Circulating tumour DNA analysis for early detection of lung cancer: a systematic review
Highlight box
Key findings
• Different molecular features of circulating tumour DNA (ctDNA) have been evaluated for early detection of lung cancer. The diagnostic performance metrics of analyses based on the different molecular features were revealed to be variable in the current systematic review. This could be attributed to the difference in the molecular testing approaches, study design and the heterogeneity of cancer and control samples.
What is known and what is new?
• Various molecular features of ctDNA were utilized for lung cancer detection in liquid biopsy. Some studies evaluated a single molecular feature, while others utilized different combinations of molecular features and/or circulating protein biomarkers. Regarding the study design, some studies were designed for detection of lung cancer only, while others targeted for pan-cancer detection.
• The systematic review revealed the diverse study designs and the high heterogeneity of case and control cohorts included in the different studies.
What is the implication, and what should change now?
• The high heterogeneity may affect the direct comparison of diagnostic performance metrics across studies and the generalizability of the studies’ conclusions to routine clinical settings.
Introduction
Lung cancer is characterised by its high incidence and poor overall prognosis. It ranks among the top cancer types in both incidence and mortality in different parts of the world (1,2). Much effort has been devoted to improving the survival outcome of patients with lung cancer, including development of early detection methods. The basis for early detection of lung cancer, similar to other types of cancer, is the better survival noted among early-stage disease. The five-year survival rate for localised lung cancer is about 60%, but only less than 10% for metastatic diseases. Unfortunately, only 40% of lung cancer cases are diagnosed as localised or regional diseases at presentation. This highlights an unmet need for improving early detection of localised diseases (1).
Circulating tumour DNA (ctDNA) refers to DNA released into the circulation/blood from cancer cells and could be used to inform genetic and epigenetic alterations present in the tumour (3). There has been intense research on ctDNA analysis for screening, prognostication and surveillance in cancer diagnostics (4). Specifically for the screening purpose, the minimal invasiveness and simple logistic arrangement of a blood draw required for ctDNA analysis provides a readily available screening biomarker (5). In contrast, for screening of lung cancer, low dose computed tomography (LDCT) is another option but remains underutilised despite evidence suggesting survival benefits through radiological screening among high-risk individuals (6,7). Utility of ctDNA for early detection of lung cancer has been explored in a number of studies, including those which focused on lung cancer only or pan-cancer detection (8,9). Given the highly variable degrees of evidence supporting use and adoption of ctDNA analysis, we have conducted this systematic review to evaluate the available evidence on various methods of ctDNA assessment for screening of lung cancer.
Biology of ctDNA
Before the systematic review analysis, the fundamental concepts of ctDNA (and plasma DNA in general) are to be discussed. Plasma DNA is a mixture of DNA fragments released from different organs or cell types [as a result of apoptosis or necrosis (10)]. Therefore, identifying ctDNA at the background of plasma DNA released from other normal cell and tissue types would pose a challenge on sensitivity, especially for small tumours in the early detection setting when the level of ctDNA is expected to be low (11).
Cancer-associated somatic mutation and aberrant methylation are the two most studied molecular features of ctDNA in cancer diagnostics (12,13) (Figure 1). Detection of somatic mutations in plasma is aimed at cancer-derived mutation. However, there is a lack of common hotspot mutations for virtually all cancer types (including lung cancer). Therefore, multiple targets (over multiple genes) have been included with an attempt to improve the detection sensitivity (8,14). The specificity of somatic mutations detected in plasma DNA as surrogates of tumour-derived DNA is another issue. These somatic mutations may be derived from conditions other than tumour cells, such as clonal hematopoiesis of indeterminate potential (CHIP) (15). CHIP describes the expansion of a subclone of haemopoietic cells which carry leukemogenic mutations. Though associated with an increased risk of hematological malignancies, it is yet to be defined as malignant. It is common among the elderly population with a prevalence of up to 10% among adults older than 70 years old. Cancer-associated aberrant methylation is another frequently explored ctDNA marker. Compared to somatic mutation analysis, methylation profiling of plasma DNA could yield the tissue-of-origin information (16,17), which would largely facilitate the establishment of a blood-based pan-cancer screening test (18,19). Methylation analysis forms the core technology of the now commercially available multi-cancer early detection (MCED) test branded as GalleriTM test by Grail, Inc.
As mentioned, plasma DNA exists as fragments of DNA in the circulation (20,21). The size distribution of plasma DNA exhibits a modal peak of ~160 bp that corresponds to a mononucleosomal size (21). Specifically, ctDNA is suggested to be shorter than its normal counterparts in plasma (21). The realization of non-random fragmentation has fuelled research on the fragmentation biology and the simultaneous exploration of ‘fragmentomics’ markers with diagnostics potentials (Figure 1). These markers are signatures of differential fragmentation of ctDNA and DNA derived from normal cells possibly because of the underlying pathophysiological process. They include fragment size (22,23), fragment ends (24), end motifs (25) and jagged ends (26) and all of them have demonstrated potential clinical utilities in a cancer model.
Analytical methods and pre-analytical considerations
Differentiating ctDNA from non-tumor circulating DNA requires detection of cancer-associated genetic, epigenetic and/or fragmentomic alterations as discussed above. Digital polymerase chain reaction (dPCR) and massively parallel sequencing (MPS), also known as next generation sequencing (NGS), are the most commonly employed methods (27). Adaptations to the techniques enable detection of the properties of ctDNA.
Each of the molecular techniques, dPCR and NGS, has its own advantages and disadvantages. dPCR offers precise and sensitive detection of variants even at low allelic fractions, and its downstream analysis and interpretation are relatively straightforward. However, it relies on prior knowledge of the presence of variants in the tumor, and it is less efficient in detecting multiple variants in a single assay (27,28). These limitations hinder the utility of dPCR in early cancer detection where the presence of analytical targets is unknown. In contrast, NGS enables multiplexing, allowing the simultaneous detection of multiple variants within or across samples. This enables untargeted or semi-targeted detection of multiple variants at a lower average cost. Nonetheless, NGS requires more complex downstream bioinformatic analysis, longer analysis time, and higher upfront instrumental costs (29).
Pre-analytical factors also impose a significant impact on the results. These factors include types of blood tubes used, post-collection handling of specimens and delay which could affect the degree of ex-vivo release of DNA from blood cells into plasma (30). In addition to the above-mentioned factors, method of cfDNA isolation would influence relative abundance of ctDNA within samples, with subsequent impacts on diagnostic performance on the assays (31). We present this article in accordance with the PRISMA reporting checklist (available at https://atm.amegroups.com/article/view/10.21037/atm-23-1572/rc).
Methods
Literature search
We performed a systematic review on publications of PubMed before 1 January 2023. Search terms were “(cell-free DNA (cfDNA) OR circulating tumour DNA (ctDNA)) AND (lung cancer) AND ((screening) OR (detection) OR (diagnosis))”. Only publications in English were reviewed. Titles and abstracts were screened and full-texts were reviewed when applicable. We also screened all referenced articles of the selected studies. Studies which evaluated ctDNA analysis for lung cancer detection in symptomatic/asymptomatic patients with no known previous history of lung cancer (primary but not recurrent cancer) were included. Abstracts were reviewed by the authors independently. Discrepancies were resolved with discussion and reaching a consensus.
Data analysis
Information on the study design and the inclusion and exclusion criteria of the selected studies were reviewed. For lung cancer cases, the cancer stage and histologic subtype information were retrieved. For control cases, the clinical status information including smoking habits, chronic obstructive pulmonary disease (COPD) status and presence of lung nodules were retrieved, if available.
Clinical end points
For evaluation of diagnostic performance, the primary outcome of interest was the ability of ctDNA analysis in differentiating patients with lung cancer from individuals without lung cancer, including the sensitivity, specificity, positive and negative predictive values and area under the curve value in receiver operating characteristics curve analysis. For the majority of diagnostic studies, tissue biopsy served as the reference method for determination of cancer statuses in participants.
Quality assessment
Quality assessment for the identified diagnostic studies was performed with the Quality Assessment of Diagnostic Accuracy Studies-2 tool (32).
Results
Study selection is shown in the Preferred Reporting Items for Systematic Reviews and Meta-Analysis (PRISMA) flowchart in Figure 2. Among the 797 articles identified in the database search, 56 were included in the systematic review. A summary table on the ctDNA properties, study cohort (study size, target population and control group), methodology and findings was provided (Table 1).
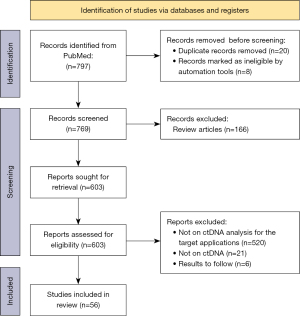
Table 1
PMID | Study (references) | Cancer detection | cfDNA property and other types of biomarkers | Details of cfDNA properties and other biomarkers | Lung cancer patients | Controls | Finding: sen. (sensitivity), spec. (specificity), AUC, etc. |
---|---|---|---|---|---|---|---|
26854716 | Szpechcinski et al., 2016 (33) | Lung cancer | cfDNA concentration and integrity | cfDNA concentration and integrity (difference in concentrations in products from PCR with the 100-bp amplicon vs. the 400-bp amplicon) | N=65: stage I/II/IIIA =30/23/12, histological subtypes (LUAD/LUSC/others) =28/27/10 | Benign lung tumours (N=28); healthy controls (N=16) | NSCLC cases vs. patients with benign pulmonary nodules and healthy individuals |
cfDNA concentration: sen./spec. =86.4%/61.4%, AUC =0.80 | |||||||
cfDNA integrity: sen./spec. =91%/68.2%, AUC not reported | |||||||
29113814 | Leng et al., 2018 (34) | Lung cancer | cfDNA concentration and integrity | cfDNA concentration (ALU115) and integrity (ratio of ALU-115bp to ALU-247bp) | N=106: stage =NA, histological subtypes (NSCLC) =106 | Tuberculosis (N=105); healthy controls (N=107) |
NSCLC vs. healthy controls |
cfDNA concentration (ALU115): sen./spec. =57.5%/89.7%, AUC =0.747 | |||||||
cfDNA integrity: sen./spec. =79.2%/67.3%, AUC =0.759 | |||||||
NSCLC vs. tuberculosis | |||||||
cfDNA concentration (ALU115): sen./spec. =57.5%/64.8%, AUC =0.628 | |||||||
cfDNA integrity: sen./spec. =55.7%/82.9%, AUC =0.722 | |||||||
17449174 | Gautschi et al., 2007 (35) | Lung cancer | cfDNA variants | KRAS gene codon 12 mutation | N=180: stage I/II/III/IV =15/11/63/91, histological subtypes (LUAD/LUSC/large cell carcinoma/undifferentiated) =79/47/36/18 | No control group | Sen. =9% |
27377626 | Fernandez-Cuesta et al., 2016 (36) | Lung cancer | cfDNA variants | TP53 gene mutations | N=51: stage I/II/III/IV =7/7/28/9, histological subtype (SCLC) =51 | Group 1: non-cancer controls (N=123) | SCLC vs. controls in Group 1: sen./spec. = 49%/88.6% |
Independent control group: non-cancer controls (N=102) | SCLC vs. controls in independent control group: spec. =89.2% | ||||||
27018799 | Newman et al., 2016 (37) | Lung cancer | cfDNA variants | 292 predefined somatic mutation hotspots of 29 genes via iDES-enhanced CAPP-seq | N=24 (pretreatment NSCLC): stage IB/IIA/IIB/IIIA/IIIB/IV =3/2/1/4/2/12, histological subtypes (LUAD/NSCLC/LUSC/other/unknown) =16/1/1/1/5 | Healthy controls (N=18) | Sen.: I–II/III/IV =~30%/~50%/~100% (estimated from the manuscript figure); spec. =94.4% |
31069172 | Tailor et al., 2019 (38) | Lung cancer | cfDNA variants | 10 pre-selected mutations | Malignant nodule (N=17): stage I/II/III/IV =8/2/5/2, histological subtypes (LUAD/LUSC/large-cell neuroendocrine carcinoma) =10/6/1 | Benign nodule (N=16) | Sen./spec. =82.4%/100% |
31100334 | Savli et al., 2019 (39) | Pan-cancer | cfDNA variants | Hostspot regions on 11 genes related (ALK, BRAF, EGFR, ERBB2, KRAS, MAP2K1, MET, NRAS, PIK3CA, ROS1, TP53 genes) | N=96: stage (unspecified), histological subtype (unspecified) | No control group | Sen. =84.4% |
31739500 | Peng et al., 2019 (40) | Lung cancer | cfDNA variants | cfDNA copy number variation detection (EGFR, ERBB2, MET genes) | Group 1 (N=48): stage IIIB-IV, histological subtype (NSCLC) | Group 1: healthy controls (N=10) | EFGR amplification: Group 1: sen./spec. =35%/100%; Group 2: sen. = 6.47% |
Group 2 (N=5980): stage (unspecified), histological subtype (NSCLC) | ERBB2 amplification: Group 1: sen./spec. =37.5%/100%; Group 2: sen. =1.56% | ||||||
MET amplification: Group 1: sen./spec. =40%/100%; Group 2: sen. =1.97% | |||||||
32269342 | Chabon et al., 2020 (41) | Lung cancer | cfDNA variants | 255 genes via CAPP-seq Lung Cancer Likelihood in Plasma (Lung-CLiP) model: an ensemble classification framework integrating the outputs of two constituent SNV and CNV models | Training group (N=104): stage IA/IB/IIA/IIB/IIIA/IIIB =21/28/12/16/17/10, histological subtypes (LUAD/LUSC/not specified/large cell carcinoma) =71/23/7/3 | Training group (N=98): risk-matched controls (N=56)/low-risk controls (N=42) | Training group: stage I/II/III sen. (at 0.98 spec.) =0.41/0.54/0.67, stage I/II/III AUC =0.82/0.85/0.87 |
Testing group (N=46): stage IA/IIB/IIA/IIB/IIIA/IIIB =22/10/0/9/2/3, histological subtype (LUAD/LUSC) =36/10 | Testing group (N=48): risk-matched controls | Testing group: sen. (at 0.98 spec.): stage IA/IB/II/III =0.2/0.50/0.30/0.60 (estimated from the manuscript figure); stage I/II/III AUC =0.69/0.71/0.98 | |||||
34217228 | Qvick et al., 2021 (42) | Lung cancer | cfDNA variants | 197 genes (AVENIO ctDNA surveillance kit) | N=60: stage I–IIIa/IIIb–IV =19/41, histological subtype (LUAD/LUSC/SCLC) =37/15/8 | Benign lung disease (N=16) | Significantly more variants detected in lung cancer cases compared to controls |
Significantly more variants were detected in patients with advanced stage disease (stage IIIb–IV) | |||||||
34439258 | Ris et al., 2021 (43) | Pan-cancer | cfDNA variants | 3,062 genomic variants | N=30: stage =NA, histological subtype =NA | Controls without known cancer diagnosis (N=415) | Sen. at 95% spec. =67% |
Sen. at 99% spec. =53% | |||||||
25922721 | Gao et al., 2015 (44) | Lung cancer | cfDNA methylation markers | APC and RASSF1A gene methylation | N=58: stage I =58, histological subtype (LUSC/LUAD/undifferentiated/SCLC) =23/18/15/2 | Benign lung disease (N=31); healthy controls (N=23) | cfDNA RASSF1A and/or APC methylation: lung cancer vs. benign lung disease: sen./spec. =56.9%/90.3%, AUC =0.81 |
26311076 | Powrózek et al., 2016 (45) | Lung cancer | cfDNA methylation markers | DCLK1 gene promoter methylation | N=65: stage (NSCLC): IIA/IIB/IIIA/IIIB/IV =3/4/12/10/17, stage (SCLC): III/IV =9/10; histological subtype (LUAD/LUSC/Large cell carcinoma/SCLC) =22/20/4/19 | Healthy controls (N=95) | Sen./spec.: 49.2%/91.6% |
27485611 | Powrózek et al., 2016 (46) | Lung cancer | cfDNA methylation markers | RTEL1 and PCDHGB6 gene promoter methylation | N=70: stage (NSCLC): I/II/IIIA/IIIB/IV =8/12/10/9/16, stage (SCLC): IIIB or IV =15; histological subtypes (LUAD/LUSC/SCLC) =25/30/15 | Healthy controls (N=80) | Methylation of at least one out of studied genes (RTEL1 and PCDHGB6) |
Sen./spec. =62.9%/90%, AUC =0.755 | |||||||
28855354 | Ooki et al., 2017 (47) | Lung cancer | cfDNA methylation markers | CDO1, HOXA9, AJAP1, PTGDR, UNCX, and MARCH11 genes | N=83: stage I =83, histological subtypes (LUAD/LUSC) =43/40 | Healthy controls (N=42) | Sen.: LUAD/LUSC =72.1%/60%; spec.: 71.4% |
30429608 | Shen et al., 2018 (48) | Pan-cancer | cfDNA methylation markers | Up to 2,100 DMRs selected for model training by cfMeDIP-seq | Training group (N=25): stage (III–IV/unknown =22/3, histological subtype =NA | Training group: healthy controls (N=24) | Training group: all lung cancers vs. healthy controls and other cancers: AUC =0.8 (estimated from the manuscript figure) |
Testing group (N=55): stage (I–II/III–IV =32/23, histological subtype =NA | Testing group: healthy controls (N=62) | Testing group: early-stage LCs (N=32): AUC =0.975; late-stage LCs (N=23): AUC =0.966 | |||||
31436249 | Zhao et al., 2019 (49) | Lung cancer | cfDNA methylation markers | RUNX3 and RASSF1A genes | N=58: stage: malignant small SPN ≤10 mm; histological subtype (unspecified) | Benign SPNs (N=89) | Significantly higher rate of serum RUNX3 and RASSF1A gene methylation in malignant SPNs than that in benign SPNs: 65.5% vs. 12.3%, and 67.2% vs. 10.1%, respectively |
30516882 | Yang et al., 2019 (50) | Lung cancer | cfDNA methylation markers | 8 genes: CDH13, WT1, CDKN2A, HOXA9, PITX2, CALCA, RASSF1A, and DLEC1 | N=39: stage: early stage =39, histological subtypes =NA | Non-lung cancer patients (N=11) | Methylation of any of the 8 genes sen./spec. =72%/91% |
31037156 | Liang et al., 2019 (51) | Lung cancer | cfDNA methylation markers | 9 DMRs were selected after training | Training group (N=40): stage: unspecified, histological subtypes: NA | Training group: benign samples (N=26) | 9 DMRs: training group: AUC =0.839 |
Testing group (N=39): stage IA/IB/IIA/Later stages/unknown =20/7/1/10/1, histological subtypes =NA | Testing group: benign samples (N=27), age and gender matched normal plasma samples (N=118) | Testing group: sen./spec.: 79.9%/85.2%, AUC =0.816 | |||||
Lung cancer cases vs. age and gender matched normal plasma samples (N=118): spec. =93.2% | |||||||
31888670 | Zang et al., 2019 (52) | Lung cancer | cfDNA methylation markers | IDH1, SHOX2 and PTGER4 genes | Training group (N=115): stage =NA, histological subtypes (LUAD/LUSC/SCLC/others) =90/14/9/2 | Training group: healthy controls (N=55) | Training group: sen./spec. =86.1%/67.3%, AUC =0.835 |
Testing group (N=35): stage =NA, histological subtypes (LUAD/LUSC/SCLC/others) =22/5/6/2 | Testing group: Healthy controls (N=16) | Testing group: sen./spec. =80.0%/87.5%, AUC =0.905 | |||||
32138766 | Chen et al., 2020 (53) | Lung cancer | cfDNA methylation markers | CDO1, TAC1, SOX17, HOXA7, HOXA9, GATA4, GATA5, and PAX5 genes | N=163: stage =NA, histological subtype (LUAD/LUSC/NOS) =139/22/2 | Non-cancerous lesions (N=83) | Combination of CDO1, SOX17, and HOXA7 genes methylation: sen./spec. =90%/71%, AUC =0.88 |
31775567 | Vrba et al., 2020 (54) | Lung cancer | cfDNA methylation markers | MIR129-2, LINC01158, CCDC181, PRKCB, TBR1, ZNF781, MARCH11, VWC2, SLC9A3, HOXA7 genes | N=18: stage I/II/III/IV =5/3/2/8, histological subtype (LUAD/LUSC) =15/3 | Healthy controls (N=47) | 10 DNA methylation biomarkers: sen. 83% at 95% spec., AUC =0.956; 5 DNA methylation biomarkers subgroup: AUC =0.97 |
33506766 | Liu et al., 2020 (19) | Pan-cancer | cfDNA methylation markers | 103,456 distinct regions (17.2 Mb in the genome) covering 1,116,720 CpGs | The CCGA study (training group) (N=260): stage (I/II/III/IV =59/23/72/106, histological subtypes: NA | CCGA study (training group): healthy controls (N=1,521) | CCGA study (training group): sen.: I/II/III/IV =~20%/~80%/~87%/~90% (estimated from the manuscript figure); spec. =99.8% |
Testing group (N=111): stage (I/II/III/IV =27/11/31/42, histological subtypes: NA | Testing group: healthy controls (N=610) | Testing group: sen.: I/II/III/IV =~24%/~48%/~82%/~88% (estimated from the manuscript figure); spec. =99.3% | |||||
34176681 | Klein et al., 2021 (18) | Pan-cancer | cfDNA methylation markers | 103,456 distinct regions (17.2 Mb in the genome) covering 1,116,720 CpGs | N=404: stage I/II/III/IV/NA =96/44/118/145/1, histological subtypes: NA | Non-cancer (N=1,254) | Lung cancer (N=404) vs. non-cancer (N=1,254): Sen.: I/II/III/IV/missing =21.9%/79.5%/90.7%/95.2%/100%, spec. =99.5% |
Lung cancer vs. other cancer types: sen.: 91.7% (220/240) | |||||||
34131323 | Liang et al., 2021 (55) | Lung cancer | cfDNA methylation markers | 80,672 CpG sites which were segregated into 8,312 blocks (2,473 blocks used for ctDNA analysis) | Training-testing group (N=140): stage IA/IB/II/III =74/16/33/17, histological subtypes (LUAD/LUSC/others) =109/22/9 | Training-testing group: healthy controls (N=124) | Training-testing group: sen./spec. =69%/96%, AUC =0.93 |
Single-blind testing group (N=168): stage IA/IB/II/III =83/26/33/16, histological subtype (LUAD/LUSC/others) =126/34/8 | Single-blind testing group: healthy controls (N=122)/benign (N=15) | Single-blind testing group: sen./spec. =58%/(normal controls: 97% and benign patients: 93%), AUC =0.90 | |||||
32516173 | Ponomaryova et al., 2021 (56) | Lung cancer | cfDNA concentration and cfDNA methylation markers | cfDNA concentration (LINE-1) and cfDNA methylation markers (LINE-1) | N=23: stage I/II/III =1/5/17, histological subtype (LUSC/LUAD) =13/10 | Healthy controls/COPD/bronchitis =16/15/16 | LINE-1 index of methylation: lung cancer vs. joint control group: sen./spec. =64.3%/94.4%, AUC =0.832 |
34251068 | Qi et al., 2021 (57) | Lung cancer | cfDNA methylation markers | Top 300 DMR identified via cfMeDIP-seq | Lung cancers (tumor size >3 cm, N=32): stage: NA (tumor size >3 cm), histological subtype (LUAD/invasive LUAD/LUSC/SCLC) =18/2/9/3 | Healthy individuals without pulmonary nodules (N=7)/benign pulmonary nodules (N=23) | Lung cancers (tumor size >3 cm, N=32) vs. healthy individuals without pulmonary nodules (N=7): sen./spec. =100%/100% |
Malignant pulmonary nodules detected by CT scan (nodule size <3 cm, N=35): stage: NA; LUAD/adenocarcinoma in situ/Invasive LUAD/micro-invasive LUAD/poor-differential carcinoma/LUSC =9/5/10/7/1/3 | All cases of pulmonary malignant tumors (N=67) vs. controls (N=30): sen./spec. =91.0%/93.3%, AUC =0.963 | ||||||
35450968 | Magenheim et al., 2022 (58) | Lung cancer | cfDNA methylation markers | 17 loci with lung-specific methylation patterns | N=26: stage III–IV, histological subtype: NSCLC NOS/LUAD/poorly differentiated carcinoma/LUAC/SCLC =1/15/1/8/1 | Healthy controls (N=30) | AUC =0.835 |
34830765 | Huang et al., 2021 (59) | Pan-cancer | cfDNA methylation markers | 3 selected DMCGIs | cfMBD-seq group (N=12): stage III–IV =12, histological subtypes: LUAD/Others =9/3 | cfMBD-seq group: non-lung cancer (N=16) | cfMeDIP-seq group (3 DMCGIs): lung cancer vs. non cancer: AUC =0.949 |
cfMeDIP-seq group (N=80): stage I–II/III–IV/unknown =3/22/55, histological subtypes: NA | cfMeDIP-seq group: non-lung cancer (N=86) | ||||||
35126793 | Zhao et al., 2022 (60) | Lung cancer | cfDNA methylation markers | 10-DMR marker panel | CfDNA group1 (MethylationEPIC data) (N=4): stage: unspecified, histological subtype: NSCLC | cfDNA group1 (MethylationEPIC data): healthy controls (N=12); | cfDNA group2 (10-DMR marker panel): sen./spec. =92%/92.3%, AUC =0.922 |
CfDNA group2 (RRBS data) (N=29): stage: unspecified, histological subtype: unspecified | CfDNA group2 (RRBS data): healthy controls (N=74) | ||||||
35538556 | Markou et al., 2022 (61) | Lung cancer | cfDNA methylation markers | 5 selected gene promoters (APC, RASSFIA1, FOXA1, SLFN11, SHOX2 genes) | N=42: stage IA–IIIA, histological subtype (LUAD/LUSC/undfferentiated carcinoma) =14/24/4 | Healthy controls (N=12) | Methylation of at least one gene promoter (APC, RASSFIA1, FOXA1, SLFN11, SHOX2): sen./spec. =33.3%/83.3% |
28820176 | Song et al., 2017 (62) | Pan-cancer | cfDNA 5hmC markers | 2082 differentially hydroxymethylated genes | N=15: stage I–II/III–IV/NA =3/10/2, histological subtypes (NSCLC/SCLC) =14/1 | Healthy controls (N=8) | A progressive global loss of 5hmC enrichment was observed from healthy subjects to early stage non-metastatic lung cancer to late stage metastatic lung cancer |
30010036 | Zhang et al., 2018 (63) | Lung cancer | cfDNA 5hmC markers | cfDNA 5hmC markers (2459 differential 5hmC genes) (7 serum protein markers (CEA, AFP, CA19-9, CA15-3, CA125, NSE, CYFRA21-1) for comparison) | N=66: stage I/II/III/IV/NA =26/17/18/1/4, histological subtypes (LUAD/LUSC/adenosquamous carcinoma) =46/17/3 | Healthy controls (N=67) | Training group (51 tumors and 42 healthy controls): AUC =0.927 |
Testing group (17 lung cancers and 24 healthy controls): AUC =0.96 | |||||||
32694610 | Chen et al., 2020 (64) | Pan-cancer | cfDNA 5hmC markers | 477 cancer-specific DMRs (associated with 657 genes and 10,613 CpG sites) for training | 'Pre-diagnosis' lung cancer patients (cancer diagnosis not yet confirmed at the time of blood collection): N=24 | Healthy controls (N=207) | Pre-diagnosis lung cancer patients: sen. =96% |
'Post-diagnosis' lung cancer patients (cancer diagnosis already confirmed at the time of blood collection): N=28 | Post-diagnosis lung cancer patients: sen. =96%, spec. =96.1% | ||||||
Stage: NA | |||||||
Histological subtypes: NA | |||||||
34689838 | Zhou et al., 2021 (65) | Pan-cancer | cfDNA 5hmC markers | 39 tissue-shared 5hmC-modified lncRNA gene markers | N=66: stage I/II/III/IV/NA:26/17/18/1/4, LUAD/LUSC/adenosquamous carcinoma =46/17/3 | Healthy controls (N=67) | 5hmC-LncRNA diagnostic score (5hLD-score) model based on the 39 markers |
Lung cancer vs. healthy controls: AUC =0.851 | |||||||
35073982 | Hu et al., 2022 (66) | Lung cancer | cfDNA 5hmC markers and cfDNA fragmentomics patterns | 37 5hmC markers and cfDNA fragmentomics patterns | N=157: stage I/II/III/IV/NA: 3/9/49/82/14, histological subtypes: LUAD/LUSC/SCLC/Others =62/48/25/22 | Healthy controls (N=189) | 37 5hmC biomarkers |
Testing group (48 lung cancers vs. 62 healthy controls): sen./spec. =87.50%/83.87%, AUC =0.89 | |||||||
External testing group (66 lung cancers vs. 67 controls from Zhang et al., 2018): sen./spec. =72.7%/80.6%, AUC =0.85 | |||||||
Fragmentation profiles (48-feature fragmentation model) | |||||||
Training group (109 lung cancers vs. 127 healthy controls): sen./spec. =91.74%/93.70%, AUC =0.9837 | |||||||
Testing group (48 lung cancers vs. 62 healthy controls): sen./spec. =87.50%/80.65%, AUC=0.9257 | |||||||
External testing group (66 lung cancers vs. 67 controls from Zhang et al., 2018): sen./spec. =78.79%/76.12%, AUC =0.822 | |||||||
Integration of 5hmC features and fragmentation profiles | |||||||
Training group (109 lung cancers vs. 127 healthy controls): AUC =1 | |||||||
Testing group (48 lung cancers vs. 62 healthy controls): sen./spec. =87.50%/90.30%, AUC =0.9432 | |||||||
External testing group (66 lung cancers vs. 67 controls from Zhang et al., 2018): sen./spec. =83.33%/77.61%, AUC =0.8639 | |||||||
26425700 | Wielscher et al., 2015 (67) | Lung cancer | cfDNA methylation markers and cfDNA concentration | 63 methylation markers and total cfDNA concentration | N=33: stage I&II/III&IV/unknown =9/15/9, histological subtypes (LUAD/LUSC/SCLC/large cell lung cancer) =11/8/7/7 | N=171: healthy control/COPD 0/COPD/ILD =27/34/42/68 | Integrated model using methylation of 63 candidate loci and cfDNA amounts |
Original group: | |||||||
Lung cancer vs. healthy controls: sen./spec. =0.82/0.89, AUC =0.91 | |||||||
Lung cancer vs. non-cancer and healthy controls: sen./spec. =87.8%/90.2% | |||||||
Lung cancer vs. ILD: spec. =88% | |||||||
Lung cancer vs. COPD: spec. =88% | |||||||
27544059 | Weiss et al., 2017 (68) | Lung cancer | cfDNA methylation markers and protein markers | cfDNA methylation markers [SHOX2 and PTGER4] and protein markers (CYFRA 21-1, CEA, SCC and NSE genes) | Training group (N=117): stage I/II/III/IV/unknown =26/21/42/24/4, histological subtype (LUAD/LUSC/other/SCLC) = 46/58/8/5 | Training group: healthy control (N=212) | Methylation of SHOX2 and PTGER4 |
Training group: AUC =0.93 | |||||||
Testing group (N=50): stage I/II/III/IV =12/11/16/11, histological subtype (LUAD/LUSC/other/SCLC) =18/25/7/0 | Testing group: patients with nonmalignant lung disease (N=50)/healthy patients (N=72) | Testing group: | |||||
Lung cancer (N=50) vs. nonmalignant lung disease (N=50) and healthy controls (N=72): AUC =0.88 | |||||||
Lung cancer (N=50) vs. nonmalignant disease (N=50): AUC =0.86 | |||||||
Lung cancer (N=50) vs. healthy controls (N=72): AUC =0.91 | |||||||
Protein panel (CYFRA 21-1, CEA, SCC and NSE) | |||||||
Subgroup of training group (59 lung cancer cases vs. 92 healthy controls): AUC =0.79 | |||||||
33901468 | Park et al., 2021 (69) | Pan-cancer | cfDNA methylation markers, cfDNA concentration and integrity | LINE-1 hypomethylation, Alu cell-free DNA concentration, Alu index | N=64: stage T0/T1/T2/T3 =1/27/31/5, histological subtypes: unspecified | Healthy controls (N=64) | Combination of Alu index, LINE-1 methylation level, and Alu longer and shorter fragments DNA concentrations |
Lung cancer vs. healthy controls: sen./spec. =89.06%/82.81%, AUC =0.895 | |||||||
31142840 | Cristiano et al., 2019 (70) | Pan-cancer | cfDNA fragmentomics patterns | cfDNA fragmentomics patterns (DELFI score) | N=12: stage I/II =3/9, histological subtypes: LUAD/adenosquamous carcinoma/LUSC =9/1/2 | Healthy controls (N=215) | DNA evaluation of fragments for early interception (DELFI) score utilizing fragmentation patterns |
Lung cancer vs. healthy controls: sen./spec. =100%/98%, AUC =1 | |||||||
34417454 | Mathios et al., 2021 (71) | Lung cancer | cfDNA fragmentomics patterns, protein marker and clinical information | cfDNA fragmentomics patterns, CEA, age, smoking history, and presence of COPD | LUCAS group (N=129): stage IA/IB/IIA/IIB/IIIA/IIIB/IIIC/IV =11/4/2/5/17/15/3/72, histological subtypes (LUAD/LUSC/SCLC/adenosquamous/NSCLC, NOS/mixed small cell and NSCLC/mesothelioma/neuroendocrine/metastasis from other organ/unknown) =62/29/11/3/3/1/1/1/15/3 | LUCAS group: non-cancer (N=239, including 158 patients with no prior, baseline, or future cancers); independent testing group: non-cancer (N=385) | DNA evaluation of fragments for early interception (DELFI) score utilizing fragmentation profiles |
LUCAS group: lung cancer (N=129) vs. healthy controls (N=158): AUC =0.90 (stage I/II/III/IV =0.76/0.89/0.92/0.92) | |||||||
Independent testing group (N=46): LUAD/large cell/LUSC/adenosquamous/small cell/mixed small cell and NSCLC =27/9/7/1/1/1 | Independent testing group: lung cancer (N=46) vs. healthy controls (N=358): sen. (I/II/III–IV) =0.57/0.6/1 (estimated from manuscript figure) | ||||||
DELFImulti: multimodal model combining genome-wide cfDNA fragmentation features with CEA levels, age, smoking history, and presence of COPD | |||||||
LUCAS group: overall AUC =0.93 (I/II/III/IV =0.78/0.95/0.94/0.95) | |||||||
DELFImulti approach followed by LDCT: lung cancer (N=129) vs. healthy controls (N=158): spec. =94% at sen. of 80% | |||||||
35690859 | Bao et al., 2022 (72) | Pan-cancer | cfDNA fragmentomics patterns | cfDNA fragmentomics patterns (FSC, FSD, EDM, BPM) and CNV | Training group (N=146): stage I =146, histological subtype: LUAD | Training group: healthy controls (N=122) | Testing group: model using the 5 features sen./spec. =90.4%/95.0%, AUC =0.973 |
Testing group (N=146): stage I =146, histological subtype: LUAD | Testing group: healthy controls (N=121) | ||||||
27555497 | Chen et al., 2016 (73) | Lung cancer | cfDNA variants, clinical features, total cfDNA concentration, and serum protein markers | cfDNA variants [50 genes], cfDNA concentration, clinical features and serum protein markers (CA125, CA19-9, CEA, CYFRA21-1, NSE) | N=58: stage IA/IB/IIA =30/16/12, histological subtype (LUAD/LUSC) =51/7 | Disease-free individuals (N=4) | cfDNA analysis only: Sen. = 89.7% Serum protein marker only: Sen. = 43.1% |
28814544 | Phallen et al., 2017 (74) | Pan-cancer | cfDNA variants and cfDNA concentration | cfDNA variants (58 cancer-related genes) and total cfDNA concentration | N=71: stage I/II/III/IV =29/32/4/6, histological subtype (LUAD/adenosquamous carcinoma/carcinoma/large cell carcinoma/small cell-large cell adenocarcinoma/SCLC/LUSC) =38/4/1/7/1/1/19 | Healthy controls (N=44) | cfDNA mutation (58 cancer related genes) only: sen. (I/II/III/IV): 44.83%/71.88%/75.00%/83.33% |
cfDNA concentration only: significantly higher concentration of cfDNA in plasma from 65 lung cancer patients than healthy individuals | |||||||
29348365 | Cohen et al., 2018 (75) | Pan-cancer | cfDNA variants and circulating protein markers | CancerSEEK combining cfDNA variants and circulating protein markers: 16 genes (NRAS, CTNNB1, PIK3CA, FBXW7, APC, EGFR, BRAF, CDKN2A, PTEN, FGFR2, HRAS, KRAS, AKT1, TP53, PPP2R1A, GNAS genes), 8 protein biomarkers (CA125, CA19-9, CEA, HGF, myeloperoxidase, OPN, prolactin, TIMP-1) | N=104: stage I/II/III =46/27/31, histological subtype: NSCLC (N=103)/SCLC (N=1) | Healthy controls (N=812) | Performance for lung cancer detection (estimated from the manuscript figure): sen. =~58% (at spec. of 99%) |
30981987 | Peng et al., 2019 (76) | Lung cancer | cfDNA variants, protein markers and clinical features | cfDNA variants [65 lung cancer-related genes], 6 protein markers (NSE, CYFRA 21–1, CEA, ProGRP, CA-125 and SCC), clinical features and patient age] | N=136: stage I/II/III/IV =87/29/17/4, histological subtype (LUAD/LUSC/SCLC/others) =100/28/1/7 | Non-lung cancer patients (N=56) | Combination of cfvariants, serum protein markers and patient age: sen./spec. =80%/99% |
32345712 | Lennon et al., 2020 (9) | Pan-cancer | cfDNA variants and circulating protein markers | cfDNA variants and circulating protein markers: 16 genes (AKT1, APC, BRAF, CDKN2A, CTNNB1, EGFR, FBXW7, FGFR2, GNAS, HRAS, KRAS, NRAS, PIK3CA, PPP2R1A, PTEN, TP53 genes); 9 protein biomarkers (AFP, CA125, CA15-3, CA19-9, CEA, HGF, OPN, Prolactin, TIMP-1) | Prospective recruitment of participants with no known history of malignancy (N=9,911) | Non-cancer subjects in the prospective group | Out of 134 screen-positive subjects (defined as either positive for ctDNA or protein biomarkers), 9 lung cancer cases were identified (Stage I/II/III/IV distribution=1/2/2/4) Another 12 lung cancer cases were identified from the group within the same study period Sen. = 42.9% (9/21) Spec. (for detection of all type of cancer) = 98.9% |
33514352 | Chen et al., 2021 (14) | Lung cancer | cfDNA variants, cfDNA methylation markers and serum protein | cfDNA variants: weighted summation allele fractions of all variants cfDNA methylation markers: regional methylation ratio of 54 selected DMRs serum CEA level | N=128: stage 0/IA/IB/II/III/IV =2/54/29/17/19/7, histological subtype (LUAD/LUSC/large cell carcinoma/SCLC) =97/23/3/5 | Benign lung nodule (BLN) patients: (N=94) | Best performance for the combined analysis of cfDNA mutation, methylation and serum CEA: sen. =76.9%, spec. =58.3%, AUC =0.78 |
34258160 | Liu et al., 2021 (8) | Lung cancer | cfDNA variants, cfDNA methylation markers, clinical features and protein cancer biomarkers | cfDNA variants [29 genes]; 8 protein markers (CA125, CA 15‐3, CEA, CYFRA 21‐1, NSE, PROGRP, SCC, and SF) 30 methylation‐correlated blocks; 10 clinical features | Discovery group (N=70): stage Ia/Ib/IIb/IIIa/IIIb =19/41/4/4/2, histological subtype: carcinoid/LUSC/large cell carcinoma/LUAD =1/6/2/3/58 | Discovery group: benign (N=28) | An integrative multianalytical machine learning model based on patient clinical features, cfDNA mutation, cfDNA methylation, and protein cancer biomarkers: |
Independent testing group (N=15): stage Ia/Ib =6/9, histological subtype: LUAD | Independent testing group: benign (N=14) | Discovery group: AUC =0.85 | |||||
Testing group: sen./spec. =80%/85.7%, AUC =0.86 | |||||||
34800919 | Metzenmacher et al., 2022 (77) | Lung cancer | cfDNA variants and methylation markers | cfDNA methylation markers-methylation of a CpG locus (cg03287111) on the GLI2 gene body (variants of KRAS, BRAF, and EGFR have also been analysed) | N=109: stage I–III/IV = 48/61, histological subtype: NSCLC | Healthy controls (N=39) | cfDNA methylation (only) by AUC =0.94 for early-stage cases; AUC =0.96 for late-stage cases |
36379302 | Kim et al., 2022 (78) | Lung cancer | cfDNA methylation markers and cfDNA fragmentomics | 6,243 lung-tumor-specific CpG markers; Short fragment ratio (100–150 bp/151–220 bp); 5′ end-motif profile; | Model training and testing group (N=139): stage I/II/III/IV=19/23/35/62, histological subtype: NSCLC | Healthy controls (N=97) | TOF score which is based on an ensemble learning model using the logistic regression of three features, ctDNA methylation candidate count, end-motif, and short fragment ratio: test group in the model training and testing group: (40 NSCLC vs. 30 healthy): sen./spec.=95%/96.7%, AUC =0.98 |
Independent testing group (N=62): stage: "limited stage"/"extensive stage" =21/36, histological subtype: SCLC | |||||||
36346614 | Wang et al., 2023 (79) | Lung cancer | cfDNA fragmentomics patterns | CNV; FSC; FSD; 6bp EDM; 6bp BPM | Training group (N=113): stage I/II/III/IV =66/26/20/1, histological subtype: LUAD/LUSC =96/17 | Healthy controls: training group (N=113) | Stacked ensemble model using five features (CNV, FSC, FSD, EDM, and BPM): |
Testing group I (N=81): stage I/II/III/IV =46/16/16/3, histological subtype (LUAD/LUSC) =66/15; | Testing group I (N=47) | Testing group I: sen./spec. =91.4%/95.7%, AUC =0.984 | |||||
Testing group II (N=118): stage I/II/III =85/32/1, histological subtype (LUAD) =118 | Testing group II (N=70) | Testing group II: sen./spec. =84.7%/98.6%, AUC =0.987 | |||||
Additional testing group (N=120): stage I/II/III/IV =35/26/28/31, histological subtype (LUAD/LUSC) =105/15 | Additional testing group(N=120) | Additional testing group: sen./spec. =92.5%/94.2%, AUC =0.974 | |||||
35780566 | Guo et al., 2022 (80) | Lung cancer | cfDNA fragmentomics patterns | 6bp BPM | Training group (N=150): stage I, histological subtype: LUAD | Healthy controls: training group (N=115) | Logistic regression model of 6bp breakpoint motifs: |
Internal testing group (N=102): stage I, histological subtype: LUAD | Internal testing group (N=75) | Internal testing group: sen./spec. =98.0%/94.7%, AUC =0.985 | |||||
External testing group (N=40): stage I, histological subtype: LUAD | External testing group (N=40) | External testing group: sen./spec. =92.5%/90.0%, AUC =0.954 | |||||
36175411 | Stackpole et al., 2022 (81) | Pan-cancer | cfDNA methylation markers | 23,748 cancer-specific hypermethylation markers, 28,197 cancer-specific hypomethylation markers, 7,547 tissue-specific hypermethylation markers, 7,212 tissue-specific hypomethylation markers | Lung cancer (N=126), other cancers (N=150); stage I/II/III/IV =33/23/30/40, histological subtype (LUAD/LUSC) =77/49 | Non-cancer (healthy individuals and patients of various noncancer diseases (e.g., cirrhosis, pancreatitis, hepatitis, diabetes, etc.), N=203) | Integrating four marker types (all cancer types (n=217) vs. noncancer (n=191): sen./spec.=80.7%/97.9%, AUC =0.974 |
35804466 | Zhang et al., 2022 (82) | Pan-cancer | cfDNA methylation markers | 173 pre-selected ribosomal DNA CpG sites | Lung cancer (N=29): stage: NA, histological subtype: NA | Healthy controls (N=75) | 173 pre-selected ribosomal DNA CpG sites: AUC =0.84 |
35361996 | Esfahani et al., 2022 (83) | Lung cancer | cfDNA fragmentomics patterns | cfDNA fragmentomics patterns (promoter fragmentation entropy) | Training group (N=67): stage II/III/IV =7/30/30, histological subtype: NSCLC | Noncancer: training group (N=71), testing group (N=23) | Predicted RNA expression by the promoter fragmentation entropy of 144 TSS sites from 117 genes: training group: AUC =0.91, testing group: AUC =0.83 |
Testing group(N=20): histological subtype: NSCLC |
ctDNA, circulating tumour DNA; cfDNA, cell-free DNA; PCR, polymerase chain reaction; AUC, area under the curve; LUAD, lung adenocarcinoma; LUSC, lung squamous cell carcinoma; NSCLC, non-small cell lung carcinoma; NA, not applicable; SCLC, small cell lung carcinoma; DMRs, differentially methylated regions; cfMeDIP, cell-free methylated DNA immunoprecipitation sequencing; SPN, solitary pulmonary nodule; LCs, lung cancers; NOS, not otherwise specified; CpGs, cytosine-guanine sites; CCGA, Circulating Cell-free Genome Atlas; LINE-1, long interspersed nuclear elements-1; DMCGIs, differentially methylated CG islands; CEA, carcinoembryonic antigen; AFP, alpha fetoprotein; CA19-9, carbohydrate antigen 19-9; CYFRA21-1, cytokeratin fragment antigen 21-1; ILD, interstitial lung disease; SCC, squamous cell carcinoma; DELFI, DNA evaluation of fragments for early interception; COPD, chronic obstructive pulmonary disease; LUCAS, lung cancer screening study; FSC, fragmentation size coverage; FSD, fragmentation size distribution; EDM, end motif; BPM, breakpoint motif; CNV, copy number variation; HGF, hepatocyte growth factor; OPN, osteopontin; TIMP-1, tissue Inhibitor of metalloproteinase-1; PROGRP, progastrin-releasing peptide; SF, serum ferritin.
Study design
A case-control study design was adopted in all except one study in the evaluation of the diagnostic performance of ctDNA analysis for detection of lung cancer. In the remaining study, the group prospectively analyzed ctDNA variants and circulating protein biomarkers [by CancerSEEK (75)] for pan-cancer detection including lung cancer (9). Among these case-control studies, the majority included samples of both early- (stage I–II) and advanced- (stage III–IV) stage cancer, except for a few that targeted early-stage (or stage I) cancer only (44,47,72,80). There were 39 studies that were designed for detection of lung cancer only, and 17 studies for pan-cancer early detection. Different histological subtypes of lung cancer had been included in the cancer cohorts. However, the performance of the various ctDNA analyses was not separately reported for the different lung cancer subtypes in most studies (53,68). The control groups used in the studies were also heterogenous, while some studies included ‘healthy’ controls (37,66,70,78) and other studies included individuals with a benign lung nodule or other pulmonary diseases, e.g., COPD (56,67,81). Such comparison with benign lung disease cases could be used to address the utility of ctDNA analysis under certain clinical scenarios, such as management of patients with lung nodules of unknown nature (56,67). Given the variability in case selection (distribution of early versus advanced cases) and the control groups used, it would be difficult to directly compare the diagnostic performances of the different ctDNA assays for lung cancer detection.
ctDNA properties and analytical methods
Various molecular features of cell-free DNA (cfDNA) were utilized to differentiate lung cancer and non-cancer samples. Quantification of cfDNA concentration was employed in 7 studies (33,34,56,67,69,73,74); cfDNA mutation was employed in 17 studies (8,9,14,35-43,73-77); cfDNA methylation in 29 (Table 1); cfDNA hydroxymethylation in 5 (62-66); and cfDNA fragmentation profiles in 8 (66,70-72,78-80,83). Among the selected studies, 17 studies utilised different combinations of the above cfDNA molecular features and/or cfDNA concentration and/or circulating protein biomarkers (8,9,14,33,34,56,63,66-69,73-78).
For detection of cfDNA mutations (8,9,14,35-43,73-77), most studies have targeted multiple genes (usually driver genes) in order to enhance sensitivity for lung cancer detection. In contrast to mutation analysis, methylation analysis of cfDNA offers the advantage to inform the tissue of origin based on the cancer type- and/or tissue-specific methylation profile and therefore favours the development of a pan-cancer early detection test (18). The performance of mutation-based or methylation-based analysis have been evaluated in both types of studies specifically designed for lung cancer and for pan-cancer detection. In general, for both modalities, lower sensitivities were reported for detection of early-stage cancer, which were below 50%. In a large-scale, case-control, pan-cancer detection study based on cfDNA methylation analysis, the sensitivities for stage I and II lung cancer were about 20% and 50%, and those for stage III and IV cancer were over 80%, at a specificity of 99.3% (validation data) (19).
As mentioned, ‘fragmentomics’ analysis of cfDNA is based on the pathophysiologically associated fragmentation patterns of cfDNA and it has been explored for cancer diagnostics (20). Such cfDNA fragmentomics analysis have also been evaluated for lung cancer detection (66,70-72,78-80,83). Cristiano et al. (70) and Mathios et al. (71). have developed a prediction score, known as the DELFI (DNA evaluation of fragments for early interception) score, based on fragmentation size and coverage characteristics in windows throughout the genome, as well as chromosomal arm and mitochondrial DNA copy numbers. Using the DELFI prediction score, they could achieve a sensitivity of about 60% and a specificity of about 80% for detection of early-stage (stage I and II) lung cancer in an independent validation cohort (70,71).
In order to enhance the diagnostic performance, some researchers have explored the potential synergistic effect of combining the analyses of different molecular features of cfDNA, circulating protein biomarkers and clinical features (8,9,14,33,34,56,63,66-69,73-78). In one study, Liu et al. demonstrated that the integrated model which incorporated the analyses of cfDNA mutation, methylation and protein biomarkers achieved the highest diagnostic performance than individual models with a single type of biomarker (8). Similarly, in the prospective liquid biopsy-based pan-cancer screening study, the cancer cases (not only limited to lung cancer) identified were either ctDNA biomarker-positive or protein biomarker-positive except one case (9). This finding might suggest the advantage of a multi-modality testing approach. At the same time, it would also be interesting to study the difference in tumour characteristics (84), if any, between those that are ctDNA biomarker positive versus protein-biomarker positive.
Complementary diagnostic role with low-dose computed tomography (LDCT)
LDCT was proven to be an effective screening tool for lung cancer but remained underutilized (6,7). The clinical role of liquid biopsy to complement LDCT for lung cancer screening might be partly addressed in one recently published study by Mathios et al. (71) and another aforementioned prospective pan-cancer screening study (9). In the prospective pan-cancer screening study, 9,911 asymptomatic participants with no known history of malignancies had received blood testing involving 9 protein biomarkers and cfDNA targeted sequencing for mutation analysis. In addition to the 3 cancer cases picked up by LDCT (unknown total number of participants who underwent LDCT), 9 more lung cancer cases (1 at stage I; 2 at stage II; 2 at stage III and 4 at stage IV) were identified by the blood test (9). In another study by Mathios et al. (71), the researchers have modelled the performance of incorporating ctDNA analysis (based on plasma DNA fragmentation and also clinical information) to select high-risk subjects for subsequent LDCT. These attempts were aimed to improve the low adherence rate of LDCT. All these would suggest the potential complementary role of ctDNA-based (or liquid biopsy-based) test to the standard modality for cancer screening.
Emerging applications
Leveraging the ability of NGS in untargeted, multiplex detection of ctDNA, multi-cancer detection represents a reasonable and promising next step in the development of the technology. For instance, Klein et al. (18) and Liu et al. (19) employed detection of methylation markers in cfDNA for detection and differentiation of tissue of origin, with diagnostic performances comparable to single-cancer detection. This represents an attractive approach which improves cost-effectiveness via simultaneous detection of multiple malignancies.
Discussion
In cancer management, applications of ctDNA analysis such as therapeutic prediction and disease monitoring (in cases with known driver mutations) have already been adopted for routine clinical uses (85-87). However, challenges remain in early detection of lung cancer without common driver mutations, in which defining a tumour-specific genetic/epigenetic alteration as a biomarker would be more costly and less readily available. Ascertaining their clinical utilities necessitates large-scale clinical studies and integration with the diagnostic and/or management flow, which require significant time and resources. Nevertheless, ctDNA analysis could potentially address the unmet clinical need of early detection in lung cancer. This has prompted us to conduct this systematic review to evaluate the performance of the different ctDNA analysis techniques reported for lung cancer detection.
As reviewed in this systematic review, the design of studies is very heterogenous. The high heterogeneity may affect the generalizability of the studies’ conclusions to routine clinical settings. Although the objectives of most studies were aimed to evaluate ctDNA for early detection of lung cancer, the study populations of some studies were usually made up of large proportions of cases at advanced stages, while some studies did not specify the proportion of cases at each clinical stage. Tests tend to be more accurate at differentiating patients at advanced stages from healthy subjects. Performance metrics calculated from a population with the majority having advanced diseases tend to be an over-estimate when applied to screening populations, where a large proportion of subjects are healthy or at early stages of disease.
Various methodologies have been used for detection and quantitation of specific molecular features of ctDNA. cfDNA methylation/hydroxymethylation profiling and mutation detection in a panel of genes represent approaches unlimited to exclusive detection of cancers with specific driver mutations. The differing approaches confer different sensitivities and specificities to the analyses, compromising comparability between studies and generalizability of their conclusions. As the field evolves and the repertoire of analytical techniques expands, complexity in the issue is expected to further increase and further validation across different populations would be required. In addition, ctDNA analysis is highly influenced by pre-analytical conditions as mentioned, including blood tubes, storage conditions, DNA extraction methods etc. Standardization and cross-method comparisons would be required to confirm the performance as reported (88-90).
Similarly, studies have utilized various bioinformatics tools to detect the mutational status of target genes and the methylation status of target genes or genomic regions. In addition, various machine learning models have been built to differentiate lung cancer patients and healthy subjects based on various cfDNA properties. High sensitivity and specificity were reported in some of the studies. However, the majority of these studies had relatively small training cohorts. A machine learning model generated from training groups of a larger scale with external validation would better reflect the performance in separating lung cancer patients from both healthy subjects and subjects with other cancer types.
Studies have also investigated the utility of cfDNA analysis for detection of multiple types of cancers (9,18,19,39,43,48,53,59,62,65,69,70,72,74,75,81,82). While this could represent an approach that is expected to be more cost-effective, further studies are needed to address the manner in which the testing could be integrated to current workflows.
One study limitation is that only one search engine is included for the search of eligible studies, though most studies that analysed the different ctDNA markers should have been covered.
Conclusions
ctDNA demonstrates great potential for early detection of lung cancer. While various analytical methodologies have shown promise for clinical translation, validation in prospective cohorts is necessary to confirm the performance. This would help defining the target screening population and integration into clinical care.
Acknowledgments
Funding: This work was supported by
Footnote
Provenance and Peer Review: This article was commissioned by the Guest Editor (Calvin S. H. Ng) for the series “Lung Cancer Management—The Next Decade” published in Annals of Translational Medicine. The article has undergone external peer review.
Reporting Checklist: The authors have completed the PRISMA reporting checklist. Available at https://atm.amegroups.com/article/view/10.21037/atm-23-1572/rc
Peer Review File: Available at https://atm.amegroups.com/article/view/10.21037/atm-23-1572/prf
Conflicts of Interest: All authors have completed the ICMJE uniform disclosure form (available at https://atm.amegroups.com/article/view/10.21037/atm-23-1572/coif). The series “Lung Cancer Management—The Next Decade” was commissioned by the editorial office without any funding or sponsorship. W.K.J.L. has filed multiple patents on cell-free DNA analyses for cancer diagnostics. W.K.J.L. is a member of the Data Advisory Committee of the Hong Kong Genome Institute. W.K.J.L. is a Board member of DRA and hold equities in Illumina. P.J. holds equities in Grail/Illumina, KingMed Future and Take2. PJ is a consultant to KingMed Future and receives consulting fees. P.J. is Director of DRA, Take2, KingMed Future, and Insighta. P.J. has filed various patents or patent applications related to cell-free DNA and receives royalties from Illumina, Take2, DRA, and KingMed Future. The authors have no other conflicts of interest to declare.
Ethical Statement: The authors are accountable for all aspects of the work in ensuring that questions related to the accuracy or integrity of any part of the work are appropriately investigated and resolved.
Open Access Statement: This is an Open Access article distributed in accordance with the Creative Commons Attribution-NonCommercial-NoDerivs 4.0 International License (CC BY-NC-ND 4.0), which permits the non-commercial replication and distribution of the article with the strict proviso that no changes or edits are made and the original work is properly cited (including links to both the formal publication through the relevant DOI and the license). See: https://creativecommons.org/licenses/by-nc-nd/4.0/.
References
- Surveillance Epidemiology and End Results (SEER) Program (www.seer.cancer.gov) SEER*Stat Database. Cancer Stat Facts: Lung and Bronchus Cancer. SEER. 2020. Available online: https://seer.cancer.gov/statfacts/html/lungb.html. Accessed 03 September 2022.
- de Groot PM, Wu CC, Carter BW, et al. The epidemiology of lung cancer. Transl Lung Cancer Res 2018;7:220-33. [Crossref] [PubMed]
- Wan JCM, Massie C, Garcia-Corbacho J, et al. Liquid biopsies come of age: towards implementation of circulating tumour DNA. Nat Rev Cancer 2017;17:223-38. [Crossref] [PubMed]
- Heitzer E, Haque IS, Roberts CES, et al. Current and future perspectives of liquid biopsies in genomics-driven oncology. Nat Rev Genet 2019;20:71-88. [Crossref] [PubMed]
- Lam WKJ, Chan KCA. Plasma DNA for early cancer detection - opportunities and challenges. Expert Rev Mol Diagn 2019;19:5-7. [Crossref] [PubMed]
- Jonas DE, Reuland DS, Reddy SM, et al. Screening for Lung Cancer With Low-Dose Computed Tomography: Updated Evidence Report and Systematic Review for the US Preventive Services Task Force. JAMA 2021;325:971-87. [Crossref] [PubMed]
- Pham D, Bhandari S, Pinkston C, et al. Lung Cancer Screening Registry Reveals Low-dose CT Screening Remains Heavily Underutilized. Clin Lung Cancer 2020;21:e206-11. [Crossref] [PubMed]
- Liu QX, Zhou D, Han TC, et al. A Noninvasive Multianalytical Approach for Lung Cancer Diagnosis of Patients with Pulmonary Nodules. Adv Sci (Weinh) 2021;8:2100104. [Crossref] [PubMed]
- Lennon AM, Buchanan AH, Kinde I, et al. Feasibility of blood testing combined with PET-CT to screen for cancer and guide intervention. Science 2020;369:eabb9601. [Crossref] [PubMed]
- Thierry AR, El Messaoudi S, Gahan PB, et al. Origins, structures, and functions of circulating DNA in oncology. Cancer Metastasis Rev 2016;35:347-76. [Crossref] [PubMed]
- Bettegowda C, Sausen M, Leary RJ, et al. Detection of circulating tumor DNA in early- and late-stage human malignancies. Sci Transl Med 2014;6:224ra24. [Crossref] [PubMed]
- Keller L, Belloum Y, Wikman H, et al. Clinical relevance of blood-based ctDNA analysis: mutation detection and beyond. Br J Cancer 2021;124:345-58. [Crossref] [PubMed]
- Elazezy M, Joosse SA. Techniques of using circulating tumor DNA as a liquid biopsy component in cancer management. Comput Struct Biotechnol J 2018;16:370-8. [Crossref] [PubMed]
- Chen K, Sun J, Zhao H, et al. Non-invasive lung cancer diagnosis and prognosis based on multi-analyte liquid biopsy. Mol Cancer 2021;20:23. [Crossref] [PubMed]
- Jaiswal S, Fontanillas P, Flannick J, et al. Age-related clonal hematopoiesis associated with adverse outcomes. N Engl J Med 2014;371:2488-98. [Crossref] [PubMed]
- Sun K, Jiang P, Chan KC, et al. Plasma DNA tissue mapping by genome-wide methylation sequencing for noninvasive prenatal, cancer, and transplantation assessments. Proc Natl Acad Sci U S A 2015;112:E5503-12. [Crossref] [PubMed]
- Moss J, Magenheim J, Neiman D, et al. Comprehensive human cell-type methylation atlas reveals origins of circulating cell-free DNA in health and disease. Nat Commun 2018;9:5068. [Crossref] [PubMed]
- Klein EA, Richards D, Cohn A, et al. Clinical validation of a targeted methylation-based multi-cancer early detection test using an independent validation set. Ann Oncol 2021;32:1167-77. [Crossref] [PubMed]
- Liu MC, Oxnard GR, Klein EA, et al. Sensitive and specific multi-cancer detection and localization using methylation signatures in cell-free DNA. Ann Oncol 2020;31:745-59. [Crossref] [PubMed]
- Lo YMD, Han DSC, Jiang P, et al. Epigenetics, fragmentomics, and topology of cell-free DNA in liquid biopsies. Science 2021;372:eaaw3616. [Crossref] [PubMed]
- Lo YM, Chan KC, Sun H, et al. Maternal plasma DNA sequencing reveals the genome-wide genetic and mutational profile of the fetus. Sci Transl Med 2010;2:61ra91. [Crossref] [PubMed]
- Mouliere F, Chandrananda D, Piskorz AM, et al. Enhanced detection of circulating tumor DNA by fragment size analysis. Sci Transl Med 2018;10:eaat4921. [Crossref] [PubMed]
- Jiang P, Chan CW, Chan KC, et al. Lengthening and shortening of plasma DNA in hepatocellular carcinoma patients. Proc Natl Acad Sci U S A 2015;112:E1317-25. [Crossref] [PubMed]
- Jiang P, Sun K, Tong YK, et al. Preferred end coordinates and somatic variants as signatures of circulating tumor DNA associated with hepatocellular carcinoma. Proc Natl Acad Sci U S A 2018;115:E10925-33. [Crossref] [PubMed]
- Jiang P, Sun K, Peng W, et al. Plasma DNA End-Motif Profiling as a Fragmentomic Marker in Cancer, Pregnancy, and Transplantation. Cancer Discov 2020;10:664-73. [Crossref] [PubMed]
- Jiang P, Xie T, Ding SC, et al. Detection and characterization of jagged ends of double-stranded DNA in plasma. Genome Res 2020;30:1144-53. [Crossref] [PubMed]
- Postel M, Roosen A, Laurent-Puig P, et al. Droplet-based digital PCR and next generation sequencing for monitoring circulating tumor DNA: a cancer diagnostic perspective. Expert Rev Mol Diagn 2018;18:7-17. [Crossref] [PubMed]
- Olmedillas-López S, Olivera-Salazar R, García-Arranz M, et al. Current and Emerging Applications of Droplet Digital PCR in Oncology: An Updated Review. Mol Diagn Ther 2022;26:61-87. [Crossref] [PubMed]
- Singh RR. Next-Generation Sequencing in High-Sensitive Detection of Mutations in Tumors: Challenges, Advances, and Applications. J Mol Diagn 2020;22:994-1007. [Crossref] [PubMed]
- Agrawal L, Engel KB, Greytak SR, et al. Understanding preanalytical variables and their effects on clinical biomarkers of oncology and immunotherapy. Semin Cancer Biol 2018;52:26-38. [Crossref] [PubMed]
- Krasic J, Abramovic I, Vrtaric A, et al. Impact of Preanalytical and Analytical Methods on Cell-Free DNA Diagnostics. Front Cell Dev Biol 2021;9:686149. [Crossref] [PubMed]
- Whiting PF, Rutjes AW, Westwood ME, et al. QUADAS-2: a revised tool for the quality assessment of diagnostic accuracy studies. Ann Intern Med 2011;155:529-36. [Crossref] [PubMed]
- Szpechcinski A, Rudzinski P, Kupis W, et al. Plasma cell-free DNA levels and integrity in patients with chest radiological findings: NSCLC versus benign lung nodules. Cancer Lett 2016;374:202-7. [Crossref] [PubMed]
- Leng S, Zheng J, Jin Y, et al. Plasma cell-free DNA level and its integrity as biomarkers to distinguish non-small cell lung cancer from tuberculosis. Clin Chim Acta 2018;477:160-5. [Crossref] [PubMed]
- Gautschi O, Huegli B, Ziegler A, et al. Origin and prognostic value of circulating KRAS mutations in lung cancer patients. Cancer Lett 2007;254:265-73. [Crossref] [PubMed]
- Fernandez-Cuesta L, Perdomo S, Avogbe PH, et al. Identification of Circulating Tumor DNA for the Early Detection of Small-cell Lung Cancer. EBioMedicine 2016;10:117-23. [Crossref] [PubMed]
- Newman AM, Lovejoy AF, Klass DM, et al. Integrated digital error suppression for improved detection of circulating tumor DNA. Nat Biotechnol 2016;34:547-55. [Crossref] [PubMed]
- Tailor TD, Rao X, Campa MJ, et al. Whole Exome Sequencing of Cell-Free DNA for Early Lung Cancer: A Pilot Study to Differentiate Benign From Malignant CT-Detected Pulmonary Lesions. Front Oncol 2019;9:317. [Crossref] [PubMed]
- Savli H, Sertdemir N, Aydin D, et al. TP53, EGFR and PIK3CA gene variations observed as prominent biomarkers in breast and lung cancer by plasma cell-free DNA genomic testing. J Biotechnol 2019;300:87-93. [Crossref] [PubMed]
- Peng H, Lu L, Zhou Z, et al. CNV Detection from Circulating Tumor DNA in Late Stage Non-Small Cell Lung Cancer Patients. Genes (Basel) 2019;10:926. [Crossref] [PubMed]
- Chabon JJ, Hamilton EG, Kurtz DM, et al. Integrating genomic features for non-invasive early lung cancer detection. Nature 2020;580:245-51. [Crossref] [PubMed]
- Qvick A, Stenmark B, Carlsson J, et al. Liquid biopsy as an option for predictive testing and prognosis in patients with lung cancer. Mol Med 2021;27:68. [Crossref] [PubMed]
- Ris F, Hellan M, Douissard J, et al. Blood-Based Multi-Cancer Detection Using a Novel Variant Calling Assay (DEEPGEN(TM)): Early Clinical Results. Cancers (Basel) 2021;13:4104. [Crossref] [PubMed]
- Gao L, Xie E, Yu T, et al. Methylated APC and RASSF1A in multiple specimens contribute to the differential diagnosis of patients with undetermined solitary pulmonary nodules. J Thorac Dis 2015;7:422-32. [PubMed]
- Powrózek T, Krawczyk P, Nicoś M, et al. Methylation of the DCLK1 promoter region in circulating free DNA and its prognostic value in lung cancer patients. Clin Transl Oncol 2016;18:398-404. [Crossref] [PubMed]
- Powrózek T, Krawczyk P, Kuźnar-Kamińska B, et al. Analysis of RTEL1 and PCDHGB6 promoter methylation in circulating-free DNA of lung cancer patients using liquid biopsy: A pilot study. Exp Lung Res 2016;42:307-13. [Crossref] [PubMed]
- Ooki A, Maleki Z, Tsay JJ, et al. A Panel of Novel Detection and Prognostic Methylated DNA Markers in Primary Non-Small Cell Lung Cancer and Serum DNA. Clin Cancer Res 2017;23:7141-52. [Crossref] [PubMed]
- Shen SY, Singhania R, Fehringer G, et al. Sensitive tumour detection and classification using plasma cell-free DNA methylomes. Nature 2018;563:579-83. [Crossref] [PubMed]
- Zhao J, Cui X, Huang X, et al. Methylation of RUNX3 and RASSF1A and the risk of Malignancy in small solitary pulmonary nodules. J Cancer Res Ther 2019;15:899-903. [Crossref] [PubMed]
- Yang Z, Qi W, Sun L, et al. DNA methylation analysis of selected genes for the detection of early-stage lung cancer using circulating cell-free DNA. Adv Clin Exp Med 2019;28:355-60. [Crossref] [PubMed]
- Liang W, Zhao Y, Huang W, et al. Non-invasive diagnosis of early-stage lung cancer using high-throughput targeted DNA methylation sequencing of circulating tumor DNA (ctDNA). Theranostics 2019;9:2056-70. [Crossref] [PubMed]
- Zang R, Wang X, Jin R, et al. Translational value of IDH1 and DNA methylation biomarkers in diagnosing lung cancers: a novel diagnostic panel of stage and histology-specificity. J Transl Med 2019;17:430. [Crossref] [PubMed]
- Chen C, Huang X, Yin W, et al. Ultrasensitive DNA hypermethylation detection using plasma for early detection of NSCLC: a study in Chinese patients with very small nodules. Clin Epigenetics 2020;12:39. [Crossref] [PubMed]
- Vrba L, Oshiro MM, Kim SS, et al. DNA methylation biomarkers discovered in silico detect cancer in liquid biopsies from non-small cell lung cancer patients. Epigenetics 2020;15:419-30. [Crossref] [PubMed]
- Liang N, Li B, Jia Z, et al. Ultrasensitive detection of circulating tumour DNA via deep methylation sequencing aided by machine learning. Nat Biomed Eng 2021;5:586-99. [Crossref] [PubMed]
- Ponomaryova AA, Rykova EY, Azhikina TL, et al. Long interspersed nuclear element-1 methylation status in the circulating DNA from blood of patients with malignant and chronic inflammatory lung diseases. Eur J Cancer Prev 2021;30:127-31. [Crossref] [PubMed]
- Qi J, Hong B, Tao R, et al. Prediction model for malignant pulmonary nodules based on cfMeDIP-seq and machine learning. Cancer Sci 2021;112:3918-23. [Crossref] [PubMed]
- Magenheim J, Rokach A, Peretz A, et al. Universal lung epithelium DNA methylation markers for detection of lung damage in liquid biopsies. Eur Respir J 2022;60:2103056. [Crossref] [PubMed]
- Huang J, Soupir AC, Schlick BD, et al. Cancer Detection and Classification by CpG Island Hypermethylation Signatures in Plasma Cell-Free DNA. Cancers (Basel) 2021;13:5611. [Crossref] [PubMed]
- Zhao H, Zhang H, Xu W, et al. A Sight of the Diagnostic Value of Aberrant Cell-Free DNA Methylation in Lung Cancer. Dis Markers 2022;2022:9619357. [Crossref] [PubMed]
- Markou A, Londra D, Tserpeli V, et al. DNA methylation analysis of tumor suppressor genes in liquid biopsy components of early stage NSCLC: a promising tool for early detection. Clin Epigenetics 2022;14:61. [Crossref] [PubMed]
- Song CX, Yin S, Ma L, et al. 5-Hydroxymethylcytosine signatures in cell-free DNA provide information about tumor types and stages. Cell Res 2017;27:1231-42. [Crossref] [PubMed]
- Zhang J, Han X, Gao C, et al. 5-Hydroxymethylome in Circulating Cell-free DNA as A Potential Biomarker for Non-small-cell Lung Cancer. Genomics Proteomics Bioinformatics 2018;16:187-99. [Crossref] [PubMed]
- Chen X, Gole J, Gore A, et al. Non-invasive early detection of cancer four years before conventional diagnosis using a blood test. Nat Commun 2020;11:3475. [Crossref] [PubMed]
- Zhou M, Hou P, Yan C, et al. Cell-free DNA 5-hydroxymethylcytosine profiles of long non-coding RNA genes enable early detection and progression monitoring of human cancers. Clin Epigenetics 2021;13:197. [Crossref] [PubMed]
- Hu X, Luo K, Shi H, et al. Integrated 5-hydroxymethylcytosine and fragmentation signatures as enhanced biomarkers in lung cancer. Clin Epigenetics 2022;14:15. [Crossref] [PubMed]
- Wielscher M, Vierlinger K, Kegler U, et al. Diagnostic Performance of Plasma DNA Methylation Profiles in Lung Cancer, Pulmonary Fibrosis and COPD. EBioMedicine 2015;2:929-36. [Crossref] [PubMed]
- Weiss G, Schlegel A, Kottwitz D, et al. Validation of the SHOX2/PTGER4 DNA Methylation Marker Panel for Plasma-Based Discrimination between Patients with Malignant and Nonmalignant Lung Disease. J Thorac Oncol 2017;12:77-84. [Crossref] [PubMed]
- Park MK, Lee JC, Lee JW, et al. Alu cell-free DNA concentration, Alu index, and LINE-1 hypomethylation as a cancer predictor. Clin Biochem 2021;94:67-73. [Crossref] [PubMed]
- Cristiano S, Leal A, Phallen J, et al. Genome-wide cell-free DNA fragmentation in patients with cancer. Nature 2019;570:385-9. [Crossref] [PubMed]
- Mathios D, Johansen JS, Cristiano S, et al. Detection and characterization of lung cancer using cell-free DNA fragmentomes. Nat Commun 2021;12:5060. [Crossref] [PubMed]
- Bao H, Wang Z, Ma X, et al. Letter to the Editor: An ultra-sensitive assay using cell-free DNA fragmentomics for multi-cancer early detection. Mol Cancer 2022;21:129.
- Chen KZ, Lou F, Yang F, et al. Circulating Tumor DNA Detection in Early-Stage Non-Small Cell Lung Cancer Patients by Targeted Sequencing. Sci Rep 2016;6:31985. [Crossref] [PubMed]
- Phallen J, Sausen M, Adleff V, et al. Direct detection of early-stage cancers using circulating tumor DNA. Sci Transl Med 2017;9:eaan2415. [Crossref] [PubMed]
- Cohen JD, Li L, Wang Y, et al. Detection and localization of surgically resectable cancers with a multi-analyte blood test. Science 2018;359:926-30. [Crossref] [PubMed]
- Peng M, Xie Y, Li X, et al. Resectable lung lesions malignancy assessment and cancer detection by ultra-deep sequencing of targeted gene mutations in plasma cell-free DNA. J Med Genet 2019;56:647-53. [Crossref] [PubMed]
- Metzenmacher M, Hegedüs B, Forster J, et al. Combined multimodal ctDNA analysis and radiological imaging for tumor surveillance in Non-small cell lung cancer. Transl Oncol 2022;15:101279. [Crossref] [PubMed]
- Kim YJ, Jeon H, Jeon S, et al. A method for early diagnosis of lung cancer from tumor originated DNA fragments using plasma cfDNA methylome and fragmentome profiles. Mol Cell Probes 2022;66:101873. [Crossref] [PubMed]
- Wang S, Meng F, Li M, et al. Multidimensional Cell-Free DNA Fragmentomic Assay for Detection of Early-Stage Lung Cancer. Am J Respir Crit Care Med 2023;207:1203-13. [Crossref] [PubMed]
- Guo W, Chen X, Liu R, et al. Sensitive detection of stage I lung adenocarcinoma using plasma cell-free DNA breakpoint motif profiling. EBioMedicine 2022;81:104131. [Crossref] [PubMed]
- Stackpole ML, Zeng W, Li S, et al. Cost-effective methylome sequencing of cell-free DNA for accurately detecting and locating cancer. Nat Commun 2022;13:5566. [Crossref] [PubMed]
- Zhang X, Jia X, Zhong B, et al. Evaluating methylation of human ribosomal DNA at each CpG site reveals its utility for cancer detection using cell-free DNA. Brief Bioinform 2022;23:bbac278. [Crossref] [PubMed]
- Esfahani MS, Hamilton EG, Mehrmohamadi M, et al. Inferring gene expression from cell-free DNA fragmentation profiles. Nat Biotechnol 2022;40:585-97. [Crossref] [PubMed]
- Lo YMD, Lam WKJ. Towards multi-cancer screening using liquid biopsies. Nat Rev Clin Oncol 2020;17:525-6. [Crossref] [PubMed]
- Wang H, Zhou F, Qiao M, et al. The Role of Circulating Tumor DNA in Advanced Non-Small Cell Lung Cancer Patients Treated With Immune Checkpoint Inhibitors: A Systematic Review and Meta-Analysis. Front Oncol 2021;11:671874. [Crossref] [PubMed]
- Ricciuti B, Jones G, Severgnini M, et al. Early plasma circulating tumor DNA (ctDNA) changes predict response to first-line pembrolizumab-based therapy in non-small cell lung cancer (NSCLC). J Immunother Cancer 2021;9:e001504. [Crossref] [PubMed]
- Mok TS, Wu Y-L, Ahn M-J, et al. Osimertinib or Platinum-Pemetrexed in EGFR T790M-Positive Lung Cancer. N Engl J Med 2017;376:629-40. [Crossref] [PubMed]
- Nikolaev S, Lemmens L, Koessler T, et al. Circulating tumoral DNA: Preanalytical validation and quality control in a diagnostic laboratory. Anal Biochem 2018;542:34-9. [Crossref] [PubMed]
- Meddeb R, Pisareva E, Thierry AR. Guidelines for the Preanalytical Conditions for Analyzing Circulating Cell-Free DNA. Clin Chem 2019;65:623-33. [Crossref] [PubMed]
- Merker JD, Oxnard GR, Compton C, et al. Circulating Tumor DNA Analysis in Patients With Cancer: American Society of Clinical Oncology and College of American Pathologists Joint Review. J Clin Oncol 2018;36:1631-41. [Crossref] [PubMed]