Surgical research using national databases
Introduction
Surgical procedures represent one of the largest expenditures in healthcare and are projected to constitute over 7% of the US gross domestic product by 2025 (1). With the progressive shift towards bundled payments, healthcare institutions are increasingly tracking perioperative complications, readmissions, and overall costs. The enactment of the Patient Protection Affordable Care Act has placed an increased emphasis on institutions being held accountable for patient outcomes, and surgeon performance is now being monitored through various quality measures (2). This shift towards tracking perioperative outcomes along with the advent of “big data” accrual technology has led to the increased utilization of national clinical databases in surgical research.
As healthcare reform continues to evolve, surgeon reimbursement is projected to transition toward bundled payment programs and pay-for-performance models. Inevitably there is a vested interest in quantifying the expected risks and benefits of a given procedure in a particular cohort of patients (3-5). This interest has spurred the use of large-volume national databases to assess perioperative complications, costs, and resource consumption for surgical procedures across multiple subspecialties (6).
Large-volume databases
Large-volume databases can include all patients or a predefined sample of patients from a broad (national) or narrow (state) geographic area, and are composed of patient-encounter records from more than one specialty or procedure. These databases should not be confused with smaller registries that typically collect data prospectively for a particular diagnosis (diabetes) or procedure (joint replacement) (7,8). While smaller registries often record disease or procedure-specific outcomes such as functional scores, patient-satisfaction, and radiographic reports; large-volume databases contain more generalized information such as demographics, comorbidities, cost, length of stay, as well as procedure and diagnosis codes.
Large-volume databases have several unique advantages that have fueled their popularity amongst surgeon-researchers. They allow for the investigation of rare disease states (e.g., hemophilia) and uncommon complications (e.g., deep venous thrombosis), which is not always possible with prospective clinical studies due to limitations in sample size, efficiency, and ethical considerations (9). They also facilitate studies analyzing surgical procedure variation by region, gender, age, ethnicity, socioeconomic status, comorbidities, insurance-type, and institution-type (i.e., private versus academic, for-profit versus non-profit). Large-volume databases are also ideal for examining temporal trends for the management of a specific surgical procedure or the utilization of a given surgical procedure. Several large-volume databases have also been utilized to develop preoperative and postoperative risk stratification protocols for a given procedure. The results from large database studies provide better evidence-based guidelines for decisions made regarding patient care preoperatively, intraoperatively, and postoperatively (10-12).
Although databases have the ability to answer an array of surgical hypotheses, the information contained within different national databases is highly variable and, as such, the questions that can be answered and the conclusions that can be reached are limited by the extent of the available data. For example, some databases only track inpatient surgeries which may lead to a selection bias if a study is examining a procedure that is performed on both an outpatient and inpatient basis. Appropriate database selection mandates a complete understanding of a database’s composition, as this will allow for a thorough assessment of its strengths and limitations.
Large-volume national databases can broadly be categorized as either administrative or clinical (13). These categorizations are mostly dependent on where the data is derived from (13). Examples of each of database category are presented in Figure 1. Administrative databases are generally compiled from billing information and were not originally created for clinical research (13). These databases obtain their information typically from two sources: requests to insurers for healthcare payments and claims for clinical services (13). Clinical databases, on the other hand, are composed of a given patient population with defined patient information (13). These databases were designed to record and track information, allowing for the investigation of specific clinical questions.
The critical first step when using a national database is to design a research question that can be answered appropriately using the specific database. Research protocols have been developed to facilitate studies using large-volume national databases (14), but these protocols do not obviate the need to fully understand the limitations of a database. The second step is to then select an appropriate database that can test a given hypothesis, but does not compromise the quality of the study by its inherent limitations. In this review we will highlight the composition, strengths, and weaknesses of the most commonly used large-volume national databases for surgical research that are not unique to any particular subspecialty (Table 1).
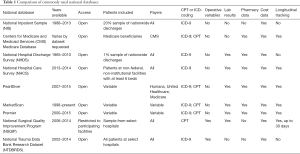
Full table
National administrative databases
National Inpatient Sample (NIS)
The Healthcare Cost and Utilization Project (HCUP) is a federally mandated program managed by the Agency of Healthcare Research and Quality. The HCUP is composed of several regional and national databases, the largest of which is the NIS (15). Across all publicly available databases, the NIS is the largest all-payer inpatient database in the United States. The database aggregates information from over 1,000 hospitals, over 8 million discharges, and dates back to 1988.
The database does not record each individual discharge from a given institution but rather records a 20% stratified systemic random sample of all discharges. Each discharge can contain over 100 data points allowing for the stratification of variables by region, ownership (i.e., public versus private), location (i.e., urban versus rural), size, and resident educational programs. The data provided by NIS is unweighted, therefore when conducting studies investigating nationwide trends, it is important to use appropriate weighting variables that are provided by HCUP and determined by an institution’s relative contribution to the NIS. Stratification of patient or procedural information is achieved through International Classification of Diseases, 9th edition (ICD-9) procedural and diagnostic codes.
Significant strengths of the NIS database are founded in its overall number of patients and the volume of data recorded with each discharge. Additionally, access to the database is relatively affordable at $160–$350 per year of data requested. Currently, it is the largest national database composed of all payers, and is frequently used for nationwide analysis of a given procedure (16,17).
There are several limitations of the NIS database that are important to consider in any study utilizing this database. First, the NIS database only records inpatient events and does not allow for longitudinal tracking of patients. Therefore, only inpatient complications may be assessed for a given procedure, which likely underestimates the true complication rate. A second major limitation is the database only contains pre-discharge information, making it difficult to distinguish comorbidities from complications (18). Lastly, it does not contain operative variables such as anesthesia type, length of surgery, or blood loss which can be important variables to consider in surgical research. Despite the limitations of the NIS database, it remains commonly utilized in surgical research.
Centers for Medicare and Medicaid Services (CMS) Medicare database
The CMS runs Medicare, a national social insurance program of the United States (19). The administrative CMS Medicare database can be utilized to conduct surgical research (20). The database is composed of Medicare enrollees—citizens of the United States who are disabled, have end-stage renal disease or amyotrophic lateral sclerosis, or are over age 65.
The administrative database is composed of information extracted by coders reviewing filed claims for Medicare patients and many datasets are available from the year 2000 and after (9,21). This database contains information from various time points, allowing patients to be tracked longitudinally as they progress through the various phases of healthcare. This allows for the assessment of both pre and post-operative comorbidities and complications. Data can be stratified from inpatient hospitalizations, outpatient surgeries, outpatient clinic visits, physical therapy, and pharmaceutical consumption. A major, and perhaps underutilized, advantage of this CMS database is that it can be linked with other databases such as cancer registries and other payers (Medicaid). Lastly, the CMS database can be searched using both ICD-9 and current procedural terminology (CPT) codes, which can allow for more specific identification of diagnoses and associated procedures in comparison to isolated ICD-9 code stratification.
Although the CMS Medicare database contains both inpatient and outpatient information, it is limited to specific comorbidities and patients over 65 years old. This limits the generalizability of the findings obtained from this database. It does not contain information on patients with healthcare managed organization (HMO) insurance, private insurance or the uninsured, thereby further limiting the generalizability of studies utilizing this database (22). Additionally, it only contains information extracted from billable events; unbilled procedures may not be captured. Lastly, obtaining a comprehensive dataset can exceed $200,000, but individual datasets are for the most part cost-free (23). Overall, the CMS Medicare database is a valuable resource for surgical research, particularly due to its ability for extensive and comprehensive longitudinal tracking of a patient through various phases of care before and after a given surgical procedure.
National Hospital Discharge Survey (NHDS) and National Hospital Care Survey (NHCS)
The NHDS was published annually from 1965 to 2010 by the National Center for Health Statistics at the Centers for Disease Control and Prevention (24). The database is a national probability survey from a systematic random sample of discharges from 438 non-federal hospitals across all fifty states. Data was collected through both manual and automated systems. Overall, the NHDS represented 1% of all discharges in the United States.
Data from the NHDS is currently available for years 1970 to 2009 allowing for extensive temporal trend analysis; however, most sample abstracts are limited to seven diagnosis codes and four procedure codes. Additionally, the NHDS excluded hospitals from participating in the survey based on average length of stay, potentially resulting in a disproportionate representation from short-stay facilities. Similar to the NIS, the NHDS only allows for analysis of inpatient comorbidities and complications. Lastly, the NHDS does not allow investigators to follow patients longitudinally which limits study questions applicable to this database.
Currently, data collected in the NHDS has been integrated into the new NHCS (25). The NHCS began data collection in 2011 and has several advantages over the NHDS. First, it allows for the longitudinal tracking of patients through various phases of care, similar to the CMS Medicare database. Second, it no longer uses average length of stay as an exclusion criterion, thereby sampling a greater variety of hospitals than the NHDS. Third, it now utilizes electronic data collection providing more timely availability of research data. Fourth, healthcare institution characteristics are recorded, allowing for analysis of procedures stratified by facility characteristics. The NHCS also collects data from the National Hospital Ambulatory Medical Care Survey (NHAMCS), which is composed of data from the emergency, outpatient, and ambulatory surgery patient care settings (26). As of 2016, only unweighted datasets from 2013 and 2014 NHCS data have been available.
Private analytics databases
The digitization of healthcare through electronic medical records has allowed private analytics companies to extract and analyze substantial amounts of data from insurance claims and hospital-level data sources. These companies have synthesized and packaged this data for commercial purchase. The sources from which these private companies obtain their data is highly variable with respect to payer, hospitals included, and healthcare documents analyzed. Some of the more commonly used private national databases for surgical research include PearlDiver (Pearl-Diver Technologies, Fort Wayne, IN, USA), MarketScan (Truven Health Analytics, Ann Arbor, MI, USA), and Premier (Premier Inc., Charlotte, NC, USA).
The PearlDiver database currently contains over 4 billion patient records obtained from analysis of private insurance claims from Humana and United Healthcare, as well as government claims from Medicare (27). The MarketScan database extracts information primarily from administrative claims and enrollment data for patients in employer-sponsored health plans in the United States (28). The Premier Healthcare Database contains information from over 700 private and academic hospitals in the United States which corresponds to approximately 20% of all hospitalizations in the country (29).
Although these private analytics databases have resulted in a contribution to surgical research, they are not without limitations. The populations of these databases are not randomly sampled and thus generalizations and trend analysis must be interpreted with caution. Additionally, access to private databases can be expensive, ranging from $5,000–$50,000 per year or study cohort requested (23).
National clinical databases
National Surgical Quality Improvement Program (NSQIP)
The NSQIP database has its origins in the Veterans Affairs (VA) healthcare system (30). In the 1980s, the United States government developed the Veterans Affairs Surgical Quality Improvement Program (VASQIP) after noticing high rates of morbidity and mortality following surgical procedures in VA patients (31). After the noted success of the VASQIP in decreasing perioperative complications, its methods were implemented in private sector hospitals and found to be similarly effective (32). The current NSQIP database was developed by the American College of Surgeons to improve the quality of surgical care. It is a nationally validated database, and studies have demonstrated the ability of the NSQIP to improve surgical outcomes (33-35).
The NSQIP database is peer-controlled and collects information on patients who undergo elective and select trauma surgical procedures. Most high energy mechanisms of trauma such as motorcycle accidents or gunshot injuries are excluded. Contributing institutions are located both within the United States [680] and abroad [81] (36). The database records a wide array of clinical information in addition to surgery-specific variables such as operative time, blood transfusion, anesthesia type, and estimated blood loss. The data recorded in the NSQIP database dates back to 2006. A major strength of the database is that data is abstracted by thoroughly trained “nurse reviewers” who use a standardized set of definitions, and inclusion and exclusion criteria. These reviewers may track greater than 135 data points for a given patient undergoing a surgical procedure. The inter-rater reliability of data extraction by these reviewers proves to be consistent (37).
The primary limitation of the NSQIP database is it only tracks 30-day morbidity and mortality after a surgical procedure. The database also does not provide specific information regarding severity of comorbidities, indications for surgery, or specific outcomes related to a given procedure. Lastly, free access to the database is limited to contributing institutions, and the infrastructure to contribute to the NSQIP may exceed $100,000 as a full-time “nurse reviewer” is needed. This high cost has led to a disproportionate contribution to the NSQIP from large teaching hospitals (38). Aside from its limitations, the NSQIP database is widely believed to be the most precise and accurate database available for measuring patient outcomes after surgery given its high rate of complete data sets, operative data points, validation, and inter-rater reliability (39).
National Trauma Data Bank (NTDB) Research Dataset (RDS)
The NTDB RDS is a national database that was created by the American College of Surgeons in 1997 (40). The database is structured as a national probability sample from both level I and II trauma centers in the United States. It currently contains over five million cases from over 900 contributing trauma centers, and current datasets date back to 2002.
A major strength of the National Trauma Data Bank Research Dataset (NTDBRDS) is the ability to analyze trauma-specific outcomes such as mechanism of injury and injury severity score in a large number of patients from all payers. Operative variables are also searchable, and data is collected based on established definitions by a trained data abstractor. The database also contains demographic parameters, discharge status, and ICD-9 procedure and diagnosis codes. The cost to obtain NTDBRDS is $300 per dataset requested.
Similar to the NIS database, the NTDBRDS only contains inpatient data limited to the index hospitalization, and stratification by CPT codes is not available. Others have criticized the distribution of contributing institutions to the NTDBRDS, stating participating hospitals may not be representative of the national population (41). Lastly, individuals treated for traumatic injuries at trauma centers of level III, IV, or V designation are excluded from the database.
Limitations of national databases in surgical research
Although national databases have contributed significantly to surgical research over the past decade, the data used to obtain these results is subject to many of the aforementioned limitations that must be recognized by all parties interpreting the results. A common limitation shared by the majority of these databases is that they are dependent on ICD-9 and/or CPT coding to isolate comorbidities, diagnoses, procedures, and complications. These codes were not originally developed for research purposes and their use may only be valid for certain diagnoses, procedures, or complications (42,43). Furthermore, the extraction of these CPT and ICD-9 codes often comes from insurance claims or hospital-level records which may be influenced by reimbursement strategies or coded by non-medical team members. Two recent studies demonstrated higher rates of postoperative complications in the NIS database when compared to the NSQIP database for the same procedures, despite NIS only capturing inpatient complications while NSQIP captures morbidity and mortality up to 30 days after the index procedure (44,45). As stated before, the NIS database uses billing records as a component of its data extraction while the NSQIP data is collected by a trained reviewer using established definitions.
Secondly, the dependence on ICD-9 and CPT codes results in less reproducible and less reliable surgical research due to their high variability. Variations in coding may be due to clerical errors or various interpretations of discharge summaries, operative records, or other healthcare documentation. Variation in coding can also be due to annual updates of ICD-9 and CPT codes, in which the definition of a particular code may change. For example, in 2009 the ICD-9 code for deep venous thrombosis changed to differentiate acute and chronic deep venous thrombosis events (9). Additional codes may also be added to more thoroughly describe new procedures as seen with hip arthroscopy. The replacement of ICD-9 with ICD-10 coding will cause initial confusion, but will eventually allow for the isolation of more specific diagnoses and procedures, particularly with regards to laterality.
With regard to patient sampling, differing strategies across different databases can inevitably contribute to differing results for similar patient cohorts undergoing the same procedure. A single database may also change its own sampling strategy, such as the NIS in 2012 and NTDB in 2006, further complicating studies that analyze temporal trends. Additionally, databases may define certain complications or comorbidities differently. The heterogeneity of these databases due to the aforementioned reasons cannot be overstated. A recent meta-analysis found that database study results can change from statistical significance in one direction to the opposite direction depending on the database utilized (46).
The establishment of statistically significant differences is likely when analysis is performed on such a large sample size. However, statistical significance does not equivocate clinical significance. Authors must clearly define a meaningful clinically significant difference in their methodology prior to conducting the analysis.
Lastly, the use of databases to analyze and compare the cost of certain surgical procedures can lead to improved efficiency and resource utilization. However, many of the databases used in surgical research report the billed amount on the insurance claim as the “cost”. The amount billed is highly variable and is dependent on a given institution’s charge master which is not readily available or transparent. In most cases the billed amount will not reflect the reimbursement actually paid to the hospital or provider. Reimbursement rates are highly variable based on region and insurance-type, therefore studies reporting cost may be inaccurate.
Future directions
The recent advent of database research has advanced the study of both common and rare procedures across numerous surgical subspecialties. National databases provide a relatively cost-effective and efficient resource to conduct potentially well-executed studies. As these databases continue to evolve and amass more data, further studies are needed to validate their findings by comparing them to internal chart reviews, prospective studies, and other independent databases.
As health informatics continues to improve, the exchange and extraction of data should become more efficient, allowing for a greater number of more accurately recorded clinical variables to be analyzed. Additionally, the integration of electronic medical records into national databases may allow for superior longitudinal tracking of patients, thereby providing a better understanding of factors contributing to post-surgical outcomes and complications. The creation of more multidisciplinary databases that factor in elements of primary care, anesthesiology, and rehabilitative medicine would increase the quality of research possible through national databases. Lastly, national databases need to place an increased emphasis on recording procedure-specific outcomes (such as weight-loss after bariatric surgery or functional outcome scores after joint replacement) to enhance the application of such database studies in clinical practice.
Conclusions
The use of national databases for surgical research has allowed for a better understanding of perioperative complications, temporal trends, risk stratification, and cost-analysis. As national databases continue to expand and include more accurate and procedure-specific information, database-driven surgical research will continue to improve in quality and clinical meaningfulness. However, a thorough understanding of a database’s structure, sampling methodology, and inherent limitations will be needed to ensure accurate and reproducible results as well as retain a sophisticated interpretation of study results.
Acknowledgements
None.
Footnote
Conflicts of Interest: The authors have no conflicts of interest to declare.
References
- Muñoz E, Muñoz W 3rd, Wise L. National and surgical health care expenditures, 2005-2025. Ann Surg 2010;251:195-200. [Crossref] [PubMed]
- Rogers F, Horst M, To T, et al. Factors associated with patient satisfaction scores for physician care in trauma patients. J Trauma Acute Care Surg 2013;75:110-4. [Crossref] [PubMed]
- Birkmeyer NJ, Birkmeyer JD. Strategies for improving surgical quality--should payers reward excellence or effort? N Engl J Med 2006;354:864-70. [Crossref] [PubMed]
- Greenwald AS, Bassano A, Wiggins S, et al. Alternative Reimbursement Models: Bundled Payment and Beyond: AOA Critical Issues. J Bone Joint Surg Am 2016;98:e45. [Crossref] [PubMed]
- Hackbarth G, Reischauer R, Mutti A. Collective accountability for medical care--toward bundled Medicare payments. N Engl J Med 2008;359:3-5. [Crossref] [PubMed]
- Adkinson JM, Casale MT, Kim JY, et al. So You Have a Research Idea: A Survey of Databases Available for Plastic Surgery Research. Plast Reconstr Surg 2016;137:680-9. [Crossref] [PubMed]
- Clements MA, Foster NC, Maahs DM, et al. Hemoglobin A1c (HbA1c) changes over time among adolescent and young adult participants in the T1D exchange clinic registry. Pediatr Diabetes 2016;17:327-36. [Crossref] [PubMed]
- Cafri G, Paxton EW, Love R, et al. Is There a Difference in Revision Risk Between Metal and Ceramic Heads on Highly Crosslinked Polyethylene Liners? Clin Orthop Relat Res 2016. [Epub ahead of print]. [Crossref] [PubMed]
- Patel AA, Singh K, Nunley RM, et al. Administrative Databases in Orthopaedic Research: Pearls and Pitfalls of Big Data. J Am Acad Orthop Surg 2016;24:172-9. [Crossref] [PubMed]
- McMillan MT, Allegrini V, Asbun HJ, et al. Incorporation of Procedure-specific Risk Into the ACS-NSQIP Surgical Risk Calculator Improves the Prediction of Morbidity and Mortality After Pancreatoduodenectomy. Ann Surg 2016. [Epub ahead of print]. [Crossref] [PubMed]
- Dasenbrock HH, Smith TR, Gormley WB, et al. 108 Adverse Events After Carotid Endarterectomy: A National Surgical Quality Improvement Program Analysis. Neurosurgery 2016;63 Suppl 1:146. [Crossref] [PubMed]
- Ventral Hernia Outcome Collaborative, Mitchell TO, Holihan JL, et al. Do risk calculators accurately predict surgical site occurrences? J Surg Res 2016;203:56-63. [Crossref] [PubMed]
- Murphy M, Alavi K, Maykel J. Working with existing databases. Clin Colon Rectal Surg 2013;26:5-11. [Crossref] [PubMed]
- Chang DC, Talamini MA. A review for clinical outcomes research: hypothesis generation, data strategy, and hypothesis-driven statistical analysis. Surg Endosc 2011;25:2254-60. [Crossref] [PubMed]
- Healthcare Cost and Utilization Project: Overview of the National (Nationwide) Inpatient Sample (NIS). Available online: http://www.hcup-us.ahrq.gov/nisoverview.jsp, accessed July 16, 2016.
- Dimick JB, Welch HG, Birkmeyer JD. Surgical mortality as an indicator of hospital quality: the problem with small sample size. JAMA 2004;292:847-51. [Crossref] [PubMed]
- Kotwall CA, Maxwell JG, Brinker CC, et al. National estimates of mortality rates for radical pancreaticoduodenectomy in 25,000 patients. Ann Surg Oncol 2002;9:847-54. [Crossref] [PubMed]
- Bekkers S, Bot AG, Makarawung D, et al. The National Hospital Discharge Survey and Nationwide Inpatient Sample: the databases used affect results in THA research. Clin Orthop Relat Res 2014;472:3441-9. [Crossref] [PubMed]
- Marjoua Y, Bozic KJ. Brief history of quality movement in US healthcare. Curr Rev Musculoskelet Med 2012;5:265-73. [Crossref] [PubMed]
- Centers for Medicare and Medicaid Services. Medicare Coverage Database. Available online: https://www.cms.gov/medicare-coverage-database/, accessed July 16, 2016.
- Research Data Assistance Center. Data Availability. Available online: http://www.resdac.org/cms-data/file-availability, accessed July 16, 2016.
- Fisher ES, Baron JA, Malenka DJ, et al. Overcoming potential pitfalls in the use of Medicare data for epidemiologic research. Am J Public Health 1990;80:1487-90. [Crossref] [PubMed]
- Pugely AJ, Martin CT, Harwood J, et al. Database and Registry Research in Orthopaedic Surgery: Part I: Claims-Based Data. J Bone Joint Surg Am 2015;97:1278-87. [Crossref] [PubMed]
- Centers for Disease Control and Prevention. National Center for Health Statistics. National Hospital Discharge Survey. Available online: http://www.cdc.gov/nchs/nhds/, accessed July 16, 2016.
- Centers for Disease Control and Prevention. National Center for Health Statistics. National Hospital Care Survey. Available online: http://www.cdc.gov/nchs/nhcs/index.htm, accessed July 20, 2016.
- Centers for Disease Control and Prevention. National Center for Health Statistics. Ambulatory Health Care Data. Available online: http://www.cdc.gov/nchs/ahcd/index.htm, accessed July 16, 2016.
- PearlDiver. About PearlDiver. Available online: http://www.pearldiverinc.com/about-us.php, accessed July 17, 2016.
- Truven Health Analytics. Life Sciences. Research and Data Analytic. Available online: http://truvenhealth.com/markets/life-sciences/products/data-tools, accessed July 17, 2016.
- Premier Research Services. Available online: https://www.premierinc.com/transforming-healthcare/healthcare-performance-improvement/premier-research-services/, accessed July 17, 2016.
- Khuri SF, Daley J, Henderson W, et al. The National Veterans Administration Surgical Risk Study: risk adjustment for the comparative assessment of the quality of surgical care. J Am Coll Surg 1995;180:519-31. [PubMed]
- Khuri SF, Daley J, Henderson W, et al. The Department of Veterans Affairs' NSQIP: the first national, validated, outcome-based, risk-adjusted, and peer-controlled program for the measurement and enhancement of the quality of surgical care. National VA Surgical Quality Improvement Program. Ann Surg 1998;228:491-507. [Crossref] [PubMed]
- Khuri SF, Henderson WG, Daley J, et al. Successful implementation of the Department of Veterans Affairs' National Surgical Quality Improvement Program in the private sector: the Patient Safety in Surgery study. Ann Surg 2008;248:329-36. [Crossref] [PubMed]
- Hall BL, Hamilton BH, Richards K, et al. Does surgical quality improve in the American College of Surgeons National Surgical Quality Improvement Program: an evaluation of all participating hospitals. Ann Surg 2009;250:363-76. [PubMed]
- Berenguer CM, Ochsner MG Jr, Lord SA, et al. Improving surgical site infections: using National Surgical Quality Improvement Program data to institute Surgical Care Improvement Project protocols in improving surgical outcomes. J Am Coll Surg 2010;210:737-41, 741-3.
- Cohen ME, Liu Y, Ko CY, et al. Improved Surgical Outcomes for ACS NSQIP Hospitals Over Time: Evaluation of Hospital Cohorts With up to 8 Years of Participation. Ann Surg 2016;263:267-73. [Crossref] [PubMed]
- American College of Surgeons. ACS NSQIP Hospitals. Available online: https://www.facs.org/search/nsqip-participants?allresults=, accessed July 15, 2016.
- Shiloach M, Frencher SK Jr, Steeger JE, et al. Toward robust information: data quality and inter-rater reliability in the American College of Surgeons National Surgical Quality Improvement Program. J Am Coll Surg 2010;210:6-16. [Crossref] [PubMed]
- Sheils CR, Dahlke AR, Kreutzer L, et al. Evaluation of hospitals participating in the American College of Surgeons National Surgical Quality Improvement Program. Surgery 2016. [Epub ahead of print]. [Crossref] [PubMed]
- Kim L, Mabry C, Klimberg VS. Quality of benchmarks for assessment of care will influence outcome. Ann Surg 2007;245:672-3. [Crossref] [PubMed]
- American College of Surgeons. NTDB Research Data Set and National Sample Program. Available online: https://www.facs.org/quality-programs/trauma/ntdb/datasets, accessed July 17, 2016.
- Pugely AJ, Martin CT, Harwood J, et al. Database and Registry Research in Orthopaedic Surgery: Part 2: Clinical Registry Data. J Bone Joint Surg Am 2015;97:1799-808. [Crossref] [PubMed]
- Goff SL, Pekow PS, Markenson G, et al. Validity of using ICD-9-CM codes to identify selected categories of obstetric complications, procedures and co-morbidities. Paediatr Perinat Epidemiol 2012;26:421-9. [Crossref] [PubMed]
- Best WR, Khuri SF, Phelan M, et al. Identifying patient preoperative risk factors and postoperative adverse events in administrative databases: results from the Department of Veterans Affairs National Surgical Quality Improvement Program. J Am Coll Surg 2002;194:257-66. [Crossref] [PubMed]
- Bohl DD, Russo GS, Basques BA, et al. Variations in data collection methods between national databases affect study results: a comparison of the nationwide inpatient sample and national surgical quality improvement program databases for lumbar spine fusion procedures. J Bone Joint Surg Am 2014;96:e193. [Crossref] [PubMed]
- Bohl DD, Basques BA, Golinvaux NS, et al. Nationwide Inpatient Sample and National Surgical Quality Improvement Program give different results in hip fracture studies. Clin Orthop Relat Res 2014;472:1672-80. [Crossref] [PubMed]
- Madigan D, Ryan PB, Schuemie M, et al. Evaluating the impact of database heterogeneity on observational study results. Am J Epidemiol 2013;178:645-51. [Crossref] [PubMed]