Emerging role of radiogenomics in genetically triggered thoracic aortic aneurysm and dissection: a narrative review
Introduction
Thoracic aortic aneurysm and dissection (TAAD) and its complications are life-threatening conditions with high mortality. However, patients with aortic aneurysm usually do not have any symptoms until they present with sudden onset of severe back or chest pain due to rupture or dissection. If these at-risk patients can be identified earlier, pharmacological treatment may be able to halt or slow down the progression of aneurysm and intervention can be done in a prophylactic manner to reduce the mortality and morbidity. The use of point-of-care ultrasound (POCUS) for screening of TAAD in patients with hypertension was advocated but it has not been widely practiced (1).
The pathogenesis and genetics of TAAD are not fully understood. Efforts have been made over the years to better understand the disease and its clinical manifestation. The International Registry of Acute Aortic Dissection (IRAD) was established in 1996 for information collections, performing statistical analysis and clinical research (2). Currently it has 30 large referral centers in 11 countries participating in the registry. It aims to evaluate the clinical presentation, etiology, management and outcomes of patients with acute aortic dissection. Similarly, the Registry of Aortic Dissection in China (Sino-RAD) was first established in 2011 in China. A study by Duan et al. on the Sino-RAD database found that the patients with acute type A aortic dissection (ATAAD) were significantly younger than patients with type A intra-mural hematoma (TAIMH) (3). More patients of TAIMH had hypertension than that of ATAAD. These results indicated that hypertension could not solely account for the cause and progression of aortic dissection. Several surrogate markers were explored for risk prediction for TAAD. Jasjit et al. proposed the use of central blood pressure (BP) in which arterial applanation tonometry of the carotid artery was used for measurement which showed better correlation with aneurysm size enlargement and future growth (4). Apart from the BP measurement, the biomechanical property of the aorta has been studied to characterize the mechanism of aneurysm and its progression. An ex vivo mechanic study using both healthy aortic tissue and diseased aorta were used by Chiu et al. (5). The result showed that relative strain is a novel predictor of aneurysmal degeneration of the thoracic aorta. It was also suggested that the relative strain can be possibly calculated by measuring the minute differences in aortic distension throughout the cardiac cycle using electrocardiogram (ECG)-gated computed tomography (CT) or 4-dimensional CT aortogram (CTA).
Researchers believe both degenerative and genetic factors contribute to the etiology of TAAD. In a study on male veteran patients with known ascending aortic aneurysm, with high prevalence of smoking, hypertension and hyperlipidemia, most patients did not experience significant aneurysm growth over 5 years of follow-up (6). Despite small population size devoid of female patients with limited duration of follow-up, the findings may indicate aortic size with obvious risk factors alone cannot solely account for the risk of TAAD. It was also observed that the average age in this cohort was 68.1±6 years old, however, it is noted that there is a significant proportion of patients with TAAD younger than 50 years old and without history of hypertension. Thus, reinforcing the importance of genetic factors contribution and an alternative measure for risk stratification in the progression of TAAD is required. We present this article in accordance with the Narrative Review reporting checklist (available at https://atm.amegroups.com/article/view/10.21037/atm-22-6149/rc).
Methods
The literatures search was performed on PubMed, EMBASE and Cochrane database using keywords radiomics, radiogenomics, artificial intelligence, aortic aneurysm, and dissection, with search string “((aortic aneurysm) or (aortic dissection)) and ((radiomics) or (artificial intelligence) or (radiogenomics))”. Studies over the last 10 years, from year 9/2012 to 9/2022 and only English language, on human subjects and utilizing the radiomics or radiogenomics on thoracic aorta were included in the review. The details of the search strategy summary were listed in Table 1.
Table 1
Items | Specification |
---|---|
Date of search | 27/11/2022 |
Databases and other sources searched | PubMed, EMBASE, Cochrane |
Search terms used | “((aortic aneurysm) or (aortic dissection)) and ((radiomics) or (artificial intelligence) or (radiogenomics))” |
Timeframe | 9/2012 to 9/2022 |
Inclusion and exclusion criteria | Inclusion: English language, on human subjects and utilizing the radiomics or radiogenomics on thoracic aorta |
Selection process | Search and selection conducted by RTW Lum |
Key content and findings
There were only 13 studies on radiomics and 4 studies on radiogenomics integration retrieved from the search and it signifies there is still a significant knowledge gap in this field of translational medicine. The summaries and findings of the studies are summarized in Table 2 and Table 3.
Table 2
Study | Objective | Study population | Algorithm | Results | Comment |
---|---|---|---|---|---|
Monti et al. (7) | To evaluate the CNN algorithm performance in aortic diameters measurement in a heterogeneous population |
250 ECG-gated CT +/− contrast data from heterogeneous population with or without aortic pathologic findings | Manual and AI using CNN measurement of aortic diameters at 9 locations and maximum aortic diameter were made | No significant difference was found in maximum aortic diameter between manual and automatic measurements (P=0.48) | Strength: good reproducibility at 9 AHA sites and maximum aortic diameters were demonstrated using CNN algorithm |
Weakness: the reproducibility was affected by contrast enhancement, site, positioning, and local pathologic finding. There was a tendency in measurement of the internal diameter by the automatic software. Presence of aortic dissection makes it difficult to detect and measure the aortic diameter because of the presence of two lumina | |||||
Wobben et al. (8) | 3 segmentation models using 3D residual U-Net for segmentation of the TL, FL, and FLT | Retrospective study of 164 CTA scans from 43 patients with uTBAD who underwent baseline and surveillance imaging | Segmentation models using 3D residual U-Net | DSC of 0.85 (0.77–0.88) and 0.84 (0.82–0.87), for TL and FL respectively | Strength: automated and robust segmentation of the TL and FL |
Weakness: small sample size; difficulty to differentiate between slow blood flow and FLT or a thick flap and thrombus around the flap causing the reduced reproducibility of FLT segmentation | |||||
Guo et al. (9) | Clinical data-driven machine learning was used to predict the in-hospital mortality of acute aortic dissection using AI | A cohort of 1,344 acute aortic dissection patients (1,071 patients in the survivor group and 273 patients in non-survivor group) | ML algorithms using logistic regression, decision tree, K nearest neighbor, Gaussian naive bayes, and XGBoost were included | XGBoost model was the most effective model with the greatest ROC (0.927, 95% CI: 0.860–0.968) | Strength: 5 ML models were trained and developed to predict the in-hospital mortality. Comparison was made among the 5 ML models |
Weakness: small dataset collected from a single source | |||||
Lyu et al. (10) | Deep learning-based algorithm to segment dissected aorta on CTA images | The CTA volumes for training and evaluation were collected from 42 AD patients including 37 males and 5 females | 3D CNN is applied to divide the 3D volume into two anatomical portions | Dice index >92% on average | Strength: the segmentation performance improved using combination of 3D and 2D models Weakness: variations in the segmented arch parts led to notable errors on some CTA volumes; lower accuracy due to multiple local intimal flaps |
Adam et al. (11) | Evaluate an automatic, deep learning-based method ARVA to obtain maximum aortic diameter, to give cross sectional outer to outer aortic wall measurements | Training database consist of 489 CTA which was used to train for automatic external aortic wall measurements using neural networks. Another database of 62 CTA was used for validation | Deep learning-based method ARVA | The median absolute difference with reference to expert’s measurements ranged 1–2 mm among all annotators, while ARVA reported a median absolute difference of 1.2 mm | Strength: ARVA measurement on the diameter in the plane perpendicular to the centerline and automatic method in maximum aortic diameter fall within the inter-annotator variability |
Weakness: Small samples size with only 62 scans involved; performances were reduced on portal phase scanners; the algorithm can output outlier measurements that are out of the variability range of annotators | |||||
Beetz et al. (12) | AI-based tissue segmentation to analyze body composition using in patients with Marfan syndrome | 25 patients aged ≤50 years with Marfan syndrome without prior aortic repair | There was a significantly increased SMD measured in HUs in patients with aortic enlargement using AI-based automated analysis of body composition at L3 | A significant prediction of aortic enlargement for SMD (P=0.05) and PMI (P=0.04) was shown using multivariate logistic regression | Strength: CT angiography using AI-based analysis of body composition at L3 was feasible and easily available in Marfan patients |
Weakness: retrospective dataset and the relatively small cohort; muscle density on CT reflecting the body fitness and muscles structure may be influenced by the amount of contrast medium uptake | |||||
Zhou et al. (13) | Investigation on the diagnostic value of CT scan-based radiomics model for acute aortic dissection | 50 patients with acute aortic dissection +50 non-dissection patients were retrospectively selected (70 in the training group and 30 in the validation group) | 3 types of characteristics (first-order statistics, geometric descriptive features, and texture features) were extracted using PyRadiomic | Accuracy, sensitivity, specificity, and AUC of the training group were 94.3%, 91.2%, 97.2%, and 0.988 (95% CI: 0.970–0.998), respectively. The respective values for the validation group were 90.0%, 94.1%, 84.6%, and 0.952 (95% CI: 0.883–0.986) | Strength: non-contrast-enhanced CT imaging is convenient; good performance and high accuracy |
Weakness: only the largest lesion layer was used, thus unable to cover all the layers in the lesion in the ROI | |||||
Bratt et al. (14) | Evaluate the performance of trained standard deep learning segmentation model in measurement of aortic volume and diameter | Deep learning training set consisted of 3,051 CTA volume were used. Quality evaluation on 50 scans and 57 with 207 scans patients was used to evaluate the temporal reproducibility | The 2D deep learning segmentation model using U-Net backbone with an EfficientNet-B3 encoder of depth seven | Deep learning temporal showed better reproducibility for measures of both volume (P<0.008) and diameter (P<1e-5) compared with previously reported values of manual inter-rater variability | Strength: deep learning temporal showed better reproducibility for measurement |
Weakness: manual plane selection was required to extract measurements; not all clinically relevant and canonical aortic stations diameters were assessed | |||||
Yu et al. (15) | Segmentation and diameter measurement of TBAD using automatic method | 139 consecutive patients with TBAD CT angiographic images | 3D deep CNN network | Mean dice coefficient scores were 0.958, 0.961, and 0.932 for EA, TL, and FL, respectively | Strength: DL method showed better performance with higher accuracy for the EA, TL, and the FL at all positions compared with the manual method |
Weakness: only TBAD patients involved; not included TBAD with thrombus in the FL | |||||
Harris et al. (16) | Assess the likelihood of aortic dissection and rupture in prospective patients using CNN trained model | 34,689 post-contrast chest CT data was studied using the aortic dissection model | CNN model trained on aortic dissection and rupture | Sensitivity and specificity of 87.8% and 96.0% for aortic dissection and 100% and 96.0% for aortic rupture | Strength: high-volume inference system for identifying and prioritizing aortic dissection and rupture studies was implemented |
Weakness: some abdominal aortic dissections in abdomen-pelvis studies intentionally not routed through the aortic injury model might have missed; difficult to identify on post-contrast CT images where dissections near the aortic valve ended and where the valve itself began because both can look similar | |||||
Wojnarski et al. (17) | Using ML to identify the patterns of aortopathy and establish the association with valve morphology and patient characteristics | 3D CT reconstruction for 656 patients undergoing ascending aorta surgery with BAV | To cluster aortic dimension using unsupervised partitioning around medoids. Polytomous random forest analysis was used to identify the group differences | Phenotypes were visually recognizable with 94% accuracy | Strength: unsupervised analysis has yielded aortic phenotype descriptors that are sufficiently distinct to be reliably recognized by rather simple visual rules |
Weakness: patients in the studies had advanced aortopathy requiring surgical ascending aorta replacement, and might not be representative of all patients with a bicuspid aortic valve | |||||
Liang et al. (18) | To study the geometric features of the aorta that associated with risk of rupture | A total of 729 shapes were obtained from the shape distribution | SSM | An average risk classification accuracy of 95.58% and an average regression error of 0.0332 | Strength: a novel study on geometric risk stratification derived from the association between shape features and aneurysm risk predictions using FEA with machine learning algorithm |
Weakness: only using shape features as risk predictors and the other variables were kept constant; the threshold of tissue failure was different among different patients. Difficult to model material strength | |||||
Guo et al. (19) | To create an effective screening system for thoracic aortic dissection using non-contrast CT-based radiomic features | 378 patients from 4 medical centers underwent non-contrast chest CT scans as well as CT angiography or MRI |
mRMR and the LASSO were used to select the radiomic features | The AUCs was 0.91 (95% CI: 0.86–0.95), 0.92 (95% CI: 0.86–0.98) and 0.90 (95% CI: 0.82–0.98) in the training set, validation set and in the external test set respectively | Strength: the study illustrated the diagnosis of thoracic AD can be effectively derived from non-contrast CT using radiomic signature |
Weakness: potential selection bias in patients who underwent imaging studies may lead to a high positive rate of thoracic AD |
2D, two-dimensional; 3D, three-dimensional; AD, aortic dissection; AHA, American Heart Association; AI, artificial intelligence; ARVA, augmented radiology for vascular aneurysm; AUC, area under the curve; BAV, bicuspid aortic valve; CI, confidence interval; CNN, convolutional neural network; CT, computed tomography; CTA, computed tomography angiogram; DSC, Dice similarity coefficients; EA, entire aorta; ECG, electrocardiogram; FEA, finite element analysis; FL, false lumen; FLT, false lumen thrombosis; HUs, Hounsfield units; LASSO, least absolute shrinkage and selection operator; ML, machine learning; MRI, magnetic resonance imaging; mRMR, max-relevance and min-redundancy; PMI, psoas mass index; ROC, receiver operating characteristic; ROI, region of interest; SMD, skeletal muscle density; SSM, statistical shape model; TBAD, type B aortic dissection; TL, true lumen; uTBAD, uncomplicated type B aortic dissection; XGBoost, extreme gradient boost.
Table 3
Study | Objective | Study population | Results | Comments |
---|---|---|---|---|
Benjamins et al. (20) | Identify genetic loci that involved in mechanisms affecting AAo size and function in the general population | GWAS on up to 37,910 individuals from the UK Biobank using AAo maximum area, AAo minimum area and AAo distensibility | Total of 107 SNPs in 78 loci revealed a causal association with aneurysm development, but not with other vascular diseases were shown using Mendelian randomization analysis | Strength: the use of AI-approach to analyse large quantities of CMR-data, which subsequently allowed us to perform a GWAS on AAo size and function in the largest cohort to date |
Provide genetic evidence for their role in aortic aneurysm development | Weakness: unable to verify all images and predictions manually due to large sample size and quantity of images; unable to differentiate between syndromic, familial and sporadic occurrences of AAo enlargement in the current analyses | |||
Pirruccello et al. (21) | Identify genetic loci associated with ascending and descending aortic diameter | UK Biobank, 43,000 participants with MRI | A total of 82 loci in ascending and 47 loci in descending thoracic aortic diameter were identified, there were 14 loci overlapped | Strength: illustrated the efficacy of deep learning in deriving quantitative phenotypes using raw signal data at a population level; demonstrate the use of quantitative traits study to have a better understanding on disease mechanism of aneurysm and dissection; a polygenic score was developed for ascending aortic size, which is an independent risk factor for aneurysmal development of aorta |
There was association between thoracic aortic aneurysm in 385,621 UK Biobank participants and polygenic score for ascending aortic diameter |
Weakness: majority of the study population consisted of European-ancestry UK Biobank participants which limited the generalizability; the aortic measurements were derived using trained deep learning model using cardiologist-annotated segmentation data, however, majority of images were not manually reviewed | |||
Miner et al. (22) | To identify the genetic causality and association on different morphologic subtype | 126 AAA patients with normal descending thoracic aorta and 93 AAA patients with radiological features of thoracic aortic disease | The genetic pathways in double strain DNA break repair, variants in vitamin D signaling cholesterol metabolism and extracellular matrix breakdown were associated with AAA patients with thoracic aortic diseases | Strength: illustrated the use of next generation sequencing and CTA analysis for evaluating endo-phenotypes possibly related to the etiology of AAA |
Weakness: limited applicability as only patients with confirmed European ancestry were included to detect genetic variation; limited sample size; lack of tissue sample to evaluate the outcomes of the variants on transcription and translation | ||||
Francis et al. (23) |
GWAS of aortic distensibility for aortic aneurysms and brain white matter hyperintensities | The GWAS of aortic distensibility and area from UK Biobank cardiac MRI data of up to 32,590 Caucasian population | A total of 102 loci (including 27 novel associations) related to cardiovascular development, extracellular matrix production, smooth muscle cell contraction and heritable aortic diseases were identified | Strength: a significant heritable component was shown from large-scale studies in GWAS of ascending and descending aortic distensibility; the evidence of mechanistic associations of TGF-β, IGF, PDGF and VEGF signaling pathways with aortic distensibility were shown |
Four signaling pathways (TGF-β, IGF, VEGF and PDGF) associated with aortic distensibility were found using functional analysis | Weakness: only Caucasian individuals from UK Biobank were included, which was not representative of the whole UK population; the use of non-invasive blood pressure as proxies for central blood pressure measurement might affect the accuracy of the distensibility calculations |
GTAAD, genetically triggered thoracic aortic aneurysm and dissection; AAA, abdominal aortic aneurysm; AAo, ascending aorta; AI, artificial intelligence; CMR, cardiovascular magnetic resonance; CTA, computed tomography angiogram; GWAS, genome-wide association studies; IGF, insulin-like growth factor; MRI, magnetic resonance imaging; PDGF, platelet-derived growth factor; SNPs, single nucleotide polymorphisms; TGF-β, transforming growth factor β; VEGF, vascular endothelial growth factor.
Discussion
Decades ago, before the genomic era, syndromic and familial diseases were diagnosed based on history and phenotypic assessment. It was discovered that certain hereditary diseases were associated with TAAD. The advancement in technology accelerated gene discovery in establishing correlation between phenotypes and genotypes which provide us more insights into the genetic contribution in thoracic aortic diseases (24). Hence, the Genetically Triggered Thoracic Aortic Aneurysm and Cardiovascular Conditions (GenTAC) alliance was established to collect data and specimens from patients who had a confirmed or suspected genetic condition that increased their chances of developing thoracic aortic aneurysms (25).
To step forward, by identifying the underlying genetic variants may enable to identify asymptomatic individuals at risk for TAAD to facilitate potential preventive measures or treatment. With the latest update in 2015, the American College of Medical Genetics and Genomics (ACMG) developed guidance for the interpretation of sequence variants (26). Variants are classified into five tiers: pathogenic, likely pathogenic, uncertain significance, likely benign, and benign.
Genetically triggered thoracic aortic aneurysm and dissection (GTAAD)
It is believed that both environmental and genetic factors contributed to the development of aortic diseases. Chou et al. reviewed the aortopathies may be grossly grouped by anatomic distribution (27). Descending thoracic and abdominal aortic aneurysms (AAA) are mostly contributed by risk factors of acquired cardiovascular diseases, including advanced age, smoking, hypertension, and hypercholesterolemia. On the contrary, TAAD in the ascending aorta are commonly associated with hereditary predisposition.
Hereditary factors play a crucial role in aortic diseases. A number of genes were proven to be associated with syndromic features with increased risk of aortic aneurysm and dissection. Classically, syndromic conditions refer to well-described phenotypic features associated with connective tissue disorders involving in the circulatory, musculoskeletal, oculo-facial, integumentary and nervous systems. Syndromic aortic aneurysm conditions such as Marfan syndrome (MFS), Loeys-Dietz syndrome (LDS), and vascular Ehlers-Danlos syndrome (vEDS) has been extensively studied in literatures.
Apart from the syndromic cause of TAAD, other GTAAD conditions have been discovered. The genetic basis of TAAD was first published in 1997 by Biddinger et al. (28). It highlighted higher prevalence of aortic aneurysm and dissection in the first-degree relatives of the proband. With the advances in next-generation sequencing (NGS), increasing numbers of potential genes were identified that permit us to have a better understanding on the pathophysiology of aortic disease. From an early study by Yale University, 102 patients who had TAAD underwent routine whole exome sequencing (WES), 27.5% were identified to have novel genetic mutations responsible for aortic pathology (29). Another study from South China utilized WES to examine 129 cases of sporadic TAAD and found 116 variants in 47 TAAD-related genes in 62.3% of their patients (30).
Syndromic TAAD
Syndromic connective tissue disorder usually in the form of autosomal dominant patterns are often associated with aortic diseases. The dysfunction genetic defect and signaling pathway causing dilation, dissection, and ruptured aorta.
Classically, the mechanism of genetic defects involves (31):
- Genes encoding the extracellular matrix (ECM), e.g., FBN1, MFAP5, MAT2A;
- Genes encoding the TGF-β signaling pathway, e.g., TGFBR1/2, TGFB2/3, SMAD2/3;
- Genes encoding the smooth muscle cell contractile apparatus, e.g., ACTA2, MYH11, MYLK, PRKG1.
MFS is the most common hereditary autosomal dominant causing TAAD. It involves mutation in the FBN1 gene. It is diagnosed with modified Ghent criteria (32) which takes into account family history, genetic and clinical features. The missense mutation in FBN1 was first discovered to be associated with MFS in 1991. Currently, over 200 genetic variants have been identified in literature. The mutations in FBN1 gene also found in non-syndromic cases and variants of FBN1 are indeed the most common mutated genes in both syndromic and non-syndromic patients with TAAD.
Current guidelines on clinical management relies on the size, symptoms, aneurysm progression, and the presence of connective tissue disease. However, it was found that aortic dissection occurred in at-risk patients even when aorta size was normal or minimally dilated. Even in patients with MFS, literature revealed that certain types of mutations exert high probability of aortic dilatation and dissection (33). In this way, specified groups of patients may benefit from surgery at smaller diameters. Therefore, better understanding the characteristics of certain mutations can help us tailor the timing for intervention.
Non-syndromic TAAD
In non-syndromic TAAD, the phenotypes are manifested solely in the cardiovascular system and these conditions feature autosomal dominant inheritance in most circumstances; however, other modes of inheritance can be possible as reported in literature (33). It was noted that about 20% of TAAD patients were identified to have a novel genetic mutation responsible for aortic pathology.
If the first-degree relatives were not affected, they are referred to as having sporadic TAAD disease. Despite the majority of TAAD cases being sporadic and seemingly not exhibited any genetic heritance, recent research indeed showed these patients harbored genetic variants closely related to pathogenic loci such as FBN1.
In a case series of WES evaluation of sporadic aortic dissection in China, 99 patients (94 males and 5 females) with an average age of 47.4 years with suspected sporadic aortic dissections were included (34). They identified 20 candidate genes as MYH11, FBN1, and ACTA2 which were consistent with previous studies and new genetic loci were identified. Although the sample size was small with male predominance, most patients had hypertension (82/99), this cohort provided us with new insight on research and approach to identify pathogenic loci on sporadic TAAD patients for aortic dissection.
Similarly, Chen et al. discovered in his study of Chinese patients with sporadic type A aortic dissection may harbor mutation in known pathogenic genes of aorta disease (35). The finding from this cohort highlights the need for cascade screening in non-hypertensive patients with age less than 50 years and positive family history of aortic disease.
Due to significant genetic heterogeneity of TAAD, it would be a challenging task to identify all the possible genes and variants. On the other hand, the identified variants could be pathogenic, variant of unknown significance, low penetrant risk or non-pathogenic. With the evolving technology in radiomics with the use of deep machine learning algorithms, this research on genetic triggered TAAD progress can be accelerated using radiogenomics.
A genome-wide association study (GWAS) is used to identify the genetic variations associated with particular trait or diseases. Specifically, single nucleotide polymorphism (SNP) referred to a genomic variant at a single base position in the DNA and is the most common type of genetic variation among people. In this way, detection of SNPs from whole genome sequencing (WGS) was typically used to establish correlation between phenotype and genotype. Open-source toolkits such as PLINK can be used to explore the genetic loci. In view of multiple testing being involved, the significant p value is adjusted with Bonferroni correction.
However, it was found that the majority of GWAS loci resided in non-coding portions of the human genome with unknown function (36). With the advancement in high-throughput sequencing technologies, it revealed the non-coding regions played an important genomic regulatory role in the transcriptional activities of genes. The degree of expression of genes dictates the pathophysiology of certain diseases.
Transcriptome-wide association study (TWAS) is a research approach that investigates the effect of genetically regulated gene expression on complex diseases by integrating GWAS with gene expression dataset. Due to the complexity, different strategies like PrediXcan (37) and FUSION (38), and MOTWAS (39) were developed to interpret and establish the gene-trait associations.
The use of radiomics in TAAD
The term “radiomics” was first described by Lambin et al. in 2012 (40), in which assumes medical images contain specific information of the disease that may not be visible with human eye but are recognized by computer algorithms. Therefore, by extracting the features represented by pixel distribution and its interrelationship can be used to better characterize the pathology (41). With the use of artificial intelligence (AI) with deep learning neural networks, it enables high throughput features extraction and further statistical analysis for prognostic modeling (42). In view of growing numbers of radiomics research and application, a standardization for radiomics features is essential. Zwanenburg et al. proposed the use of Image Biomarker Standardization Initiative (IBSI) which validated consensus-based reference values for radiomics features, thus enabling calibration and verification of radiomics software (43).
For patients with TAAD, CTA is the most common imaging modality for investigation (3). The dimensions of aorta, extent of dissection, relationships between the true and false lumen, locations of tears, as well as complications such as organs malperfusion or ruptured can be obtained and analyzed using radiomics (7,8,16). The objective of radiomics is to extract quantitative features from medical images for analysis to predict the risk or treatment outcome, help in differential diagnosis, guide clinical decision and management. Apart from CT, different varieties of medical imaging can be used for analysis such as MRI or positron emission tomography (PET). The workflow of radiomics includes image acquisition, segmentation, features extraction, analysis, and outcome prediction.
Image acquisition
Common medical imaging modalities available for aortic topology include CT or MRI in DICOM format. Image data in the form of pixels and the quality of images rely on the resolution and number of slices. Time information such as 4-dimension scans can also be incorporated in the analysis. Dynamic scan like cardiac functional MRI or ECG-gated CT taken at different snapshots of cardiac cycle can be obtained. On the other hand, static imaging data taken at different time intervals can be used to calculate the progression of aneurysm. One major obstacle during imaging acquisition is the motion artifacts with respiration, aortic pulsation, and patient’s movement as well as radio-opaque foreign bodies which jeopardize the quality of images and make the subsequent analyses difficult. Also, the imaging orientation and alignment, the size of the aorta would be affected if the measurement is not taken perpendicular to the axis of centerline.
Segmentation
After imaging acquisition, the region of interest (ROI) is selected, anatomical structures are then annotated, and the process is known as segmentation. It is important to select the precise ROI to limit the memory usage and optimize the computing power. The image can be resized or re-aligned to facilitate for subsequent purposes. Special filters can be used to enhance the ROI and filter out artifacts. Given the large amount of imaging data, manually segmentation is a labor intensive and time-consuming process especially in situations involving complex anatomical variations. Furthermore, it is subjected to inter or intra observer variability. With the aid of AI with machine learning and deep learning capability, semi-automated and fully automated algorithms were developed for segmentation (8).
Features extraction
Radiomics features like sizes, volume can be easily obtained after defining target anatomical structure with ROI. The radiomics features can be classified as shape-based features, first-order statistic features and texture-based features (41). Shape-based features describe two-dimensional (2D)- and three-dimensional (3D)-geometric properties like area, volume, perimeter, contours irregularity, and compactness which are independent of gray level intensity. First-order statistical features are extracted from the gray-level distribution within the ROI, without focus on spatial relationships. Texture-based features are extracted from the spatial relationship between neighboring voxels with different matrices.
van Griethuysen et al. developed an open-source PyRadiomics platform for features extraction which composed of five classes: a class for first-order statistics, a class for shape descriptors, and texture classes gray level co-occurrence matrix (GLCM), gray level run length matrix (GRLR) and gray level size zone matrix (44). A study by Ding et al. demonstrated texture base features showed superior predictive ability over traditional clinical and imaging evaluation for later aneurysm growth in post-endovascular aortic aneurysm repair (45).
With the use of AI technology, the radiomics features can be extracted and processed via machine learning. Machine learning is a subset of AI that enables valuable information to be extracted from a large set of raw data (data mining), which features pattern recognition function from a predefined framework, generating outcome and formulating a prediction model. It can be broadly classified into supervised learning and unsupervised depending on how annotation was done. Supervised learning in which data was annotated with properties, characteristics or classifications. On the contrary, un-supervised learning has no labeling or tagged data (46).
Analysis and outcome prediction
The extracted radiomics features are then analyzed using statistical methods. Usually, the study cohort was split into two datasets, namely training/development dataset and validation/testing dataset.
Zhou et al. proposed the use of radiomics in non-contrast CT for the diagnosis of acute aortic dissection (13). Non-contrast CT in 50 patients with aortic dissection together with another 50 patients without dissection were included for analysis. Seventy patients from the cohort were randomly selected as a training group with radiomics features were extracted based on non-contrast CT to construct a radiomics model, the remaining 30 patients were assigned as a validation group. The accuracy, sensitivity, specificity and AUC of radiomics model were 94.3%, 91.2%, 97.2%, and 0.988 [95% confidence interval (CI): 0.970–0.998], respectively. The respective values for the validation group were 90.0%, 94.1%, 84.6%, and 0.952 (95% CI: 0.883–0.986). Another similar multicenter retrospective study by Guo et al. involving 378 patients with non-contrast CT screen for thoracic aortic dissection using radiomic with the training and validation sets were from 3 centers, while the external test set was from a 4th center (19). It demonstrated AUC of 0.91 (95% CI: 0.86–0.95) in the training set, 0.92 (95% CI: 0.86–0.98) in the validation set, and 0.90 (95% CI: 0.82–0.98) in the external test set. In the external test group, the diagnostic accuracy, sensitivity, specificity, positive predictive value, and negative predictive value were 90.5%, 85.7%, 91.7%, 70.6%, and 96.5%, respectively.
Radiogenomic integration for GTAAD
The radiomics profile and genomic information can be integrated to achieve more powerful predictive value. It aims to assist genetic diagnosis, providing information on disease prognosis and understand the spectrum and frequency of genomic variants and generate and construct a risk predictive model to guide clinical management and decision making. The use of radiogenomics was initially applied in oncology (47) and it was then later used in cardiovascular systems in coronary heart disease (46) and GTAAD.
Benjamins et al. developed an AI-based segmentation system for the ascending aorta to extract the diameters from cardiac MRI from UK Biobank (20). The maximum and minimum size of aorta as well as the calculated aorta dispensability were carried on for GWAS analysis and identified 107 SNPs in 78 loci. Mendelian randomization studies illustrate the evidence in genetic association between the aortic anatomy and development of aneurysm in a general population. From this study, it illustrated certain cardiovascular traits can be easily obtained from an imaging database using automated AI systems. Subsequent analysis can be done after incorporating GWAS and establishing genetic association with the aortic disease.
Similarly, Pirruccello et al. demonstrated the use of deep learning model to extract dimensions of ascending and descending aorta using MRI imaging data from the UK Biobank (21), with 82 and 47 loci associated with ascending and descending thoracic aortic diameter respectively using GWAS. Polygenic score was constructed from 89 autosomal SNPs from the ascending aorta GWAS. It showed there was a strong association with 685 incident cases of thoracic aneurysm or dissection over medium follow-up of 11.2 years. Another 46 autosomal lead SNPs was used to build the descending diameter polygenic score, however, the association was weaker [hazard ratio (HR) 1.15, 95% CI: 1.07–1.24, P=2.9×10−4]. This might reflect a more complex mechanism existing in the descending aortic pathology. This study showed deep learning using AI is a robust platform for formulating quantitative phenotypes using raw data from population databases.
Implication of radiogenomics studies
These studies provide evidence that WGS and whole genome annotation enable us to identify pathogenic variants and its genetic loci for genetically triggered aortic aneurysm and dissection. They enrich our knowledge on the causal relationships between the phenotype and genotype of TAAD. Brooke et al. recommend clinical genetic testing in non-hypertensive patients with early onset (<50 years old) thoracic aortic dissection with positive family history (48).
Currently, there is no guideline on the timing for surveillance imaging for patients with TAAD. It has been observed that some patients previously treated with interposition graft for type A aortic dissection showed aneurysmal dilation of the remaining aorta despite optimization of medical treatment during follow-up. If patients harbor the pathogenic variant, more frequent follow-up imaging as well as prophylactic surgical intervention is warranted to minimize the risk of unplanned emergency operation in the case of ruptured or dissection. Research is carried out on the use of imaging and computer simulations to predict the fate of aortic false lumen regression and remodeling (9). The use of radiomics enables us to capture parameters that may predispose to biomechanical failure from morphological and hemodynamic features (18).
Moreover, better understanding on the pathophysiology of TAAD and its associated genetic loci can also provide information on pharmacological mechanisms for drug development and testing. Deleeuw et al. reviewed the investigational and experimental drug treatment strategies for MFS indicating possible drug targets in genetically modified mouse models carrying mutations in FBN1 variants (49).
Furthermore, more and more incidental findings of thoracic aneurysm are being picked up by imaging which create a burden to the health care system for the follow-up and management. With the help of radiogenomics, the risk of disease progression can be better estimated, thus providing a cost-effective solution for image surveillance and work up. In certain cases, with high-risk features identified, cascade testing can be initiated in which relatives of the proband are involved in the analysis using imaging or genetic testing. The proposed schematic summary of radiogenomics study workflow is shown in Figure 1.
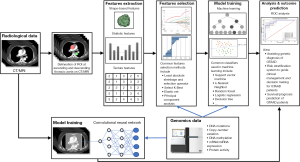
Challenge and limitation
One of the major limitations of the current radiogenomics model is the generalizability. In other words, the result from one study is not replicable to other studies and thus hinder the research development across multi-centers. There was no standardization on data and image format, heterogeneity existed among different equipment and software. Particularly the low resolution of images may limit the applicability of machine learning algorithms and affect the accuracy of analysis. There is also lack of transparency in the AI tools which involves complex statistical manipulation, and the user interface was not user-friendly for clinicians and researchers (46). The use of an open-source platform like PyRadiomics (44) might be a possible solution. The algorithms and source codes are open for access and transparent to researchers, developers, and users. It facilitates different stakeholders to get involved into the development process to come up with a consensus and unified protocol. Moreover, specific features such as the user interface can be tailor made with special toolkits that can be add-on accordingly.
For the machine learning part, a large number of samples from the development dataset are involved in order to build up a robust predictive model. There might be errors introduced during manual segmentation which involve discrepancy in interpretation by different clinicians or researchers. Moreover, it was labor intensive and time consuming for annotations in the establishment of supervised machine learning models. Although the use of automatic segmentation can minimize the inter-personal discrepancy and improve the efficiency to some extent, the accuracy of interpretation cannot be guaranteed.
So far, our understanding in the mechanism of the identified genetic variants is limited and there are plenty of missing links to be discovered via future research.
Future work
In the contemporary era, radiogenomics has emerged as a state-of-the art approach to establish statistical correlation by utilizing radiomics features with genomic information. With the effort in genetic testing, refining the AI algorithm, improving imaging resolution and quality, more pathogenic variants causing TAAD can be identified, thus enabling us to have a better understanding on the pathophysiology of genetically triggered TAAD. Clinical databases can be updated and further verified accordingly to construct sophisticated and precise risk prediction models. The management of aortic aneurysm and dissection can be individualized according to the risk stratification model for personalized medicine.
Conclusions
With advancements of genomic sequencing and computing technology, the standardization of the workflow, and the development of more robust and precise AI algorithm, radiogenomics is expected to provide more information on genetic triggered aortic aneurysm and dissection for patients and doctors. We hope to see in one day after a CT scan is performed for a patient, there will be an automatic analysis of the imaging data and the associated radiomics features, and together with patient’s ethnicity, basic demographics, family history etc., a polygenic score can be generated in predicting the patient is of low, moderate or high risk of developing aortic event. If the patient exhibits high-risk radiomics features, WGS can be done to confirm the diagnosis. Currently, there is already guideline to recommend surgical intervention at different aortic aneurysm size based on the type of genetically triggered thoracic aortic aneurysm. In conclusion, radiogenomics could potentially provide a rapid and timely guidance of the indication and timing of operation/interventions and personalized treatment strategy.
Acknowledgments
Funding: None.
Footnote
Reporting Checklist: The authors have completed the Narrative Review reporting checklist. Available at https://atm.amegroups.com/article/view/10.21037/atm-22-6149/rc
Peer Review File: Available at https://atm.amegroups.com/article/view/10.21037/atm-22-6149/prf
Conflicts of Interest: All authors have completed the ICMJE uniform disclosure form (available at https://atm.amegroups.com/article/view/10.21037/atm-22-6149/coif). RHLW serves as an unpaid editorial board member of Annals of Translational Medicine from September 2022 to August 2024. The other authors have no conflicts of interest to declare.
Ethical Statement: The authors are accountable for all aspects of the work in ensuring that questions related to the accuracy or integrity of any part of the work are appropriately investigated and resolved.
Open Access Statement: This is an Open Access article distributed in accordance with the Creative Commons Attribution-NonCommercial-NoDerivs 4.0 International License (CC BY-NC-ND 4.0), which permits the non-commercial replication and distribution of the article with the strict proviso that no changes or edits are made and the original work is properly cited (including links to both the formal publication through the relevant DOI and the license). See: https://creativecommons.org/licenses/by-nc-nd/4.0/.
References
- Wong RHL, Yang F, Fujikawa T, et al. Pocket-size Mobile Echocardiography screening for thoracic aortic aneurysm in hypertensive patients. Ann Thorac Surg 2021;111:1554-9. [Crossref] [PubMed]
- Evangelista A, Isselbacher EM, Bossone E, et al. Insights From the International Registry of Acute Aortic Dissection: A 20-Year Experience of Collaborative Clinical Research. Circulation 2018;137:1846-60. [Crossref] [PubMed]
- Duan WX, Wang WG, Xia L, et al. Clinical profiles and outcomes of acute type A aortic dissection and intramural hematoma in the current era: lessons from the first registry of aortic dissection in China. Chin Med J (Engl) 2021;134:927-34. [Crossref] [PubMed]
- Rooprai J, Boodhwani M, Beauchesne L, et al. Central Hypertension in Patients With Thoracic Aortic Aneurysms: Prevalence and Association With Aneurysm Size and Growth. Am J Hypertens 2022;35:79-86. [Crossref] [PubMed]
- Chiu P, Lee HP, Dalal AR, et al. Relative strain is a novel predictor of aneurysmal degeneration of the thoracic aorta: An ex vivo mechanical study. JVS Vasc Sci 2021;2:235-46. [Crossref] [PubMed]
- Gulati A, Leach J, Wang Z, et al. Ascending thoracic aortic aneurysm growth is minimal at sizes that do not meet criteria for surgical repair. Quant Imaging Med Surg 2022;12:333-40. [Crossref] [PubMed]
- Monti CB, van Assen M, Stillman AE, et al. Evaluating the Performance of a Convolutional Neural Network Algorithm for Measuring Thoracic Aortic Diameters in a Heterogeneous Population. Radiol Artif Intell 2022;4:e210196. [Crossref] [PubMed]
- Wobben LD, Codari M, Mistelbauer G, et al. Deep Learning-Based 3D Segmentation of True Lumen, False Lumen, and False Lumen Thrombosis in Type-B Aortic Dissection. Annu Int Conf IEEE Eng Med Biol Soc 2021;2021:3912-5. [Crossref] [PubMed]
- Guo T, Fang Z, Yang G, et al. Machine Learning Models for Predicting In-Hospital Mortality in Acute Aortic Dissection Patients. Front Cardiovasc Med 2021;8:727773. [Crossref] [PubMed]
- Lyu T, Yang G, Zhao X, et al. Dissected aorta segmentation using convolutional neural networks. Comput Methods Programs Biomed 2021;211:106417. [Crossref] [PubMed]
- Adam C, Fabre D, Mougin J, et al. Pre-surgical and Post-surgical Aortic Aneurysm Maximum Diameter Measurement: Full Automation by Artificial Intelligence. Eur J Vasc Endovasc Surg 2021;62:869-77. [Crossref] [PubMed]
- Beetz NL, Maier C, Shnayien S, et al. Artificial intelligence-based analysis of body composition in Marfan: skeletal muscle density and psoas muscle index predict aortic enlargement. J Cachexia Sarcopenia Muscle 2021;12:993-9. [Crossref] [PubMed]
- Zhou Z, Yang J, Wang S, et al. The diagnostic value of a non-contrast computed tomography scan-based radiomics model for acute aortic dissection. Medicine (Baltimore) 2021;100:e26212. [Crossref] [PubMed]
- Bratt A, Blezek DJ, Ryan WJ, et al. Deep Learning Improves the Temporal Reproducibility of Aortic Measurement. J Digit Imaging 2021;34:1183-9. [Crossref] [PubMed]
- Yu Y, Gao Y, Wei J, et al. A Three-Dimensional Deep Convolutional Neural Network for Automatic Segmentation and Diameter Measurement of Type B Aortic Dissection. Korean J Radiol 2021;22:168-78. [Crossref] [PubMed]
- Harris RJ, Kim S, Lohr J, et al. Classification of Aortic Dissection and Rupture on Post-contrast CT Images Using a Convolutional Neural Network. J Digit Imaging 2019;32:939-46. [Crossref] [PubMed]
- Wojnarski CM, Roselli EE, Idrees JJ, et al. Machine-learning phenotypic classification of bicuspid aortopathy. J Thorac Cardiovasc Surg 2018;155:461-469.e4. [Crossref] [PubMed]
- Liang L, Liu M, Martin C, et al. A machine learning approach to investigate the relationship between shape features and numerically predicted risk of ascending aortic aneurysm. Biomech Model Mechanobiol 2017;16:1519-33. [Crossref] [PubMed]
- Guo Y, Chen X, Lin X, et al. Non-contrast CT-based radiomic signature for screening thoracic aortic dissections: a multicenter study. Eur Radiol 2021;31:7067-76. [Crossref] [PubMed]
- Benjamins JW, Yeung MW, van de Vegte YJ, et al. Genomic insights in ascending aortic size and distensibility. EBioMedicine 2022;75:103783. [Crossref] [PubMed]
- Pirruccello JP, Chaffin MD, Chou EL, et al. Deep learning enables genetic analysis of the human thoracic aorta. Nat Genet 2022;54:40-51. [Crossref] [PubMed]
- Miner GH, Renton AE, Taubenfeld E, et al. Whole genome sequencing identifies loci specifically associated with thoracic aortic wall defects and abdominal aortic aneurysms in patients with European ancestry. JVS Vasc Sci 2020;1:233-45. [Crossref] [PubMed]
- Francis CM, Futschik ME, Huang J, et al. Genome-wide associations of aortic distensibility suggest causality for aortic aneurysms and brain white matter hyperintensities. Nat Commun 2022;13:4505. [Crossref] [PubMed]
- Cecchi AC, Drake M, Campos C, et al. Current state and future directions of genomic medicine in aortic dissection: A path to prevention and personalized care. Semin Vasc Surg 2022;35:51-9. [Crossref] [PubMed]
- Murana G, Pantaleo A, Parolari A, et al. Genetically Triggered Thoracic Aortic Aneurysms and Cardiovascular Conditions (GenTAC) registry predicting predictors for aortic dissection: a new thought around the corner? J Thorac Dis 2016;8:E1093-5. [Crossref] [PubMed]
- Richards S, Aziz N, Bale S, et al. Standards and guidelines for the interpretation of sequence variants: a joint consensus recommendation of the American College of Medical Genetics and Genomics and the Association for Molecular Pathology. Genet Med 2015;17:405-24. [Crossref] [PubMed]
- Chou EL, Lindsay ME. The genetics of aortopathies: Hereditary thoracic aortic aneurysms and dissections. Am J Med Genet C Semin Med Genet 2020;184:136-48. [Crossref] [PubMed]
- Biddinger A, Rocklin M, Coselli J, et al. Familial thoracic aortic dilatations and dissections: a case control study. J Vasc Surg 1997;25:506-11. [Crossref] [PubMed]
- Ziganshin BA, Bailey AE, Coons C, et al. Routine Genetic Testing for Thoracic Aortic Aneurysm and Dissection in a Clinical Setting. Ann Thorac Surg 2015;100:1604-11. [Crossref] [PubMed]
- Li Y, Fang M, Yang J, et al. Analysis of the contribution of 129 candidate genes to thoracic aortic aneurysm or dissection of a mixed cohort of sporadic and familial cases in South China. Am J Transl Res 2021;13:4281-95. [PubMed]
- Evangelista A, Teixido-Tura G. Genetically Triggered Aortic Disease Outcomes: On the Long Road to Personalized Medicine. J Am Coll Cardiol 2022;79:2082-4. [Crossref] [PubMed]
- Ramlingam G, Natarajasundaram UM. Ghent Criteria an Aid to Diagnose Latent Systemic Diseases in Marfan Syndrome. J Clin Diagn Res 2015;9:ZJ01-2. [Crossref] [PubMed]
- Ostberg NP, Zafar MA, Ziganshin BA, et al. The Genetics of Thoracic Aortic Aneurysms and Dissection: A Clinical Perspective. Biomolecules 2020;10:182. [Crossref] [PubMed]
- Wang Z, Zhuang X, Chen B, et al. 99-Case Study of Sporadic Aortic Dissection by Whole Exome Sequencing Indicated Novel Disease-Associated Genes and Variants in Chinese Population. Biomed Res Int 2020;2020:7857043. [Crossref] [PubMed]
- Chen ZR, Bao MH, Wang XY, et al. Genetic variants in Chinese patients with sporadic Stanford type A aortic dissection. J Thorac Dis 2021;13:4008-22. [Crossref] [PubMed]
- Li B, Ritchie MD. From GWAS to Gene: Transcriptome-Wide Association Studies and Other Methods to Functionally Understand GWAS Discoveries. Front Genet 2021;12:713230. [Crossref] [PubMed]
- Gamazon ER, Wheeler HE, Shah KP, et al. A gene-based association method for mapping traits using reference transcriptome data. Nat Genet 2015;47:1091-8. [Crossref] [PubMed]
- Gusev A, Ko A, Shi H, et al. Integrative approaches for large-scale transcriptome-wide association studies. Nat Genet 2016;48:245-52. [Crossref] [PubMed]
- Bhattacharya A, Li Y, Love MI. MOSTWAS: Multi-Omic Strategies for Transcriptome-Wide Association Studies. PLoS Genet 2021;17:e1009398. [Crossref] [PubMed]
- Lambin P, Rios-Velazquez E, Leijenaar R, et al. Radiomics: extracting more information from medical images using advanced feature analysis. Eur J Cancer 2012;48:441-6. [Crossref] [PubMed]
- Vieira Santos-Silva M, Sousa-Nunes F, Fernando Teixeira J, et al. The Use of Radiomic Analysis in Cardiovascular Diseases. Port J Card Thorac Vasc Surg 2022;29:45-50. [PubMed]
- Zhang X, Zhang Y, Zhang G, et al. Deep Learning With Radiomics for Disease Diagnosis and Treatment: Challenges and Potential. Front Oncol 2022;12:773840. [Crossref] [PubMed]
- Zwanenburg A, Vallières M, Abdalah MA, et al. The Image Biomarker Standardization Initiative: Standardized Quantitative Radiomics for High-Throughput Image-based Phenotyping. Radiology 2020;295:328-38. [Crossref] [PubMed]
- van Griethuysen JJM, Fedorov A, Parmar C, et al. Computational Radiomics System to Decode the Radiographic Phenotype. Cancer Res 2017;77:e104-7. [Crossref] [PubMed]
- Ding N, Hao Y, Wang Z, et al. CT texture analysis predicts abdominal aortic aneurysm post-endovascular aortic aneurysm repair progression. Sci Rep 2020;10:12268. [Crossref] [PubMed]
- Infante T, Cavaliere C, Punzo B, et al. Radiogenomics and Artificial Intelligence Approaches Applied to Cardiac Computed Tomography Angiography and Cardiac Magnetic Resonance for Precision Medicine in Coronary Heart Disease: A Systematic Review. Circ Cardiovasc Imaging 2021;14:1133-46. [Crossref] [PubMed]
- Shui L, Ren H, Yang X, et al. The Era of Radiogenomics in Precision Medicine: An Emerging Approach to Support Diagnosis, Treatment Decisions, and Prognostication in Oncology. Front Oncol 2020;10:570465. [Crossref] [PubMed]
- Wolford BN, Hornsby WE, Guo D, et al. Clinical Implications of Identifying Pathogenic Variants in Individuals With Thoracic Aortic Dissection. Circ Genom Precis Med 2019;12:e002476. [Crossref] [PubMed]
- Deleeuw V, De Clercq A, De Backer J, et al. An Overview of Investigational and Experimental Drug Treatment Strategies for Marfan Syndrome. J Exp Pharmacol 2021;13:755-79. [Crossref] [PubMed]