Apple Watch, Wearables, and Heart Rhythm: where do we stand?
Introduction
Atrial fibrillation (AF) poses a major health concern in the United States by affecting over 5 million people (1). It is associated with significant mortality and morbidity by increasing the risk of stroke 5-fold compared to general population (2). In the United States, AF accounts for at least 15% to 25% of strokes (3). It can be asymptomatic or subclinical with its first presentation being stroke in 18%, and AF being newly detected at the time of stroke (4). Furthermore, the prevalence of AF increases with age, being 65 or older places the prevalence around 4.4% with people who have previously undiagnosed AF making 1.4% of it (5). Estimates of people in the United States who may have previously unknown, asymptomatic AF are around 700,000, with an estimated cost burden of 3.2 billion dollars (6,7).
With evidence of subclinical AF associated with increased risk of ischemic stroke (8), previously only being detected by ambulatory electrocardiogram (EKG) monitoring either with temporary patches for short periods of time or implantable recorders for longer periods, there might be a place for wearable technology, which is typically used on a regular basis, in aiding diagnosis of subclinical AF. As the popularity of smart watches increases, with more than 325 million devices sold in 2016 alone (9) and 13% of the US population owning smartwatches, with 40% of the public showing interest in getting one (10), these devices are becoming a commodity rapidly finding its way on everyone’s wrist and in pockets. The use of these wearables needs to be effective in diagnosing subclinical AF, with false positives increasing health costs by unwarranted ER visits or anxiety to the patient, or with false negatives missing the diagnosis which may lead to morbidity or mortality.
Recent developments indeed point towards wearables, especially smart watches, being quite effective and being a novel method for screening for silent AF in the general population. Though the technology is not only restricted to smart watches, single lead EKGs that are portable and phone applications that detect heart rate can serve as event recorders during an episode of symptoms like palpitations, but may be restricted by not providing continuous monitoring.
Discussion
In this review, we will discuss the technology behind the wearables and its efficacy. Further implementation of this technology in different formats will also be addressed.
Photoplethysmography (PPG)
PPG is the measure of the volumetric change of the heart by measuring light transmission or reflection. During systole, the rise in pressure forces a forward pulse into the blood vessel. The device shines light on the skin to detect a pulse by measuring the difference in the quantity of light reflected back to the sensor. The sensor is usually placed on the skin in the areas where the artery is proximal to the skin. Thus, the heart rate is measured using an algorithm. PPG technology can be used in physical sensors, smart watches, or even phone apps to measure heart rate.
PPG which is deemed as medical grade is accurate at measuring heart rate in sinus rhythm (correlation coefficient of 0.96) (11). However, PPG is limited by it underestimation of heart rate during sinus tachycardia and accuracy suffers during physical activity. On testing consumer grade PPG technology (Apple watch, Fitbit, etc.), its accuracy was also high in sinus rhythm (12). A small study analyzing PPG data from smart watches with a deep neural network to detect AF compared to a standard 12 lead EKG yielded a sensitivity of 98.0% and specificity of 90.2% (13). This proves the data obtained by PPG technology in the wearables when analyzed by algorithms can be effective in diagnosing AF.
Implementation of the PPG technology through certain apps in phones can also be used to measure heart rate. With 77% of the US population owning a smartphone (14), this can be implemented as a measure to detect irregular heart rate in general population. Cardiio Rhythm app for I-phone implemented the phone’s camera as a light sensor to obtain heart rate measurements. An outpatient study involving 1,013 participants with hypertension, diabetes mellitus, and/or aged ≥65 years, revealed that the app was able to detect Atrial fibrillation with a sensitivity of 92.9% (95% CI: 77–99%), specificity of 97.7% (95% CI: 97–99%), with a positive predictive value (PPV) of 53.1% (95% CI: 38–67%), and a negative predictive value (NPV) of 99.8% (95% CI: 99–100%) (15). Although this app cannot get continuous heart rate data from the person, random heart rate checks can uncover subclinical AF or even during symptoms palpitations act like an event recorder to pick up an episode of AF.
Apple watch study
The Apple watch study was a prospective, single arm, open label study with 419,297 participants conducted with a goal of evaluating the ability of irregular pulse detection by the Apple watch and subsequent irregular pulse notification algorithm to identify AF (16). The primary endpoint was AF >30 seconds on EKG patch and simultaneous AFib on EKG patch and tachogram. The heart rate is recorded as PPG waveforms by the Apple watch during periods of minimal arm movement. Tachograms, which are heart rate over a period of time, are created. If a tachogram detects irregular heart rate, then the algorithm is triggered to get 4 confirmatory tachograms with irregular pulse rates during minimal arm movements. After 4 confirmatory tachograms, 5 in total including the initial irregular tachogram, the participant is alerted through a phone notification. The notification encourages the participant to connect to a Telehealth doctor, who based on the data from the Apple watch readings and symptoms will advise the patient to go to the ED if he deems the patient requires immediate medical attention or will recommend an EKG patch that will be shipped to the participant to be worn concurrently with the watch for up to 7 days and returned at the end of the study period for analysis.
The study population consisted of people aged ≥22 years without history of AF or atrial flutter and current use of anticoagulation. Of the study population, the mean age was 41 with the age group of 22 to 39 years old making 52% of the study population. About 2,161 people got notified of irregular heart rate and 658 of them ended up getting the EKG patch with only 450 returned for analysis. Notification rate was highest among the older population (≥65). AF was detected in 34% of the cohort, who received a notification and wore the EKG patch. This does not negate the fact that more people may have been diagnosed with AFib with longer EKG patch monitoring. Based on the analysis of the PPG technology used in the Apple watch validated by the concurrent use of the EKG patch, which is the current standard for diagnosing paroxysmal arrhythmias, the PPV of the tachograms was found to be 0.71 (95% CI: 0.69–0.74), and the PPV of notifications, triggered by 5 tachograms, was found to be 0.84 (95% CI: 0.76–0.92).
Although the study shows Apple watch might be a viable initial diagnostic tool in subclinical AF, the majority of the cohort was made up of young participants with the study failing to reach the target enrollment of 75,000 aged 65 or older. The population was also relatively healthier with 21% having hypertension, 5% having diabetes mellitus. The study also relied on self-reporting of the patients who are presented with the notification, though the post study survey reveals that 57% of the study population sought medical help outside of the Tele-health system implemented in the study, of which 36% underwent additional testing, with 33% being referred to a specialist and 28% being started on a new medication. This can be a sign that majority did indeed take the notification seriously to get it further investigated by a physician.
Kardia Band (KB)
KB is a product made by AliveCor designed to be used as an Apple Watch accessory. It mimics lead I and can record a rhythm strip for 30 s. The KB application can then use an algorithm to detect whether the strip shows atrial fibrillation (AF) or not. Bumgarner et al. (17) conducted a single center, prospective, nonrandomized, adjudicator-blinded study with 100 participants to assess whether KB algorithms could distinguish AF from sinus rhythm as well as electrophysiologist-interpreted 12-lead EKGs can. The three labels the KB algorithm can give a rhythm strip are “possible AF” (based on rhythm irregularity and P-wave absence), “normal” (no AF detected and heart rate is 50–100 beats/min), and “unclassified” (no AF detected and heart rate is <50 or >100 beats/min or recording is either noisy or >30 seconds, making up 34% of the recordings). The study population consisted of people aged 18–90 years with a history of AF who presented for a scheduled elective electrical cardioversion. Of the study population, the mean age was 68; 169 simultaneous KB and EKG recordings were made of each patient before and after cardioversion; 15 patients ended up not undergoing cardioversion with 8 of them cancelling due to being in sinus rhythm rather than AF.
Among the positive readings, the KB algorithm correctly detected AF with 93% sensitivity (95% CI: 86–99%), 84% specificity (95% CI: 73–95%), and k coefficient of 0.77 (95% CI: 0.65–0.89) when compared to electrophysiologist-interpreted 12-lead EKG recordings. Electrophysiologists who examined all 169 of the KB recordings (in total, they could interpret 147; 22 of them were non-interpretable due mainly to baseline artifact) correctly detected AF with 99% sensitivity (95% CI: 96–100%), 83% specificity (95% CI: 74–92%), and k coefficient of 0.83 (95% CI: 0.74–0.92) when compared to electrophysiologist-interpreted 12-lead EKG recordings. Thus, the study demonstrates that he KB algorithm is pretty effective in detecting AF, with increase in sensitivity when supported by physician review.
However, the authors note limitations of this study such as the small sample size and patients all having AF significant enough to be scheduled for electric cardioversion. Generalizability may also be limited due to participants being 17% female, socioeconomic status not being examined, patients with implanted pacemakers or defibrillators being excluded, and participants never having used KB before. There could have been less unclassified readings if participants had more practice with KB, but KB performance may decrease outside these study settings as the participants were taught how to use KB immediately before each supervised recording. The study including patients with already known AF limits it from commenting about its efficiency in detecting subclinical AF.
Handheld EKG devices
Another way Smartphones can be used as EKG monitors is by interfacing with peripherals such as a special smartphone case with embedded electrodes to acquire, store, and transfer single-channel EKG rhythms like AliveCor Heart Monitor (AHM), which has already been US Food and Drug Administration cleared and Conformite Europeenne (CE) marked, and My Diagnostick handheld EKG stick.
The Chan et al. outpatient study also analyzed single-lead EKG recorded by using the AliveCor heart monitor with tracings reviewed subsequently by 2 cardiologists to provide the reference standard in which Patients with hypertension, with diabetes mellitus, and/or aged ≥65 years were recruited. The study found that The diagnostic sensitivity of the AliveCor automated algorithm 71.4% (95% CI: 51–87%), the specificity 99.4% (95% CI: 99–100%), the PPV 76.9% (95% CI: 56–91%), NPV 99.2% (95% CI: 98–100%) (15).
To determine the usability, accuracy, and cost-effectiveness of two handheld single-EKG devices for AF screening in a hospital population; a study was conducted in which 445 hospitalized patients at cardiological or geriatric wards were screened for AF by two handheld EKG devices (My Diagnostick and AliveCor) (18). The performance of the automated algorithm of each device was evaluated against a full 12-leador 6-lead EKG recording. After the exclusion of patients with an implanted device, sensitivity and specificity of the automated algorithms were suboptimal (Cardiology: 81.8% and 94.2%, respectively, for MyDiagnostick; 54.5% and 97.5%, respectively, for AliveCor) (Geriatrics: 89.5% and 95.7%, respectively, for MyDiagnostick; 78.9% and 97.9%, respectively, for AliveCor).
Manual interpretation increases sensitivity, but decreases specificity, doubling the cost per detected patient, but remains cheaper than sole 12-lead EKG screening.
The accuracy of blinded clinician interpretation of single-lead smartphone electrocardiograms was investigated by conducting a prospective, blinded, observational cohort study in which Consecutive patients 18 years and older undergoing electrical cardioversions for AF and atrial flutter were recruited over 12 months (19). AHM was paired with an iPhone 6-Plus smartphone using the Kardia application. Three consecutive 30-second lead I recordings (iEKG) were obtained with finger placement on the 2 electrodes at the back of the iPhone. Following each AHM recording, the automated rhythm analysis recorded the rhythm as either possible AF, normal sinus rhythm, or unclassified. A 12-lead EKG was obtained prior to the iEKG tracings, both pre- and post- cardioversion. On assessing the accuracy of AHM on all tracings, including “unclassified” readings (marked as incorrect), the AHM demonstrated reduced sensitivity (77%) and specificity (76%).
These devices receive most interest for opportunistic screening, as they are easy to use, portable, low-cost, allow fast rhythm strip recordings, do not require experienced personnel, and often have built-in algorithms that provide an immediate interpretation of the EKG. There are also limitations for their use. The majority of false positives results originated from pulse waveforms that were corrupted by finger movement artifacts that may have affected the detection algorithm. This issue may lead to a reduction in specificity when the smartphone application is used outside the clinic because of potentially more motion artifacts in an unsupervised setting, e.g., using it repeatedly at home over a period of weeks or even longer. A high number of false positives could create additional work for clinicians to rule out AF. Another drawback of the application is the inability to detect atrial flutter that may also confer some risk of stroke and frequently accompanies AF.
Conclusions
The fact that there is a significant increase in consumer use of wearables, smart watches; which can serve as health monitoring devices that can be used as a non-invasive, ambulatory assessment of heart rate and rhythm, is definitely novel. The different wearable technology and its efficacy in detecting AF (Table 1) is well demonstrated in different studies. The demonstration of majority of the study population in the apple study seeking medical help after being notified is encouraging. On the flip side, false positives can cause undue anxiety to the user and may drive up health costs with unwarranted ER visits. The system of irregular rhythm notification in people without a known diagnosis of AF supported by physician review can help in uncovering the subclinical AF population.
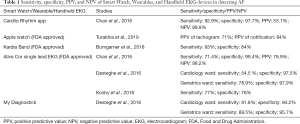
Full table
Recent evidence shows that intermittent short EKG recordings repeated over a longer-term period produced significantly better sensitivity for AF detection, with 4 times as many cases diagnosed compared with a single time-point measurement (20). This further reiterates the fact that these wearables serve as an excellent resource for continuous EKG monitoring with data saved in the cloud which can be assessed from anywhere, as compared to other devices as Holter monitors and event monitors, limited by the intermittent nature of monitoring and the need to be returned for analysis. In addition, they also are non-invasive methods for prolonged periods of screening compared to the invasive implantable loop recorders. Data also shows that preference of patients lean more towards wearable technology as they are perceived as convenient by 98%, while 90% conveyed they were likely to use these devices to determine cardiac rhythm during symptomatic episodes as opposed to conventional Holter monitoring system (21).
The technology may however be limited by patient specific barriers, like age. The prevalence of AF markedly increases with age, though older population may be more resistant in adopting these wearable technologies. The cohort in the Apple heart study were overwhelmingly young and healthier. Furthermore, studies are required to adjudicate the effectiveness of the wearables in detecting AF in high risk population. Plus, compared the smart watch technology, where there is an element of passive detection, the smart phone apps and handheld EKG have an active aspect of user participation for recording strips of EKG. Improper use may not yield proper data. Even the smartwatches have to overcome improper data measurements triggered by motion and hand movement, which may yield false positives. The technological shortcomings are bound to get better with more research and development poured into these devices and the detection algorithms. Future iterations of these devices will improve its efficiency to better detect the subclinical AF.
These devices have even shown to be cost-effective when used in a community screening program (22,23) and they can be used for the follow-up assessment of the effectiveness of AF treatment. Thus, the wearable technology may not serve as the ultimate tool for diagnosis of AF, rather a nidus for the general population to seek medical advice on being notified of having an irregular rhythm for confirmation leading to prevention of morbidity and mortality associated with it.
Acknowledgments
None.
Footnote
Conflicts of Interest: The authors have no conflicts of interest to declare.
Ethical Statement: The authors are accountable for all aspects of the work in ensuring that questions related to the accuracy or integrity of any part of the work are appropriately investigated and resolved.
References
- Miyasaka Y, Barnes ME, Gersh BJ, et al. Secular Trends in Incidence of Atrial Fibrillation in Olmsted County, Minnesota, 1980 to 2000, and Implications on the Projections for Future Prevalence. Circulation 2006;114:119-25. [Crossref] [PubMed]
- Wolf PA, Abbott RD, Kannel WB. Atrial fibrillation as an independent risk factor for stroke: the Framingham Study. Stroke 1991;22:983-8. [Crossref] [PubMed]
- Marini C, De Santis F, Sacco S, et al. Contribution of atrial fibrillation to incidence and outcome of ischemic stroke: results from a population-based study. Stroke. 2005;36:1115-9. [Crossref] [PubMed]
- Lin HJ, Wolf PA, Benjamin EJ, et al. Newly diagnosed atrial fibrillation and acute stroke. The Framingham Study. Stroke 1995;26:1527-30. [Crossref] [PubMed]
- Lowres N, Neubeck L, Redfern J, et al. Community screening programs to identify unknown atrial fibrillation: a systematic review. Eur Heart J 2012;33:61. [PubMed]
- Turakhia MP, Shafrin J, Bognar K, et al. Estimated prevalence of undiagnosed atrial fibrillation in the United States. PLoS One 2018;13:e0195088. [Crossref] [PubMed]
- Turakhia MP, Shafrin J, Bognar K, et al. Economic Burden of Undiagnosed Nonvalvular Atrial Fibrillation in the United States. Am J Cardiol 2015;116:733-9. [Crossref] [PubMed]
- Healey JS, Connolly SJ, Gold MR, et al. Subclinical atrial fibrillation and the risk of stroke. N Engl J Med 2012;366:120-9. [Crossref] [PubMed]
- IDC Forecasts Shipments of Wearable Devices to Nearly Double by 2021 as Smart Watches and New Product Categories Gain Traction. Available online: https://www.idc.com/getdoc.jsp?containerId=prUS43408517
- Lamkin P. Smartwatch popularity booms with fitness trackers on the slide. Forbes. Available online: https://www. forbes.com/sites/paullamkin/2018/02/22/smartwatch-popularity-booms-with-fitness-trackers-on-the-slide
- Koshy AN, Sajeev JK, Nerlekar N, et al. Utility of photoplethysmography for heart rate estimation among inpatients. Intern Med J 2018;48:587-91. [Crossref] [PubMed]
- Koshy AN, Sajeev JK, Nerlekar N, et al. Smart watches for heart rate assessment in atrial arrhythmias. Int J Cardiol 2018;266:124-7. [Crossref] [PubMed]
- Tison GH, Sanchez JM, Ballinger B, et al. Passive Detection of Atrial Fibrillation Using a Commercially Available Smartwatch. JAMA Cardiol 2018;3:409-16. [Crossref] [PubMed]
- Smith A. Record shares of Americans now own smartphones, have home broadband. Pew Internet & American Life Project 2017. Available online: https://www.pewresearch.org/fact-tank/2017/01/12/evolution-of-technology/
- Chan PH, Wong CK, Poh YC, et al. Diagnostic Performance of a Smartphone-Based Photoplethysmographic Application for Atrial Fibrillation Screening in a Primary Care Setting. J Am Heart Assoc 2016. [Crossref] [PubMed]
- Turakhia MP, Desai M, Hedlin H, et al. Rationale and design of a large-scale, app-based study to identify cardiac arrhythmias using a smartwatch: The Apple Heart Study. Am Heart J 2019;207:66-75. [Crossref] [PubMed]
- Bumgarner J, Lambert C, Cantillon D, et al. Assessing The Accuracy Of An Automated Atrial Fibrillation Detection Algorithm Using Novel Smartwatch Technology Among Patients Presenting For Elective Cardioversion. JACC 2018. [Crossref]
- Desteghe L, Heidbuchel H. Performance of handheld electrocardiogram devices to detect atrial fibrillation in a cardiology and geriatric ward setting: authors response. Europace 2017;19:1408-9. [PubMed]
- Koshy AN, Sajeev JK, Negishi K, et al. Accuracy of blinded clinician interpretation of single-lead smartphone electrocardiograms and a proposed clinical workflow. Am Heart J 2018;205:149-53. [Crossref] [PubMed]
- Svennberg E, Engdahl J, Al-Khalili F, et al. Mass Screening for Untreated Atrial Fibrillation: The STROKESTOP Study. Circulation 2015;131:2176-84. [Crossref] [PubMed]
- Rajakariar K, Koshy A, Sajeev J, et al. Increased preference of wearable devices compared with conventional cardiac monitoring. Heart, Lung Circulation 2018;27:S170. [Crossref]
- Levin LÅ, Husberg M, Sobocinski PD, et al. A cost-effectiveness analysis of screening for silent atrial fibrillation after ischaemic stroke. Europace 2015;17:207-14. [Crossref] [PubMed]
- Aronsson M, Svennberg E, Rosenqvist M, et al. Cost-effectiveness of mass screening for untreated atrial fibrillation using intermittent ECG recording. Europace 2015;17:1023-9. [Crossref] [PubMed]