Study on the pharmacological mechanisms of sodium-glucose co-transporter 2 inhibitors in obesity-related atrial fibrillation based on network pharmacology and experimental verification
Highlight box
Key findings
• Pharmacological network analysis and in vivo experiments demonstrated that sodium-glucose co-transporter 2 inhibitors (SGLT2i) may treat obesity-related atrial fibrillation (AF) by inhibiting the advanced glycation end product (AGE)-receptor for advanced glycation end product (RAGE) signaling pathway.
What is known and what is new?
• SGLT2i are known to be effective at weight loss in patients with type 2 diabetes, and administration of SGLT2i is related to a decreased probability of AF occurrence or recurrence.
• The results herein suggested that the mechanisms of SGLT2i in treating obesity-related AF may involve the AGE-RAGE signal pathway and other signal pathways.
What is the implication, and what should change now?
• These studies shed new light on the treatment of obesity-related AF and contribute to our understanding of the role of SGLT2i. Further clinical studies are warranted to verify these results.
Introduction
Atrial fibrillation (AF) is the most prevalent persistent cardiac arrhythmia in adults. It is associated with heightened morbidity and mortality, and exerts a significant financial burden on international health budgets (1,2). The efficacy of current treatments are limited, and measures aimed at reducing the risk of AF onset and progression are urgently required to halt the AF epidemic and improve outcomes for those who are affected (3,4). While there are many risk factors for AF, numerous studies have demonstrated that obesity is an independent risk factor. Furthermore, the incidence of AF rises as the body mass index (BMI) increases (4-6). Thus, the global burden of AF will likely increase in the ensuring decades due to the current obesity epidemic (7). Despite the obesity paradox, factors such as age, lean body mass, and follow-up duration need to be considered (8). The so-called obesity paradox places more emphasis on people who are overweight, have high BMIs, and yet, are nevertheless “metabolically healthy” (9). Before overt metabolic derangement and the emergence of cardiovascular illnesses, this condition might only be temporary (9), and therefore, interventions aimed at weight reduction are important in decreasing the risk of AF (10,11).
A prospective study with 11.2 years of follow-up showed that the risk of AF was about 40.0% and 70.0% higher in diabetic men and women, respectively, compared with non-diabetic individuals (12). Sodium glucose cotransporter 2 inhibitors (SGLT2i) are novel oral medications used to treat people with type 2 diabetes mellitus (T2DM), and regardless of the underlying medication, clinical trials have indicated that SGLT2i cause individuals with T2DM to lose, on average, 2 to 3 kg of weight (13). In addition, some large randomized controlled trials (RCTs) and meta-analyses have shown that SGLT2i confer a benefit on cardiovascular outcomes (14-16). Tabulated data from 31 RCTs (involving 75,279 participants with a mean follow-up time of 2.6 years) showed that SGLT2i were associated with a decreased probability of AF occurrence or recurrence (17). A prospective follow-up study of medical data from a multi-center healthcare provider in Taiwan showed that SGLT2i use were associated with a lower risk of new AF compared with DPP4i (18). In a meta-analysis involving 22 trials and 52,115 patients, SGLT2i were associated with an 18% reduction in the risk of AF/atrial flutter (AFL) (19). However, the therapeutic targets and molecular processes behind the action of SGLT2i in the therapy of AF have not yet been fully uncovered (20,21), particularly in individuals with obesity.
With the rapid advancement of systems biology, bioinformatics, and multivariate polypharmacology, network pharmacology can effectively analyze the relationship between medicines, diseases, and targets through various types of databases, high-throughput omics data processing, and computer simulations (22). Consequently, this current investigation used network pharmacology to explore the genes and biological pathways targeted by SGLT2i in treating obesity-related AF. In vivo studies were conducted to further verify the mechanisms of SGLT2i in treating obesity-related AF. The overall flowchart of this investigation is shown in Figure 1. We present the following article in accordance with the ARRIVE reporting checklist (available at https://atm.amegroups.com/article/view/10.21037/atm-23-1321/rc).
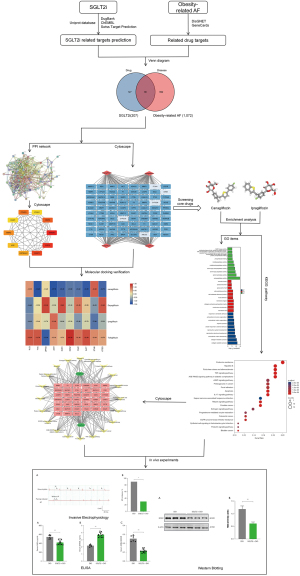
Methods
Prediction of SGLT2i-related targets
A protocol was prepared before the study without registration. Pubchem (https://pubchem.ncbi.nlm.nih.gov/) is a publicly available online chemical database with 96,502,248 constituents, of which, 3,151,393 have undergone testing. The database was used to access the chemical structures of four SGLT2i, namely, canagliflozin, dapagliflozin, ipragliflozin, and tofogliflozin. The chemical structural formulas of the four SGLT2i were input into the SwissTargetPrediction platform (http://www.swisstargetprediction.ch/). This online tool predicts targets by using measures of similarity with known ligands in two and three dimensions to forecast targets and potential targets. DrugBank (https://www.drugbank.ca/), a noteworthy cheminformatics and bioinformatics database with 11,628 pharmaceuticals and related drug-targets, chemical details, protein target data, and other information, was also used to find the targets of SGLT2i as a supplement (23).
ChEMBL is a manually managed database of bioactive compounds that possess drug-like characteristics. By combining biological activity, pharmacological activity, and genomic data, it helps to translate genomic information into efficient novel medications. The targets of SGLT2i drugs were queried in this database as a supplement. The retrieval function of Universal Protein database (Uniprot) was used to rectify and convert the targets of SGLT2i, to ensure consistency in the symbols of the linked genes for subsequent analyses.
The acquisition of potential therapeutic targets for SGLT2i in treating obesity-related AF
Target genes associated with obesity and AF were searched in GeneCards databases (https://www.genecards.org) and DisGeNET databases (https://www.disgenet.org/) using the keywords “obesity” and “atrial fibrillation”. More than 7,000 human genes are contained in the GeneCards database, and each gene is assigned a recognized gene symbol (24). DisGeNET includes the whole range of human disorders in addition to typical and aberrant traits. The current release includes 117,000 genome-scale reconstructions, 17,000 genes, and more than 24,000 illnesses and characteristics (25). The targets of SGLT2i in treating obesity-related AF were derived from the intersection of diseases and drug targets. Microsoft Excel 2017 was used to eliminate duplicate values in drug targets and diseases, and the two targets were input into the Venn diagram drawing function module of Xiantao Academic Online Tool (https://www.xiantao.love/) to obtain the drugs and diseases intersection targets for SGLT2i in treating obesity-related AF. The study was conducted in accordance with the Declaration of Helsinki (as revised in 2013).
Construction of the protein-protein interaction (PPI) network and acquisition of hub genes
STRING is a large online database that annotates each PPI with one or more “scores”. The annotative scores are indicators of confidence. The confidence increases as the score rises. In this platform, “Homo sapiens” was chosen as the organism and “Multiple proteins” mode was selected. The “drug-disease” targets were entered into the “List Of names” for retrieval and analysis, thereby establishing a PPI network diagram of SGLT2i in treating obesity-related AF. The threshold for scoring was set at >0.40, and the network’s unconnected nodes were concealed. The obtained results were then exported to the TSV format for subsequent analysis using Cytoscape V3.7.1. Cytoscape is a powerful software program for efficiently expressing genetic, protein-DNA, and protein-protein interactions, and visualizing complex network relationships. It further analyses the potential relationship between proteins, target genes, etc.
The nodes of the PPI network represent proteins. A single protein-coding gene locus is represented by a node, which contains all the proteins produced by that locus. The edges are intended to reflect specific and significant PPIs. Edges are from curated databases or experimentally determined, and these include predicted interactions such as gene co-occurrence, gene neighborhood, etc. To obtain the hub genes of SGLT2i in treating obesity-related AF, the CytoHubba plugin in Cytoscape 3.7.1 software was used, which provides Degree Method, Maximum Clique Centrality (MCC), BottleNeck, Betweenness, Closeness, Density of Maximum Neighborhood Component (DMNC), EcCentricity, Edge Percolated Component (EPC), Maximum Neighborhood Cmomponent (MNC), Radiality, and Stress.
Screening core drugs
The Network Analyzer computes topological parameters for directed networks as well as undirected networks, and can calculate each node’s Degree, Clustering Coefficient, Closeness Centrality, Betweenness Centrality, and Neighborhood Connectivity. Combined with the targets of SGLT2i, we used Cytoscape3.7.1 to establish a Drug-Target network. To more effectively screen out the core drug, we performed topology analysis on the nodes in the Drug-Target network using the network analyzer calculation feature of the program.
Gene Ontology (GO) and Kyoto Encyclopedia of Genes and Genomes (KEGG) pathway enrichment analysis and the construction of “core drug-targets-KEGG” network
GO uses the study of biological processes (BPs), cell components (CCs), and molecular function (MFs) to define and describe the function of a gene product. The KEGG is a database that combines data on systematic, chemical, and genomic functions. R language is an effective programming language for statistics and analysis. There are many different installation packages available for different scenarios and requirements, one of which is the clusterProfiler package that is often used for pathway functional enrichment analysis of genome and GO. In this study, enrichment analysis using clusterProfiler packages (adjusted P<0.05) was performed on the screened core drugs to gain a more intuitive understanding of the GO and KEGG pathway involved in the treatment of obesity-related AF. As a software for visualizing network relationships, Cytoscape can draw network diagrams based on data imported by users. The establishment of the network requires an understanding of the relationships of each node, such as the targets of drug action and the targets contained in the KEGG pathway. These were imported into Cytoscape to visualize the “core drug-targets-KEGG” network.
Molecular docking of hub genes
The hub genes identified in the PPI network were used as protein receptors for molecular docking, and SGLT2i were used as molecular docking ligands. Raw files (MOL2 format) of SGLT2i compounds were obtained from the PubChem database (https://pubchem.ncbi.nlm.nih.gov/). The raw files (PDB format) of the top 10 hub genes were downloaded from RCSB Protein Data (https://www.rcsb.org/) and processed accordingly using the AutoDockVina tool. Molecular docking reflects the binding affinity between molecules. The smaller the value, the stronger the binding affinity between small molecule ligands and macromolecular receptors.
In vivo experiments
Animal studies
Healthy C57BL/6J male mice, aged 4 weeks, were purchased from SPF Biotechnology Co., Ltd (Beijing, China) and housed under a 12-hour light/dark cycle, with free access to food and water. After acclimatization for two weeks, the mice were randomly divided into two groups, namely, the diet-induced obese (DIO) group and the SGLT2i + DIO group. Both groups received a high-fat diet (HFD, 60% of calories from fat, D12492, Research Diets) for 14 weeks to induce obesity. During these 14 weeks, the DIO group was given a vehicle solution of 0.5% methylcellulose daily, by oral gavage. Over the same period of time, the SGLT2i + DIO group was given canagliflozin (Selleckchem, Houston, TX, 60 mg/kg/d), dissolved in 0.5% methylcellulose solution, daily via gavage for 14 weeks. The body weight, as well as the water and food intake were recorded once a week. Animal experiments were performed under a project license (No. 2020-X16-101) granted by ethics board of Chinese PLA General Hospital Medical Laboratory Animal Center, in compliance with institutional guidelines for the care and use of animals.
Invasive electrophysiology
AF was induced in mice through esophagus atrial pacing, as previously described (26). Briefly, mice were anesthetized using 1–2% isoflurane in 95% oxygen. A surface 3-lead electrocardiogram (ECG) was obtained by attaching cutaneous clips to each limb. Through esophagus atrial pacing was performed on all mice. The 1.1Fr octapolar catheter (EPR-800, Millar Instruments, Houston, TX, USA) was inserted in the esophagus near the left atrium. Using a PowerLab instrument (AD Instruments Ltd, AUS), an intra-esophageal electrogram, as well as a three-lead ECG on the body surface, were digitally recorded. Atrial pacing was produced using a stimulator (Master-8, AMPI). A fast, irregular atrial rhythm that lasted at least three seconds was considered to be an episode of AF. The mice were considered to have AF if at least one burst elicited an episode. Otherwise, AF was deemed to be non-inducible.
Enzyme-linked immunosorbent assay (ELISA)
The Mouse Advanced Glycation End Products ELISA KIT (ED-20673, Lun Chang Shuo Biotech) and the Mouse Receptor for Advanced Glycation End Products ELISA KIT (ED-20674, Lun Chang Shuo Biotech) were used to measure serum advanced glycation end products (AGEs) and soluble receptor for advanced glycation end products (sRAGE), respectively, as per the manufacturer’s protocols. The level of AGEs (pg/mL) was divided by the level of sRAGE (ng/mL) to obtain a ratio.
Western blotting
Cryopreserved mouse heart tissue samples were ground in liquid nitrogen, homogenized in lysis buffer, and the protein levels were measured using the commercial kit (Thermo Fisher, USA). Protein samples were separated on 10% sodium dodecyl sulfate (SDS)-polyacrylamide gels (20 µg/lane) under denaturing conditions, and transferred to polyvinylidene fluoride (PVDF) membranes, followed by blocking with 5% skim milk prepared in 1× Tris buffered saline Tween (TBST) for 1–2 hours. Membranes were then incubated with the primary antibodies overnight or for 24 hours at 4 °C, followed by a secondary horseradish peroxidase (HRP)-conjugated antibody. The primary antibodies used were anti-NOX2 (ab129068, Abcam, England) and anti-β-actin (20536-1-AP, Proteintech Group, Chicago, IL, USA), while the secondary antibodies used were HRP-goat anti-rabbit IgG (074-1506, KPL, USA) and HRP-goat anti-mouse IgG (074-1806, KPL, USA). The protein bands were visualized using the ECL luminescent developer.
Statistical analysis
Categorical data are shown as counts and percentages, while continuous data are presented as mean ± standard deviation (SD). The χ2 test for independence and the Fisher exact test were used to compare two categorical variables when sample sizes were less than 5. Bivariate analysis of a continuous and categorical variable was performed using 2 sample t tests or repeated measures analysis of variance (ANOVA), as applicable. A P value <0.05 was two-sided and considered statistically significant. GraphPad Prism 9.0 software was used for all statistical analyses.
Results
Network pharmacology analysis
Probable relevant targets of SGLT2i in treating obesity-related AF
Using the PubChem system, the molecular structures of canagliflozin, dapagliflozin, ipragliflozin, and tofogliflozin were determined. Detailed information and structural formulas for the four SGLT2i are shown in Table S1.
The structures of the four SGLT2i were imported into the SwissTargetPrediction database to predict and match targets. A total of 229 targets were identified with probability >0, which included 53 targets for canagliflozin, 60 targets for dapagliflozin, 59 targets for ipragliflozin, and 57 targets for tofogliflozin. Furthermore, the relevant targets of the four SGLT2i were analyzed using the DrugBank database and 19 targets were found, with 8 targets for canagliflozin, 11 targets for danagliflozin, and 0 targets for ipragliflozin and tofogliflozin. The targets of SGLT2i were queried in the ChEMBL database. The targets relating to “Homo sapiens” were selected. In addition, the targets that did not satisfy the requirements for inclusion and duplicated targets were excluded. A total of 116 targets were obtained. By merging the targets attained from the SWisstar Prediction, DrugBank, and ChEMBL databases, 207 targets were obtained after eliminating the duplicates.
After mining the public database of diseases, the information about each target database related to AF is as follows: GeneCards (number: 1,659), and DisGeNET (number: 939). The information about each target database related to obesity is as follows: GeneCards (number: 5,095), and DisGeNET (number: 2,821). A total of 1,072 disease targets associated with obesity and AF were obtained by intersecting the above targets. The intersection of the predicted targets of SGLT2i and the targets of the disease represented the potential targets for SGLT2i in treating obesity-related AF. As depicted in Figure 2, we constructed a Venn diagram to screen the overlapping targets, with 80 targets obtained.
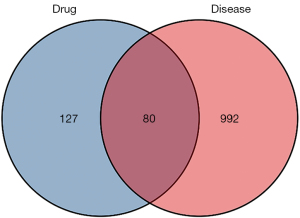
Construction of the PPI network for SGLT2i in treating obesity-related AF and identification of the hub genes
Using the STRING database, we set the species as “Homo sapiens” and inputted 80 potential targets of SGLT2i in treating obesity-related AF to produce a PPI network, involving 80 nodes and 606 sides (Figure 3A). The TSV files obtained in the STRING online analysis tool were imported into Cytoscape 3.7.1 for visualization and analysis (Figure 3B). Using the aforementioned PPI network, we conducted research using the Cytohubba plugin for Cytoscape software, which at the moment supports 12 topological analysis techniques. Based on the intensity of the degree value, the top 10 hub genes were screened (Figure 3C).
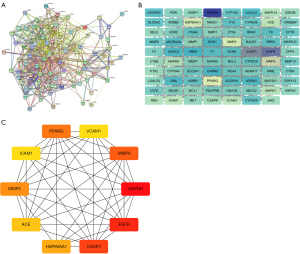
GO and KEGG pathway enrichment analysis and “core drug-targets-KEGG” network
According to the target and basic information of drug action, the “drug-target” network (Figure 4) was plotted using Cytoscape3.7.1, and the topological value features of the drug nodes were calculated using the computing function of a network analyzer, with the degree taken as the screening criterion. The top two drugs, canagliflozin and ipragliflozin, were selected as core drugs.
GO and KEGG pathway enrichment analyses were used to investigate biological processes, signaling pathways, and important genes related to SGLT2i in treating obesity-related AF. GO analysis on the potential therapeutic targets of canagliflozin and ipragliflozin in treating obesity-related AF revealed 1,274 items, including 1,126 BPs, 64 CCs, and 84 MFs (adjusted P<0.05). Figure 5 shows the top ten enrichment results and the basic information is shown in Table S2.
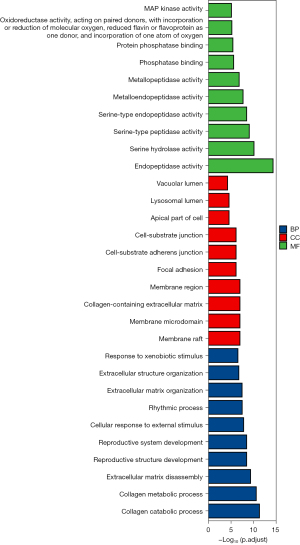
Similarly, KEGG pathway analysis of the potential targets of canagliflozin and ipragliflozin in the treatment of obesity-related AF resulted in a total of 141 items (adjusted P<0.05). The top 20 KEGG signaling pathways were endocrine resistance (hsa01522), AGE-RAGE signaling pathway in diabetic complications (hsa04933), tumor necrosis factor (TNF) signaling pathway (hsa04668), interleukin (IL)-17 signaling pathway (hsa04657), fluid shear stress and atherosclerosis (hsa05418), prostate cancer (hsa05215), hepatitis B (hsa05161), apoptosis (hsa04210), bladder cancer (hsa05219), relaxin signaling pathway (hsa04926), epidermal growth factor receptor (EGFR) tyrosine kinase inhibitor resistance (hsa01521), colorectal cancer (hsa05210), focal adhesion (hsa04510), proteoglycans in cancer (hsa05205), cAMP signaling pathway (hsa04024), progesterone-mediated oocyte maturation (hsa04914), prolactin signaling pathway (hsa04917), epithelial cell signaling in Helicobacter pylori infection (hsa05120), estrogen signaling pathway (hsa04915), and Kaposi sarcoma-associated herpesvirus infection (hsa05167) (Figure 6). Subsequently, the Cytoscape software was used to visualize the network relationship between potential therapeutic targets and pathways of canagliflozin and ipragliflozin in the treatment of obesity-related AF (Figure 7). Table S3 provides more details on the 20 channels.
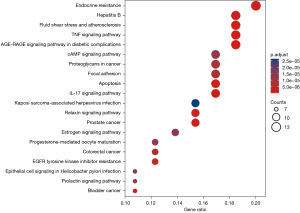
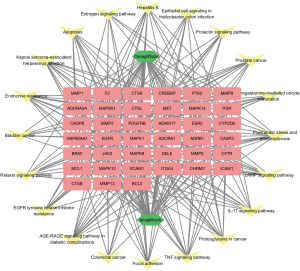
Findings from hub gene molecular docking
The top 10 hub genes identified in the PPI network were used as receptor macromolecules and SGLT2i were used as the ligand small molecule for molecular docking. Based on the binding energy score, a heat map was drawn (Figure 8). The binding energy ranged from −9.70 to 16.60 kcal·mol-1, indicating that the small molecule ligand (SGLT2i) bound well to the top hub genes.
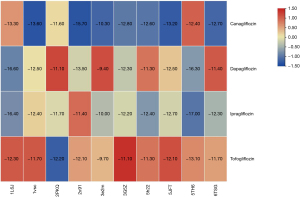
In vivo experimental verification
Effects of SGLT2i on inducibility of obesity-related AF
The induction rate of AF in mice was observed by transesophageal stimulation. Mice in the DIO group had a higher rate of AF induction compared to mice in the SGLT2i + DIO group (90.0% vs. 30.7%, P<0.05; Figure 9). The surface ECG showed loss of P waves and increased RR interval variability (Figure 9).
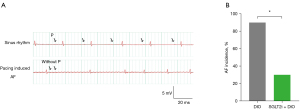
Serum levels of AGEs and sRAGE
The ELISA results demonstrated that the SGLT2i + DIO group had decreased level of serum AGEs, increased level of serum sRAGE, and decreased AGEs/sRAGE ratio when compared to the DIO group (P<0.05; Figure 10).
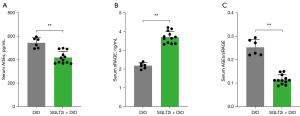
NOX2 expression in atrial tissues
Western blot analyses revealed that the SGLT2i + DIO group had lower NOX2 expression in atrial tissue compared to the DIO group (P<0.05; Figure 11).
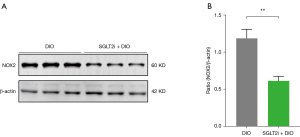
Discussion
Obesity and AF are significant public health issues (27). The increasing number of researches have indicated that obesity is an independent risk factor for AF (28-30), and with the burgeoning worldwide obesity epidemic, the burden of AF may escalate exponentially in the ensuing decades (31). Despite the obesity paradox, recent evidence suggests that the frequency of AF recurrence can be decreased with increased exercise and weight-loss programs (7). Sustained decrease of weight, especially with the prevention of weight fluctuations, was shown to be advantageous for a dose-dependent reduction in AF burden and maintenance of sinus rhythm (11). Therefore, obesity-targeted public health interventions could help combat the global burden of AF (32).
SGLT2i, a new hypoglycemic agent, is one of the most effective drugs used to decrease the weight of patients with T2DM (33). Of note, in patients with T2DM, body weight decrease (body weight loss ≥5.0%) after SGLT2i therapy was conducive to reducing the risk of newly developing AF (34). Furthermore, SGLT2i may help to reduce particular atrial flutter or AF not only in T2DM patients, but also in other high-risk groups (20,35). Interestingly, SGLT2i reduces body weight independently of diabetes (36). Relevant studies have shown that SGLT2i exerts cardioprotective effects through multiple mechanisms that are independent of its hypoglycemic effects (34). SGLT2i can lessen the structural and electrical remodeling of the atrium (37-39), reduce epicardial adipose tissue (EAT) volume or thickness (40), and exert a mitochondrial protective role (39). In addition, it has a certain effect on lowering blood pressure and uric acid levels (41,42), and prevents hypomagnesemia by regulating urinary magnesium excretion (43). Furthermore, by preventing ROS and NLRP3 inflammasome activation, SGLT2i treatment slows the development of liver steatosis and inflammation (44). However, the exact mechanisms by which SGLT2i lowers AF risk in patients with obesity-related AF remain unclear.
This current study explored the potential mechanisms of SGLT2i in treating obesity-related AF. Two main SGLT2i, including canagliflozin and ipragliflozin, were selected based on drug-target networks. A meta-analysis showed that canagliflozin was associated with a reduction in AF/atrial flutter (AFL) and related complications in patients with no previous AF/AFL history (45). Canagliflozin has also been demonstrated to be useful for preventing AF and suppressing the promotion of atrial remodeling as an AF substrate (46). As a highly selective SGLT2i, ipragliflozin causes weight loss (47). Previous literature suggested that compared to sitagliptin, ipragliflozin produced higher improvements in BMI (48). The 10 hub genes of SGLT2i in treating obesity-related AF obtained based on the PPI network were Glyceraldehyde-3-phosphate dehydrogenase (GAPDH), EGFR, Caspase-3 (CASP3), Matrix metalloproteinase-9 (MMP9), Peroxisome proliferator-activated receptor gamma (PPARG), Matrix metalloproteinase-2 (MMP2), Heat shock protein HSP 90-alpha (HSP90AA1), Angiotensin-converting enzyme (ACE), Intercellular adhesion molecule 1 (ICAM1), and Vascular cell adhesion protein 1 (VCAM1). EGFR is a member of the protein kinase superfamily and a key regulator in cell proliferation, differentiation, migration, survival, cancer development, and non-malignant disorders (49,50). In mouse models with HFD-induced cardiac injury, EGFR plays a cardioprotective role (51). Therefore, EGFR may be a potential treatment target for obesity-related AF. As a crucial downstream enzyme particularly activated in the apoptotic pathway, caspase-3 expression increases in the fibrillating atria of humans and canines (52,53). Trappe et al. suggested that by inhibiting or delaying caspase-3 using an adenovirus encoding for a respective silencing RNA (Ad-siRNA-Cas3) gene transfer in a domestic pig model of induced AF, the onset of persistent AF could be prevented (54). In addition, a previous study has shown that elevated levels of MMP-9 lead to excessive degradation of the extracellular matrix and increased tissue remodeling, which can cause myocardial fibrosis and micro-reentrants, ultimately leading to AF (55). A meta-analysis showed that compared to those without AF, MMP-9 was significantly higher in AF patients (56). High-fat feeding increases PPARG expression (57). Moreover, its downregulation has been associated with anti-obesity effects on healthy people (58). Therefore, targeting PPARG may be helpful in the treatment of obesity-related AF.
KEGG analysis revealed that the AGE-RAGE signaling pathway was a significant pathway of SGLT2i in treating obesity-related AF. In addition, SGLT2i reduced the risk of AF in vivo, in mice, and was positively associated with decreased expression of proteins associated with the AGE-RAGE signaling pathway compared with the DIO group. In animal models, a high-fat diet has been associated with obesity and elevated levels of serum and tissue AGEs (59). AGEs are produced by non-enzymatic glycosylation and oxidation of nucleic acids, proteins, and lipids, and the main receptor is the RAGE. RAGE is a multi-ligand cell surface receptor with four main isoforms, in which sRAGE acts as a pseudo-RAGE receptor by trapping RAGE ligand and inhibiting RAGE activation (60,61). It has been demonstrated that AGEs interact with the receptor for AGEs (RAGE) to cause inflammatory, thrombotic, and fibrotic responses in a number of cells (62-64). Additionally, according to studies, inflammatory responses may contribute to the buildup of AGEs in the left appendage, which would then play a role in atrial remodeling in AF (65,66). Various signaling pathways involving NADPH oxidase and protein kinase C can be activated by AGE-RAGE signaling pathway (67,68). AGE-RAGE interaction produces oxidative stress mainly through a NADPH oxidase-dependent mechanism to produce reactive oxygen species (ROS) (69). NADPH oxidase (NOX) is an enzyme complex that has attracted much attention as key molecules in the development of vascular dysfunction (70). The NOX family has seven members, with NOX2 being the predominant isoform in the heart (71) which is considered to be the major source of ROS (70). McCauley et al. reported that the expression of NOX2 protein in atrial tissue of DIO mice increased, and the induction rate of atrial fibrillation increased (72). Joseph et al. found that the absence of NOX2 or the pharmacological inhibition of NOX2 could prevent arrhythmia caused by a high-fat diet, and they suggested that NOX2 inhibition may be a novel therapy for obesity-induced arrhythmia (73). Our current study demonstrated that SGLT2i could downregulate NOX2 expression through the AGE-RAGE signaling pathway and thus reduce the occurrence of obesity-related AF (Figure 12). In addition, a relevant study has shown that SGLT2i can prevent the occurrence of atrial fibrillation by reducing inflammatory cytokines (74).
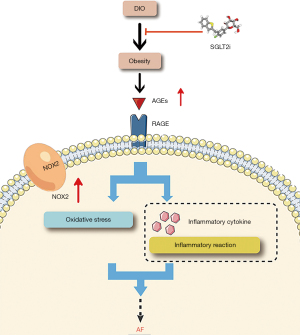
Limitations
The findings herein offer a theoretical foundation for using SGLT2i as a therapeutic agent for individuals with obesity-related AF. However, further studies are warranted to confirm its safety and effectiveness. Studies involving the knockdown and overexpression of pathway target molecules should be conducted to verify these results. Further clinical and experimental investigations are required to examine the mechanisms of SGLT2i in treating obesity-related AF, and to assess the onset and evolution of AF in well-defined groups of patients with or without obesity.
Conclusions
Our study elaborated on the potential mechanisms of SGLT2i in treating obesity-related AF by methodically predicting, screening, and analyzing targets and pathways that may be significant in biological processes and further validating the findings via in vivo experiments. Canagliflozin and ipragliflozin were determined to be effective drugs in treating obesity-related AF, and GAPDH, EGFR, CASP3, MMP9, PPARG, MMP2, HSP90AA1, ACE, ICAM1, VCAM1 were identified as hub genes. This investigation found that SGLT2i treated obesity-related AF by inhibiting the AGE-RAGE signaling pathway. In vivo experiments corroborated the above results, which may represent a novel therapeutic approach in obesity-related AF.
Acknowledgments
Funding: This work was supported by grants from the National Natural Science Foundation of China (Nos. 82070328, 81870249).
Footnote
Reporting Checklist: The authors have completed the ARRIVE reporting checklist. Available at https://atm.amegroups.com/article/view/10.21037/atm-23-1321/rc
Data Sharing Statement: Available at https://atm.amegroups.com/article/view/10.21037/atm-23-1321/dss
Peer Review File: Available at https://atm.amegroups.com/article/view/10.21037/atm-23-1321/prf
Conflicts of Interest: All authors have completed the ICMJE uniform disclosure form (available at https://atm.amegroups.com/article/view/10.21037/atm-23-1321/coif). The authors have no conflicts of interest to declare.
Ethical Statement: The authors are accountable for all aspects of the work in ensuring that questions related to the accuracy or integrity of any part of the work are appropriately investigated and resolved. The study was conducted in accordance with the Declaration of Helsinki (as revised in 2013). Animal experiments were performed under a project license (No. 2020-X16-101) granted by ethics board of Chinese PLA General Hospital Medical Laboratory Animal Center, in compliance with institutional guidelines for the care and use of animals.
Open Access Statement: This is an Open Access article distributed in accordance with the Creative Commons Attribution-NonCommercial-NoDerivs 4.0 International License (CC BY-NC-ND 4.0), which permits the non-commercial replication and distribution of the article with the strict proviso that no changes or edits are made and the original work is properly cited (including links to both the formal publication through the relevant DOI and the license). See: https://creativecommons.org/licenses/by-nc-nd/4.0/.
References
- Blomstrom Lundqvist C, Lip GY, Kirchhof P. What are the costs of atrial fibrillation? Europace 2011;13:ii9-12. [Crossref] [PubMed]
- Brüggenjürgen B, Rossnagel K, Roll S, et al. The impact of atrial fibrillation on the cost of stroke: the berlin acute stroke study. Value Health 2007;10:137-43. [Crossref] [PubMed]
- Brundel BJJM, Ai X, Hills MT, et al. Atrial fibrillation. Nat Rev Dis Primers 2022;8:21. [Crossref] [PubMed]
- Lau DH, Nattel S, Kalman JM, et al. Modifiable Risk Factors and Atrial Fibrillation. Circulation 2017;136:583-96. [Crossref] [PubMed]
- Middeldorp ME, Pathak RK, Meredith M, et al. PREVEntion and regReSsive Effect of weight-loss and risk factor modification on Atrial Fibrillation: the REVERSE-AF study. Europace 2018;20:1929-35. [Crossref] [PubMed]
- Wong CX, Sullivan T, Sun MT, et al. Obesity and the Risk of Incident, Post-Operative, and Post-Ablation Atrial Fibrillation: A Meta-Analysis of 626,603 Individuals in 51 Studies. JACC Clin Electrophysiol 2015;1:139-52. [Crossref] [PubMed]
- Lavie CJ, Pandey A, Lau DH, et al. Obesity and Atrial Fibrillation Prevalence, Pathogenesis, and Prognosis: Effects of Weight Loss and Exercise. J Am Coll Cardiol 2017;70:2022-35. [Crossref] [PubMed]
- Proietti M, Guiducci E, Cheli P, et al. Is There an Obesity Paradox for Outcomes in Atrial Fibrillation? A Systematic Review and Meta-Analysis of Non-Vitamin K Antagonist Oral Anticoagulant Trials. Stroke 2017;48:857-66. [Crossref] [PubMed]
- Goossens GH. The Metabolic Phenotype in Obesity: Fat Mass, Body Fat Distribution, and Adipose Tissue Function. Obes Facts 2017;10:207-15. [Crossref] [PubMed]
- Abed HS, Wittert GA, Leong DP, et al. Effect of weight reduction and cardiometabolic risk factor management on symptom burden and severity in patients with atrial fibrillation: a randomized clinical trial. JAMA 2013;310:2050-60. [Crossref] [PubMed]
- Pathak RK, Middeldorp ME, Meredith M, et al. Long-Term Effect of Goal-Directed Weight Management in an Atrial Fibrillation Cohort: A Long-Term Follow-Up Study (LEGACY). J Am Coll Cardiol 2015;65:2159-69. [Crossref] [PubMed]
- Smith JG, Platonov PG, Hedblad B, et al. Atrial fibrillation in the Malmö Diet and Cancer study: a study of occurrence, risk factors and diagnostic validity. Eur J Epidemiol 2010;25:95-102. [Crossref] [PubMed]
- Brown E, Wilding JPH, Barber TM, et al. Weight loss variability with SGLT2 inhibitors and GLP-1 receptor agonists in type 2 diabetes mellitus and obesity: Mechanistic possibilities. Obes Rev 2019;20:816-28. [Crossref] [PubMed]
- Perkovic V, Jardine MJ, Neal B, et al. Canagliflozin and Renal Outcomes in Type 2 Diabetes and Nephropathy. N Engl J Med 2019;380:2295-306. [Crossref] [PubMed]
- Zelniker TA, Wiviott SD, Raz I, et al. SGLT2 inhibitors for primary and secondary prevention of cardiovascular and renal outcomes in type 2 diabetes: a systematic review and meta-analysis of cardiovascular outcome trials. Lancet 2019;393:31-9. [Crossref] [PubMed]
- Böhm M, Slawik J, Brueckmann M, et al. Efficacy of empagliflozin on heart failure and renal outcomes in patients with atrial fibrillation: data from the EMPA-REG OUTCOME trial. Eur J Heart Fail 2020;22:126-35. [Crossref] [PubMed]
- Pandey AK, Okaj I, Kaur H, et al. Sodium-Glucose Co-Transporter Inhibitors and Atrial Fibrillation: A Systematic Review and Meta-Analysis of Randomized Controlled Trials. J Am Heart Assoc 2021;10:e022222. [Crossref] [PubMed]
- Ling AW, Chan CC, Chen SW, et al. The risk of new-onset atrial fibrillation in patients with type 2 diabetes mellitus treated with sodium glucose cotransporter 2 inhibitors versus dipeptidyl peptidase-4 inhibitors. Cardiovasc Diabetol 2020;19:188. [Crossref] [PubMed]
- Li HL, Lip GYH, Feng Q, et al. Sodium-glucose cotransporter 2 inhibitors (SGLT2i) and cardiac arrhythmias: a systematic review and meta-analysis. Cardiovasc Diabetol 2021;20:100. [Crossref] [PubMed]
- Li WJ, Chen XQ, Xu LL, et al. SGLT2 inhibitors and atrial fibrillation in type 2 diabetes: a systematic review with meta-analysis of 16 randomized controlled trials. Cardiovasc Diabetol 2020;19:130. [Crossref] [PubMed]
- Zheng RJ, Wang Y, Tang JN, et al. Association of SGLT2 Inhibitors With Risk of Atrial Fibrillation and Stroke in Patients With and Without Type 2 Diabetes: A Systemic Review and Meta-Analysis of Randomized Controlled Trials. J Cardiovasc Pharmacol 2022;79:e145-52. [Crossref] [PubMed]
- Ning K, Zhao X, Poetsch A, et al. Computational Molecular Networks and Network Pharmacology. Biomed Res Int 2017;2017:7573904. [Crossref] [PubMed]
- Wishart DS, Feunang YD, Marcu A, et al. HMDB 4.0: the human metabolome database for 2018. Nucleic Acids Res 2018;46:D608-17. [Crossref] [PubMed]
- Rebhan M, Chalifa-Caspi V, Prilusky J, et al. GeneCards: a novel functional genomics compendium with automated data mining and query reformulation support. Bioinformatics 1998;14:656-64. [Crossref] [PubMed]
- Piñero J, Ramírez-Anguita JM, Saüch-Pitarch J, et al. The DisGeNET knowledge platform for disease genomics: 2019 update. Nucleic Acids Res 2020;48:D845-55. [PubMed]
- Chen WJ, Chang SH, Chan YH, et al. Tachycardia-induced CD44/NOX4 signaling is involved in the development of atrial remodeling. J Mol Cell Cardiol 2019;135:67-78. [Crossref] [PubMed]
- Tsang TS, Barnes ME, Miyasaka Y, et al. Obesity as a risk factor for the progression of paroxysmal to permanent atrial fibrillation: a longitudinal cohort study of 21 years. Eur Heart J 2008;29:2227-33. [Crossref] [PubMed]
- Wang TJ, Parise H, Levy D, et al. Obesity and the risk of new-onset atrial fibrillation. JAMA 2004;292:2471-7. [Crossref] [PubMed]
- Frost L, Hune LJ, Vestergaard P. Overweight and obesity as risk factors for atrial fibrillation or flutter: the Danish Diet, Cancer, and Health Study. Am J Med 2005;118:489-95. [Crossref] [PubMed]
- Staerk L, Sherer JA, Ko D, et al. Atrial Fibrillation: Epidemiology, Pathophysiology, and Clinical Outcomes. Circ Res 2017;120:1501-17. [Crossref] [PubMed]
- Huxley RR, Lopez FL, Folsom AR, et al. Absolute and attributable risks of atrial fibrillation in relation to optimal and borderline risk factors: the Atherosclerosis Risk in Communities (ARIC) study. Circulation 2011;123:1501-8. [Crossref] [PubMed]
- Chatterjee NA, Giulianini F, Geelhoed B, et al. Genetic Obesity and the Risk of Atrial Fibrillation: Causal Estimates from Mendelian Randomization. Circulation 2017;135:741-54. [Crossref] [PubMed]
- Tsapas A, Karagiannis T, Kakotrichi P, et al. Comparative efficacy of glucose-lowering medications on body weight and blood pressure in patients with type 2 diabetes: A systematic review and network meta-analysis. Diabetes Obes Metab 2021;23:2116-24. [Crossref] [PubMed]
- Chan YH, Chen SW, Chao TF, et al. The impact of weight loss related to risk of new-onset atrial fibrillation in patients with type 2 diabetes mellitus treated with sodium-glucose cotransporter 2 inhibitor. Cardiovasc Diabetol 2021;20:93. [Crossref] [PubMed]
- Okunrintemi V, Mishriky BM, Powell JR, et al. Sodium-glucose co-transporter-2 inhibitors and atrial fibrillation in the cardiovascular and renal outcome trials. Diabetes Obes Metab 2021;23:276-80. [Crossref] [PubMed]
- Wilding JPH. Medication use for the treatment of diabetes in obese individuals. Diabetologia 2018;61:265-72. [Crossref] [PubMed]
- Sato T, Aizawa Y, Yuasa S, et al. The effect of dapagliflozin treatment on epicardial adipose tissue volume. Cardiovasc Diabetol 2018;17:6. [Crossref] [PubMed]
- Verma S, Mazer CD, Yan AT, et al. Effect of Empagliflozin on Left Ventricular Mass in Patients With Type 2 Diabetes Mellitus and Coronary Artery Disease: The EMPA-HEART CardioLink-6 Randomized Clinical Trial. Circulation 2019;140:1693-702. [Crossref] [PubMed]
- Yurista SR, Silljé HHW, Rienstra M, et al. Sodium-glucose co-transporter 2 inhibition as a mitochondrial therapy for atrial fibrillation in patients with diabetes? Cardiovasc Diabetol 2020;19:5. [Crossref] [PubMed]
- Iacobellis G, Baroni MG. Cardiovascular risk reduction throughout GLP-1 receptor agonist and SGLT2 inhibitor modulation of epicardial fat. J Endocrinol Invest 2022;45:489-95. [Crossref] [PubMed]
- Bailey CJ. Uric acid and the cardio-renal effects of SGLT2 inhibitors. Diabetes Obes Metab 2019;21:1291-8. [Crossref] [PubMed]
- Zhao Y, Xu L, Tian D, et al. Effects of sodium-glucose co-transporter 2 (SGLT2) inhibitors on serum uric acid level: A meta-analysis of randomized controlled trials. Diabetes Obes Metab 2018;20:458-62. [Crossref] [PubMed]
- Gilbert RE, Mende C, Vijapurkar U, et al. Effects of Canagliflozin on Serum Magnesium in Patients With Type 2 Diabetes Mellitus: A Post Hoc Analysis of Randomized Controlled Trials. Diabetes Ther 2017;8:451-8. [Crossref] [PubMed]
- Leng W, Wu M, Pan H, et al. The SGLT2 inhibitor dapagliflozin attenuates the activity of ROS-NLRP3 inflammasome axis in steatohepatitis with diabetes mellitus. Ann Transl Med 2019;7:429. [Crossref] [PubMed]
- Li C, Yu J, Hockham C, et al. Canagliflozin and atrial fibrillation in type 2 diabetes mellitus: A secondary analysis from the CANVAS Program and CREDENCE trial and meta-analysis. Diabetes Obes Metab 2022;24:1927-38. [Crossref] [PubMed]
- Nishinarita R, Niwano S, Niwano H, et al. Canagliflozin Suppresses Atrial Remodeling in a Canine Atrial Fibrillation Model. J Am Heart Assoc 2021;10:e017483. [Crossref] [PubMed]
- Ohkura T. Ipragliflozin: A novel sodium-glucose cotransporter 2 inhibitor developed in Japan. World J Diabetes 2015;6:136-44. [Crossref] [PubMed]
- Kitazawa M, Katagiri T, Suzuki H, et al. A 52-week randomized controlled trial of ipragliflozin or sitagliptin in type 2 diabetes combined with metformin: The N-ISM study. Diabetes Obes Metab 2021;23:811-21. [Crossref] [PubMed]
- Chan HW, Smith NJ, Hannan RD, et al. Tackling the EGFR in pathological tissue remodelling. Pulm Pharmacol Ther 2006;19:74-8. [Crossref] [PubMed]
- Grimminger F, Schermuly RT, Ghofrani HA. Targeting non-malignant disorders with tyrosine kinase inhibitors. Nat Rev Drug Discov 2010;9:956-70. [Crossref] [PubMed]
- Li W, Fang Q, Zhong P, et al. EGFR Inhibition Blocks Palmitic Acid-induced inflammation in cardiomyocytes and Prevents Hyperlipidemia-induced Cardiac Injury in Mice. Sci Rep 2016;6:24580. [Crossref] [PubMed]
- Han W, Fu S, Wei N, et al. Nitric oxide overproduction derived from inducible nitric oxide synthase increases cardiomyocyte apoptosis in human atrial fibrillation. Int J Cardiol 2008;130:165-73. [Crossref] [PubMed]
- Zhao J, Li J, Li W, et al. Effects of spironolactone on atrial structural remodelling in a canine model of atrial fibrillation produced by prolonged atrial pacing. Br J Pharmacol 2010;159:1584-94. [Crossref] [PubMed]
- Trappe K, Thomas D, Bikou O, et al. Suppression of persistent atrial fibrillation by genetic knockdown of caspase 3: a pre-clinical pilot study. Eur Heart J 2013;34:147-57. [Crossref] [PubMed]
- Anné W, Willems R, Roskams T, et al. Matrix metalloproteinases and atrial remodeling in patients with mitral valve disease and atrial fibrillation. Cardiovasc Res 2005;67:655-66. [Crossref] [PubMed]
- Liu Y, Xu B, Wu N, et al. Association of MMPs and TIMPs With the Occurrence of Atrial Fibrillation: A Systematic Review and Meta-analysis. Can J Cardiol 2016;32:803-13. [Crossref] [PubMed]
- Vidal-Puig A, Jimenez-Liñan M, Lowell BB, et al. Regulation of PPAR gamma gene expression by nutrition and obesity in rodents. J Clin Invest 1996;97:2553-61. [Crossref] [PubMed]
- Imam MU, Ismail M, Ithnin H, et al. Effects of germinated brown rice and its bioactive compounds on the expression of the peroxisome proliferator-activated receptor gamma gene. Nutrients 2013;5:468-77. [Crossref] [PubMed]
- Tomino Y, Hagiwara S, Gohda T. AGE-RAGE interaction and oxidative stress in obesity-related renal dysfunction. Kidney Int 2011;80:133-5. [Crossref] [PubMed]
- Chiavaroli V, D'Adamo E, Giannini C, et al. Serum levels of receptors for advanced glycation end products in normal-weight and obese children born small and large for gestational age. Diabetes Care 2012;35:1361-3. [Crossref] [PubMed]
- Miranda ER, Fuller KNZ, Perkins RK, et al. Endogenous secretory RAGE increases with improvements in body composition and is associated with markers of adipocyte health. Nutr Metab Cardiovasc Dis 2018;28:1155-65. [Crossref] [PubMed]
- Yamagishi SI, Matsui T. Therapeutic Potential of DNA-aptamers Raised Against AGE-RAGE Axis in Diabetes-related Complications. Curr Pharm Des 2018;24:2802-9. [Crossref] [PubMed]
- Senatus LM, Schmidt AM. The AGE-RAGE Axis: Implications for Age-Associated Arterial Diseases. Front Genet 2017;8:187. [Crossref] [PubMed]
- Ferrucci L, Fabbri E. Inflammageing: chronic inflammation in ageing, cardiovascular disease, and frailty. Nat Rev Cardiol 2018;15:505-22. [Crossref] [PubMed]
- Begieneman MP, Rijvers L, Kubat B, et al. Atrial fibrillation coincides with the advanced glycation end product N(ε)-(carboxymethyl)lysine in the atrium. Am J Pathol 2015;185:2096-104. [Crossref] [PubMed]
- Ando R, Ueda S, Yamagishi S, et al. Involvement of advanced glycation end product-induced asymmetric dimethylarginine generation in endothelial dysfunction. Diab Vasc Dis Res 2013;10:436-41. [Crossref] [PubMed]
- Kay AM, Simpson CL, Stewart JA Jr. The Role of AGE/RAGE Signaling in Diabetes-Mediated Vascular Calcification. J Diabetes Res 2016;2016:6809703. [Crossref] [PubMed]
- Yang X, An N, Zhong C, et al. Enhanced cardiomyocyte reactive oxygen species signaling promotes ibrutinib-induced atrial fibrillation. Redox Biol 2020;30:101432. [Crossref] [PubMed]
- Daffu G, del Pozo CH, O'Shea KM, et al. Radical roles for RAGE in the pathogenesis of oxidative stress in cardiovascular diseases and beyond. Int J Mol Sci 2013;14:19891-910. [Crossref] [PubMed]
- Meza CA, La Favor JD, Kim DH, et al. Endothelial Dysfunction: Is There a Hyperglycemia-Induced Imbalance of NOX and NOS? Int J Mol Sci 2019;20:3775. [Crossref] [PubMed]
- Steinhorn B, Sartoretto JL, Sorrentino A, et al. Insulin-dependent metabolic and inotropic responses in the heart are modulated by hydrogen peroxide from NADPH-oxidase isoforms NOX2 and NOX4. Free Radic Biol Med 2017;113:16-25. [Crossref] [PubMed]
- McCauley MD, Hong L, Sridhar A, et al. Ion Channel and Structural Remodeling in Obesity-Mediated Atrial Fibrillation. Circ Arrhythm Electrophysiol 2020;13:e008296. [Crossref] [PubMed]
- Joseph LC, Avula UMR, Wan EY, et al. Dietary Saturated Fat Promotes Arrhythmia by Activating NOX2 (NADPH Oxidase 2). Circ Arrhythm Electrophysiol 2019;12:e007573. [Crossref] [PubMed]
- Li L, Hua C, Liu X, et al. SGLT2i alleviates epicardial adipose tissue inflammation by modulating ketone body-glyceraldehyde-3-phosphate dehydrogenase malonylation pathway. J Cardiovasc Med (Hagerstown) 2023;24:232-43. [Crossref] [PubMed]