Forecasting and validating fat mass ratio models through anthropometric measurements and health-related factors among people with HIV: a cross-sectional investigation
Highlight box
Key findings
• Our study developed predictive models of fat mass ratio (FMR) in people with human immunodeficiency virus (HIV) (PWH), regardless of sex. Model 6 demonstrated superior predictive performance among the six proposed models.
What is known and what is new?
• Prior to this study, there was limited research on predictive models of FMR in PWH.
• This study introduces the first predictive models of FMR in PWH regardless of sex, filling a significant gap in healthcare provision for this population.
What is the implication, and what should change now?
• Early diagnosis of lipodystrophy, facilitated by these models, can lead to timely intervention and prevention of non-communicable diseases, particularly atherosclerosis.
• Health professionals should incorporate these models into routine monitoring protocols for PWH, enabling the identification of abnormal changes in body composition.
• There is a need for validation of these models across different populations and countries, to enhance accessibility and demonstrate their global predictive potential.
Introduction
In 2022, it was reported that approximately 630,000 people living with human immunodeficiency virus (HIV) (PWH) died worldwide, according to World Health Organization (WHO) [2023] (1). In 1996, Brazil became one of the first low- and middle-income countries to provide universal and free-of-charge antiretroviral therapy (ART) to all PWH (2). In this Brazilian context and considering that ART controls HIV infection and the development of acquired immunodeficiency syndrome (AIDS) (3), the treatment for PWH has increased patients’ life expectancy by over 30 years, and HIV is currently considered a manageable chronic disease (4). Nonetheless, side effects from ART treatment, together with HIV infection, can cause chronic non-communicable diseases (NCDs) (5). In the last few years, the amount of research regarding NCDs in PWH because of morphological and metabolic risk factors (6), and the consequent high number of AIDS-related deaths has largely improved. A common adverse health consequence of HIV infection is the “HIV lipodystrophy syndrome” (7). This syndrome is characterized by morphological changes such as the loss of subcutaneous adipose tissue (named “lipoatrophy”), and/or the accumulation of visceral adipose tissue (named “lipohypertrophy”) (7). Lipodystrophy syndrome triggers are multifactorial and include HIV infection, clinical stage of the disease, race/ethnicity, sex, physical activity level, eating habits, effects, and time of ART use (8,9). In addition, PWH experiences metabolic dysregulation such as dyslipidemia and increased blood hyperglycemia (10-12). Consequently, the association between body composition changes in PWH and the presence of metabolic risk factors leads to NCDs, especially atherosclerosis and diabetes (13,14).
Regarding the methods for screening body composition changes over time in lipodystrophy syndrome, dual-energy X-ray absorptiometry (DXA) is highlighted in the scientific literature (15). Cutoff points for the diagnosis of lipodystrophy, considering the fat mass ratio (FMR) using DXA, have been proposed for PWH (16-19). However, even with the advancement of the use of FMR in research involving lipodystrophy, DXA is not accessible. DXA’s high acquisition and operating cost restrict its use in clinical care and in studies with large samples, especially in low- and middle-income countries such as Brazil (20).
As an alternative and considering that FMR involves values of % body fat in the upper and lower limbs, anthropometric measurements are interesting choices for diagnosing and monitoring changes in body composition in lipodystrophy (21,22). Additionally, studies report the importance and accuracy of using anthropometry to identify metabolic and body composition change in PWH (23-25). For the diagnosis of lipodystrophy by estimating the FMR, anthropometry is an objective, safe, accurate, and low-cost tool. However, there is a scarcity of FMR prediction proposals in the scientific literature, with only one study by Dos Santos et al. (26) proposing the prediction and validation of FMR through anthropometric models specific to male and female PWH. However, the prevalence of lipodystrophy among the sexes in PWH is similar (26-28). Therefore, considering the estimation of FMR in PWH through anthropometric models regardless of sex may facilitate and expedite the diagnosis and monitoring of changes in body composition in lipodystrophy. As a result, our study aims to develop models that consider anthropometric and health-related factors to predict and validate FMR in PWH, regardless of sex. We present this article in accordance with the STROBE reporting checklist (29) (available at https://atm.amegroups.com/article/view/10.21037/atm-23-1946/rc).
Methods
Study population
For this cross-sectional study, PWH under ART treatment was enrolled from the Special Unit for the Treatment of Infectious Diseases at the Clinical Hospital from the University of São Paulo, Ribeirão Preto, Brazil. Enrollment of participants occurred from November 2013 to November 2014. Inclusion criteria encompassed people diagnosed with HIV, aged between 18 and 59 years, and receiving ART for a minimum duration of 6 months. Exclusion criteria comprised ongoing treatment for cancer or opportunistic infections (e.g., pneumocystis, histoplasmosis, tuberculosis), administration of medications known to affect body composition (e.g., testosterone, growth hormone, insulin growth factor), pregnancy, limb amputation, and involvement in supervised exercise regimens within the preceding 6 months. The research received approval from the Ethics Review Board of the Clinical Hospital at the University of São Paulo, Ribeirão Preto, Brazil, under process number 7082/2011, and was conducted in accordance with the principles outlined in the Declaration of Helsinki (as revised in 2013). All participants provided written informed consent before data collection. To achieve a maximum estimate error (5%) for FMR with an accuracy of 95%, a sample size of 100 to 110 PWH was necessary (30).
Procedures
DXA
The body composition of the participants was evaluated using the DXA Hologic instrument (model QDR 4500 W; software version 11.2; Bedford, MA, USA). The DXA measurements were conducted by a trained technician in accordance with established guidelines (31). The DXA scans provided the relative values of fat mass for the trunk and lower limbs. The FMR was used as the primary variable to diagnose lipodystrophy. The FMR was calculated as the percentage of trunk fat mass divided by the percentage of lower limb fat mass (16).
Anthropometric data
The study collected anthropometric measurements using standardized procedures. Body weight (kg) and height (cm) were measured using a Filizola® electronic anthropometric scale and a Filizola® aluminium stadiometer (Filizola® Professional, São Paulo, SP, Brazil), respectively. Skinfold thickness (SK) (mm) was assessed employing a Prime® caliper (Harpenden Scientific model) at six anatomical sites: triceps, subscapular, suprailiac, abdomen (horizontal), thigh, and medial calf, adhering to the prescribed protocols delineated by Harrison et al. [1988] (32). Body circumferences (BC) (cm) were measured with a 2 m Sanny® brand (São Bernardo do Campo, SP, Brazil) metal band with a latex device at the end, which was replaced every 20 evaluated participants. The BC measurements followed the guidelines described by Callaway et al. [1988] (33) and encompassed 19 anatomical sites, including the shoulder (greatest diameter), breast (fourth costosternal junction), waist (smallest diameter), abdomen (umbilical region), hip (widest diameter), right arm extended, right arm flexed, right forearm, right wrist, left arm extended, left arm flexed, left forearm, left wrist, right thigh (proximal), right medial calf (greatest diameter), right ankle (smallest circumference proximal to malleolus), left thigh (proximal), left medial calf (greatest diameter), and left ankle (smallest circumference proximal to malleolus). The BC measurements for the right arm extended, right arm flexed, left thigh (proximal), and left medial calf (greatest diameter) were adjusted for the respective segmental SK, as outlined by Lee et al. [2000] (34), to control for the influence of muscles on predictive models. The accuracy of the anthropometric measurements was ensured by obtaining SK and BC measurements three times by the same evaluator, and the median between all attempts was used as the final score.
Health-related factors
The study also collected health-related factors, called additional predictive variables, through interviews and clinical records. These factors included age (years), self-reported skin color consistent with Brazilian guidelines (i.e., White, Black, Asian, or Pardo Brazilians), an education level (years), time of HIV diagnosis (months since the diagnosis), time of exposure to ART (months since the beginning of treatment), type of ART (whether the patient uses protease inhibitor “PI” or not), CD4+ T-cell lymphocyte count (cells per microliter), and HIV viral load (copies per mm3) assessed within the last 6 months.
Statistical analysis
The data were entered into Microsoft Excel® and validated using double-key verification to ensure the highest accuracy and quality. An exploratory analysis was performed to check for outliers and data entry errors. Measures of central tendency were calculated for all continuous variables to describe the characteristics of the sample. Parametric statistical procedures were used, considering the central limit theorem (35). Categorical variables were presented as absolute (n) and relative (%) frequency.
Principal component analysis was used to select the independent variables for the generation of predictive models in an attempt to reduce the number of variables while not compromising the assumptions expected for predictions. Information on this process has been described previously (26). Nevertheless, elevated adjusted R2 values for the predictive models were not observed (data not presented). Consequently, a stepwise linear regression analysis was performed, incorporating all anthropometric variables and additional predictor variables associated with lipodystrophy. The resulting stepwise linear regression models were utilized to forecast FMR as measured by DXA. To mitigate multicollinearity, thresholds of variance inflation factor (VIF) ≤4.0 and eigenvalues ≥0.7 were adhered to (36). The adjusted R2 value was shown for each predictive model. Bland-Altman plots were used to verify the degree of agreement between the values of the predicted models and those measured by DXA (37). We developed binary (dummy) variables for the additional predictor variables ‘skin color’, ‘sex’, and ‘type of ART’ to investigate their effect on the models. The standard error of the estimate (SEE) in relative values was used to define the accuracy of predictive models. To investigate the limits of agreement between FMR predicted by DXA and FMR estimated by predictive models, we used 95% confidence interval (CI). Finally, to be considered valid, the model should meet the criteria described by Lohman [1992] (38), which includes no significant difference with the reference method and adjusted R2>0.70. In this study, the FMR predicted by the developed models serves as the primary outcome variable. Statistical analyses were conducted using SPSS® version 20.0, with a significance level set at α=5%
Cross-validation was performed utilizing the “leave-one-out” method, derived from the predicted residual error sum of squares (PRESS), to evaluate the efficacy of each generated predictive model (21). The Q2PRESS and SEEPRESS values were calculated for all predictive models. The PRESS analysis was conducted using Minitab® software version 17.
Results
A total of 125 PWH agreed to participate in this study. However, 19 of them were excluded because they did not meet the inclusion and/or exclusion criteria. Therefore, the final sample comprised 106 PWH, of which 65 were male. Descriptive analysis of anthropometric measurements, early life, and body composition variables in a sample of 106 people living with HIV from the Clinics Hospital of Medicine Faculty in Ribeirão Preto, São Paulo, Brazil are presented in Table 1. The mean age of the participants was 46.4±9.8 years, the mean time since HIV diagnosis was 114.3±79.3 months (9.5 years), the mean time under ART was 87.6±63.5 months (7.3 years), and the mean formal education time was 8.3±3.5 years. In terms of self-reported skin color, a higher frequency was observed among white individuals (n=67; 63.3%) and Pardo Brazilians (n=20; 18.8%), followed by Black individuals (n=10; 9.4%) and Asians (n=9; 8.5%).
Table 1
Variables | Participants (n=106) |
---|---|
Male | 65 (61.3) |
Age (years) | 46.4±9.8 (44.3–48.3) |
Body weight (kg) | 67.9±12.0 (65.6–70.3) |
Height (cm) | 164.9±9.5 (163.0–166.7) |
Diagnosis of HIV (months) | 114.3±79.3 (99.4–128.3) |
Exposure to ART (months) | 87.6±63.5 (75.5–99.5) |
Education level (years) | 8.3±3.5 (7.7–9.0) |
FMRDXA (%) | 1.11±0.34 (1.03–1.20) |
Race | |
White | 67 (63.3) |
Black | 10 (9.4) |
Asian | 9 (8.5) |
Brown | 20 (18.8) |
Type of ART | |
With-PI | 46 (43.4) |
Without-PI | 60 (56.6) |
Anthropometric measurements | |
Body circumferences (cm) | |
Left arm extended | 28.4±3.4 (27.8–29.1) |
Left arm contracted | 29.4±3.3 (28.9–30.1) |
Left forearm | 25.4±2.4 (24.9–25.7) |
Left wrist | 16.3±1.9 (16.1–16.7) |
Right arm extended | 29.0±3.3 (28.4–29.7) |
Right arm extended corrected | 25.3±2.8 (24.8–25.9) |
Right arm contracted | 30.0±3.2 (29.5–30.7) |
Right arm contracted corrected | 26.4±2.8 (25.8–26.9) |
Right forearm | 25.9±2.3 (25.4–26.3) |
Right wrist | 16.4±1.4 (16.1–16.1) |
Left thigh | 51.6±9.4 (49.6–53.2) |
Left medial calf | 34.7±3.2 (34.0–35.3) |
Left ankle | 21.4±1.7 (21.1–21.8) |
Right thigh | 53.2±6.9 (51.9–54.5) |
Right thigh corrected | 48.0±4.8 (47.0–49.0) |
Right medial calf | 34.8±3.4 (34.0–35.5) |
Right medial calf corrected | 31.9±3.2 (31.3–32.7) |
Right ankle | 21.3±1.7 (21.0–21.7) |
Shoulder | 105.3±8.1 (103.7–106.9) |
Breast | 93.4±9.0 (91.7–95.1) |
Waist | 87.0±9.2 (85.3–88.7) |
Abdomen | 91.2±12.4 (88.7–93.5) |
Hip | 94.0±9.3 (92.4–96.0) |
Skinfold thickness (mm) | |
Triceps | 11.8±7.5 (10.3–13.2) |
Subscapular | 20.6±8.4 (18.9–22.2) |
Suprailiac | 19.1±9.9 (17.3–21.0) |
Horizontal abdomen | 21.3±9.2 (19.7–23.1) |
Thigh | 16.8±11.3 (14.5–18.8) |
Medial calf | 9.0±7.2 (7.5–10.3) |
Data are presented as n (%), mean ± SD (95% CI). HIV, human immunodeficiency virus; ART, antiretroviral therapy; FMRDXA, fat mass ratio by dual-energy X-ray absorptiometry; PI, protease inhibitor; SD, standard deviation; CI, confidence interval.
Predictive models to estimate FMR
We proposed six predictive models to estimate FMR for PWH (Table 2). After conducting stepwise linear regression for the models, we observed variations in the R2adjusted values between 0.43 to 0.72, SEE 0.16 to 0.22, and 95% CI: 1.03 to 1.15. Considering the Lohman [1992] criteria, model number 6 was the best predictive model. Linear regression resulted in a high R2adjusted value of 0.72, SEE 0.16%, and 95% CI: 1.06 to 1.15 (38). The variables included were: SK of the thigh, BC of the waist, time under ART in months, SK of subscapular, formal education in years, and SK of the abdomen.
Table 2
Models | Independents variables | β | R2adjusted | SEE | 95% CI | Validation | ||||||
---|---|---|---|---|---|---|---|---|---|---|---|---|
SK thigh (mm) |
BC waist (cm) |
T ART (months) |
SK subscapular (mm) | Formal education (years) | SK abdomen (mm) | Q2PRESS | SPRESS | |||||
1 | −0.017±0.002 | 1.39±0.04 | 0.43 | 0.22 | 1.03 to 1.12 | 0.40 | 0.23 | |||||
2 | −0.018±0.002 | 0.013±0.002 | 0.26±0.18 | 0.59 | 0.19 | 1.03 to 1.12 | 0.57 | 0.19 | ||||
3 | −0.016±0.002 | 0.013±0.002 | 0.001±0.000 | 0.12±0.17 | 0.64 | 0.18 | 1.03 to 1.13 | 0.62 | 0.18 | |||
4 | −0.018±0.002 | 0.010±0.002 | 0.001±0.000 | 0.008±0.003 | 0.31±0.18 | 0.67 | 0.17 | 1.05 to 1.15 | 0.64 | 0.18 | ||
5 | −0.018±0.002 | 0.010±0.002 | 0.001±0.000 | 0.008±0.003 | 0.014±0.005 | 0.20±0.17 | 0.69 | 0.16 | 1.05 to 1.15 | 0.67 | 0.17 | |
6* | −0.015±0.002 | 0.010±0.002 | 0.001±0.000 | 0.010±0.003 | 0.014±0.005 | −0.007±0.003 | 0.21±0.17 | 0.72 | 0.16 | 1.06 to 1.15 | 0.70 | 0.17 |
*, model 6: fat mass ratio = 0.214 + [SK thigh (mm) × −0.015] + [BC waist (cm) × 0.010] + [T ART (months) × 0.001] + [SK subscapular (mm) × 0.010] + [formal education (years) × 0.014] + [abdomen SK (mm) × −0.007]. FMR, fat mass ratio; HIV, human immunodeficiency virus; SK thigh, skinfold thickness of thigh; BC waist, body circumference of waist; T ART (months), time under antiretroviral therapy in months; SK subscapular, skinfold thickness of subscapular; SK abdomen, skinfold thickness of abdomen; β, beta value; R2adjusted, r square adjusted; SEE, standard error of estimate; CI, confidence interval; Q2PRESS, Q2 of the predicted residual error sum of squares; SPRESS, S of the predicted residual error sum of squares.
Figure 1 shows the Bland-Altman plots for each predictive model. The agreement between the FMR by DXA and FMR by the predictive models was accurate since the models exhibited practically no bias (−0.03 to +0.04), with reduced limits of agreement, especially for the best predictive model suggested (−0.33 and +0.30) (Figure 1F). In addition, it was found that the predictive models tended to underestimate the FMR by DXA when the FMR values were higher. No significant association (r<0.4) was identified between the x and y axes of the Bland-Altman plots, indicating the absence of heteroscedasticity. Thus, the predictive models were accurately used to estimate FMR, and the extreme values of FMR were rarely outside the limits of agreement.
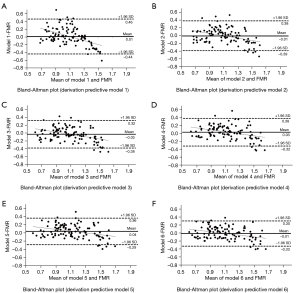
After validating the predictive models to estimate FMR, the best-suggested model “model 6” (Table 2) had a high adjusted value of Q2PRESS (0.70) and a small SPRESS (0.17). The models accurately predicted FMR due to the short interval for the limit of agreement observed in each predictive model by the Bland-Altman plots and the adjusted Q2PRESS value, mainly in the best predictive model suggested.
Discussion
The present study aimed to develop and validate sexless predictive models of FMR in PWH using anthropometric measurements and health-related factors. The models exhibited high determination coefficients and low prediction errors. Among the six proposed models, model 6 showed the best predictive performance due to the highest coefficient of determination for cross-validation and the lowest limit of agreement in the Bland-Altman plots. To the best of our knowledge, this is the first study proposing predictive models of FMR in PWH regardless of sex, which enhances the healthcare provided to this population.
A single Brazilian study has previously developed anthropometric predictive models for the diagnosis of lipodystrophy in PWH, which were sex-specific (26). Six models were generated for males (R2=0.50–0.77; SEE =0.14–0.20), in which the best model included the relationship between subscapular/medial calf SK, thigh SK, waist BC, years of education, time since HIV diagnosis, and type of ART. For females, five models were generated (R2=0.34 to 0.70; SEE =0.10–0.13), in which the best model included thigh skinfold, subscapular skinfold, time of exposure to ART, chest circumference, and race/ethnicity (Asian or non-Asians) (26). When comparing the cited models with the present research, we identified similar determination coefficients and forecast errors. However, our models did not exhibit any discrepancies in predictive power even with gender grouping in the regression equation and were developed using the same set of independent variables. In clinical practice, the same set of variables in the model can facilitate and expedite the use of equations by healthcare professionals (39), enabling targeted interventions based on the individual needs of PWH.
Given the statistical assumptions adopted in our study, the best anthropometric predictive model was model 6 due to the inclusion of important key variables for the clinical understanding of PWH. Low levels of formal education are associated with the occurrence of NCDs, as poor health knowledge can impact behavioural changes related to prevention actions, adherence to treatment, and adverse outcomes throughout life (40). Anthropometric measurements such as waist circumference, subscapular and abdominal skinfolds (lipohypertrophy in the trunk region), and fat loss (lipoatrophy) in the thigh region are associated with an increased risk of cardiovascular disease (20). About the use of ART, longer exposure times are associated with higher insulin resistance, leading to lipotoxicity and lipodystrophy, which contribute to comorbidities and cardiovascular pathogenesis (41). PWH using ART also have a higher presence of markers of accelerated ageing, such as systemic inflammation, frailty, and cardiovascular risk factors (42). Therefore, in addition to the high accuracy of model 6, we can diagnose lipodystrophy by monitoring body changes in PWH, paying attention to possible abnormalities that can lead to poor outcomes in this population.
A limitation to be considered in our study is the size and geographic location of the sample since PWH were recruited at a university hospital in the city of Ribeirão Preto, which could limit the expansion of the sample to other cities and states. At the same time, the specificity of the sample causes the representativeness to be reduced, as shown in previous studies in Brazil (12), France (16), Portugal (17), Kenya (43), Cameroon (44), and Zambia (45). Another limitation would be the use of DXA, which is not the most accurate method available to assess body fat (46). However, even estimating at the molecular level, the fat mass derived from DXA is widely used in the literature to calculate FMR and, consequently, diagnose lipodystrophy in PWH (16,21,26). Therefore, our use of DXA as a reference is consistent with the evidence available in the scientific literature.
Early diagnosis of lipodystrophy is feasible, as it will allow health professionals to properly treat changes in body composition, preventing some NCDs, especially atherosclerosis (26). With the use of anthropometric models, this diagnosis becomes simplified, as the models are objective, accurate and low cost. As shown in our study, models grouped by sex with high predictive power and low estimation error may be viable alternatives for monitoring PWH. Furthermore, our anthropometric predictive models can be useful for a large number of low- and middle-income countries with limited resources available. For widespread accessibility and dissemination, the diagnosis and monitoring of lipodystrophy utilizing our developed predictive models are available in an Excel® spreadsheet accessible via the following hyperlink: http://posgraduacao.eerp.usp.br/files/Routine_Models_FMR.xlsx.
For future investigations, we suggest validating our models in other countries and performing cross-validation between our models and those previously reported in the literature, to verify their generalizability to other populations of PWH.
Conclusions
The proposed FMR sexless models using anthropometric measurements and health-related factors in PWH have been shown to be valid. The high coefficient of determination and low estimation error, particularly in model 6 with the variables included for analysis, support the validity of our models. Therefore, our predictive models represent an advancement in the study of body composition in PWH, consolidating the use of anthropometry and health-related factors for diagnosing and monitoring lipodystrophy.
Acknowledgments
We thank you all people living with HIV whom attended this study.
Funding: This study was supported by a scholarship from
Footnote
Reporting Checklist: The authors have completed the STROBE reporting checklist. Available at https://atm.amegroups.com/article/view/10.21037/atm-23-1946/rc
Data Sharing Statement: Available at https://atm.amegroups.com/article/view/10.21037/atm-23-1946/dss
Peer Review File: Available at https://atm.amegroups.com/article/view/10.21037/atm-23-1946/prf
Conflicts of Interest: All authors have completed the ICMJE uniform disclosure form (available at https://atm.amegroups.com/article/view/10.21037/atm-23-1946/coif). A.P.d.S. received a scholarship from the Coordenação de Aperfeiçoamento de Pessoal de Nível Superior - Brasil (CAPES) - (Finance Code 001, grant No. 88882.317622/2019-01), and from the Conselho Nacional de Desenvolvimento Científico e Tecnológico (CNPq) (grant No. 201126/2022-2). Additionally, the Coordenação de Aperfeiçoamento de Pessoal de Nível Superior - Brasil (CAPES) will pay the article processing charges for this manuscript. The other authors have no conflicts of interest to declare.
Ethical Statement: The authors are accountable for all aspects of the work in ensuring that questions related to the accuracy or integrity of any part of the work are appropriately investigated and resolved. The research received approval from the Ethics Review Board of the Clinical Hospital at the University of São Paulo, Ribeirão Preto, Brazil, under process number 7082/2011, and was conducted in accordance with the principles outlined in the Declaration of Helsinki (as revised in 2013). All participants provided written informed consent before data collection.
Open Access Statement: This is an Open Access article distributed in accordance with the Creative Commons Attribution-NonCommercial-NoDerivs 4.0 International License (CC BY-NC-ND 4.0), which permits the non-commercial replication and distribution of the article with the strict proviso that no changes or edits are made and the original work is properly cited (including links to both the formal publication through the relevant DOI and the license). See: https://creativecommons.org/licenses/by-nc-nd/4.0/.
References
- WHO. HIV 2023; [cited 2024/Feb/25]. Available online: https://www.who.int/data/gho/data/themes/hiv-aids
- Ministério da Saúde. Department of Diseases, Chronic Conditions and Sexually Transmitted Infections 2022; [cited 2023 16/01]. Available online: https://www.who.int/publications/i/item/9789240053779
- Trickey A, Zhang L, Sabin CA, et al. Life expectancy of people with HIV on long-term antiretroviral therapy in Europe and North America: a cohort study. Lancet Healthy Longev 2022;3:S2. [Crossref]
- Teeraananchai S, Kerr SJ, Amin J, et al. Life expectancy of HIV-positive people after starting combination antiretroviral therapy: a meta-analysis. HIV Med 2017;18:256-66. [Crossref] [PubMed]
- Collini P, Mawson RL. A new era of HIV care for age-associated multimorbidity. Curr Opin Infect Dis 2023;36:9-14. [Crossref] [PubMed]
- Cummins NW. Metabolic Complications of Chronic HIV Infection: A Narrative Review. Pathogens 2022;11:197. [Crossref] [PubMed]
- Hengel RL, Watts NB, Lennox JL. Benign symmetric lipomatosis associated with protease inhibitors. Lancet 1997;350:1596. [Crossref] [PubMed]
- Non LR, Escota GV, Powderly WG. HIV and its relationship to insulin resistance and lipid abnormalities. Transl Res 2017;183:41-56. [Crossref] [PubMed]
- Guaraldi G, Stentarelli C, Zona S, et al. The natural history of HIV-associated lipodystrophy in the changing scenario of HIV infection. HIV Med 2014;15:587-94. [Crossref] [PubMed]
- Carr A, Samaras K, Burton S, et al. A syndrome of peripheral lipodystrophy, hyperlipidaemia and insulin resistance in patients receiving HIV protease inhibitors. AIDS 1998;12:F51-8. [Crossref] [PubMed]
- Osoti A, Temu TM, Kirui N, et al. Metabolic Syndrome Among Antiretroviral Therapy-Naive Versus Experienced HIV-Infected Patients Without Preexisting Cardiometabolic Disorders in Western Kenya. AIDS Patient Care STDS 2018;32:215-22. [Crossref] [PubMed]
- Beraldo RA, Meliscki GC, Silva BR, et al. Anthropometric measures of central adiposity are highly concordant with predictors of cardiovascular disease risk in HIV patients. Am J Clin Nutr 2018;107:883-93. [Crossref] [PubMed]
- Stein JH, Klein MA, Bellehumeur JL, et al. Use of human immunodeficiency virus-1 protease inhibitors is associated with atherogenic lipoprotein changes and endothelial dysfunction. Circulation 2001;104:257-62. [Crossref] [PubMed]
- Mayne ES, George JA. Mortal allies: human immunodeficiency virus and noncommunicable diseases. Curr Opin HIV AIDS 2017;12:148-56. [Crossref] [PubMed]
- Fourman LT, Kileel EM, Hubbard J, et al. Comparison of visceral fat measurement by dual-energy X-ray absorptiometry to computed tomography in HIV and non-HIV. Nutr Diabetes 2019;9:6. [Crossref] [PubMed]
- Bonnet E, Delpierre C, Sommet A, et al. Total body composition by DXA of 241 HIV-negative men and 162 HIV-infected men: proposal of reference values for defining lipodystrophy. J Clin Densitom 2005;8:287-92. [Crossref] [PubMed]
- Freitas P, Santos AC, Carvalho D, et al. Fat mass ratio: an objective tool to define lipodystrophy in hiv-infected patients under antiretroviral therapy. J Clin Densitom 2010;13:197-203. [Crossref] [PubMed]
- Beraldo RA, Vassimon HS, Aragon DC, et al. Proposed ratios and cutoffs for the assessment of lipodystrophy in HIV-seropositive individuals. Eur J Clin Nutr 2015;69:274-8. [Crossref] [PubMed]
- Mialich MS, Dos Santos AP, da Silva BR, et al. Relationship Between Adiposity Indices, Lipodystrophy, and Sarcopenia in HIV-Positive Individuals With and Without Lipodystrophy. J Clin Densitom 2017;20:73-81. [Crossref] [PubMed]
- Alencastro PR, Barcellos NT, Wolff FH, et al. People living with HIV on ART have accurate perception of lipodystrophy signs: a cross-sectional study. BMC Res Notes 2017;10:40. [Crossref] [PubMed]
- Santos APD, Machado DRL, Schwingel A, et al. Anthropometric cutoff points to identify lipodystrophy characteristics in people living with HIV/AIDS: an observational study. Nutr Hosp 2019;36:1315-23. [Crossref] [PubMed]
- Correia IM, Navarro AM, Corrêa Cordeiro JF, et al. Bone Mineral Content Estimation in People Living with HIV: Prediction and Validation of Sex-Specific Anthropometric Models. Int J Environ Res Public Health 2022;19:12336. [Crossref] [PubMed]
- Beraldo RA, Meliscki GC, Silva BR, et al. Comparing the Ability of Anthropometric Indicators in Identifying Metabolic Syndrome in HIV Patients. PLoS One 2016;11:e0149905. [Crossref] [PubMed]
- Farinatti P, Paes L, Harris EA, et al. A Simple Model to Identify Risk of Sarcopenia and Physical Disability in HIV-Infected Patients. J Strength Cond Res 2017;31:2542-51. [Crossref] [PubMed]
- Bicudo Bruno Nogueira A, Abreu JM, Mesquita Villela M, et al. Fat Mass Ratio in Brazilian HIV-infected Patients Under Antiretroviral Therapy and Its Relationship With Anthropometric Measurents. J Clin Densitom 2020;23:623-9. [Crossref] [PubMed]
- Dos Santos AP, Navarro AM, Schwingel A, et al. Lipodystrophy diagnosis in people living with HIV/AIDS: prediction and validation of sex-specific anthropometric models. BMC Public Health 2018;18:806. [Crossref] [PubMed]
- Freitas P, Carvalho D, Souto S, et al. Impact of Lipodystrophy on the prevalence and components of metabolic syndrome in HIV-infected patients. BMC Infect Dis 2011;11:246. [Crossref] [PubMed]
- Han SH, Zhou J, Saghayam S, et al. Prevalence of and risk factors for lipodystrophy among HIV-infected patients receiving combined antiretroviral treatment in the Asia-Pacific region: results from the TREAT Asia HIV Observational Database (TAHOD). Endocr J 2011;58:475-84. [Crossref] [PubMed]
- Cuschieri S. The STROBE guidelines. Saudi J Anaesth 2019;13:S31-4. [Crossref] [PubMed]
- Dell RB, Holleran S, Ramakrishnan R. Sample size determination. ILAR J 2002;43:207-13. [Crossref] [PubMed]
- Lorente Ramos RM, Azpeitia Armán J, Arévalo Galeano N, et al. Dual energy X-ray absorptimetry: fundamentals, methodology, and clinical applications. Radiologia 2012;54:410-23. [Crossref] [PubMed]
- Harrison GG, Buskirk ER, Carter JEL, et al. Skinfold thicknesses and measurement technique. In: Lohman TG, Roche AF, Martorell R, editors. Anthropometric standardization reference manual. Champaign, IL: Human Kinetics Books; 1988:55-70.
- Callaway CW, Chumlea W, Bouchard C, et al. Circumferences. In: Lohman TG, Roche AF, Martorell R, editors. Anthropometric standardization reference manual. Champaign, IL: Human Kinetic Books; 1988:39-54.
- Lee RC, Wang Z, Heo M, et al. Total-body skeletal muscle mass: development and cross-validation of anthropometric prediction models. Am J Clin Nutr 2000;72:796-803. [Crossref] [PubMed]
- Kwak SG, Kim JH. Central limit theorem: the cornerstone of modern statistics. Korean J Anesthesiol 2017;70:144-56. [Crossref] [PubMed]
- Myers RH. Classical and modern regression with applications. Belmont, CA: Duxbury Press; 1990;2:488.
- Bland JM, Altman DG. Statistical methods for assessing agreement between two methods of clinical measurement. Lancet 1986;1:307-10. [Crossref] [PubMed]
- Lohman TG. Advances in Body Composition Assessment. Champaign, IL: Human Kinetics Publishers; 1992.
- Seidell JC, Kahn HS, Williamson DF, et al. Report from a Centers for Disease Control and Prevention Workshop on use of adult anthropometry for public health and primary health care. Am J Clin Nutr 2001;73:123-6. [Crossref] [PubMed]
- Medley A, Kennedy C, O'Reilly K, et al. Effectiveness of peer education interventions for HIV prevention in developing countries: a systematic review and meta-analysis. AIDS Educ Prev 2009;21:181-206. [Crossref] [PubMed]
- Galescu O, Bhangoo A, Ten S. Insulin resistance, lipodystrophy and cardiometabolic syndrome in HIV/AIDS. Rev Endocr Metab Disord 2013;14:133-40. [Crossref] [PubMed]
- Han WM, Apornpong T, Gatechompol S, et al. Association of Phenotypic Aging Marker with comorbidities, frailty and inflammatory markers in people living with HIV. BMC Geriatr 2022;22:1010. [Crossref] [PubMed]
- Saito A, Karama M, Kamiya Y. HIV infection, and overweight and hypertension: a cross-sectional study of HIV-infected adults in Western Kenya. Trop Med Health 2020;48:31. [Crossref] [PubMed]
- Dimala CA, Ngu RC, Kadia BM, et al. Markers of adiposity in HIV/AIDS patients: Agreement between waist circumference, waist-to-hip ratio, waist-to-height ratio and body mass index. PLoS One 2018;13:e0194653. [Crossref] [PubMed]
- Filteau S, Kasonka L, Wells JCK, et al. Anthropometry, body composition, early growth and chronic disease risk factors among Zambian adolescents exposed or not to perinatal maternal HIV. Br J Nutr 2022; Epub ahead of print. [Crossref] [PubMed]
- Kohrt WM. Body composition by DXA: tried and true? Med Sci Sports Exerc 1995;27:1349-53. [Crossref] [PubMed]