Use of machine learning models for identification of predictors of survival and tumour recurrence in liver transplant recipients with hepatocellular carcinoma
Highlight box
Key findings
• Machine learning (ML) methods complementary to classical statistical analysis can help in developing prognostic models that quantify survival probability with the aim of helping clinicians in everyday practice and improving organ utilization in transplantation.
What is known and what is new?
• Hepatocellular carcinoma (HCC) is the most common primary liver malignant neoplasm and the third leading cause of cancer death worldwide. Liver transplantation (LT) is an established treatment in selected patients. To achieve optimal organ allocation and patient selection for LT, quantification of results and identification of predictors of survival and tumour recurrence is necessary.
• ML methods can help identify complex multidimensional and non-linear relationships between donor, recipient and tumour-specific parameters, thus improving existing metrics by creating an interpretable ranking system.
What is the implication, and what should change now?
• ML models created on large datasets can be compared to established risk scores in transplantation medicine and potentially identify risk factors in the process.
Introduction
Background
Hepatocellular carcinoma (HCC) is the most common primary liver malignant neoplasm and the third leading cause of cancer death worldwide. Liver transplantation (LT) is an established treatment in selected patients. Tumour size and number of lesions determine the acceptability criteria for LT. The Milan criteria proposed over 25 years ago are still viable and have been shown to provide good survival outcomes (>70% survival rate in 5 years, with a recurrence rate of <10% to 15%) (1,2). Over time, they were challenged with the aim to expand selection criteria and the University of California, San Francisco (UCSF) criteria emerged (1,3). HCC recurrence after LT is a major concern resulting in unsatisfactory outcomes due to continuous immunosuppression therapy. Early identification of patients at risk for HCC recurrence may lead to increased survival.
Rationale and knowledge gap
Recent studies pointed out that the morphologic characteristics of HCC alone are not sufficient and that tumour biology needs to be taken into consideration as well (4,5). It is also known that donor-recipient matching is an important factor in LT outcomes. To achieve optimal organ allocation and patient selection for LT, quantification of results and identification of predictors of survival and tumour recurrence are necessary (6). The amounts of clinical data are ever-increasing and a multidisciplinary approach with data scientists is paramount to develop better prognostic scores.
Objective
The ML approach, in addition to classical statistical methods, can be a useful tool in achieving these goals. ML methods can help identify complex multidimensional and non-linear relationships between donor, recipient and tumour-specific parameters (7-10). Survival analysis and prediction aim to analyse and predict a continuous variable. They differ from traditional regression in that a part of analysed data can only be partially observed in the defined period of time of the study. Anything that occurs after the defined end point of the study is right-censored for the study. In survival analysis the problem of right censored data is overcome by ML models adapted for survival, allowing them to be used in medical research. Random Survival Forest (RSF), Survival Support Vector Machine (SVM) and Survival Gradient Boosting are good examples and are commonly used (11,12). Random Forest consists of a large number of decision trees and each tree has an output in the form of a binary decision or real value. The final output of the whole forest is based on majority voting or mean decision of each tree (12,13). Survival SVM is a feasible method for a high number of variables, but it does not directly provide probability estimates. It inputs subsets of patient features into a high-dimensional space which is used in the decision process. These points are called support vectors and patient-patient similarity function is used for model training (7,12,14). Gradient Boosting combines several weak learners into a stronger one, and while RSF averages the prediction of independent decision trees, boosting models trains predictors consecutively and each successive predictor attempts to correct the errors of the previous ones (7,12,15). On the other hand, Coxnet, a regularized Cox regression model, is a classical Cox (proportional hazards) model upgraded with a penalty term that regularizes coefficients with little predictive value to zero. It can be appealing if coefficients of many parameters want to be estimated where the classical Cox model falls apart due to potential multicollinearity of the dataset. Coxnet can also be seen as a machine learning (ML) method (7,12). We present this article in accordance with the TRIPOD reporting checklist (available at https://atm.amegroups.com/article/view/10.21037/atm-22-6469/rc).
Methods
Patients
Data consisting of pretransplant parameters from 170 adult patients transplanted for HCC were collected in the period from March 2013 to December 2019 at the University Hospital Merkur, Zagreb. The end point of this study and the time of follow up of the patients was January 2022. All patients underwent whole liver cadaveric transplantation using grafts from Donation after Brain Death (DBD) donors. The grafts were procured using University of Wisconsin solution with aortic and portal flush. The piggy-back technique was used for implantation. Standard immunosuppressive regimen included Tacrolimus, Mycophenolate Mofetil, and steroids with steroid tapering over 3 months. All recipients underwent regular follow-up visits with surveillance for HCC recurrence using cross sectional imaging every 3 months in the first 2 years post-transplant, and at least every 6 months thereafter. Patients with incidentally found HCC as well as retransplanted patients were excluded from the study. We also excluded three patients with missing tumour burden scores. This score is composed of several variables which were missing for those patients, and imputation was not considered feasible in these cases. All transplanted patients were within UCSF criteria (single tumor ≤6.5 cm or ≤3 tumors with the largest tumor diameter ≤4.5 cm with a total tumor diameter ≤8 cm) (1,3). The study was conducted in accordance with the Declaration of Helsinki (as revised in 2013). This study was reviewed and approved by the Ethics Committee of the University Hospital Merkur, Zagreb (No. 03/1-2180). All patients signed a general informed consent agreeing to the treatment and use of their anonymized clinical data. According to national and institutional regulations, special written consent is not needed for every particular study where anonymized clinical data are used.
Data collection
Data on sex, age, blood type, body mass index (BMI), aetiology of liver disease, time of listing and laboratory Model for End-Stage Liver Disease (MELD) were collected. Tumour-specific variables included tumour burden score and alpha fetoprotein (AFP) (16-18). Donors’ laboratory findings included sodium level, alanine transaminase (ALT), gamma – glutamyltransferase (GGT), bilirubin and C-reactive protein (CRP). Neutrophil to lymphocyte ratio (NLR) and platelet to lymphocyte ratio (PLR) from the recipients’ laboratory findings were also collected (19-21). Transarterial chemoembolization (TACE) was used as a bridging treatment for patients with an expected waiting time for transplant of more than three months. Data concerning the administration and number of TACE sessions was collected, and no other bridging modalities, such as ablations or resections, were employed. The Eurotransplant Donor Risk Index (ET-DRI) as well as the Balance of Risk score (BAR) were calculated (22,23). Upon arrival to the transplant centre, all donor livers undergo a frozen section biopsy and the degree of steatosis is assessed and classified, as well as the degree of fibrosis and periportal inflammation, in order to evaluate the quality of the liver graft. Cold ischemia time was recorded with an effort to keep it as short as possible. A detailed description of recipient and donor characteristics is presented in Table 1 and Table 2.
Table 1
Variables | Value (n=170) |
---|---|
Male sex | 139 (81.8) |
Age (years) | 62 [58–67] |
BMI (kg/m2) | 27.4 [24.7–30.5] |
lab.MELD | 10 [8–13] |
AFP (ng/mL) | 11 [6–74] |
Aetiology | |
Alcoholic liver disease | 81 (47.7) |
Hepatitis C virus | 41 (24.1) |
Other | 26 (15.3) |
Hepatitis B virus | 22 (12.9) |
Days on waiting list | 23 [8–44] |
TACE (yes) | 35 (20.6) |
Number of TACE treatments | |
0 | 137 (80.6) |
1 | 18 (10.6) |
2 | 5 (2.9) |
3 | 7 (4.1) |
4 | 3 (1.8) |
NLR | 2.2 [1.5–3.5] |
PLR | 76.6 [55.4–111] |
AB0 | |
0 | 60 (35.3) |
A | 59 (34.7) |
B | 30 (17.7) |
AB | 21 (12.3) |
Within Milan criteria | 113 (66.5) |
Tumour burden score | 4.5 [3.1–6.1] |
Data are presented as median [interquartile range] or n (%). BMI, body mass index; MELD, Model for End-Stage Liver Disease; AFP, alpha-fetoprotein; TACE, transarterial chemoembolization; NLR, neutrophil to lymphocyte ratio; PLR, platelet to lymphocyte ratio.
Table 2
Variables | Value (n=170) |
---|---|
Male sex | 139 (81.8) |
Age (years) | 62.5 [50–72] |
BMI (kg/m2) | 27 [24.7–28.9] |
Anti-Hbc (positive) | 22 (12.9) |
Sodium (mEq/L) | 147 [142–153] |
ALT (U/L) | 30 [17–64] |
GGT (U/L) | 38 [21–80] |
Bilirubin (mg/dL) | 11 [8–16] |
CRP (mg/L) | 162 [108–207] |
AB0 | |
0 | 65 (38.2) |
A | 62 (36.5) |
B | 29 (17.1) |
AB | 14 (8.2) |
Pancreas offered (yes) | 24 (14.1) |
Cardiac arrest (yes) | 29 (17.1) |
Steatosis | |
Negligible (0–5%) | 107 (62.9) |
Mild (5–30%) | 56 (32.9) |
Moderate (30–60%) | 7 (4.2) |
Cold ischemia time (h) | 6.9 [5.1–8.7] |
ET-DRI | 1.8 [1.6–2] |
BAR | 4 [3–5] |
Data are presented as median [interquartile range] or n (%). BMI, body mass index; Hbc, hepatitis B core antibody; ALT, alanine aminotransferase; GGT, gamma-glutamyl transferase; CRP, C-reactive protein; ET-DRI, Eurotransplant Donor Risk Index; BAR, Balance of Risk score.
Outcome
The primary outcome of this study was recurrence-free survival which includes death or tumour recurrence, whichever occurred first. Secondary endpoints evaluated overall and graft survival, as well as whether patients would experience HCC recurrence within the study period.
Statistical analysis
Data analysis and ML modelling were performed using the Python version 3.9 programming language with open source libraries for statistics and ML (pandas, NumPy, scikit-survival, SciPy, Statsmodels, seaborn and lifelines) (11,12). Descriptive statistical analysis, including visualization and outlier detection, was conducted on the entire dataset. Preparation of data for this complex analysis is the first step, and while collecting and classifying data is not an issue, missing data can eliminate patients from a study. Imputation of missing data with substituted values is a recognized statistical method. In this analysis, imputation was performed via chained regression method and our dataset had <1% of missing data (24). Chained regression starts with replacing all missing values with the mean values of each column. The column to be imputed first is the one containing the least amount of missing data, and it is considered a dependent variable, while all other columns are independent variables (including the columns containing missing values that were replaced with mean values). A regression model is then used to impute the missing values of the current column, and then the process is moved onto the next column. This was repeated for all columns with missing values up to 10 times, to ensure that the values obtained by regression converged, i.e., stopped changing between iterations (24). In order to estimate the impact of each variable on survival, a multivariate Cox proportional hazards model was developed on the whole dataset, after a non-parametric univariate Kaplan-Meier approach. We used a forward selection process based on predictive performance concordance index (CI) of each individual variable that is fit into the Cox model. The best final model, with highest CI, is described by the top 7 variables, which was selected in a 3-fold cross-validated grid search and implemented using the scikit-survival library. The final model is built on the whole dataset (11).
Machine learning models
The data were also processed through the Coxnet: the elastic net regularized Cox regression model. Regularization is a ML procedure that reduces overfitting by controlling the growth of coefficients. This is mathematically expressed by adding a penalty term to the Cox log partial likelihood loss function. This penalty term is multiplied by a hyperparameter (alpha) that defines the weight of the penalty. When alpha is set to zero, we have a standard Cox model. As alpha increases, the coefficients are eventually shrunk to zero. Finding the ideal set of features is part of the optimization procedure of the hyperparameter alpha. After choosing a specific alpha value, we can perform prediction, either in terms of a risk score using the corresponding scikit-survival function or in terms of the survival or cumulative hazard function (Figure 1) (11,12). ML modelling was comprised from methods adapted for survival analysis on censored data. We used RSF, Survival SVM and Survival Gradient Boosting models. Random forest is an effective ML approach for both classification and regression. This method is constructing each tree with a different bootstrap sample and selecting diverse features for split criteria at each node. The final prediction is determined by aggregating the results of individual trees. The depth of trees influences the model’s ability to control overfitting. For prediction, a sample is run through each tree until reaching a terminal node. At each terminal node, data is used to non-parametrically estimate survival and cumulative hazard functions with the Kaplan-Meier and Nelson-Aalen estimators respectively (Figure 2). The data were split into 75% for training and 25% for testing, with the model based on 1,000 trees (7,8,11,13). The standard ML technique SVM can also be extended for survival data, as it has the advantage of being able to handle complex, non-linear relationships and survival using kernel functions. We used both Linear Survival SVM and Kernel SVM (with custom kernels) and in both cases, we used a cross-validated grid search to determine the optimal hyperparameter alpha. The best model was obtained by Linear Survival SVM with regression objective (11,14). Similar to random forests, gradient boosting is an ensemble-based ML method based on multiple learners, however, the way they are combined is different. The final model is obtained by combining multiple “base learners” (predictors) that should be simple and slightly better than random guessing. The final prediction is the result of an additive model, whereby each of the base models sequentially improves and “boosts” the overall model. In this study, we employed 250 base learners, using the Cox partial likelihood as a loss function, with regression trees and component-wise least-squares as base learners and with 75–25% train-test split (11,15). The evaluation and selection of the best model were performed based on the 5-fold cross-validated CI. Although our final evaluation is performed on the test set and the selection of the best model is based on results in terms of the CI, it is important to note that a comparison between ML methods and classical statistical methods using CI alone is a limited view of the results, power, and objectives of these methods (11,12).
Results
Survival
The 1-, 3-, and 5-year post-transplant recurrence-free survival rates, as reported in Figure 3, were 78%, 70%, and 65%, respectively. Kaplan-Meier curves for 1-, 3- and 5-year post-transplant recipient survival time showed survival probability of 84%, 81%, and 80% respectively (Figure 4). Graft 1-, 3-, and 5-year survival time was 82%, 78%, and 76% respectively (Figure 5). Five-year HCC recurrence was observed in 19% of patients. Median follow up time was 3.6 years. Most recurrences occurred during the first three years of follow up. The main outcome of our models was recurrence free survival and 65.9% of censored data was recorded. Due to a much higher percentage of censored data (84.4%) HCC recurrence could not be estimated as a separate outcome in this dataset.
Model selection
According to the cross-validated CI, the RSF model performed the best (CI 0.72), followed by Survival SVM (CI 0.70). Overall, ML models outperformed Cox proportional hazards model, which had CI 0.52 within the development set. Coxnet had CI 0.62 and was also outperformed by previously mentioned ML models, although providing better score than Survival Gradient Boosting model (CI 0.60). Models ranked by CI are presented in Table 3.
Table 3
Method | CI |
---|---|
Random survival forest | 0.72 |
Survival support vector machine | 0.70 |
Elastic net regularized Cox model | 0.62 |
Survival gradient boosting | 0.60 |
Cox proportional hazards | 0.52 |
CI, concordance index.
Variable selection
Regarding feature selection, RSF pointed out several parameters: AFP, donor CRP, recipient’s age and NLR (Figure 6). As previously mentioned, Survival SVM does not provide probability estimates and in this analysis did not present variable selection. Cox proportional hazards model showed some similarity with our best ML model, as donor CRP (P<0.001, hazard ratio =1.01, confidence interval: 1.0021–1.0084) and AFP (P=0.002, hazard ratio =1.0001, confidence interval: 1.0000–1.0002) were shown to be detrimental variables in the analysis. Some variables like donor age (P=0.002, hazard ratio =0.96, confidence interval: 0.9416–0.9949) and number of TACE treatments (P=0.001, hazard ratio =1.66, confidence interval: 1.1214–2.4433) were also pointed out, but not found significant in other models. Consistently, Coxnet also showed a lot of similarity concerning parameter selection with the RSF model, pointing out donor CRP, recipient’s age and NLR as the most detrimental variables (Figure 7). Whether patients were within Milan criteria or not was not found to be significant using this analysis on our dataset.
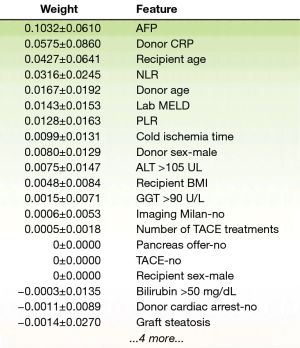
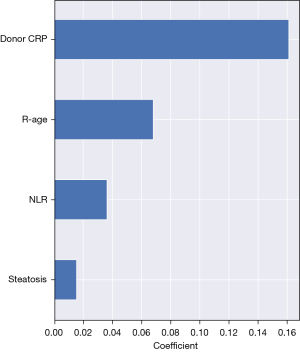
Discussion
Key findings
The volume of medical data is increasing on a daily basis and the need for a machine-driven way of processing huge amounts of data is evident. A multidisciplinary approach by clinicians and data scientists may facilitate the development of more precise prediction models. ML modelling, complementary to traditional statistical analysis, is a way to identify complex multidimensional and curvilinear relations between various parameters (7). This study has confirmed the predictive superiority of ML methods in comparison to traditional statistical analysis. We have also shown the importance of utilizing and comparing several different ML methods due to the specific ways in which they approach data processing. This study has also selected donor CRP as the most relevant predictor of recurrence free survival, which was not the case in similar studies. However, this needs to be confirmed with analysis of larger datasets and experience from other centers.
Strengths and limitations
The distinct feature of this study is that it is the first prospective analysis of a retrospectively collected dataset from our centre and that is a single centre study with homogenous management of patients. Using ML methods on smaller datasets is challenging, along with the delicacy of drawing general conclusions from the results. To maximize the number of data, imputation was done for missing parameters, excluding as few patients from the study as possible. The dataset, with objective parameters, can be affected by misclassification bias and any generalization of the results needs external validation on larger datasets. The major problem in ML is overfitting, which means that the model is well-suited to the existing training data, but performs poorly when given new, unseen data. To avoid biased conclusions, we took several steps to address that problem, which is commonly encountered with small datasets. First, we inspected the dataset for potential outliers which can be influential when there is a low number of an observation. We also selected relevant features, performed regularization and controlled the depth of tree-based models. To make the most of our dataset, we executed cross-validation (3- and 5-fold) to identify the best hyperparameters for all our ML methods. An example of finding the best hyperparameter alpha is given in Figure 8. Lastly, we tested our final models on a holdout test set, except for all Cox models, which was built on the entire dataset.
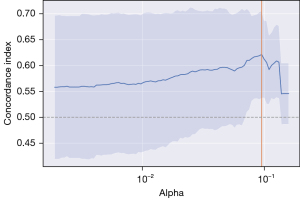
Comparison with similar studies
Even though ML has existed for decades, it is a relatively new concept in medical data analysis and its popularity is currently increasing. Comparing the ML approaches to statistical methods such as Cox proportional hazards regression is a topic often discussed in literature (25-27). Each approach has its strengths and weaknesses. ML is algorithmic in its nature and it can identify patterns in data through numerous iterations to learn the relationships between parameters. As opposed to classical statistical modelling no assumptions about underlying distributions are made. Statistical analysis relies on hypothesis testing, data analysis and explanation of the relation between variables, while ML focuses more heavily on prediction of unseen data. Learning a model can take into account a large number of variables with their complex, nonlinear relations, while statistical analysis usually focuses on a relatively small number of parameters (25). ML is increasingly becoming an invaluable tool for evaluation of pre- and post-transplant aspects of cadaveric and living-donor liver transplantation. High-performing imaging assessments enabled by ML algorithms can provide more accurate pathohistological evaluation of graft quality and streamline the process of liver segmentation. ML can also be used to facilitate timely detection of liver tumours in the setting of HCC, as well as predicting post-transplant morbidity and mortality with greater accuracy (8,28,29). Models predicting waitlist dropout are also reported, bearing particular relevance for patients with HCC (30). Transplant oncology is a rapidly evolving field, and optimizing organ allocation in tumor patients and predicting tumour recurrence after transplantation is of great significance (8,10,31). Traditional models for patient selection and prediction of recurrence in liver transplantation for HCC rely on classical statistical methods and may be limited in a complex multifactorial setting. Nam et al. developed a model (MoRAL-AI) that uses deep neural networks to predict the HCC recurrence, taking into account tumour biology, as indicated by biomarkers, and imaging-assessed tumour size (32). Ivanics et al. conducted a comparative evaluation of multiple ML models to develop the Toronto postliver transplantation HCC recurrence calculator. Their research showed that the Coxnet model had the best metrics and outperformed RSF, survival SVM, and neural networks (DeepSurv), and demonstrated the importance of exploring various ML models on data analysis (7). The application of a ML technique has the potential to make use of retrospective data to create more precise prognostic models. However, this can also be seen as a disadvantage, depending on the quality of the dataset. In comparison to similar studies, our approach was to investigate several different ML methods and the Cox proportional hazards regression on our dataset. Creating a predictive calculator or a score based on a limited number of patients from a single center can lead to imprecise outcomes due to potential biases. However, identifying predictors of survival is important for comparison with experience from other centers. External validation of similar studies is needed to obtain clinically relevant results.
Explanations of findings
Several variables stood out as having an impact on survival and tumour recurrence and some appeared in more than one model. The most detrimental variable in all the models was donor CRP. CRP is a non-specific acute phase reactant associated with various inflammatory diseases, sepsis and malignant tumours, that is widely available, inexpensive and has been in clinical use for many years. It is synthetized in hepatocytes, both in normal and HCC cells, and can reflect the degree of local inflammation since it encourages proliferation of hepatocytes and promotes HCC growth (33-36). Inflammation creates a microenvironment that favours DNA damage and neoangiogenesis, thus facilitating tumour growth and creating a vicious circle in which tumour creates inflammation that helps it develop (37). In literature, CRP is described as a prognostic factor of several types of cancer-oesophageal squamous cell carcinoma, cervical cancer and non-small cell lung cancer (38-40). Elevated CRP levels were also found to be predictive of overall survival and tumour recurrence in non-transplanted HCC patients after liver resection or treatment with locoregional therapies (41). Albeit the evidence for influence of cadaveric donor CRP on LT is scarce in literature, previous studies have reported that an elevated CRP in HCC patients undergoing living donor LT is predictive of a poor outcome (42,43). NLR as an index has demonstrated its value in infections, cardiovascular and inflammatory diseases, and in several types of primary and metastatic cancer. The role of NLR in HCC has emerged after the observation that sorafenib treatment in HCC patients had significantly better survival benefit in those with low NLR (19,20,44). It is considered that NLR represents the balance between the protumour inflammatory status and the antitumour adaptive immunity. Increase in NLR is suggestive of an increase in overall inflammatory status or a decrease in adaptive immunity. Hence inflammation, a stimulating factor in tumour microenvironment and a well-known indicator of tumour progression, was found important in survival and tumour recurrence in our group through two separate variables in our analysis. We considered NLR as a continuous variable with a reference range according to Forget et al., even though the optimal “cut-off point” in clinical settings has been debated in literature (45-47).
AFP is a widely accepted marker with prognostic significance in HCC, and also the only tumour marker routinely used for prognostication and treatment selection of patients with HCC. Even though exact tumour staging can only be confirmed after histologic study of the explanted liver (tumour size and number, vascular invasion, differentiation), AFP is considered to be a representative parameter that correlates with vascular invasion, and thus can be very predictive of HCC recurrence (48-50). In some of the recent studies, it was not determined that AFP is a good prognostic marker for patient survival, but the rate of tumour recurrence showed a positive correlation with elevated AFP values (51,52). In our group, the median value of AFP was 11 ng/mL and HCC recurrence was reported in 19% of patients. Since AFP was found to be significant in the RSF model as well as in the Cox proportional hazards multivariate analysis, we can conclude that moderate elevation of AFP may be associated with increased incidence of HCC recurrence.
The share of elderly people in the general population is increasing and so is the age of transplant recipients. The elderly tend to have more comorbid conditions that can affect postoperative complications and survival (53,54). The mean age in our cohort was 62.3±7.1 years which supports the claim that LT is successful and feasible in older population. Although older age has been highlighted as a risk factor in our models, our results suggest that recipients should be carefully selected according to their comorbidities and that old age alone is not an exclusion factor for LT. Identifying other risk factors in combination with age could help in optimal patient selection.
Implications and actions needed
Besides previously mentioned and used ML models, deep learning with artificial neural networks is a well-established and popular method that is increasingly used in many different professional areas today (8). Unfortunately, it was not applicable to our dataset due to the small number of patients (for this method) in our cohort. In our opinion, rather than one method replacing the other, ML modelling is better considered as complementary to classical statistics. The strength of the Cox proportional hazard model lies in its interpretable parameter estimates, which have a straightforward meaning in terms expected hazard rate. Although this model works well with small datasets, the feature selection process requires careful consideration and expert medical knowledge, and should be based on the confidence interval of individual variables or statistical significance (P<0.05). ML methods, such as we used in this study, offer more flexible alternatives for analyzing large, complex, heterogeneous data with a nonlinear relationship. They can intrinsically perform feature selection providing a list of more influential variables without straightforward interpretation. We must be cognizant of the different objectives of both ML and classical statistical methods. Classical statistics is better suited for interpretation and describing the relationships between variables and the outcome of survival, while ML techniques (e.g., using a holdout test set and cross-validating hyperparameter search) are focused on creating the best possible model for accurate predictions of time of event, without intending to explicitly state the relationships between variables.
Conclusions
In conclusion, ML methods complementary to classical statistical analysis can help in developing prognostic models to quantify survival probability with the aim of helping clinicians in everyday practice and improving organ utilization in transplantation. Also, ML models created on large datasets can be compared to established risk scores in transplantation medicine and potentially identify risk factors in the process.
Acknowledgments
We would like to thank Ivan Stresec, Faculty of Electrical Engineering and Computing, Department of Electronics, Microelectronics, Computer and Intelligent Systems, Zagreb, Croatia, for his help with the implementation of the data imputation procedure. His expertise was instrumental in the success of this project.
Funding: This research has been supported by
Footnote
Reporting Checklist: The authors have completed the TRIPOD reporting checklist. Available at https://atm.amegroups.com/article/view/10.21037/atm-22-6469/rc
Data Sharing Statement: Available at https://atm.amegroups.com/article/view/10.21037/atm-22-6469/dss
Peer Review File: Available at https://atm.amegroups.com/article/view/10.21037/atm-22-6469/prf
Conflicts of Interest: All authors have completed the ICMJE uniform disclosure form (available at https://atm.amegroups.com/article/view/10.21037/atm-22-6469/coif). All authors report that the article processing charge has been supported by the European Regional Development Fund under the grant KK.01.1.1.01.0009 (DATACROSS). The authors have no other conflicts of interest to declare.
Ethical Statement: The authors are accountable for all aspects of the work in ensuring that questions related to the accuracy or integrity of any part of the work are appropriately investigated and resolved. The study was conducted in accordance with the Declaration of Helsinki (as revised in 2013). This study was reviewed and approved by the Ethics Committee of the University Hospital Merkur, Zagreb (No. 03/1-2180). All patients signed a general informed consent agreeing to the treatment and use of their anonymized clinical data. According to national and institutional regulations, special written consent is not needed for every particular study where anonymized clinical data are used.
Open Access Statement: This is an Open Access article distributed in accordance with the Creative Commons Attribution-NonCommercial-NoDerivs 4.0 International License (CC BY-NC-ND 4.0), which permits the non-commercial replication and distribution of the article with the strict proviso that no changes or edits are made and the original work is properly cited (including links to both the formal publication through the relevant DOI and the license). See: https://creativecommons.org/licenses/by-nc-nd/4.0/.
References
- Bento de Sousa JH, Calil IL, Tustumi F, et al. Comparison between Milan and UCSF criteria for liver transplantation in patients with hepatocellular carcinoma: a systematic review and meta-analysis. Transl Gastroenterol Hepatol 2021;6:11. [Crossref] [PubMed]
- Mazzaferro V, Bhoori S, Sposito C, et al. Milan criteria in liver transplantation for hepatocellular carcinoma: an evidence-based analysis of 15 years of experience. Liver Transpl 2011;17:S44-57. [Crossref] [PubMed]
- Patel SS, Arrington AK, McKenzie S, et al. Milan Criteria and UCSF Criteria: A Preliminary Comparative Study of Liver Transplantation Outcomes in the United States. Int J Hepatol 2012;2012:253517. [Crossref] [PubMed]
- Mehta N, Bhangui P, Yao FY, et al. Liver Transplantation for Hepatocellular Carcinoma. Working Group Report from the ILTS Transplant Oncology Consensus Conference. Transplantation 2020;104:1136-42. [Crossref] [PubMed]
- Andreou A, Gül S, Pascher A, et al. Patient and tumour biology predict survival beyond the Milan criteria in liver transplantation for hepatocellular carcinoma. HPB (Oxford) 2015;17:168-75. [Crossref] [PubMed]
- Briceño J, Ciria R, de la Mata M. Donor-recipient matching: myths and realities. J Hepatol 2013;58:811-20. [Crossref] [PubMed]
- Ivanics T, Nelson W, Patel MS, et al. The Toronto Postliver Transplantation Hepatocellular Carcinoma Recurrence Calculator: A Machine Learning Approach. Liver Transpl 2022;28:593-602. [Crossref] [PubMed]
- Khorsandi SE, Hardgrave HJ, Osborn T, et al. Artificial Intelligence in Liver Transplantation. Transplant Proc 2021;53:2939-44. [Crossref] [PubMed]
- Ayllón MD, Ciria R, Cruz-Ramírez M, et al. Validation of artificial neural networks as a methodology for donor-recipient matching for liver transplantation. Liver Transpl 2018;24:192-203. [Crossref] [PubMed]
- Schoenberg MB, Bucher JN, Koch D, et al. A novel machine learning algorithm to predict disease free survival after resection of hepatocellular carcinoma. Ann Transl Med 2020;8:434. [Crossref] [PubMed]
- Pedregosa F, Varoquaux G, Gramfort A, et al. Scikit-learn: Machine Learning in Python. J Mach Learn Res 2011;12:2825-30.
- Pölsterl S. scikit-survival: A Library for Time-to-Event Analysis Built on Top of scikit-learn. J Mach Learn Res 2020;21:1-6.
- Breiman L. Random forests. Mach Learn 2001;45:5-32. [Crossref]
- Huang S, Cai N, Pacheco PP, et al. Applications of Support Vector Machine (SVM) Learning in Cancer Genomics. Cancer Genomics Proteomics 2018;15:41-51. [PubMed]
- Zhang Z, Zhao Y, Canes A, et al. Predictive analytics with gradient boosting in clinical medicine. Ann Transl Med 2019;7:152. [Crossref] [PubMed]
- Tsilimigras DI, Moris D, Hyer JM, et al. Hepatocellular carcinoma tumour burden score to stratify prognosis after resection. Br J Surg 2020;107:854-64. [Crossref] [PubMed]
- Moris D, Shaw BI, McElroy L, et al. Using Hepatocellular Carcinoma Tumor Bur-den Score to Stratify Prognosis after Liver Transplantation. Cancers (Basel) 2020;12:3372. [Crossref] [PubMed]
- Notarpaolo A, Layese R, Magistri P, et al. Validation of the AFP model as a predictor of HCC recurrence in patients with viral hepatitis-related cirrhosis who had received a liver transplant for HCC. J Hepatol 2017;66:552-59. [Crossref] [PubMed]
- Kwon HM, Moon YJ, Jung KW, et al. Neutrophil-to-lymphocyte ratio is a predictor of early graft dysfunction following living donor liver transplantation. Liver Int 2019;39:1545-56. [Crossref] [PubMed]
- Johnson PJ, Dhanaraj S, Berhane S, et al. The prognostic and diagnostic significance of the neutrophil-to-lymphocyte ratio in hepatocellular carcinoma: a prospective controlled study. Br J Cancer 2021;125:714-16. [Crossref] [PubMed]
- Hu DH, Yu SM. Association between platelet to lymphocyte ratio (PLR) and overall survival (OS) of hepatocellular carcinoma (HCC): A meta-analysis. Cell Mol Biol (Noisy-le-grand) 2017;63:30-2. [Crossref] [PubMed]
- Braat AE, Blok JJ, Putter H, et al. The Eurotransplant donor risk index in liver transplantation: ET-DRI. Am J Transplant 2012;12:2789-96. [Crossref] [PubMed]
- Martínez JA, Pacheco S, Bachler JP, et al. Accuracy of the BAR score in the pre-diction of survival after liver transplantation. Ann Hepatol 2019;18:386-92. [Crossref] [PubMed]
- Little R, Rubin D. Statistical analysis with missing data. 3rd ed. Hoboken, NJ, USA: Wiley; 2019.
- Wingfield LR, Ceresa C, Thorogood S, et al. Using Artificial Intelligence for Predicting Survival of Individual Grafts in Liver Transplantation: A Systematic Review. Liver Transpl 2020;26:922-34. [Crossref] [PubMed]
- Ley C, Martin RK, Pareek A, et al. Machine learning and conventional statistics: making sense of the differences. Knee Surg Sports Traumatol Arthrosc 2022;30:753-7. [Crossref] [PubMed]
- Rajula HSR, Verlato G, Manchia M, et al. Comparison of Conventional Statistical Methods with Machine Learning in Medicine: Diagnosis, Drug Development, and Treatment. Medicina (Kaunas) 2020;56:455. [Crossref] [PubMed]
- Veerankutty FH, Jayan G, Yadav MK, et al. Artificial Intelligence in hepatology, liver surgery and transplantation: Emerging applications and frontiers of re-search. World J Hepatol 2021;13:1977-90. [Crossref] [PubMed]
- Tran J, Sharma D, Gotlieb N, et al. Application of machine learning in liver transplantation: a review. Hepatol Int 2022;16:495-508. [Crossref] [PubMed]
- Kwong A, Hameed B, Syed S, et al. Machine learning to predict waitlist dropout among liver transplant candidates with hepatocellular carcinoma. Cancer Med 2022;11:1535-41. [Crossref] [PubMed]
- Nitski O, Azhie A, Qazi-Arisar FA, et al. Long-term mortality risk stratification of liver transplant recipients: real-time application of deep learning algorithms on longitudinal data. Lancet Digit Health 2021;3:e295-305. [Crossref] [PubMed]
- Nam JY, Lee JH, Bae J, et al. Novel Model to Predict HCC Recurrence after Liver Transplantation Obtained Using Deep Learning: A Multicenter Study. Cancers (Basel) 2020;12:2791. [Crossref] [PubMed]
- Carr BI, Ince V, Bag HG, et al. CRP is a superior and prognostically significant inflammation biomarker for hepatocellular cancer patients treated by liver transplantation. Clin Pract (Lond) 2021;18:1626-32. [PubMed]
- Meischl T, Rasoul-Rockenschaub S, Györi G, et al. C-reactive protein is an independent predictor for hepatocellular carcinoma recurrence after liver transplantation. PLoS One 2019;14:e0216677. [Crossref] [PubMed]
- Fink M, Starkey G, Perini M, et al. C-reactive protein before liver transplantation is predictive of post-transplant mortality. Transplantation 2018;102:S872. [Crossref]
- Sieghart W, Pinter M, Hucke F, et al. Single determination of C-reactive protein at the time of diagnosis predicts long-term outcome of patients with hepatocellular carcinoma. Hepatology 2013;57:2224-34. [Crossref] [PubMed]
- Hernandez-Gea V, Toffanin S, Friedman SL, et al. Role of the microenvironment in the pathogenesis and treatment of hepatocellular carcinoma. Gastroenterology 2013;144:512-27. [Crossref] [PubMed]
- Shimada H, Nabeya Y, Okazumi S, et al. Elevation of preoperative serum C-reactive protein level is related to poor prognosis in esophageal squamous cell carcinoma. J Surg Oncol 2003;83:248-52. [Crossref] [PubMed]
- Polterauer S, Grimm C, Seebacher V, et al. The inflammation-based Glasgow Prognostic Score predicts survival in patients with cervical cancer. Int J Gynecol Cancer 2010;20:1052-7. [Crossref] [PubMed]
- O'Dowd C, McRae LA, McMillan DC, et al. Elevated preoperative C-reactive protein predicts poor cancer specific survival in patients undergoing resection for non-small cell lung cancer. J Thorac Oncol 2010;5:988-92. [Crossref] [PubMed]
- Imai N, Kinoshita A, Onoda H, et al. Persistent elevated C-reactive protein after treatment is an independent marker of a poor prognosis in patients with hepatocellular carcinoma. Clin Transl Oncol 2013;15:575-81. [Crossref] [PubMed]
- Na GH, Kim DG, Han JH, et al. Inflammatory markers as selection criteria of hepatocellular carcinoma in living-donor liver transplantation. World J Gastroenterol 2014;20:6594-601. [Crossref] [PubMed]
- Mori S, Choi Y, Park MS, et al. Usefulness of preoperative C-reactive protein and alpha-fetoprotein levels for prognostication of patients with hepatocellular carcinoma after living donor liver transplantation. Hepatogastroenterology 2014;61:2353-58. [PubMed]
- Bruix J, Cheng AL, Meinhardt G, et al. Prognostic factors and predictors of sorafenib benefit in patients with hepatocellular carcinoma: Analysis of two phase III studies. J Hepatol 2017;67:999-1008. Erratum in: J Hepatol 2018;69:990-1. [Crossref] [PubMed]
- Forget P, Khalifa C, Defour JP, et al. What is the normal value of the neutrophil-to-lymphocyte ratio? BMC Res Notes 2017;10:12. [Crossref] [PubMed]
- Vano YA, Oudard S, By MA, et al. Optimal cut-off for neutrophil-to-lymphocyte ratio: Fact or Fantasy? A prospective cohort study in metastatic cancer patients. PLoS One 2018;13:e0195042. [Crossref] [PubMed]
- Liu J, Lin PC, Zhou BP. Inflammation fuels tumor progress and metastasis. Curr Pharm Des 2015;21:3032-40. [Crossref] [PubMed]
- Koch C, Bette T, Waidmann O, et al. AFP ratio predicts HCC recurrence after liver transplantation. PLoS One 2020;15:e0235576. [Crossref] [PubMed]
- Mazzaferro V, Sposito C, Zhou J, et al. Metroticket 2.0 Model for Analysis of Competing Risks of Death After Liver Transplantation for Hepatocellular Carcino-ma. Gastroenterology 2018;154:128-39. [Crossref] [PubMed]
- Zhang X, Li J, Shen F, et al. Significance of presence of microvascular invasion in specimens obtained after surgical treatment of hepatocellular carcinoma. J Gastroenterol Hepatol 2018;33:347-54. [Crossref] [PubMed]
- Schraiber LDS, de Mattos AA, Zanotelli ML, et al. Alpha-fetoprotein Level Predicts Recurrence After Transplantation in Hepatocellular Carcinoma. Medicine (Baltimore) 2016;95:e2478. [Crossref] [PubMed]
- Hakeem AR, Young RS, Marangoni G, et al. Systematic review: the prognostic role of alpha-fetoprotein following liver transplantation for hepatocellular carcinoma. Aliment Pharmacol Ther 2012;35:987-99. [Crossref] [PubMed]
- Gil E, Kim JM, Jeon K, et al. Recipient Age and Mortality After Liver Transplantation: A Population-based Cohort Study. Transplantation 2018;102:2025-32. [Crossref] [PubMed]
- Gómez-Gavara C, Charco R, Tapiolas I, et al. Recipient Age for Liver Transplantation: Should It Be Limited? A Propensity Score Matching Analysis of a Large European Series. Transplant Proc 2020;52:1442-49. [Crossref] [PubMed]