Compositional alterations of the gut microbiota in acute myocardial infarction patients with type 2 diabetes mellitus
Highlight box
Key findings
• The composition and predictive function of the gut microbiota differed between patients with acute myocardial infarction (AMI) with and without type 2 diabetes mellitus (T2DM).
What is known and what is new?
• The prognosis of patients with AMI and T2DM (AMIDM) is worse than that of those with AMI but without T2DM (AMINDM). To date, very few studies have examined the possible mechanisms underlying this interaction, especially from the gut microbiota perspective.
• Changes in the composition of the gut microbiota in patients with AMIDM may be critical in influencing the severity of metabolic disorders and may account for the poorer clinical outcomes and disease progression of patients with AMIDM compared to patients with AMINDM.
What is the implication, and what should change now?
• Commensal bacteria involved in nucleotide metabolism may contribute to the progression of AMIDM and may serve as a biomarker for predicting disease prognosis.
Introduction
Previous studies have shown that multiple risk factors, such as type 2 diabetes mellitus (T2DM), hypercholesterolemia, hypertension, and age, contribute to the progression of acute myocardial infarction (AMI) (1). Compared to patients with AMI but without T2DM (AMINDM), the clinical profile of patients with AMI and T2DM (AMIDM) is more complex and the treatment more difficult (2). Notably, patients with T2DM have an earlier onset of AMI, poorer heart function, and broad coronary lesions, and worse outcomes than those without T2DM (3). However, few studies have examined the mechanisms underlying this relationship, especially from the gut microbiota perspective.
The incidence of AMI is increasing worldwide; however, in recent years, very little research has been conducted on the relationship between gut microbiota and AMI (4,5). There is also a strong relationship between T2DM and AMI. However, the pathogenesis of T2DM is not yet fully understood. In addition to insulin resistance, recent studies have shown that the “second genome” of the human body (i.e., the gut microbiota) plays a critical role in the development of metabolic diseases, such as obesity and diabetes, as the gut microbiota is involved in the processes of digestion and absorption (6), glucose and lipid metabolism (7), and immunity (8).
Gut microbiota plays a crucial role in the progression of AMIDM. Thus, it was hypothesized that the gut microbiota composition and predicted function of patients with AMIDM would differ to that of patients with AMINDM and might be associated with a poor prognosis in patients with AMIDM. In this study, we investigated the gut microbiota profiles of patients with AMIDM. We present this article in accordance with the MDAR reporting checklist (available at https://atm.amegroups.com/article/view/10.21037/atm-22-3521/rc).
Methods
Study participants
A total of 30 AMI patients admitted to the Shenzhen Hospital of Huazhong University of Science and Technology were enrolled in this study from January 2022 to May 2022. The diagnosis of AMI was made with reference to the World Health Organization’s definition and the third universal definition of myocardial infarction (9). This definition includes patients with ischemic symptoms, cardiac laboratory biomarkers, electrocardiographic findings, and invasive coronary angiography or coronary computed tomography angiography. Patients were included in this study if they met the diagnostic criteria for AMI and if their data included a staging diagnosis of both ST-segment elevation and non-ST-segment elevation AMI. Patients were excluded from the study if they met any of the following exclusion criteria: (I) had thyroid dysfunction, including symptoms of hypothyroidism, hyperthyroidism, or were using thyroid hormones or antithyroid drugs; (II) had multiple aortitis; (III) were pregnant or lactating; (IV) had abnormal liver function (>5.0 times the upper limit of normal); (V) had severe chronic obstructive pulmonary disease or respiratory failure; (VI) had a history of gastrointestinal, respiratory, or other organ bleeding; (VII) had a severe infection; (VIII) had a severe frailty, such as a malignancy; (IX) had a neuropsychiatric disorder; (X) had used oral antibiotics or probiotic agents in the last 3 months; and/or (XI) had a previous organic or functional digestive disorder. The study was conducted in accordance with the Declaration of Helsinki (as revised in 2013). This study was approved by the Ethics Committee of the Shenzhen Hospital of Huazhong University of Science and Technology (No. KY-2021-042) and was registered in the China Clinical Trials Registry Center (No. ChiCTR2100054025). Informed consent was obtained from all individual participants.
Sample collection and DNA extraction
Stool samples were collected in fresh stool collecting tubes and stored in a refrigerator at –80 ℃ Fahrenheit awaiting testing. The bacterial DNA was extracted from the fecal samples. The associated clinical data were collected simultaneously.
16S rDNA gene V4 region sequencing
High-throughput sequencing was used for the gene sequencing of the V4 fragment in the 16S ribosomal DNA (rDNA) gene. The target amplicon was recovered, and the interrupted sticky ends were repaired into flat ends using T4 DNA polymerase, Klenow DNA polymerase, and T4 polynucleotide kinase (T4 PNK), after which base adenine (A) was added at the 3' end. This process allowed the DNA fragment to be ligated to the 3' end with the base thymine. A double-indexed sequence fusion primer containing the sequencing junction was then designed and used as a template for the polymerase chain reaction using genomic DNA. The target amplicon was screened using magnetic beads, and a qualified library was used for the DNA cluster preparation and sequencing.
Statistical analyses
SPSS 25.0 (IBM Corp, Armonk, NY, USA) was used to compare and analyze the general clinical data. The measurement data are expressed as the mean ± standard error of the mean and were analyzed using a t-test. The quantitative data are expressed as the rate (%) and were analyzed using the chi-square test. A P value <0.05 indicated a statistically significant difference. QIIME2 software (Version 1.7.0) (https://qiime2.org/) was used to analyze the gut microbiota α diversity, and the α diversity indicators included the Chao1 index, Ace index, Shannon index, and Simpson index. β diversity was analyzed by presenting the species diversity matrix based on the binary Jaccard algorithm and plotting the sample partial least squares discriminant analysis (PLS-DA) results based on data from the R language platform (Bell Laboratories, Inc., Murray Hill, New York, USA). Metastats software (http://metastats.cbcb.umd.edu/) was used to compare the relative abundance of bacteria at different levels between the 2 groups. A t-test of the species abundance data between the 2 groups was used to obtain the P value, and the P value was corrected to obtain the Q value. Finally, the species responsible for the difference in the sample composition between the 2 groups were screened according to their P (or Q) values, and a default P value of ≥0.05 was set as the criteria. Linear discriminant analysis effect size (LEfSe) was used to compare the relative abundance of the flora at the species and genus levels between the 2 groups, and a logarithmic linear discriminant analysis (LDA) score of 2.0 indicated a significant difference. LEfSe was used to compare the microbiota abundance at the species and genus levels between the 2 groups, and a logarithmic LDA score of 2.0 indicated a significant difference. LDA was used to estimate the magnitude of the effect of the abundance of each component species on the differential effect to identify the species that differed significantly in terms of abundance between the groups. Pearson correlation coefficients were used to analyze the correlations between patients’ clinical data and the most abundant 20 species at the species level.
Results
Baseline characteristics
Table 1 summarizes the general characteristics of the 2 groups. Among the patients with AMIDM, 5 had ST-segment elevation myocardial infarction (STEMI) and 10 had non-ST-segment elevation myocardial infarction (NSTEMI), and 7 had left anterior descending (LAD) coronary artery stenosis identified as an infarct-related artery (IRA), and 12 had ≥2 coronary artery stenoses. Among the patients with AMINDM, 6 had STEMI and 9 had NSTEMI, and 9 had LAD coronary artery stenosis identified as IRA, and 8 had ≥2 coronary artery stenoses. There were no significant differences between the patients with AMIDM and those with AMINDM in terms of sex, age, body mass index, smoking history, and alcohol consumption (P>0.05). Patients with AMIDM had increased serum fasting glucose, hemoglobin A1c (HbAlc), and triglycerides (P<0.05) and decreased left ventricular ejection fraction (P=0.059), but no significant differences in the other clinical parameters were observed (P>0.05; Table 1).
Table 1
Demographics | AMINDM (n=15) | AMIDM (n=15) | P value |
---|---|---|---|
Sex, males | 11 (73.3) | 13 (86.7) | 0.651 |
Age (years) | 57.1±1.9 | 54.9±2.2 | 0.447 |
BMI (kg/m2) | 24.9±0.5 | 26.7±0.9 | 0.083 |
STEMI | 6 (40.0) | 5 (33.3) | 1.000 |
IRA (LAD) | 9 (60.0) | 7 (46.7) | 0.715 |
≥2 coronary stenoses | 8 (53.3) | 12 (80.0) | 0.245 |
NYHA class (I/II/III/IV) | 10/3/0/2 | 11/2/1/1 | 0.664 |
LVEF (%) | 58.1±2.5 | 50.3±3.1 | 0.059 |
SBP (mmHg) | 128.7±4.5 | 134.3±5.6 | 0.444 |
DBP (mmHg) | 81.1±2.5 | 82.6±2.1 | 0.643 |
Hypertension | 4 (26.7) | 8 (53.3) | 0.264 |
Smoking | 6 (40.0) | 4 (26.7) | 0.700 |
Alcohol consumption | 1 (6.7) | 3 (20.0) | 0.598 |
TnI (ng/mL) | 53.4±29.1 | 34.6±13.5 | 0.561 |
NT-proBNP (pg/mL) | 1351.3±495.1 | 1041.9±325.6 | 0.601 |
GLU (mmol/L) | 6.6±0.4 | 10.3±1.4 | 0.022* |
HbAlc (%) | 5.8±0.2 | 8.2±0.5 | <0.001* |
CHOL (mmol/L) | 4.0±0.2 | 4.4±0.2 | 0.174 |
TRIG (mmol/L) | 1.4±0.2 | 2.6±0.5 | 0.043* |
HDL-C (mmol/L) | 0.9±0.1 | 0.8±0.0 | 0.148 |
LDL-C (mmol/L) | 2.5±0.2 | 2.7±0.3 | 0.557 |
BUN (mg/dL) | 6.1±0.7 | 6.0±0.5 | 0.794 |
Cre (mg/dL) | 79.8±4.1 | 83.0±6.0 | 0.654 |
UA (μmol/L) | 350.0±22.0 | 391.0±21.5 | 0.192 |
Data are presented as n (%) or mean ± SEM. The differences between the patients with AMIDM and those with AMINDM were compared using Fisher’s exact test for sex, age, STEMI, LVEF, NYHA class, hypertension, smoking, and alcohol consumption, and a t-test was used for the other variables. *, statistical significance (P<0.05). AMIDM, acute myocardial infarction with type 2 diabetes mellitus; AMINDM, acute myocardial infarction without type 2 diabetes mellitus; BMI, body mass index; BUN, blood urea nitrogen; CHOL, cholesterol; Cre, creatinine; DBP, diastolic blood pressure; GLU, glucose; HbA1c, hemoglobin A1c; HDL-C, high-density lipoprotein cholesterol; IRA, infarction related artery; LAD, left anterior descending coronary; LDL-C, low-density lipoprotein cholesterol; LVEF, left ventricular ejection fraction; NT-proBNP, N-terminal pro B-type natriuretic peptide; NYHA, New York Heart Association; SBP, systolic blood pressure; SEM, standard error of the mean; STEMI, ST-segment elevation myocardial infarction; TnI, troponin I; TRIG, triglycerides; UA, uric acid.
Diversity of the gut microbiota
Gut microbiota with ɑ diversity was evaluated using the Ace index, Shannon index, Simpson index, and Chao1 index. PLS-DA was used to assess microbiota β diversity. No statistical differences were observed in the Ace index, Shannon index, Simpson index, and Chao1 index between the 2 groups (Figure 1A-1D). The PLS-DA results revealed a different pattern in the gut microbiota composition of the patients with AMIDM compared to those with AMINDM (Figure 1E).
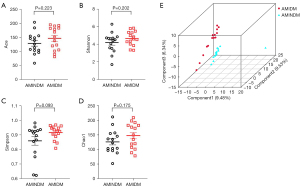
The microbiota at the phylum level
We compared the overall distribution of the 15 most abundant phyla, and found that among all the identified operational taxonomic units (OTUs), Firmicutes, Bacteroidetes, Proteobacteria, and Actinobacteria were the 4 most abundant phyla in the patients with AMIDM and AMINDM overall (Figure 2A,2B). The most abundant phylum in the patients with AMIDM was Firmicutes, which accounted for 74.0% in the AMIDM patients and 52.2% the AMINDM patients. The second most abundant phylum in the patients with AMIDM was Bacteroidetes, which accounted for 17.9% in the AMIDM patients and 26.2% in the patients with AMIDM (Figure 2B). A significant increasing trend was observed in the Firmicutes to Bacteroidetes ratio in the patients with AMIDM (Figure 2C). In summary, at the phylum level, the abundance of Actinobacteria (2.12% vs. 1.05%) was higher in the patients with AMIDM than patients with AMINDM. Compared to patients with AMINDM, patients with AMIDM had lower abundances of Proteobacteria (4.78% vs. 15.90%), Verrucomicrobiota (0.77% vs. 1.62%), Desulfobacterota (0.32% vs. 1.12%), Campylobacterota (0.08% vs. 0.12%), Acidobacteriota (0.00% vs. 0.13%), and Myxococcota (0.00% vs. 0.12%) (Figure 2B).
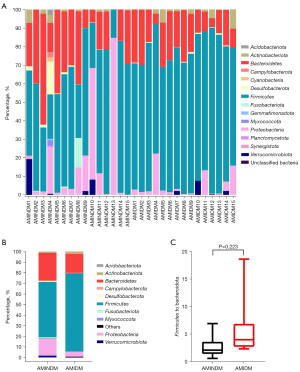
The characteristics of bacteria in the patients with AMIDM
A random-forest model was used to characterize the specific bacteria at the genus level. Figure 3A shows the 30 most abundant bacteria in the patients with AMIDM and patients with AMINDM at the genus level. Among them, we found that the relative richness of UCG-005, Coprococcus, and Barnesiella was higher in the patients with AMIDM, than those with AMINDM, and the relative richness of Fusobacterium was lower in the patients with AMIDM than those with AMINDM (Figure 3A,3B). Further, we found the relative richness of genus Companilactobacillus and genus Defluvitaleaceae UCG-011 and UCG-009 was more increased in the patients with AMIDM than the patients with AMINDM, while the relative richness of genus Phascolarctobacterium and CAG 56 was lower in the patients with AMIDM than patients with AMINDM (Figure 3B).
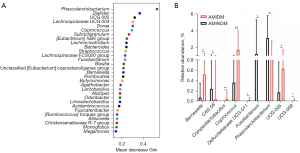
To examine the gut microbial community of the patients with AMIDM, a LEfSe analysis with a cut-off value of 2.0 was conducted to compared the microbial composition of the patients with AMIDM to that of patients with AMINDM at the species level (Figure 4A,4B), and a significant difference was found in the gut microbiota compositions between the patients with AMIDM and those with AMINDM at the species level. The relative abundance of the unclassified NK4A214 group, Bacteroides clarus, Coprococcus comes, unclassified Defluviltaleaceae UCG-011, uncultured rumen bacterium, unclassified CAG 56, Barnesiella intestinihominis, Lachnospiraceae bacterium, Bacteroides nordii, unclassified UCG-009, and the Family XIII AD 3011 group in the patients with AMIDM was higher than that in the patients with AMINDM. The species Phascolarctobacterium faecium, Fusobacterium varium, and Tyzzerella nexilis were more abundant in the patients with AMINDM than those with AMIDM (Figure 4B).
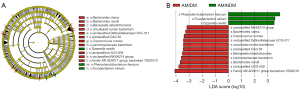
Predictive functional differences in gut microbiota
The PICRUst (http://picrust.github.io/picrust/) was used to predict the functional gene composition of the samples by comparing the species composition information obtained from the 16S sequencing data. Data from the Kyoto Encyclopedia of Genes and Genomes database indicated that nucleotide metabolism functioning was significantly increased in the patients with AMIDM compared to those with AMINDM (Figure 5A,5B). Bugbase (https://bugbase.cs.umn.edu/) is an algorithm that predicts the bio-level coverage of functional pathways within a complex microbiome and bio-explanatory phenotypes, such as oxygen tolerance, gram staining, and pathogenic potential. The Bugbase analysis indicated that the gram-positive bacterial mean proportion was significantly increased in the patients with AMIDM compared to those with AMINDM. Conversely, the gram-negative bacterial mean proportion was significantly decreased in the patients with AMIDM compared to those with AMINDM (Figure 5C).
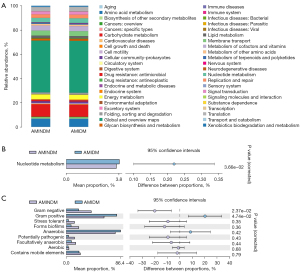
Correlation analysis of the clinical indexes and microbiota at the species level
A Pearson correlation analysis was performed to examine the relationship between the bacterial abundance at the species level and the clinical data of the patients. We found that Faecalibacterium prausnitzii, Dorea longicatena, and Ruminococcus species (sp.) were positively correlated with HbA1c, while Phascolarctobacterium faecium was negatively correlated with HbA1c. Dialister massiliensis was positively correlated with blood urea nitrogen (BUN). Parabacteroides distasonis, Ruminococcaceae bacterium LM158, Trichuris trichiura human whipworm, Trichuris trichiura human whipworm, Akkermansia muciniphila, Alistipes putredinis, and Ruminococcus sp. were positively correlated with serum creatinine (Cre). Escherichia coli was positively correlated with serum uric acid (UA), while Faecalibacterium sp. was negatively correlated with UA. Parabacteroides distasonis, Phascolarctobacterium faecium, Phocaeicola vulgatus, Bifidobacterium pseudocatenulatum, Megamonas funiformis, and Dialister massiliensis were positively correlated with high-density lipoprotein cholesterol (HDL-C), while Phocaeicola dorei was negatively correlated with HDL-C. Dialister succinatiphilus and Alistipes finegoldii were positively correlated with cholesterol (CHOL) (Figure 6).
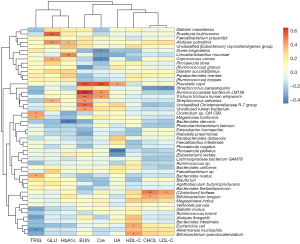
Discussion
In this study, we used 16S rDNA sequencing to examine the different compositions and functions of the gut microbiota from 15 patients with AMIDM and 15 patients with AMINDM. Our results revealed the different gut microbiota compositions of the 2 groups and predicted the functions between the 2 groups. We also found significant correlations between some bacteria at the species level and the clinical parameters of the AMI patients. This study is the first to provide evidence of the gut microbiota characteristics of patients with AMIDM.
The shaping and colonization of the gut microbiota begin at birth, and changes in its composition depends on various factors, including hereditary, nutritional, and environmental factors (10). All the participants in present study lived in the same region and shared a similar lifestyle. it has been well shown that dietary habits, medications, and genetics can induce dysbiosis of the gut microbiota and thus contribute to T2DM (11,12). Thus, we conjecture that the differences in the gut microbiota compositions between the 2 groups are derived from dietary habits and genetic factors.
The different gut microbiota compositions between the 2 groups were confirmed by the β diversity analysis. At the phylum level, Firmicutes and Bacteroidetes were the 2 most dominant phyla. Research has shown that when altered, Firmicutes and Bacteroidetes are strongly associated with the progression of cardiovascular and metabolic diseases (13,14). Our results also showed a higher relative abundance of Firmicutes and a lower relative abundance of Bacteroidetes in the patients with AMIDM accompanied by a higher Firmicutes/Bacteroidetes ratio. An elevated Firmicutes/Bacteroidetes ratio has been identified as a possible biomarker of gut dysbiosis and various diseases, such as T2DM, obesity, and coronary artery atherosclerotic heart disease (15-17). most bacteria belonging to the phylum Firmicutes feature a gram-positive cell-wall structure, and are associated with unhealthy lifestyles, such as a high-fat diet (18), abnormal energy balance (19), and being overweight (20). Bacteroidetes consist of gram-negative bacteria whose richness increases with fiber intake and a high carbohydrate diet, and decreases with the progression of atherosclerosis (21-23). Various studies have been conducted on the specific microbial profiles of coronary artery disease, and these studies have observed that the most prevalent result in the patients with coronary artery disease is an increase in the Firmicutes/Bacteroidetes ratio (15,24,25). Patients with AMIDM had a higher Firmicutes/Bacteroidetes ratio than those with AMINDM, which might be because the systemic chronic inflammation and insulin resistance caused by diabetes alters the intestinal environment and worsens gut microbiota dysbiosis.
A random-forest model was used to characterize specific bacteria at the genus level. Compared to the patients with AMINDM, those with AMIDM had a higher relative abundance of Coprococcus and Barnesiella, and a lower relative abundance of Fusobacterium. Coprococcus is a strictly anaerobic gram-positive coccus that belongs to the Firmicutes phylum, is involved in the fermentation of carbohydrates, and can produce butyric acid (26). Thus, we speculate that the poor prognosis of patients with AMIDM might be associated with a high abundance of Coprococcus. The genus Bartonella consists of a small group of 2 bacteria that usually only exist at moderately low levels in the intestinal tract and comprise <1% of an individual’s gut microbiota (27). Bartonella has also been linked to C. difficile infection (28). A previous study also reported an association between Bartonella and the initiation of type 1 diabetes (29). Moreover, Bartonella has been shown to be positively associated with plasma CHOL concentrations and may mediate the regulation of CHOL absorption, biosynthesis, and circulating CHOL levels in the host’s body, leading to low hepatic CHOL synthesis and a high intestinal absorption pattern, and thus Bartonella may be a biomarker for coronary artery disease (30).
Further, we used LEfSe to conduct a differential taxonomic expression analysis to examine differences at the species level, and found that several commensal species, such as Phascolarctobacterium faecium, Fusobacterium varium, and Tyzzerella nexilis, were depleted in the gut microbiota of patients with AMIDM. These symbiotic species can maintain cardiovascular status through various methods, such as the metabolic production of short-chain fatty acids (SCFAs) (31). Phascolarctobacterium faecium can produce SCFAs and is associated with the host’s metabolic state (32). In recent years, the gut microbiota and its metabolites have been found to be involved in the development of cardiovascular diseases, including atherosclerosis, heart failure, and hypertension (31). By modifying the composition of the gut microbiota and its metabolites, the process of cardiovascular diseases can be improved. This therapeutic approach has emerged as a new direction in the treatment of cardiovascular disease (33). There is increasing evidence that the end-products of SCFAs (including acetate, propionate, and butyrate) interact with cardiovascular diseases by maintaining intestinal integrity, anti-inflammation, modulating glucolipid metabolism and blood pressure, and activating gut-brain axis (31,34). SCFAs exert beneficial cardiovascular effects via free fatty acid receptor 3 (35,36). In addition, a ketogenic diet can improve cardiac energy expenditure and reduce oxygen use to improve cardiovascular diseases, such as high blood pressure (37). This might be because β-hydroxybutyric acid exerts a protective effect on the endothelium and blood vessels (37-39).
Decreased Phascolarctobacterium faecium is presumed to be associated with disturbances in lipid metabolism (40). Unlike many intestinal microorganisms, the butyrate-producing anaerobic bacterial Fusobacterium varium ferments amino acids and glucose, and it has been implicated in both beneficial and pathological microbial-host interactions (41,42). Some commensal species, such as Bacteroides clarus, Bacteroides nordii, Coprococcus comes, Barnesiella intestinihominis, and Lachnospiraceae bacterium, were enriched in the gut microbiota of the patients with AMIDM. Among these, the species Bacteroides clarus and Bacteroides nordii appear to be associated with increased blood inflammatory cytokines (43,44). Barnesiella intestinihominis might contribute to the glucose tolerance associated with gut-specific glucose transporter type 1 (glut1) inactivation (45). Together, these findings suggest that gut microbiota alterations are associated with a poor prognosis in patients with AMIDM. An increased drug resistance, lipid metabolism disturbance, and worse glucose tolerance might mediate these associations. Overall, these results provide new insights into possible microbiome profiles for the future treatment of patients with AMIDM. However, additional studies with larger cohorts of patients need to be conducted to confirm our results.
The PICRUst was used to conduct the functional analysis. We found that nucleotide metabolism functioning was significantly increased in the patients with AMIDM. An increase in the nucleotide metabolism of gut microbiota results in a decrease in nucleotide absorption and an increase in some metabolites, such as UA (46). Emerging evidence suggests that intracellular nucleotides are important substances that serve as intracellular-energy and extracellular-signaling molecules in the purinergic signaling cascade (47). Notably, adenosine, which is an important substance comprising nucleotides that protect the myocardium against ischemic and reperfusion injury, slows atrial and atrioventricular conduction, resulting in a decreased heart rate, coronary vasodilatation, and diminished β-adrenergic receptor stimulation (48,49). Thus, adenosine helps to rebalance the myocardial oxygen supply and demand (47). This functional pathway plays an essential role in reperfusion injury in AMI and the postinfarction repair process. Thus, the symbiotic bacteria involved in nucleotide metabolism may contribute to the progression of AMIDM and may serve as a biomarker for predicting disease prognosis.
We also examined the relationship between the clinical parameters and gut microbiota of the 30 AMI patients and found that at the species level, some commensal bacteria were positively correlated with the clinical parameters, while others were negatively correlated with the clinical parameters. Notably, we found that HbA1c was a potential predictor of clinical outcomes following AMI, Dorea longicatena was positively correlated with HbA1c, and Phascolarctobacterium faecium was negatively correlated with HbA1c. These findings are similar to those obtained in diabetes mellitus (DM) patients (50,51). Thus, we inferred that the Phascolarctobacterium faecium species was a favorable factor and the Dorea longicatena was a detrimental factor in AMIDM. A previous study examined the incidence and prognostic significance of metabolite disturbances among AMI patients with and without T2DM (52). The higher prevalence of metabolic disorders among patients with AMIDM could explain, at least partially, why patients with AMIDM have worse outcomes compared to those with AMINDM. Overall, these key findings from the current study suggest that the commensal bacteria associated with glucose and lipid metabolism are potential biomarkers of poor outcomes in patients with AMIDM.
The present study had a number of limitations. First, it was an observational study, and its sample size was relatively small. Second, only experimental participants from China were recruited, and no research was conducted with people of other races or ethnicities. However, this was the first study to present the gut microbiota profiles of patients with AMIDM. Third, these samples were only collected at one time point, which prevented us from assessing whether there were any volatilities in gut microbiota composition. However, the use of the 16S rDNA sequencing method improved the quality of the study, enabling discussion at a species level. In the future, integrated multiomics analyses need to be conducted to explore the role of the gut microbiota in the pathophysiological processes of AMIDM. Studies of animal models also need to be conducted to explore the specific molecular biological mechanisms of the gut microbiota involved in the poor outcomes of patients with AMIDM.
Conclusions
In the context of AMI, DM represents an important negative prognostic marker and a therapeutic challenge. Considerable efforts have been made to identify prognostic markers and targets for interventions to improve the prognosis of these patients. The present study identified significant differences in the composition and predictive function of the gut microbiota between the patients with AMIDM and those with AMINDM. Notably, the involvement of commensal bacteria in nucleotide metabolism may contribute to the progression of AMIDM and may serve as a biomarker for predicting disease prognosis. Some commensal bacteria at the species level were closely correlated with the clinical parameters of the AMI patients. Overall, our results showed that the composition and function of the gut microbiota differed significantly between the patients with AMIDM and those with AMINDM. Gut microbiota could be a promising diagnostic biomarker or a therapeutic target.
Acknowledgments
We would like to thank Xiaojia Huang and the staff of the Department of Scientific Research for improving the clinical trial design protocol of this study. We would also like to thank all the participants who participated in this study.
Funding: This work was funded by the National Natural Science Foundation of China (No. 81670480).
Footnote
Data Sharing Statement: Available at https://atm.amegroups.com/article/view/10.21037/atm-22-3521/dss
Reporting Checklist: The authors have completed the MDAR reporting checklist. Available at https://atm.amegroups.com/article/view/10.21037/atm-22-3521/rc
Conflicts of Interest: All authors have completed the ICMJE uniform disclosure form (available at https://atm.amegroups.com/article/view/10.21037/atm-22-3521/coif). The authors have no conflicts of interest to declare.
Ethical Statement: The authors are accountable for all aspects of the work in ensuring that questions related to the accuracy or integrity of any part of the work are appropriately investigated and resolved. The study was conducted in accordance with the Declaration of Helsinki (as revised in 2013).
Open Access Statement: This is an Open Access article distributed in accordance with the Creative Commons Attribution-NonCommercial-NoDerivs 4.0 International License (CC BY-NC-ND 4.0), which permits the non-commercial replication and distribution of the article with the strict proviso that no changes or edits are made and the original work is properly cited (including links to both the formal publication through the relevant DOI and the license). See: https://creativecommons.org/licenses/by-nc-nd/4.0/.
References
- Anderson JL, Morrow DA. Acute Myocardial Infarction. N Engl J Med 2017;376:2053-64. [Crossref] [PubMed]
- Jacoby RM, Nesto RW. Acute myocardial infarction in the diabetic patient: pathophysiology, clinical course and prognosis. J Am Coll Cardiol 1992;20:736-44. [Crossref] [PubMed]
- Milazzo V, Cosentino N, Genovese S, et al. Diabetes Mellitus and Acute Myocardial Infarction: Impact on Short and Long-Term Mortality. Adv Exp Med Biol 2021;1307:153-69. [Crossref] [PubMed]
- Han Y, Gong Z, Sun G, et al. Dysbiosis of Gut Microbiota in Patients With Acute Myocardial Infarction. Front Microbiol 2021;12:680101. [Crossref] [PubMed]
- Wu ZX, Li SF, Chen H, et al. The changes of gut microbiota after acute myocardial infarction in rats. PLoS One 2017;12:e0180717. [Crossref] [PubMed]
- Craciun CI, Neag MA, Catinean A, et al. The Relationships between Gut Microbiota and Diabetes Mellitus, and Treatments for Diabetes Mellitus. Biomedicines 2022;10:308. [Crossref] [PubMed]
- Yan F, Li N, Shi J, et al. Lactobacillus acidophilus alleviates type 2 diabetes by regulating hepatic glucose, lipid metabolism and gut microbiota in mice. Food Funct 2019;10:5804-15. [Crossref] [PubMed]
- Tanase DM, Gosav EM, Neculae E, et al. Role of Gut Microbiota on Onset and Progression of Microvascular Complications of Type 2 Diabetes (T2DM). Nutrients 2020;12:3719. [Crossref] [PubMed]
- Mendis S, Thygesen K, Kuulasmaa K, et al. World Health Organization definition of myocardial infarction: 2008-09 revision. Int J Epidemiol 2011;40:139-46. [Crossref] [PubMed]
- Gomaa EZ. Human gut microbiota/microbiome in health and diseases: a review. Antonie Van Leeuwenhoek 2020;113:2019-40. [Crossref] [PubMed]
- Gurung M, Li Z, You H, et al. Role of gut microbiota in type 2 diabetes pathophysiology. EBioMedicine 2020;51:102590. [Crossref] [PubMed]
- Scheithauer TPM, Rampanelli E, Nieuwdorp M, et al. Gut Microbiota as a Trigger for Metabolic Inflammation in Obesity and Type 2 Diabetes. Front Immunol 2020;11:571731. [Crossref] [PubMed]
- Da Silva CC, Monteil MA, Davis EM. Overweight and Obesity in Children Are Associated with an Abundance of Firmicutes and Reduction of Bifidobacterium in Their Gastrointestinal Microbiota. Child Obes 2020;16:204-10. [Crossref] [PubMed]
- Robles-Vera I, Toral M, de la Visitación N, et al. Probiotics Prevent Dysbiosis and the Rise in Blood Pressure in Genetic Hypertension: Role of Short-Chain Fatty Acids. Mol Nutr Food Res 2020;64:e1900616. [Crossref] [PubMed]
- Sawicka-Smiarowska E, Bondarczuk K, Bauer W, et al. Gut Microbiome in Chronic Coronary Syndrome Patients. J Clin Med 2021;10:5074. [Crossref] [PubMed]
- Huang L, Thonusin C, Chattipakorn N, et al. Impacts of gut microbiota on gestational diabetes mellitus: a comprehensive review. Eur J Nutr 2021;60:2343-60. [Crossref] [PubMed]
- Stojanov S, Berlec A, Štrukelj B. The Influence of Probiotics on the Firmicutes/Bacteroidetes Ratio in the Treatment of Obesity and Inflammatory Bowel disease. Microorganisms 2020;8:1715. [Crossref] [PubMed]
- Bisanz JE, Upadhyay V, Turnbaugh JA, et al. Meta-Analysis Reveals Reproducible Gut Microbiome Alterations in Response to a High-Fat Diet. Cell Host Microbe 2019;26:265-272.e4. [Crossref] [PubMed]
- Schwiertz A, Taras D, Schäfer K, et al. Microbiota and SCFA in lean and overweight healthy subjects. Obesity (Silver Spring) 2010;18:190-5. [Crossref] [PubMed]
- Gomes AC, Hoffmann C, Mota JF. The human gut microbiota: Metabolism and perspective in obesity. Gut Microbes 2018;9:308-25. [Crossref] [PubMed]
- Faith JJ, McNulty NP, Rey FE, et al. Predicting a human gut microbiota's response to diet in gnotobiotic mice. Science 2011;333:101-4. [Crossref] [PubMed]
- Lozupone CA, Stombaugh JI, Gordon JI, et al. Diversity, stability and resilience of the human gut microbiota. Nature 2012;489:220-30. [Crossref] [PubMed]
- Jie Z, Xia H, Zhong SL, et al. The gut microbiome in atherosclerotic cardiovascular disease. Nat Commun 2017;8:845. [Crossref] [PubMed]
- Emoto T, Yamashita T, Sasaki N, et al. Analysis of Gut Microbiota in Coronary Artery Disease Patients: a Possible Link between Gut Microbiota and Coronary Artery Disease. J Atheroscler Thromb 2016;23:908-21. [Crossref] [PubMed]
- Cui L, Zhao T, Hu H, et al. Association Study of Gut Flora in Coronary Heart Disease through High-Throughput Sequencing. Biomed Res Int 2017;2017:3796359. [Crossref] [PubMed]
- Altemani F, Barrett HL, Gomez-Arango L, et al. Pregnant women who develop preeclampsia have lower abundance of the butyrate-producer Coprococcus in their gut microbiota. Pregnancy Hypertens 2021;23:211-9. [Crossref] [PubMed]
- Ubeda C, Bucci V, Caballero S, et al. Intestinal microbiota containing Barnesiella species cures vancomycin-resistant Enterococcus faecium colonization. Infect Immun 2013;81:965-73. [Crossref] [PubMed]
- Milani C, Ticinesi A, Gerritsen J, et al. Gut microbiota composition and Clostridium difficile infection in hospitalized elderly individuals: a metagenomic study. Sci Rep 2016;6:25945. [Crossref] [PubMed]
- Marietta EV, Gomez AM, Yeoman C, et al. Low incidence of spontaneous type 1 diabetes in non-obese diabetic mice raised on gluten-free diets is associated with changes in the intestinal microbiome. PLoS One 2013;8:e78687. [Crossref] [PubMed]
- Le Roy T, Lecuyer E, Chassaing B, et al. The intestinal microbiota regulates host cholesterol homeostasis. BMC Biol 2019;17:94. [Crossref] [PubMed]
- Chen XF, Chen X, Tang X. Short-chain fatty acid, acylation and cardiovascular diseases. Clin Sci (Lond) 2020;134:657-76. [Crossref] [PubMed]
- Wu F, Guo X, Zhang J, et al. Phascolarctobacterium faecium abundant colonization in human gastrointestinal tract. Exp Ther Med 2017;14:3122-6. [Crossref] [PubMed]
- Sanchez-Gimenez R, Ahmed-Khodja W, Molina Y, et al. Gut Microbiota-Derived Metabolites and Cardiovascular Disease Risk: A Systematic Review of Prospective Cohort Studies. Nutrients 2022;14:2654. [Crossref] [PubMed]
- Hu T, Wu Q, Yao Q, et al. Short-chain fatty acid metabolism and multiple effects on cardiovascular diseases. Ageing Res Rev 2022;81:101706. [Crossref] [PubMed]
- Lymperopoulos A, Suster MS, Borges JI. Short-Chain Fatty Acid Receptors and Cardiovascular Function. Int J Mol Sci 2022;23:3303. [Crossref] [PubMed]
- Lu Y, Zhang Y, Zhao X, et al. Microbiota-derived short-chain fatty acids: Implications for cardiovascular and metabolic disease. Front Cardiovasc Med 2022;9:900381. [Crossref] [PubMed]
- Dowis K, Banga S. The Potential Health Benefits of the Ketogenic Diet: A Narrative Review. Nutrients 2021;13:1654. [Crossref] [PubMed]
- Luong TV, Abild CB, Bangshaab M, et al. Ketogenic Diet and Cardiac Substrate Metabolism. Nutrients 2022;14:1322. [Crossref] [PubMed]
- Costa TJ, Linder BA, Hester S, et al. The janus face of ketone bodies in hypertension. J Hypertens 2022;40:2111-9. [Crossref] [PubMed]
- Panasevich MR, Morris EM, Chintapalli SV, et al. Gut microbiota are linked to increased susceptibility to hepatic steatosis in low-aerobic-capacity rats fed an acute high-fat diet. Am J Physiol Gastrointest Liver Physiol 2016;311:G166-79. [Crossref] [PubMed]
- Potrykus J, White RL, Bearne SL. Proteomic investigation of amino acid catabolism in the indigenous gut anaerobe Fusobacterium varium. Proteomics 2008;8:2691-703. [Crossref] [PubMed]
- Sekizuka T, Ogasawara Y, Ohkusa T, et al. Characterization of Fusobacterium varium Fv113-g1 isolated from a patient with ulcerative colitis based on complete genome sequence and transcriptome analysis. PLoS One 2017;12:e0189319. [Crossref] [PubMed]
- Costa LM, Mendes MM, Oliveira AC, et al. Dietary inflammatory index and its relationship with gut microbiota in individuals with intestinal constipation: a cross-sectional study. Eur J Nutr 2022;61:341-55. [Crossref] [PubMed]
- Sun Z, Song ZG, Liu C, et al. Gut microbiome alterations and gut barrier dysfunction are associated with host immune homeostasis in COVID-19 patients. BMC Med 2022;20:24. [Crossref] [PubMed]
- He Q, Zhang Y, Ma D, et al. Lactobacillus casei Zhang exerts anti-obesity effect to obese glut1 and gut-specific-glut1 knockout mice via gut microbiota modulation mediated different metagenomic pathways. Eur J Nutr 2022;61:2003-14. [Crossref] [PubMed]
- Fang XY, Qi LW, Chen HF, et al. The Interaction Between Dietary Fructose and Gut Microbiota in Hyperuricemia and Gout. Front Nutr 2022;9:890730. [Crossref] [PubMed]
- Burnstock G, Novak I. Purinergic signalling and diabetes. Purinergic Signal 2013;9:307-24. [Crossref] [PubMed]
- Usta CK, Adan G, Ozdem SS. The effects of adenosine on isolated right atrial preparations from streptozotocin-diabetic rats. J Auton Pharmacol 2001;21:191-5. [Crossref] [PubMed]
- Headrick JP, Peart JN, Reichelt ME, et al. Adenosine and its receptors in the heart: regulation, retaliation and adaptation. Biochim Biophys Acta 2011;1808:1413-28. [Crossref] [PubMed]
- Lv M, Li L, Li W, et al. Mechanism research on the interaction regulation of Escherichia and IFN-gamma for the occurrence of T2DM. Ann Palliat Med 2021;10:10391-400. [Crossref] [PubMed]
- Liu X, Cheng YW, Shao L, et al. Gut microbiota dysbiosis in Chinese children with type 1 diabetes mellitus: An observational study. World J Gastroenterol 2021;27:2394-414. [Crossref] [PubMed]
- Shiyovich A, Gilutz H, Plakht Y. Serum electrolyte/metabolite abnormalities among patients with acute myocardial infarction: comparison between patients with and without diabetes mellitus. Postgrad Med 2021;133:395-403. [Crossref] [PubMed]
(English Language Editors: C. Mullens and L. Huleatt)