Precision medicine with electronic medical records: from the patients and for the patients
The ideas of incorporating individual variabilities for prevention and treatment of diseases are getting paramount importance nowadays in modern medicine, otherwise known as precision medicine. Precision medicine aims at revolutionising modern human treatment by combining some or all of the available behavioral, biomarker, cellular, molecular, clinical, environmental, genetic, phenotypic, genomic, metabolomic, proteomic, transcriptomic, antibodyomic, physiological and psychological parameters of patients (1,2). It promotes targeted treatment and side-effect minimization of individual patients based on various related characteristics that distinguish one patient from others with similar kind of clinical presentations (3). It is true that every physician tries best to prescribe appropriate treatment with minimum side effects for patients, but there is no golden standard or protocol for it. There are a few research works to support such ideas and very few full-proof ready-made tool/software/system (4) to guide them. Precision medicine aims at such standardizations and developments at a global level by incorporating data taken from the patients for their better treatment.
Precision medicine redefines and unites taxonomic systems with different sets of variables by which the medical and scientific communities may classify complex diseases. It aims at predictive, preventive, personalized and participatory (P4) treatment of each individual patient (5). However, a few researchers have also advocated for inclusion of the population perspective (an additional P) with these ‘P4’ for better results (6,7). Recently we have come across a paper by Li et al. (8) which emphasizes on Identification of type 2 diabetes (T2D) subgroups through topological analysis of a population of 11,200 individual electronic medical record (EMR) and genotype patient data.
T2D is a chronic progressive metabolic disease. It represents a syndrome with disordered carbohydrate and fat metabolism. The disease is based on genetic, environmental and life-style related factors. With obesity epidemics, the chance of T2D is becoming a threat to human population. The disease is multi-faceted, with increasing prevalence world-wide. It has already been associated with a cluster of metabolic syndromes (9), obesity (10) and impaired life style (11) among others. According to World Health Organization (http://www.who.int/mediacentre/factsheets/fs312/en/, visited on 27th September 2016 at 4:34 PM, Indian Standard Time), diabetes will be the seventh leading cause of death in 2030.
In this scenario, Li et al. (8) have done an introspective study on a 2,551 T2D unsupervised patient-patient similarity network, where associations were based on 73 clinical features identified from EMR data. They have mapped the complexity of patient populations and identified three distinguished disease-enriched clusters representing three subtypes of T2D with specific clinical and genetic characteristics. The distinct subtypes were then studied for disease-comorbidity, SNP association, gene association, gene-phenotype association, pathway enrichment and toxicity function enrichment (Table 1). Each level of this information has opened a separate avenue of further research and investigation for T2D. The established protocol of their study has the flexibility to be extended into temporal as well as longitudinal patient data of other complex diseases. It has highlighted utility of higher-dimensional clinical data for defining clinical phenotypes of complex diseases and facilitating genetic marker discovery.
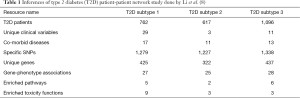
Full table
Similar investigations have been carried out on a similar but smaller scale for other anomalies, i.e., a designed year-long cohort study of 2,022 individuals for arrhythmia (12), a case study of lung cancer (13), a pilot report study of 250 people with cancer (14) and a case series study of three adults with polypharmacy (15) among others. Use of EMRs has also found in better identification and treatment of depression (16), rheumatoid arthritis (17) and colorectal cancer (18) among others. A plethora of machine learning techniques, including natural language processing (16,18), classification algorithm incorporating notes of physicians (17), clustering (8) and text mining (19) among others have been found to be in use for precision medicine related predictions.
However, approaches of precision medicine have their limitations (20). Some limitations can be overlooked, but others loom large in the face of a clear minimalistic treatment of a patient. All the hospital systems do not incorporate or fall back on EMRs for patient treatment. Patients always drop out of treatment. Sometimes exact start time of a disease in a patient cannot be covered with EMRs, especially when a patient comes after certain treatments at other hospitals. It happens due to lack of a proper data integration system among multiple hospitals and health care institutions. Partial EMRs are a limitation for precision medicine studies. Another major obstacle is the storage of patient data in varied electronic record platforms and formats. Integration of these platforms becomes sometimes difficult and results in data loss. A universal standard in this regard is highly recommended.
Different instruments and protocols are used for measurement of a clinical variable. Sometimes the quality of measurement is compromised for various test methods, technical reasons, cost and human errors. A person dealing with only EMRs is unable to distinguish these minute differences. Some clinical variables like blood pressure vary throughout the day. Thus, the time of sample collection of these variables should be included in EMRs. Often temporal aspect of sample collection is not depicted in old EMRs, making them incomplete. Moreover, finding a good sample size of patients with similar kind of phenotype is difficult. Even defining an ideal sample size for a wide-range study is tricky as EMRs do not guarantee exhaustive data of an area or population. EMR based precision medicine research heavily falls back on machine learning techniques for study and analysis purposes, although they come with their own set of limitations (false positives, over-fitting and many others).
Considering all these facts, precision medicine seems like a huge leap of faith in predictive science at present. It is a fast-paced, large-scale and integrated approach. It can be a bright future of medical movement and patient care. But, are we becoming over-optimistic here? Precision medicine may end up with little significant achievements, as nature, human beings, their genes, diseases and disease causative agents, all of them are a part of a continuous ongoing evolution process. It is true that by associating genotype information with EMRs, we are creating huge amount of data, which may help in better treatment of our future generations. But, are we exhausting our resources in a suboptimal manner by primarily focusing on precision medicine? Should we not focus on other avenues of improving human health as well? Many researchers have reservations regarding these questions (21).
A few baby steps have been taken towards precision medicine with relevant research works, which make us pause and think about their findings and future promises. Individual hospitals maintain their own EMRs. Area wise health systems like the Geisinger Health System (GHS) (4) also exist. GHS is located in north central and northeastern Pennsylvania. It has a system-wide biobanking program, called MyCode, to link clinical samples and EMR data. It creates resources for modern cost-effective flexible translational research for precision medicine. However, population coverage of these studies is still very limited. Future wide range P5 (predictive, preventive, personalized, participatory and population-wise) studies will demand better coverage with huge monetary, research and human resource investments universally. At present, only a handful of developed countries use and apply EMR for their health care around the world. A lot of awareness has to be generated world-wide, especially in developing and under-developed countries where the maintenance of health records is neglected. The winding road towards precision medicine is long, unknown and uncertain. Nonetheless, precision medicine will invariably lead us towards a global standard of patient care.
Acknowledgements
Losiana Nayak, one of the authors, acknowledges University Grants Commission, India for a UGC Post-Doctoral Fellowship [No. F.15-1/2013-14/PDFWM-2013-14-GE-ORI-19068(SA-II)].
Footnote
Provenance: This is a Guest Commentary commissioned by Section Editor Hui Kong, MD, PhD (Department of Respiratory Medicine, The First Affiliated Hospital of Nanjing Medical University, Nanjing, China).
Conflicts of Interest: The authors have no conflicts of interest to declare.
References
- Collins FS, Varmus H. A new initiative on precision medicine. N Engl J Med 2015;372:793-5. [Crossref] [PubMed]
- Chen R, Snyder M. Promise of personalized omics to precision medicine. Wiley Interdiscip Rev Syst Biol Med 2013;5:73-82. [Crossref] [PubMed]
- Jameson JL, Longo DL. Precision Medicine—Personalized, Problematic, and Promising. Obstetrical & Gynecological Survey 2015;70:612-4. [Crossref]
- Carey DJ, Fetterolf SN, Davis FD, et al. The Geisinger MyCode community health initiative: an electronic health record-linked biobank for precision medicine research. Genet Med 2016;18:906-13. [Crossref] [PubMed]
- Hood L, Flores M. A personal view on systems medicine and the emergence of proactive P4 medicine: predictive, preventive, personalized and participatory. N Biotechnol 2012;29:613-24. [Crossref] [PubMed]
- Khoury MJ, Gwinn ML, Glasgow RE, et al. A population approach to precision medicine. Am J Prev Med 2012;42:639-45. [Crossref] [PubMed]
- Austin ED, West J, Loyd JE, et al. Molecular Medicine of Pulmonary Arterial Hypertension: From Population Genetics to Precision Medicine and Gene Editing. Am J Respir Crit Care Med 2016. [Epub ahead of print]. [Crossref] [PubMed]
- Li L, Cheng WY, Glicksberg BS, et al. Identification of type 2 diabetes subgroups through topological analysis of patient similarity. Sci Transl Med 2015;7:311ra174. [Crossref] [PubMed]
- Kong X, Zhang X, Xing X, et al. The Association of Type 2 Diabetes Loci Identified in Genome-Wide Association Studies with Metabolic Syndrome and Its Components in a Chinese Population with Type 2 Diabetes. PLoS One 2015;10:e0143607. [Crossref] [PubMed]
- Kim YJ, Lee HS, Kim YK, et al. Association of Metabolites with Obesity and Type 2 Diabetes Based on FTO Genotype. PLoS One 2016;11:e0156612. [Crossref] [PubMed]
- Tuomilehto J, Lindström J, Eriksson JG, et al. Prevention of type 2 diabetes mellitus by changes in lifestyle among subjects with impaired glucose tolerance. N Engl J Med 2001;344:1343-50. [Crossref] [PubMed]
- Van Driest SL, Wells QS, Stallings S, et al. Association of Arrhythmia-Related Genetic Variants With Phenotypes Documented in Electronic Medical Records. JAMA 2016;315:47-57. [Crossref] [PubMed]
- Vargas AJ, Harris CC. Biomarker development in the precision medicine era: lung cancer as a case study. Nat Rev Cancer 2016;16:525-37. [Crossref] [PubMed]
- Rubin MA. Health: Make precision medicine work for cancer care. Nature 2015;520:290-1. [Crossref] [PubMed]
- Finkelstein J, Friedman C, Hripcsak G, et al. Potential utility of precision medicine for older adults with polypharmacy: a case series study. Pharmgenomics Pers Med 2016;9:31-45. [Crossref] [PubMed]
- Perlis RH, Iosifescu DV, Castro VM, et al. Using electronic medical records to enable large-scale studies in psychiatry: treatment resistant depression as a model. Psychol Med 2012;42:41-50. [Crossref] [PubMed]
- Liao KP, Cai T, Gainer V, et al. Electronic medical records for discovery research in rheumatoid arthritis. Arthritis Care Res (Hoboken) 2010;62:1120-7. [Crossref] [PubMed]
- Denny JC, Choma NN, Peterson JF, et al. Natural language processing improves identification of colorectal cancer testing in the electronic medical record. Med Decis Making 2012;32:188-97. [Crossref] [PubMed]
- Warrer P, Hansen EH, Juhl-Jensen L, et al. Using text-mining techniques in electronic patient records to identify ADRs from medicine use. Br J Clin Pharmacol 2012;73:674-84. [Crossref] [PubMed]
- Laper SM, Restrepo NA, Crawford DC. The challenges in using electronic health records for pharmacogenomics and precision medicine research. Pac Symp Biocomput 2016;21:369-80. [PubMed]
- Rubin R. Precision medicine: the future or simply politics? JAMA 2015;313:1089-91. [Crossref] [PubMed]