income nations (7). As a developed nation, the United States appears to have a more standardized electronic system and a more advanced infrastructure. China has experienced a sharp surge in AI research over the last 4 years. China has more publications than any other country, but its citations and H-index rank second.
Even though China has produced a large number of publications, the number of citations is low, and the quality of research on this topic needs to improve. This finding may be due to China’s late start and uneven medical and scientific research resources. Furthermore, incomplete electronic systems may impede data collection. However, it is worth noting that China and India are populous countries that have facilitated AI studies based on a vast number of data sets and considerably accelerated the growth of their research.
The top 2 authors who contributed the most articles, Ting DSW and Wong TY, were affiliated with Duke-NUS Medical School and collaborated with each. There is no doubt they were the pioneers in this field. They focused more on automatic diagnosis and identification of abnormalities in fundus photos, exploring the predictive value of systemic diseases and leading the charge in the latest research in AI development (4,5,8,22). In addition, Weinreb RN contributed significantly to the research of glaucoma disease and made a breakthrough in the use of AI in the classification and progress prediction of glaucoma in multiple dimensions and methods (23,24). Keane PA focused on the diversity and interpretability of AI models, as well as the transformation and clinical application of AI (25,26). Sun Yat-sen University’s Lin HT has made significant contributions to the advancement of AI ophthalmology in China. He established a platform for cataract classification and diagnosis, which has greatly aided in the realization of telemedicine and led to the development of AI in the direction of myopia and cataracts (27-29). Bowd C has been committed to AI research in glaucoma (30), with Medeiros FA, Zangwill LM, and Weinreb RN cooperating closely in glaucoma (23,30,31). The research of Asaoka (32) has also mainly focused on glaucoma. These researchers are pioneers of AI research in ophthalmology, and their work will continue to influence current and upcoming studies and applications.
Publication patterns
As a result of an population, healthcare systems worldwide are facing additional difficulties. The Food and Drug Administration (FDA, UAS) approved the use of AI algorithms in primary care settings to diagnose DR in 2018 (33). This was a significant milestone in the development of AI research in ophthalmology, and it will assist in reducing pressure on the medical system. Telemedicine has been used by numerous facilities worldwide against the backdrop of the global COVID-19 pandemic (34,35). As a result, from a global standpoint, the fusion of AI with telemedicine may open new possibilities for relieving the workload on ophthalmologists.
The innovative issues being examined and the high citation count of publications have resulted in ophthalmology-related AI research becoming a favored topic in the more prestigious ophthalmology journals. The development of AI has been facilitated by the advancement and maturity of computer science and engineering. However, while up to 85% of AI research derives from institutional publishing in developed nations, 80% of humanity lives in the developing world. This imbalance is a consequence of several obstacles, including financing, research capacity, infrastructure, and language.
Focuses of research activities
Academically significant published works were regularly cited. Table 2 lists the top 10 papers on AI research in ophthalmology. According to the top keywords in the identified clusters, DR, glaucoma, and OCT images were the top areas in AI research. The main focus of AI research has been on ocular disorders with a high incidence rate that threaten vision. It is generally accepted that one of the primary global causes of severe visual impairment and blindness is retinal disease. It is predicted that 288 million people will have AMD by 2040, while 600 million people will have diabetes, one-third of whom will develop DR, and the number of patients with glaucoma will rise by 111.8 million, resulting in a significant global disease burden (5,36,37). Therefore, scientists are devoted to adopting cutting-edge technologies to promote early disease identification and management. AI is making inroads into the realm of ophthalmic therapy, from automatic screening and diagnosis to classification and disease progression prediction.
Table 2
Title | Corresponding authors | Journal | Publication year | Total citations |
---|---|---|---|---|
Development and validation of a deep learning algorithm for detection of diabetic retinopathy in retinal fundus photographs | Lily Peng | JAMA | 2016 | 2,569 |
Clinically applicable deep learning for diagnosis and referral in retinal disease | Julien Cornebise, Pearse A Keane, Olaf Ronneberger | Nature Medicine | 2018 | 827 |
Development and validation of a deep learning system for diabetic retinopathy and related eye diseases using retinal images from multiethnic populations with diabetes | Tien Yin Wong | JAMA | 2017 | 761 |
A new supervised method for blood vessel segmentation in retinal images by using gray-level and moment invariants–based features | Arturo Aquino | IEEE Transactions on Medical Imaging | 2011 | 588 |
Prediction of cardiovascular risk factors from retinal fundus photographs via deep learning | Ryan Poplin, Avinash V Varadarajan, Lily Peng, Dale R Webster | Nature Biomedical Engineering | 2018 | 544 |
Segmenting retinal blood vessels with deep neural networks | Paweł Liskowski | IEEE Transactions on Medical Imaging | 2016 | 476 |
A cross-modality learning approach for vessel segmentation in retinal images | Ping Liang, Huisheng Zhang and Tianfu Wang | IEEE Transactions on Medical Imaging | 2016 | 325 |
Artificial intelligence and deep learning in ophthalmology | Daniel Shu Wei Ting | British Journal of Ophthalmology | 2019 | 300 |
Automated detection of diabetic retinopathy on digital fundus images | Thomas Williamson | Diabetic Medicine | 2002 | 297 |
Automatic segmentation of 9 retinal layer boundaries in OCT images of patients with nonexudative AMD using deep learning and graph search | Leyuan Fang | Biomedical Optics Express | 2017 | 279 |
OCT, optical coherence tomography; AMD, age-related macular degeneration.
Recently, there has been a surge of AI research on anterior segment diseases and imaging. Kamiya et al. (38) reported that DL could be used to identify keratoconus and grade the disease’s severity. Another emerging area of study is the diagnosis and categorization of infectious keratitis. Recently, Chinese scholar Wu and his colleagues (29) reported a generic AI platform and a multilevel collaboration mode that demonstrated excellent cataract diagnosis performance. Another interesting area of research is myopia and refractive surgery. Currently, studies have reported that AI can help to identify vision-threatening conditions in patients with high myopia (27,39). Other scholars have used AI to predict the results of laser refractive surgery. However, the ability to accurately predict high myopia using AI is still limited. We believe that with the improvement and development of AI algorithms, this will be a promising field.
Additionally, with the development of digital innovation, AI recognition of fundus images can be used for more than just diagnosing and screening for diseases of the fundus.. A promising research hotspot for AI is the integration of systemic disorders with fundus pictures, a possibility that has been increasingly more examined. Particularly in chronic vascular-related disorders, there is a strong correlation between systemic diseases and fundus images. Among the recent developments in improving the strategies for chronic kidney disease screening is the use of an AI deep learning algorithm, as reported by Sabanayagam et al. (22), to detect chronic kidney disease from retinal images, which offers a novel means for communities and primary healthcare facilities to improve diagnosis. Furthermore, the accomplishments of Rim et al. (40) are remarkable. According to a study published in the Lancet Digital Health journal in 2020, systemic biomarkers, including serum creatinine and body composition indices, could be predicted using DL on retinal images. This finding offers new perspectives on the prospective applications of ophthalmic AI. AI can also actively participate in the analysis of retinal fundus images to identify coronary artery calcium and predict cardiovascular risk factors (41,42). Fundus imaging for systemic disease screening has reached a significant turning point. In addition, AI can successfully make up for ophthalmologists’ tendency to overlook systemic disorders.
AI-related ophthalmology research has been extensive and has become mature, but with the arrival of the digital era, new fields are gradually emerging and developing. Due to the limitations of traditional research, studies based on real-world conditions have aroused extensive interest among scholars and have gradually become a new research hotspot. Wu et al. (29) pioneered the combination of cataract AI and the multilevel referral model in the real-world, showing strong cataract diagnosis performance. Another study used data derived from the real-world to train the AI real expert (CARE) system, which showed satisfactory performance in various retinal abnormalities (28).
The research on updating AI algorithms is also an interesting new field that has attracted extensive attention from computer experts. As one of the most advanced models, the vision converter model appears to have a bright future in the grade recognition of diabetes retinopathy (43). The generative adversary network (GAN) is a deep learning model with excellent performance in image synthesis and is one of the most promising methods. Several studies have shown that GAN can be a good choice to overcome the shortage of ophthalmic data and the lack of large annotation data sets (44,45).
Strengths and limitations
To conduct a thorough and unbiased analysis of the data, we searched the WOS database to examine the literature related to use of AI in ophthalmology. In this procedure, certain limitations were unavoidable. We only evaluated English-language papers, which means we might have missed significant studies published in other languages. Additionally, some keywords were listed as top-level keywords, but they did not provide relevant information (for example, diseases, models, and systems) and could not be analyzed. Unfortunately, we do not have a deep understanding of the computer field and could not analyze all algorithms in detail. Therefore, we could only provide some referential ideas from the perspective of clinical practice.
Conclusions
Our goal was to present a comprehensive overview of ophthalmology-related AI research. This analysis offers a thorough summary of ophthalmology-related AI research over the past 20 years. With numerous searches and screenings, 3 search criteria, and a 23-year timeframe from 2000 to 2022, we are confident that we have identified a comprehensive range of ophthalmic AI research.
This analysis identified the following research trends in AI-related ophthalmic research: (I) high-income countries and those with large population bases were the main forces of ophthalmic AI research; (II) current hotspots focused on chronic ocular disease, particularly retinopathy and glaucoma; and (III) future research trends for AI research in ophthalmology included the association between eye and systemic biomarkers, telemedicine, real-world research, and new AI algorithms, such as vision transformer models.
The use of AI in ophthalmology is developing quickly, and prospective applications are being tested across a variety of medical fields. However, there are currently few instances of this technology being successfully used in clinical practice. In the future, AI research should be committed to bridging the gap between research and clinical application.
Acknowledgments
Funding: This work was supported by the National Natural Science Foundation of China (No. 82071005), and the Special Fund of the Pediatric Medical Coordinated Development Center of Beijing Hospitals Authority, China (No. XTCX201824).
Footnote
Conflicts of Interest: All authors have completed the ICMJE uniform disclosure form (available at https://atm.amegroups.com/article/view/10.21037/atm-22-3773/coif). The authors have no conflicts of interest to declare.
Ethical Statement: The authors are accountable for all aspects of the work in ensuring that questions related to the accuracy or integrity of any part of the work are appropriately investigated and resolved.
Open Access Statement: This is an Open Access article distributed in accordance with the Creative Commons Attribution-NonCommercial-NoDerivs 4.0 International License (CC BY-NC-ND 4.0), which permits the non-commercial replication and distribution of the article with the strict proviso that no changes or edits are made and the original work is properly cited (including links to both the formal publication through the relevant DOI and the license). See: https://creativecommons.org/licenses/by-nc-nd/4.0/.
References
- Yu KH, Beam AL, Kohane IS. Artificial intelligence in healthcare. Nat Biomed Eng 2018;2:719-31. [Crossref] [PubMed]
- Kermany DS, Goldbaum M, Cai W, et al. Identifying Medical Diagnoses and Treatable Diseases by Image-Based Deep Learning. Cell 2018;172:1122-1131.e9. [Crossref] [PubMed]
- Miller RA. Medical diagnostic decision support systems--past, present, and future: a threaded bibliography and brief commentary. J Am Med Inform Assoc 1994;1:8-27. [Crossref] [PubMed]
- Ting DSW, Pasquale LR, Peng L, et al. Artificial intelligence and deep learning in ophthalmology. Br J Ophthalmol 2019;103:167-75. [Crossref] [PubMed]
- Ting DSW, Peng L, Varadarajan AV, et al. Deep learning in ophthalmology: The technical and clinical considerations. Prog Retin Eye Res 2019;72:100759. [Crossref] [PubMed]
- Gulshan V, Peng L, Coram M, et al. Development and Validation of a Deep Learning Algorithm for Detection of Diabetic Retinopathy in Retinal Fundus Photographs. JAMA 2016;316:2402-10. [Crossref] [PubMed]
- De Fauw J, Ledsam JR, Romera-Paredes B, et al. Clinically applicable deep learning for diagnosis and referral in retinal disease. Nat Med 2018;24:1342-50. [Crossref] [PubMed]
- Ting DSW, Cheung CY, Lim G, et al. Development and Validation of a Deep Learning System for Diabetic Retinopathy and Related Eye Diseases Using Retinal Images From Multiethnic Populations With Diabetes. JAMA 2017;318:2211-23. [Crossref] [PubMed]
- Schmidt-Erfurth U, Sadeghipour A, Gerendas BS, et al. Artificial intelligence in retina. Prog Retin Eye Res 2018;67:1-29. [Crossref] [PubMed]
- Roy AG, Conjeti S, Karri SPK, et al. ReLayNet: retinal layer and fluid segmentation of macular optical coherence tomography using fully convolutional networks. Biomed Opt Express 2017;8:3627-42. [Crossref] [PubMed]
- Fang L, Cunefare D, Wang C, et al. Automatic segmentation of nine retinal layer boundaries in OCT images of non-exudative AMD patients using deep learning and graph search. Biomed Opt Express 2017;8:2732-44. [Crossref] [PubMed]
- Acharya UR, Dua S, Du X, et al. Automated diagnosis of glaucoma using texture and higher order spectra features. IEEE Trans Inf Technol Biomed 2011;15:449-55. [Crossref] [PubMed]
- Taylor S, Brown JM, Gupta K, et al. Monitoring Disease Progression With a Quantitative Severity Scale for Retinopathy of Prematurity Using Deep Learning. JAMA Ophthalmol 2019;137:1022-8. [Crossref] [PubMed]
- Ting DSJ, Ang M, Mehta JS, et al. Artificial intelligence-assisted telemedicine platform for cataract screening and management: a potential model of care for global eye health. Br J Ophthalmol 2019;103:1537-8. [Crossref] [PubMed]
- Elsawy A, Eleiwa T, Chase C, et al. Multidisease Deep Learning Neural Network for the Diagnosis of Corneal Diseases. Am J Ophthalmol 2021;226:252-61. [Crossref] [PubMed]
- Kuo BI, Chang WY, Liao TS, et al. Keratoconus Screening Based on Deep Learning Approach of Corneal Topography. Transl Vis Sci Technol 2020;9:53. [Crossref] [PubMed]
- Rathi S, Tsui E, Mehta N, et al. The Current State of Teleophthalmology in the United States. Ophthalmology 2017;124:1729-34. [Crossref] [PubMed]
- Ioannidis JPA, Baas J, Klavans R, et al. A standardized citation metrics author database annotated for scientific field. PLoS Biol 2019;17:e3000384. [Crossref] [PubMed]
- Maeda N, Klyce SD, Smolek MK, et al. Automated keratoconus screening with corneal topography analysis. Invest Ophthalmol Vis Sci 1994;35:2749-57. [PubMed]
- Topol EJ. High-performance medicine: the convergence of human and artificial intelligence. Nat Med 2019;25:44-56. [Crossref] [PubMed]
- Hogarty DT, Mackey DA, Hewitt AW. Current state and future prospects of artificial intelligence in ophthalmology: a review. Clin Exp Ophthalmol 2019;47:128-39. [Crossref] [PubMed]
- Sabanayagam C, Xu D, Ting DSW, et al. A deep learning algorithm to detect chronic kidney disease from retinal photographs in community-based populations. Lancet Digit Health 2020;2:e295-302. [Crossref] [PubMed]
- Liu H, Li L, Wormstone IM, et al. Development and Validation of a Deep Learning System to Detect Glaucomatous Optic Neuropathy Using Fundus Photographs. JAMA Ophthalmol 2019;137:1353-60. [Crossref] [PubMed]
- Goldbaum MH, Sample PA, Chan K, et al. Comparing machine learning classifiers for diagnosing glaucoma from standard automated perimetry. Invest Ophthalmol Vis Sci 2002;43:162-9. [PubMed]
- Mursch-Edlmayr AS, Ng WS, Diniz-Filho A, et al. Artificial Intelligence Algorithms to Diagnose Glaucoma and Detect Glaucoma Progression: Translation to Clinical Practice. Transl Vis Sci Technol 2020;9:55. [Crossref] [PubMed]
- Hanif AM, Beqiri S, Keane PA, et al. Applications of interpretability in deep learning models for ophthalmology. Curr Opin Ophthalmol 2021;32:452-8. [Crossref] [PubMed]
- Li Y, Feng W, Zhao X, et al. Development and validation of a deep learning system to screen vision-threatening conditions in high myopia using optical coherence tomography images. Br J Ophthalmol 2022;106:633-9. [Crossref] [PubMed]
- Lin D, Xiong J, Liu C, et al. Application of Comprehensive Artificial intelligence Retinal Expert (CARE) system: a national real-world evidence study. Lancet Digit Health 2021;3:e486-95. [Crossref] [PubMed]
- Wu X, Huang Y, Liu Z, et al. Universal artificial intelligence platform for collaborative management of cataracts. Br J Ophthalmol 2019;103:1553-60. [Crossref] [PubMed]
- Christopher M, Belghith A, Bowd C, et al. Performance of Deep Learning Architectures and Transfer Learning for Detecting Glaucomatous Optic Neuropathy in Fundus Photographs. Sci Rep 2018;8:16685. [Crossref] [PubMed]
- Bowd C, Zangwill LM, Medeiros FA, et al. Confocal scanning laser ophthalmoscopy classifiers and stereophotograph evaluation for prediction of visual field abnormalities in glaucoma-suspect eyes. Invest Ophthalmol Vis Sci 2004;45:2255-62. [Crossref] [PubMed]
- Asaoka R, Murata H, Iwase A, Araie M. Detecting Preperimetric Glaucoma with Standard Automated Perimetry Using a Deep Learning Classifier. Ophthalmology 2016;123:1974-80. [Crossref] [PubMed]
- Abràmoff MD, Lavin PT, Birch M, et al. Pivotal trial of an autonomous AI-based diagnostic system for detection of diabetic retinopathy in primary care offices. NPJ Digit Med 2018;1:39. [Crossref] [PubMed]
- Hollander JE, Carr BG. Virtually Perfect? Telemedicine for Covid-19. N Engl J Med 2020;382:1679-81. [Crossref] [PubMed]
- Ting DSW, Lin H, Ruamviboonsuk P, et al. Artificial intelligence, the internet of things, and virtual clinics: ophthalmology at the digital translation forefront. Lancet Digit Health 2020;2:e8-9. [Crossref] [PubMed]
- Pennington KL, DeAngelis MM. Epidemiology of age-related macular degeneration (AMD): associations with cardiovascular disease phenotypes and lipid factors. Eye Vis (Lond) 2016;3:34. [Crossref] [PubMed]
- Tham YC, Li X, Wong TY, et al. Global prevalence of glaucoma and projections of glaucoma burden through 2040: a systematic review and meta-analysis. Ophthalmology 2014;121:2081-90. [Crossref] [PubMed]
- Kamiya K, Ayatsuka Y, Kato Y, et al. Diagnosability of Keratoconus Using Deep Learning With Placido Disk-Based Corneal Topography. Front Med (Lausanne) 2021;8:724902. [Crossref] [PubMed]
- Tan TE, Anees A, Chen C, et al. Retinal photograph-based deep learning algorithms for myopia and a blockchain platform to facilitate artificial intelligence medical research: a retrospective multicohort study. Lancet Digit Health 2021;3:e317-29. [Crossref] [PubMed]
- Rim TH, Lee G, Kim Y, et al. Prediction of systemic biomarkers from retinal photographs: development and validation of deep-learning algorithms. Lancet Digit Health 2020;2:e526-36. [Crossref] [PubMed]
- Poplin R, Varadarajan AV, Blumer K, et al. Prediction of cardiovascular risk factors from retinal fundus photographs via deep learning. Nat Biomed Eng 2018;2:158-64. [Crossref] [PubMed]
- Rim TH, Lee CJ, Tham YC, et al. Deep-learning-based cardiovascular risk stratification using coronary artery calcium scores predicted from retinal photographs. Lancet Digit Health 2021;3:e306-16. [Crossref] [PubMed]
- Wu J, Hu R, Xiao Z, et al. Vision Transformer-based recognition of diabetic retinopathy grade. Med Phys 2021;48:7850-63. [Crossref] [PubMed]
- You A, Kim JK, Ryu IH, et al. Application of generative adversarial networks (GAN) for ophthalmology image domains: a survey. Eye Vis (Lond) 2022;9:6. [Crossref] [PubMed]
- Yoo TK, Choi JY, Kim HK. A generative adversarial network approach to predicting postoperative appearance after orbital decompression surgery for thyroid eye disease. Comput Biol Med 2020;118:103628. [Crossref] [PubMed]
(English Language Editors: C Mullens and J Gray)