Circulating microRNA biomarkers for cardiovascular risk prediction: are we approaching clinical application?
Patients at high risk of cardiovascular morbidity and mortality are still difficult to identify. Unfortunately, the most widely used standard risk prediction models predict cardiovascular disease (CVD) rather poorly, since these risk calculators are mostly driven by age. Therefore, most young subjects are regarded as low risk merely based on their age, despite sometimes quite obvious adverse risk factors. Circulating biomarkers that can help to improve the identification of individuals at risk are therefore highly needed.
MicroRNAs are short noncoding RNAs that post-transcriptionally regulate gene expression (1). When microRNAs are shed into the circulation, they remain there in a stable state and reflect ongoing processes at a cellular level in the tissues (2,3). Because of these properties, circulating microRNAs have become increasingly popular over the years.
After many small, exploratory studies with divergent results, presently, also larger studies on circulating microRNAs are being published. In a recent study, Karakas et al. (4), assessed the prognostic value of 8 circulating microRNAs that had previously been identified to facilitate the diagnosis of unstable angina pectoris (UAP) (5) in a cohort of 430 acute coronary syndrome (ACS) patients and 682 stable angina pectoris (SAP) patients. The authors concluded that 7 of these 8 measured microRNAs are strongly predictive of cardiovascular mortality with areas under the receiver-operating curves (AUCs) up to 0.76; this represents an important step forward in the circulating microRNA field. However, these studies still struggle with many common pitfalls in microRNA measurement and interpretation of the results remains challenging.
Pitfalls in the measurement of circulating microRNAs and poor reporting on reverse transcription quantitative polymerase chain reaction (RT-qPCR) methods
Reverse transcription quantitative polymerase chain reaction (RT-qPCR) is the most common way to reliably assess microRNA levels. However, the way in which RT-qPCR measurements are performed and analyzed can have a large influence on the results. It is therefore of great importance to have standardized and validated methods for RT-qPCR microRNA measurement. The authors of the study of Karakas et al. (4) thoroughly report on statistical methods, but do not report on the details of the RT-qPCR measurement nor the handling of missing data. It is important to describe the RT-qPCR methods following the MIQE guidelines (6) and to describe how missing data was handled. Differences herein can lead to serious flaws and biases in the results, as explained more in detail below.
Handling missing data and low values
MicroRNAs often circulate in a very low concentration. Even highly sensitive methods like RT-qPCR, need to be stretched to their limit of detection and sometimes certain microRNAs are even totally absent from the circulation. This most often results in missing values that complicate the measurement of microRNAs, since microRNAs can also appear to be missing due to a technical error.
It is most important to distinguish missing values due to a low concentration or complete absence of the microRNA from the circulation, from values that are missing due to a technical error. Missing data due to a low concentration is not missing at random and therefore should be substituted with a low value, whereas missing data due to technical errors occur at random and must be imputed.
The authors of Karakas et al. (4) acknowledge the issues related to a low microRNA concentration, since they substitute Ct values ≥40 with a ∆Ct of 40. Unfortunately, by doing so, they substitute the low microRNA concentrations with an unrealistically low value. We will explain this in detail. In the study, the formula Ct (microRNA) − Ct (cel-miR-39) was used to calculate the ∆Ct. To produce a reliable qPCR curve based on enough data points to calculate a Ct from, a minimum Ct value of 15 is needed. Therefore, the maximum ∆Ct that can be calculated is [(maximum Ct of the microRNA =39.99) − (minimum Ct cel-miR-39 =15)] =24.99. However, in case of a Ct value of ≥40, the authors set the ∆Ct to 40, creating a large gap between the lowest value measurable (∆Ct of 24.99) and the substituted low value. This value will contribute tremendously to the mean expression level compared to more realistic substitution values such as a ∆Ct of 26 (Figure 1A). If not accounted for, these unrealistic values can influence the results of cox or logistic regression analyses.
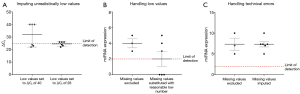
Concerning missing values, the authors do not report whether they encountered this and how this was handled. A missing value can occur because the Ct is 0, meaning that the microRNA was under the detection limit, which also represents a low concentration. Therefore, Ct values of 0 should also be imputed with a reasonably low number, instead of simply excluding them, which could lead to false higher expression levels (Figure 1B).
A missing value can also occur due to a technical error. By excluding these data, the study will lose power and meaningful differences can be lost as illustrated in Figure 1C. The best way to handle this type of missing data is by a multiple imputation method, in which a weighted average is imputed, taking into account the characteristics of the study population. Failure to handle this issue, might lead to either under- or overestimation of the effect.
Single versus duplicate or triplicate RT-qPCR measurements
Another major issue concerning the reliability of RT-qPCR measurements of microRNA data is that often single measurements per sample are performed, where duplicate or even triplicate measurements would be more appropriate. Using measurements in duplicate or triplicate highly increases the precision of the microRNA measurements. Besides, replicate measurements are essential to distinguish missing data due to the technical errors from missing data because of low expression, as explained above.
If a single microRNA measurement shows a Ct value of 0, one would falsely decide that the microRNA is not detectable within the sample, whereas if the measurement would have been done in triplicates, the other two Ct values might have had values of for instance 32. In this particular example one would conclude, that the first measurement has a technical error and that the true value is the average of the other two measurements. On the other hand, when all three triplicates have a Ct of 0, it is most likely that this microRNA cannot be measured due to low expression. Unfortunately, the authors do not report whether their RT-qPCR measurements were single or multiple measurements.
Normalization methods
As the authors state in the discussion, another potential source of bias is the normalization with cel-miR-39. Since cel-miR-39 is not incorporated in microvesicles or protein or lipid complexes, variations in extraction of microRNAs from these vesicles and complexes are not accounted for (7). Therefore, we recently proposed a normalization methods using a panel of endogenous microRNAs, best representing stability of the data and taking in to account technical failures during the RT-qPCR measurement (8). We therefore suggest using these specific panels for specific samples.
Interpretation of the study results
The authors of the paper by Karakas et al. (4) nicely show that circulating microRNAs predict cardiovascular death in coronary artery disease. This is one of the first papers addressing such an important issue in such a large population. Besides, the observed association of 7 of the 8 microRNAs related to cardiovascular mortality, is fairly strong. On the other hand, because the authors do not report on the number of death and the data handling as discussed above, the observed results might be slightly overestimated. Remarkably, this important observation is mainly present in the ACS group, since when analyzing the overall group, the observed association is slightly attenuated.
The authors speculate on the pathophysiological process behind these microRNAs and state that they could be hypoxia markers. On the other hand, it appears from Table 2 in the paper, that the expression level of all the eight microRNAs in ACS are actually lower than in SAP (higher ∆Ct = lower expression level). Therefore, it seems that this intriguing suggestion cannot directly be support by these data. The authors show another intriguing observation, namely, that the association is influenced by vessel disease and left ventricular ejection fraction (LVEF). When the authors correct their data for the number of diseased vessels and LVEF, the association becomes stronger, meaning that this association is mainly present in individuals with a lower atherosclerotic burden and/or a preserved ejection fraction. This suggests that these microRNAs might reflect another underlying pathophysiological mechanism strongly related to cardiovascular death. Taken together, Karakas et al. importantly advance this field by showing predictive power well beyond currently used risk scores, and suggest that the circulating microRNAs measured are related to cardiovascular death. Obviously, more detailed analysis of the technical details of their RT-qPCR measurement may improve signal to noise ratio and further facilitate the use of these biomarkers.
Relation to other microRNAs studies
In this study, microRNAs that were previously associated with UAP were identified (5). Although only small studies have compared UAP with controls (9-11), none of these markers that were identified in the study of Zeller et al. (5), have been found previously. Additionally, since UAP is atherosclerosis without substantial ischemia, some overlap with previously found markers for SAP in earlier studies would have been expected. However, none of the eight microRNAs that were measured in the study of Karakas et al. (4) were found in a total of 17 earlier studies on SAP markers (12). Although, the study of Zeller et al. (5) is the first to consistently show an association of microRNAs with UAP in three independent cohorts, we maintain to wonder why so many different study of microRNA biomarkers produce different results. This might be a cause of the many pitfalls of microRNA measurement, the lack of standard methods for measurements and normalization or handling of missing data. On the other hand the study of Karakas et al. (4) remains one of the largest and most thoroughly performed studies in the field of circulating microRNAs. Moreover, they found highly interesting markers for coronary artery disease risk prediction, despite the raised issues concerning data handling. However, subsequent external validation with more accurate measurements are needed to assess if these circulating microRNA biomarkers could be of value in clinical practice.
Acknowledgements
None.
Footnote
Conflicts of Interest: The authors have no conflicts of interest to declare.
References
- Bartel DP. MicroRNAs: genomics, biogenesis, mechanism, and function. Cell 2004;116:281-97. [Crossref] [PubMed]
- Gupta SK, Bang C, Thum T. Circulating microRNAs as biomarkers and potential paracrine mediators of cardiovascular disease. Circ Cardiovasc Genet 2010;3:484-8. [Crossref] [PubMed]
- Mitchell PS, Parkin RK, Kroh EM, et al. Circulating microRNAs as stable blood-based markers for cancer detection. Proc Natl Acad Sci U S A 2008;105:10513-8. [Crossref] [PubMed]
- Karakas M, Schulte C, Appelbaum S, et al. Circulating microRNAs strongly predict cardiovascular death in patients with coronary artery disease-results from the large AtheroGene study. Eur Heart J 2016. [Epub ahead of print]. [Crossref] [PubMed]
- Zeller T, Keller T, Ojeda F, et al. Assessment of microRNAs in patients with unstable angina pectoris. Eur Heart J 2014;35:2106-14. [Crossref] [PubMed]
- Bustin SA, Benes V, Garson JA, et al. The MIQE guidelines: minimum information for publication of quantitative real-time PCR experiments. Clin Chem 2009;55:611-22. [Crossref] [PubMed]
- Zampetaki A, Mayr M. Analytical challenges and technical limitations in assessing circulating miRNAs. Thromb Haemost 2012;108:592-8. [Crossref] [PubMed]
- Kok MG, Halliani A, Moerland PD, et al. Normalization panels for the reliable quantification of circulating microRNAs by RT-qPCR. FASEB J 2015;29:3853-62. [Crossref] [PubMed]
- Adachi T, Nakanishi M, Otsuka Y, et al. Plasma microRNA 499 as a biomarker of acute myocardial infarction. Clin Chem 2010;56:1183-5. [Crossref] [PubMed]
- D'Alessandra Y, Carena MC, Spazzafumo L, et al. Diagnostic potential of plasmatic MicroRNA signatures in stable and unstable angina. PLoS One 2013;8:e80345. [Crossref] [PubMed]
- Guo M, Mao X, Ji Q, et al. miR-146a in PBMCs modulates Th1 function in patients with acute coronary syndrome. Immunol Cell Biol 2010;88:555-64. [Crossref] [PubMed]
- Romaine SP, Tomaszewski M, Condorelli G, et al. MicroRNAs in cardiovascular disease: an introduction for clinicians. Heart 2015;101:921-8. [Crossref] [PubMed]