Exploration of high-risk factors for pulmonary embolism in patients undergoing postoperative anti-thrombotic therapy among gynecologic oncology surgery: a retrospective study
Introduction
Gynecologic oncology patients may develop deep venous thrombosis (DVT) and severe pulmonary embolism (PE) after surgery. About 37.9% of patients with postoperative gynecologic cancer have DVT (1). The risk of DVT in gynecologic patients undergoing surgery ranges from 7% to 45%, and in 1% of these patients, fatal PE may occur (2).
According to the National Comprehensive Cancer Network (NCCN) (3) Guidelines for Cancer-Associated Venous Thromboembolic Disease and the European Guidelines on perioperative venous thromboembolism (VTE) prophylaxis (4), patients with gynecological surgery should be treated with thromboprophylaxis. However, despite sufficient prophylactic anticoagulation therapy, there are still patients developing PE. Such cases have been confirmed in orthopedic diseases; however, few related reports on gynecology exist.
In our clinical practice, our department found that even if patients with a Caprini Score (5) of 3 or more undergo postoperative thromboprophylaxis such as low molecular weight heparin (LMWH) injections and other mechanical methods of prophylaxis, patients still experience a symptomatic PE. Also, the number of these patients increased from the previous year. Most patients did not pass gas/have flatulence within three days after surgery. Getting out of bed after surgery was not always easy for these patients, and patients with pulmonary disease are often bedridden for 3 or more days (20–26%) (6). Parvizi et al. reported that 80% of PE occurred within three days of surgery (7). At this time, these patients had discomfort such as flatulence and pain, which may have resulted in chest tightness, tachycardia, and shortness of breath. These symptoms are usually more obvious than the pain or tenderness in the patient’s legs and are easily confused with the symptoms of patients with PE. Symptoms may be more easily identified if a patient has a PE or a lower extremity deep vein thrombosis after flatulence. It is more important to find high-risk patients within three days after surgery. In order to determine the risk factors for patients who still have PE within three days after surgery—although those patients received thromboprophylaxis immediately after surgery—we conducted a retrospective single-center study. These patients underwent pulmonary computed tomography angiography (CTA) examination for suspicious PE after surgery.
Methods
Ethics statement
This study was approved by the Ethics Committee of Fujian Cancer Hospital (approval number: YKT2018-012-01), and complied with the Declaration of Helsinki.
Patient population
This is a retrospective study. The records of patients undergoing gynecologic oncology surgery for benign or malignant tumors at the Fujian Cancer Hospital were retrieved from the hospital information system, between April 3t, 2013, and January 12, 2018. PE was confirmed by the patients’ symptoms combined with pulmonary CTA within three days after surgery. These patients sometimes showed only chest tightness, abnormal breathing, and abnormal heart rhythms.
Inclusion criteria
We included patients in this study if they satisfied the following criteria: (I) patients undergoing postoperative thromboprophylaxis such as antithrombotic drug injection, early mobilization, compression stockings, and intermittent pneumatic compression (IPC); (II) within three days after the operation, pulmonary CTA examination was performed because of chest tightness, chest pain, dyspnea, no obvious cause of rapid heart rate, or syncope; (III) the Caprini score was 3 or more; (IV) patients underwent surgery in our department were diagnosed with benign or malignant tumors before surgery.
Exclusion criteria
We excluded patients if they meet at least one of the following criteria: (I) previously used oral contraceptives or hormone replacement therapy; (II) patients had preoperative varicose veins in the lower limb, lower extremity edema, or upper or lower limb thrombosis; (III) patients did not undergo venous ultrasonography to exclude thrombosis of the lower extremities before surgery; (IV) patients had cerebral infarction, PE, or atherosclerosis before surgery; (V) patients also underwent gastrointestinal surgery during hospitalization, or eventually gave up treatment; (VI) patients did not undergo gynecological pelvic surgery, such as a vulvar surgery.
Thromboprophylaxis treatment
All patients wore graduated compression stockings (GCS) before entering the operating room. Stockings were re-applied postoperatively before leaving the hospital. All patients received VTE prophylaxis with intermittent pneumatic compression (IPC) devices from the first postoperative day.
Due to concerns about the risk of postoperative bleeding, no patients received treatment with preoperative chemical prophylaxis (CP). All thromboprophylaxis drugs were used on the first postoperative day. Among the 35 patients who used postoperative antithrombotic drugs, 18 patients were LMWH, 15 were treated with dalteparin sodium, and 2 patients were treated with argatroban.
LMWH was subcutaneously injected in the abdomen (4,250 IU once daily). Dalteparin sodium was subcutaneously injected in the abdomen at a dose of 200 IU/kg of body weight once daily. Argatroban was injected intravenously at an initial infusion rate of 0.5 mg/kg/minute.
Variables included in analysis
The end-point event was a PE within 3 days after surgery. A total of 28 variables were included for analysis and are listed in Table 1.
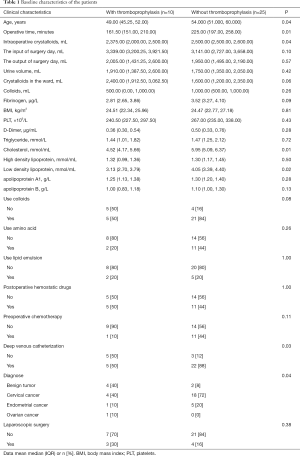
Full table
The variables of fibrinogen, D-Dimer, triglyceride, total cholesterol, high-density lipoprotein, low-density lipoprotein (LDL), apolipoprotein A1, and apolipoprotein B refer to preoperative examination results.
Statistical analysis
Data were analyzed with the use of the R statistical software (version 3.4.1; The R Project for Statistical Computing, Institute for Statistics and Mathematics, R Core Team, version 3.4.1).
Shapiro-Wilk’s test was performed to assess the normality of the data. Clinical characteristics were summarized by site using the median [interquartile range (IQR)] or percentage (composition ratio), as appropriate. The Kruskal-Wallis test was used to compare the medians among the groups of data. Fisher’s exact test was used to detect group differences using frequency (count) data.
We used the three commonly used methods for feature selection to select the high-risk factors for thromboembolism.
Method 1: a method based on the random forest classifier (8) to identify features which are in some circumstances relevant, i.e., all-relevant features; implemented in the R package, “Boruta”.
Method 2: variable selection using logistic regression and penalized likelihood ratio test (9,10), implemented in the R package, “logistf”. Compared to the ordinary maximum likelihood estimation in logistic regression, this method solves the separation problem by penalizing the likelihood with Jeffreys prior and therefore is suitable for the small and sparse datasets in our study.
Method 3: a method based on Elastic Net (11), implemented in R package, “GLMnet” where the Elastic Net addresses the problem of over-regularization by balancing between LASSO and ridge penalties.
Model selection criteria like the Bayesian Information Criterion (BIC) (12) and the Akaike Information Criterion (AIC) (13) were often used to choose between models. However, models selected with BIC or AIC may not be practical for clinicians. To compare the three predictive models with identified high-risk factors and to assess the clinical usefulness of these models, we used decision curve analysis (DCA) proposed by Vickers et al. (14,15). DCA is a method for evaluating and comparing prediction models that incorporate clinical consequences, requires only the data set on which the models are tested, and can be applied to models that have either continuous or dichotomous results. The decision curve is generated by plotting the net benefit (NB) against the threshold probability of a disease or event, where NB is defined as the true-positive prediction rate subtracted by the false-positive rate with a weight of the relative harm of a false-positive and a false-negative result. DCA is shown to have advantages over other commonly used measures and techniques.
We used a clinical impact plot (16) to show the estimated number of cases that would be associated with high risk for each risk threshold, visually showing the proportion of those who are cases of true positives. P values of <0.05 were considered significant statistically.
Results
A total of 67 patients underwent a pulmonary CTA examination in the last 5 years. A final screening of 35 patients out of 67 patients was analyzed according to the aforementioned criteria (Figure 1). Eventually, 35 patients (from April 3, 2013, to January 12, 2018) were included in the analysis according to the inclusion criteria. There were 10 patients in the PE group and 25 patients in non-PE group. The detailed data for these characteristics is summarized in . Among the 35 patients, 10 had PE, 25 had no PE, and 1 died because of PE. The measurement data did not satisfy the normality and were expressed by the median and IQR. Homogeneity testing was carried out for the count data. Levene’s test showed that variances were unequal across the groups.
As shown in Table 1, the baseline levels of both the thrombotic and non-thrombotic patients show differences in age, operative time, intraoperative rehydration, total cholesterol levels, LDL levels deep, venous catheterization, and diagnose. However, the statistical methods we used were based on the logistic regression method (17), a mixture of the lasso and ridge regression (18), and the Boruta method (8,19); all methods were tested for bias correction.
To achieve the best prediction for the high-risk factors, we tested several prediction methods (Boruta, logistf, and GLMnet). We used the Boruta package to select the variables and risk factors and screened out age, operative time, fibrinogen, crystalloids in the ward, total cholesterol, LDL, and deep venous catheterization (Figure 2). The risk factors screened out by the logistf package were colloids and total cholesterol. Risk factors identified by using a machine learning approach (“GLMnet” package) included intraoperative crystalloids, operative time, colloids, fibrinogen, crystalloids in the ward, total cholesterol, LDL, preoperative chemotherapy, and deep venous catheterization. The high-risk factor included in all three models is total cholesterol. The high-risk factors included in the two models are operative time, fibrinogen, crystalloids in the ward, LDL, deep venous catheterization, and colloids. We use the Venn diagram and UpSet diagram (20) to show the intersection of high-risk factors in different models (Figure 3).
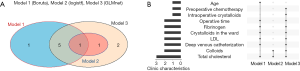
A comparison of the differences was performed using the AIC; the AIC values of the Boruta model, logistf model, and the elastic network model were 41.516, 33.997, and 44.170, respectively. It was revealed that the logistf model was superior. However, we paid high attention to the evaluation and comparison prediction models in these clinical scenarios; thus we used DCA to assess the differences in the clinical benefits between the mentioned models.
The differences in the clinical benefits of the three models using DCA discriminant models are shown in Figure 4A. The gray-thin line in the graph represents that all patients having PE (all); The horizontal line with the vertical axis of 0 is the net benefit trend for patients without PE. The net benefit trends of the Boruta model, the logistf model, and the elastic network model are mod1, mod2, and mod3, respectively. DCA showed that model 3 might result in superior net benefits between thresholds of 0.10 and 0.50 for prediction of postoperative PE. Meanwhile, the prediction performances of the different methods were similar, with model 3 (elastic network model) marginally outperforming the others. Figure 4B,C,D shows the clinical impact curve for the risk model. Of 1,000 patients, the heavy red solid line shows the total number who would be deemed high risk for each risk threshold. The thin red line shows a 95% confidence interval (CI). The blue dashed line shows how many of those would be true positives (cases). The blue thin dashed line shows 95% CI. The graph shows an estimated high-risk value for each risk threshold and shows the proportion of the true positives. For example, if a 20% risk threshold was used, then out of 1,000 patients screened, about 600 would be deemed high-risk patients in model 1, >600 patients would be deemed so in model 2, and <600 patients would be deemed so in model 3. About 400 of these true PE cases were found in all three models. Model 3 may be superior.
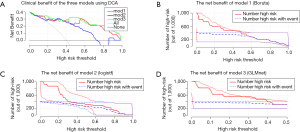
Discussion
The main purpose of this study was to investigate the risk factors for thromboembolism in gynecologic cancer patients who had undergone routine postoperative thromboprophylaxis. We proposed three models to find the high-risk factors. The high-risk factor included in all three models is the total cholesterol. The high-risk factors included in the two models are fibrinogen, LDL, operation time, deep venous catheterization, crystalloids in the ward, and colloids. PE may be persistent among the patients with these factors even with prophylactic anticoagulants. We suppose that the patients with these high-risk factors may require direct antithrombotic therapy or more active therapy after surgery.
Multivariate models can handle large numbers of covariates (confounders). Our study uses exactly three of them. When there is a baseline imbalance between two groups, the regression model in effect adjusts the outcome of the values to account for the differences in the baseline covariate (21). Therefore, the individual variables in Table 1 are not balanced and do not affect the final analysis results.
There are numerous statistical methods used for finding high-risk factors, and the results of these methods may be different. At the same time, the prognostic model with the clinical benefit is also different. Therefore, we assumed that the common high-risk factors derived from different statistical methods would be the most high-risk factors. We also analyzed the clinical utility of different models and evaluated the clinical applicability of the risk factors derived from these models.
Although the logistf model has the lowest AIC value, its clinical effect may not be as good as the other two models based on DCA results. The presence of high-risk factors in multiple models deserves our attention.
Serum lipids may contribute to the development of DVT. Vayá et al. (22) conducted a case-control study and found that in 143 DVT patients, idiopathic DVT patients had higher cholesterol levels than secondary patients. Mi et al. found in their systematic review that total cholesterol was significantly higher in VTE patients (23). Lipoproteins are macromolecular complexes that are essential for the transport of cholesterol and triglycerides in the blood. As one of the members, LDL is utilized to deliver dietary and liver triglycerides and cholesterol to peripheral tissues. Very low-density lipoproteins (VLDL) and LDL increase platelet cholesterol levels and stimulate platelet activation (24). Analysis of human VLDL and LDL proteins revealed that 25% of the protein’s active functional pathways are associated with coagulation and hemostasis in both granules. Platelet aggregation requires the participation of fibrinogen (25). IIb 3 binds fibrinogen and other ligands by fibrinogen-dependent pathways that lead to platelet aggregation (26).
Operative time and deep venous catheterization are the risk factors as determined by Caprini (5) and The American College of Obstetricians and Gynecologists (ACOG) Practice Bulletin No. 84 (27). According to the Caprini score, central venous access presents 1-point, operative time >60 minutes presents 2-point and >180 minutes presents 5-point. When the score was 3 or more, the patient was at high risk, and VTE was observed >0.97% among these patients.
In addition to intraoperative fluid replacement, postoperative rehydration in the ward is also very important, and insufficient rehydration leads to blood concentration, resulting in an increased risk of thrombosis. The safe use of starch in the perioperative period has been questioned. No evidence using colloidal resuscitation reduces the risk of death, and the use of hydroxyethyl starch may increase mortality (28). In our study, the postoperative colloidal fluid infusion was also found to be a high-risk factor. Some patients may have lower blood pressure after surgery. Thus, the colloid is added, probably because of the increase in colloid osmotic pressure leading to blood concentration.
D-dimer was not included in high-risk factors, probably because these patients were abnormal in D-dimer; therefore, they were ignored by the risk model. Patients with these high-risk factors may need more proactive methods in preventing thrombosis or early intervention before surgery. Reasonable treatments require randomized clinical trials or more research to be conducted. It is noteworthy that the surgical bases of rehydration and supplementation of colloidal fluids have also become a high-risk factor, prompting us to standardize the rehydration methods for patients.
There were some limitations to this study. Firstly, this study used pulmonary CTA as a method for diagnosing PE. PE diagnosed by pulmonary CTA may be overdiagnosed. The rate of positive diagnosis of PE on pulmonary CTA varies from 14% to 22% (29). However, this technique is mature in our hospital, and it is more appropriate for disease judgment and timely clinical treatment in emergencies.
Our hospital did not previously screen patients with hereditary thrombophilia. Therefore, the lack of identification of patients with thrombophilia is a defect in this study. Due to the low proportion of Chinese women who smoke, this factor was not collected in the medical history review.
This study aimed to identify possible high-risk factors. A real-world study is required to verify these factors. Meanwhile, even among those high-risk factors were verified, it is not still clear what the effect of each factor on the patients is. Due this remaining uncertainty, the mechanism of adopting a more effective treatment to prevent thrombosis still requires further research.
Acknowledgements
We thank Dr. Lijing Lin (Faculty of Biology, Medicine and Health, The University of Manchester) for useful discussions on the manuscript. We also thank MedSci Company for polishing the early draft of the paper.
Funding: This study was supported by Fujian Provincial Health Technology Project (No: 2017-CX-9) and the Science and Technology Program of Fujian Province, China (No: 2018Y2003).
Footnote
Conflicts of Interest: The authors have no conflicts of interest to declare.
Ethical Statement: This study was approved by the Ethics Committee of Fujian Cancer Hospital (approval number: YKT2018-012-01). This article is a retrospective study, so informed consent was been waived.
References
- Crandon AJ, Koutts J. Incidence of post-operative deep vein thrombosis in gynaecological oncology. Aust N Z J Obstet Gynaecol 1983;23:216-9. [Crossref] [PubMed]
- Oates-Whitehead RM, D’Angelo A, Mol B. Anticoagulant and aspirin prophylaxis for preventing thromboembolism after major gynaecological surgery. Cochrane Database Syst Rev 2003.CD003679. [PubMed]
- Streiff MB, Holmstrom B, Ashrani A, et al. Clinical Practice Guidelines in Oncology: Cancer-Associated Venous Thromboembolic Disease. Available online: http://excellence.acforum.org/Resource-Center/resource_files/1119-2017-12-13-101157.pdf
- Surgery and fatal pulmonary embolism. JAMA 2014;311:1163. [Crossref] [PubMed]
- Caprini JA. Risk assessment as a guide to thrombosis prophylaxis. Curr Opin Pulm Med 2010;16:448-52. [Crossref] [PubMed]
- Masuyama H, Nakamura K, Nobumoto E, et al. Inhibition of pregnane X receptor pathway contributes to the cell growth inhibition and apoptosis of anticancer agents in ovarian cancer cells. Int J Oncol 2016;49:1211-20. [Crossref] [PubMed]
- Parvizi J, Huang R, Raphael IJ, et al. Timing of Symptomatic Pulmonary Embolism with Warfarin Following Arthroplasty. J Arthroplasty 2015;30:1050-3. [Crossref] [PubMed]
- Kursa MB, Rudnicki WR. Feature selection with the Boruta package. J Stat Softw 2010;36:1-13. [Crossref]
- Heinze G, Schemper M.. A solution to the problem of separation in logistic regression. Stat Med 2002;21:2409-19. [Crossref] [PubMed]
- Heinze G, Ploner M, Beyea J. Confidence intervals after multiple imputation: combining profle likelihood information from logistic regressions. Stat Med 2013;32:5062-76. [Crossref] [PubMed]
- Friedman J, Hastie T, Tibshirani R. Regularization paths for generalized linear models via coordinate descent. J Stat Softw 2010;33:1-22. [Crossref] [PubMed]
- Schwarz G.. Estimating the Dimension of a Model. Ann Stat 1978;6:461-4. [Crossref]
- Akaike H.. A New Look at the Statistical Model Identification. IEEE Trans Automat Contr 1974;AC-19:716-23. [Crossref]
- Vickers AJ, Elkin EB. Decision curve analysis: a novel method for evaluating prediction models. Med Decis Making 2006;26:565-74. [Crossref] [PubMed]
- Vickers AJ, Cronin AM, Elkin EB, et al. Extensions to decision curve analysis, a novel method for evaluating diagnostic tests, prediction models and molecular markers. BMC Med Inform Decis Mak 2008;8:53. [Crossref] [PubMed]
- Kerr KF, Brown MD, Zhu K, et al. Assessing the Clinical Impact of Risk Prediction Models With Decision Curves: Guidance for Correct Interpretation and Appropriate Use. J Clin Oncol 2016;34:2534-40. [Crossref] [PubMed]
- Puhr R, Heinze G, Nold M, et al. Firth’s logistic regression with rare events: accurate effect estimates and predictions? Stat Med 2017;36:2302-17. [PubMed]
- Chung NC, Storey JD. Statistical significance of variables driving systematic variation in high-dimensional data. Bioinformatics 2015;31:545-54. [Crossref] [PubMed]
- Kursa MB. Robustness of Random Forest-based gene selection methods. BMC Bioinformatics 2014;15:8. [Crossref] [PubMed]
- Lex A, Gehlenborg N, Strobelt H, et al. UpSet: Visualization of Intersecting Sets. IEEE Trans Vis Comput Graph 2014;20:1983-92. [Crossref] [PubMed]
- Carlsson MO, Zou KH, Yu CR, et al. A comparison of nonparametric and parametric methods to adjust for baseline measures. Contemp Clin Trials 2014;37:225-33. [Crossref] [PubMed]
- Vayá A, Mira Y, Ferrando F, et al. Hyperlipidaemia and venous thromboembolism in patients lacking thrombophilic risk factors. Br J Haematol 2002;118:255-9. [Crossref] [PubMed]
- Mi Y, Yan S, Lu Y, et al. Venous thromboembolism has the same risk factors as atherosclerosis: A PRISMA-compliant systemic review and meta-analysis. Medicine (Baltimore) 2016;95:e4495. [Crossref] [PubMed]
- Ouweneel AB, Van Eck M. Lipoproteins as modulators of atherothrombosis: From endothelial function to primary and secondary coagulation. Vascul Pharmacol 2016;82:1-10. [Crossref] [PubMed]
- Yang H, Reheman A, Chen P, et al. Fibrinogen and von Willebrand factor-independent platelet aggregation in vitro and in vivo. J Thromb Haemost 2006;4:2230-7. [Crossref] [PubMed]
- Hou Y, Carrim N, Wang Y, et al. Platelets in hemostasis and thrombosis: Novel mechanisms of fibrinogen-independent platelet aggregation and fibronectin-mediated protein wave of hemostasis. J Biomed Res 2015.29. [PubMed]
- Committee on Practice Bulletins--Gynecology, American College of Obstetricians and Gynecologists. ACOG Practice Bulletin No. 84: Prevention of deep vein thrombosis and pulmonary embolism. Obstet Gynecol 2007;110:429-40. [Crossref] [PubMed]
- Perel P, Roberts I, Ker K. Colloids versus crystalloids for fluid resuscitation in critically ill patients. Cochrane Database Syst Rev 2013.CD000567. [PubMed]
- Hutchinson BD, Navin P, Marom EM, et al. Overdiagnosis of Pulmonary Embolism by Pulmonary CT Angiography. AJR Am J Roentgenol 2015;205:271-7. [Crossref] [PubMed]