How to improve quality of research in intensive care medicine
There are several ways to improve quality of research in the domain of intensive care medicine, such as considering the baseline standard of care; having a preceding phase introducing a minimum standard of care before the start of a trial, in particular when standard of care is too low; and having a clear distinction between implementation studies and efficacy trials. Trialists could further try to increase prognostic as well as predictive enrichment to reduce sample sizes while increasing effect sizes; trialists could also consider biological phenotyping to better identify target populations. Furthermore, to increase the robustness of statistical findings, trials should have sufficiently high numbers of patients, while having the lowest possible loss to follow up. These approaches are detailed in this commentary.
Standard of care
Baseline levels of care are an important determinant for the a priori probability to show benefits of a novel intervention. With high standards of care incremental improvements in mortality will be harder to establish. This has been implicated in the failure of several large conformational trials to show beneficial effects of, e.g., activated protein C in septic shock (1). At the other end of the spectrum, if basic best practices of care are not implemented, as can be encountered in resource-poor settings, beneficial interventions may fail to show any effect. A preceding phase for introduction of a minimum standard of care might then be warranted. An alternative is to introduce an intervention as part of a bundle, to address related management issues. For instance, a trial on low tidal volume ventilation might fail to show an effect if there is not a weaning strategy and sedation protocol in place, unnecessarily prolonging the period of mechanical ventilation. However, trialing a bundle of interventions risks variable implementation of distinct components of the bundle. When failing to show an overall beneficial effect, it will be difficult to attribute the relative contributions of the different components of the bundle to the overall effect (2).
Distinguishing design issues of implementation, effectiveness and efficacy trials is important. Implementation trials focus on methods of implementation, with rates of adoption as main outcome, and often require a cluster-randomized design. Effectiveness and efficacy trials aim for complete implementation, try to reduce threats to causal inference, have a patient-centered primary outcome and use preferably individual randomization. To increase generalizability, it is important to consider the setting of the study and ensure that the trial approaches the everyday context. Hybrid designs have been proposed to address barriers to rapid translation of efficacy trials into clinical practice (3,4).
Prognostic and predictive enrichment
Most clinical trials in critically ill patients select patients based on a syndrome definition of disorder, such as “sepsis” or “acute respiratory distress syndrome” (ARDS). Studies on promising pharmacological interventions in these populations have been exclusively “negative” (5,6). Precision medicine is an approach to treatment based on the patients’ individual traits, which could improve the design of clinical trials in three ways. First, the required sample size can be decreased when patients with more severe disease are selected because the primary endpoint is more prevalent; this is called prognostic enrichment (7). Second, syndromes like “sepsis” and “ARDS” are not defined by a singular underlying pathophysiological mechanism while therapies are used blindly as if the population is homogeneous. There is a strong logical argument for using, e.g., “anti-interleukin 6 therapy” only in patients with an up-regulated response of this pathway; this is also called predictive enrichment (7). This approach reduces the number of patients that are treated without effect and only experience side-effects and increases the number of patients that are treated effectively and thus leads to a net increase in effect size. Third, clustering of patients based on clinical and biological data may facilitate the identification of so-called endophenotypes or subphenotypes that could respond differently to treatment. This novel but very promising approach facilitates prognostic and predictive enrichment through the discovery of distinct subpopulations. Two recent post-hoc analyses of two large randomized controlled trials (RCTs) in ARDS patients (8,9) showed that a subgroup of ARDS patients is characterized by more severe inflammation, shock, and metabolic acidosis and a higher mortality (10,11): high positive end-expiratory pressure (PEEP) and a conservative fluid management reduced mortality in this endophenotype (Figure 1A) (10,11).
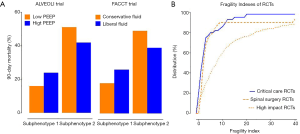
Robustness of trial results
The concept of P values evaluating RCT results is increasingly criticized. The “fragility index” (FI), the number of “non-events” that must be changed to “events” in order for the P value to equal or exceed 0.05, has been proposed as a complement to P values. A small FI means less robust results, in particular when the number of patients lost to follow-up exceeds the FI: outcomes for these patients could have changed the results from “significant” to “non-significant”. Investigators systematically reviewed the literature for high-impact journal RCTs (12), spine surgery RCTs (13), and recently also critical care multicenter RCTs to calculate their FI (Figure 1B) (14). Critical care RCTs appear to perform equally bad as spinal surgery RCTs, and worse than high-impact journal RCTs, though they had the smallest numbers of lost patients. Not surprisingly, the FI correlates positively with the number of RCT participants, and RCTs that report a P value closer to 0.05 have a lower FI.
Critical care RCTs typically are designed based on unrealistic treatment effect sizes, meaning that the power calculation suggests sufficient power with relative small numbers of patients. Consequently, they have an at times staggering low FI, and such “fragile” RCTs are more likely to change the P value-defined outcome if repeated.
Acknowledgements
None.
Footnote
Conflicts of Interest: The authors have no conflicts of interest to declare.
References
- Ranieri VM, Thompson BT, Barie PS, et al. Drotrecogin alfa (activated) in adults with septic shock. N Engl J Med 2012;366:2055-64. [Crossref] [PubMed]
- Writing Group for the CHECKLIST-ICU Investigators and the Brazilian Research in Intensive Care Network (BRICNet), Cavalcanti AB, Bozza FA, et al. Effect of a Quality Improvement Intervention With Daily Round Checklists, Goal Setting, and Clinician Prompting on Mortality of Critically Ill Patients: A Randomized Clinical Trial. JAMA 2016;315:1480-90. [Crossref] [PubMed]
- Curran GM, Bauer M, Mittman B, et al. Effectiveness-implementation hybrid designs: combining elements of clinical effectiveness and implementation research to enhance public health impact. Med Care 2012;50:217-26. [Crossref] [PubMed]
- Glasgow RE, Lichtenstein E, Marcus AC. Why don't we see more translation of health promotion research to practice? Rethinking the efficacy-to-effectiveness transition. Am J Public Health 2003;93:1261-7. [Crossref] [PubMed]
- Boyle AJ, Mac Sweeney R, McAuley DF. Pharmacological treatments in ARDS; a state-of-the-art update. BMC Med 2013;11:166. [Crossref] [PubMed]
- Marshall JC. Why have clinical trials in sepsis failed? Trends Mol Med 2014;20:195-203. [Crossref] [PubMed]
- Prescott HC, Calfee CS, Thompson BT, et al. Toward Smarter Lumping and Smarter Splitting: Rethinking Strategies for Sepsis and Acute Respiratory Distress Syndrome Clinical Trial Design. Am J Respir Crit Care Med 2016;194:147-55. [Crossref] [PubMed]
- Brower RG, Lanken PN, MacIntyre N, et al. Higher versus lower positive end-expiratory pressures in patients with the acute respiratory distress syndrome. N Engl J Med 2004;351:327-36. [Crossref] [PubMed]
- National Heart, Lung, and Blood Institute Acute Respiratory Distress Syndrome (ARDS) Clinical Trials Network, Wiedemann HP, Wheeler AP, et al. Comparison of two fluid-management strategies in acute lung injury. N Engl J Med 2006;354:2564-75.
- Calfee CS, Delucchi K, Parsons PE, et al. Subphenotypes in acute respiratory distress syndrome: latent class analysis of data from two randomised controlled trials. Lancet Respir Med 2014;2:611-20. [Crossref] [PubMed]
- Famous KR, Delucchi K, Ware LB, et al. ARDS Subphenotypes Respond Differently to Randomized Fluid Management Strategy. Am J Respir Crit Care Med 2017;195:331-8. [PubMed]
- Walsh M, Srinathan SK, McAuley DF, et al. The statistical significance of randomized controlled trial results is frequently fragile: a case for a Fragility Index. J Clin Epidemiol 2014;67:622-8. [Crossref] [PubMed]
- Evaniew N, Files C, Smith C, et al. The fragility of statistically significant findings from randomized trials in spine surgery: a systematic survey. Spine J 2015;15:2188-97. [Crossref] [PubMed]
- Ridgeon EE, Young PJ, Bellomo R, et al. The Fragility Index in Multicenter Randomized Controlled Critical Care Trials. Crit Care Med 2016;44:1278-84. [Crossref] [PubMed]