Identification of lung-adenocarcinoma-related long non-coding RNAs by random walking on a competing endogenous RNA network
Introduction
Lung cancer (LC) is mainly divided into small cell lung cancer, and non-small cell lung cancer, and its risk factors, which include tobacco, environmental pollution, toxic substances, etc., are numerous (1,2). Lung adenocarcinoma (LUAD) is also a serious lung cancer, and it accounts for ~40% of all lung cancer patients (3). In China, patients with LUAD number over 1,000,000, and over 200,000 mortalities are associated with lung cancer annually. In addition, LUAD patients are always non-smokers (4). Its rapid development and early metastasis lead to a poor prognosis, and the prevention of LUAD therefore presents a significant challenge (5), with a major obstacle being the lack of reliable biomarkers.
Long non-coding RNAs (lncRNAs) are a set of non-coding RNAs longer than 200 nt (6), constituting the largest proportion of the mammalian non-coding transcriptome. LncRNAs participate in many essential biological processes, such as genomic imprinting, the maintenance of pluripotency, immune responses, and development (7). Recently, several lncRNAs have been discovered to be involved in LUAD tumorigenesis. LUADT1 is an imprinted gene that is significantly up-regulated in LUAD due to certain epigenetic events and contributes to the oncogenesis of LUAD (8). LncRNA-HNF1A-AS1, a mediator of DNMT1 signaling, could predispose LUAD patients to metastases and may serve as a potential target for antimetastatic therapies (9). LncRNAs can be used as biomarkers for cancer diagnosis, prognosis, and classification because they have cell-type specificity superior to that of most protein-coding genes and relatively stable local secondary structures, facilitating their detection in body fluids (10,11). There is an urgent demand for the identification of LUAD-risk lncRNAs for the purposes of prognosis and diagnosis. Recently, accumulating evidence has suggested that lncRNAs function as miRNA sponges or competing endogenous RNAs (ceRNAs), reducing the availability of miRNAs for mRNA target binding (12,13). By sharing common miRNA-binding sites with mRNAs, lncRNAs compete with miRNA target genes for miRNA molecules, thereby relieving miRNA-mediated target repression (14,15). For example, lncRNA NEAT1 accelerates LUAD deterioration through mir-193a-3p as a competitive endogenous RNA (16). The ceRNA network-based method has been widely used to investigate disease-related lncRNAs and their functions in multiple cancers (17,18).
Several network-based methods have been developed to detect disease risk factor. Random walking with restart (RWR) is a method that considers global topological information in networks to prioritize candidates, thereby achieving high prediction power. Moreover, RWR can be used on multi-omics integrated networks and has been widely used in several fields, including in the detection of metabolites, genes, and lncRNAs (19-21). Therefore, it is reasonable to suppose that LUAD-risk lncRNAs can be predicted by RWR on a ceRNA network.
In this study, we first constructed a LUAD-specific ceRNA network. Then, the RWR method was performed to predict LUAD-associated-risk lncRNAs. The leave-one-out cross-validation method was employed, and an area under the curve (AUC) over 0.83 was achieved. We also verified the lncRNAs predicted by our method by referring to the literature and experimental assays. Our study provides a list of LUAD candidate lncRNAs that may serve as potential diagnostic and prognostic biomarkers.
Methods
Expression data for the genes, lncRNAs, and miRNAs associated with LUAD
The RNA-seq V2 datasets of genes and miRNAs of LUAD were downloaded from The Cancer Genome Atlas database (TCGA; http://tcga-data.nci.nih.gov/). For miRNAs, reads per million reads (RPM) from TCGA project were used directly. For gene datasets, raw read counts for each exon were derived from exon quantification files provided by the TCGA level 3 data set. Then, reads per kilobases per million (RPKM) values were recalculated for the coding/lncRNA genes according to the equation: RPKM = (raw read counts × 109)/(total reads × length of lncRNA/coding genes), with raw read counts equal to the sum of raw read counts for all exons mapped entirely within the lncRNA/coding gene loci, and total reads equal to the sum of raw read counts calculated for all exons of a single sample. An annotation file downloaded from GENCODE (22) was used to map exons to coding/lncRNA genes. Genes with an RPKM of more than 1 and lncRNAs with an RPKM of more than 0 were defined as expressed. Then, the 350 cancer samples and 50 normal samples with matched gene expression, lncRNA expression, and miRNA expression profiles were extracted for further analyses.
Construction of the LUAD ceRNA network
The construction of the LUAD ceRNA network needed to meet two conditions to confirm the ceRNA regulation. First, the interactions of miRNA-mRNA and miRNA-lncRNA were obtained. Then, we had to calculate the PCC between these interactions to confirm the negative regulation between miRNA-mRNA and miRNA-lncRNA. Furthermore, according to the ceRNA theory, mRNA-lncRNA should be positively co-expressed (14,15,23). miRNA-mRNA interactions were downloaded from TarBase (24), and mirTarBase (25), which store manually curated collections of experimentally supported miRNA targets. For miRNA-lncRNA interactions, both a experimentally validated database and the computational method were used. First, miRNA-lncRNA interactions were predicted using TargetScan (26), mi-Randa (27), PITA (28), and RNAhybrid (29) with default parameters. The miRNA-lncRNA interactions which were predicted by more than two computational methods were defined as candidates. Then, these candidate miRNA-lncRNA interactions were filtered by experimental interactions in starBase (30) and DIANA-LncBase (31), which provide high-throughput HITS and PAR-CLIP experimental data. If any experimental evidence supported candidate interaction, the interaction was preserved. Furthermore, the correlations between miRNA and mRNA/lncRNAs were calculated using Pearson’s correlation coefficient (PCC) as follows:
in which n represents sample numbers for both gene/lncRNA expression profiles and miRNA profiles; xi represents the expression value of genes/lncRNAs in sample i; yi represents the expression value of miRNA in sample i; and
Known LUAD-related genes, miRNAs, and lncRNAs
Known LUAD-related genes (mRNA) were obtained from a previous study by Mok et al. (32). Known LUAD-related miRNAs were downloaded from miR2Disease (33), which is a manually curated database for microRNA deregulation in human disease. Known lncRNAs were downloaded from Lnc2Cancer (34), which stores manually curated experimentally supported lncRNAs associated with various human cancers, including LUAD.
Random walking with restart method
RWR is a ranking algorithm (35) that simulates a random walker starting on seed nodes; at each step, it moves from the current nodes to its neighbors with probability 1-α, or goes back to seed nodes with probability α. With p0 being the initial probability vector and pk representing a vector in which the i-th element holds the probability of being at node i at step k, then the probability pk+1 is defined as follows:
where W is the column-normalized adjacency matrix of the LUAD ceRNA network. The initial probability p0 is calculated by assigning equal probability to seed nodes representing members of the disease, with the sum of it equal to 1. After several iterations, the probability achieves a steady state p∞, and the iterations are stopped until the change between pk+1 and pk falls below 10–10 (measured by the L1 norm). Then, the candidate lncRNAs can be ranked according to p∞.
The RWR method could capture the interaction information among the ceRNA network to prioritize the candidate lncRNAs according to their proximity with known disease seed nodes. Only the candidate lncRNAs were ranked. The closer the proximity with seeds, the higher the rank the candidate lncRNAs received.
Performance measurement
To examine the performance of the RWR method, the leave-one-out cross-validation (LOOCV) method was performed. In each run of cross-validation, each of the known LUAD lncRNAs was taken as one test case, being removed from the seed nodes. The seed nodes were defined as the known LUAD mRNAs, miRNAs, and other known LUAD lncRNAs. The hold-out lncRNA, and other lncRNAs in the network were considered as candidates. We then performed the RWR method and obtained scores for all of the candidate lncRNAs, including the test lncRNA, and they were ranked together. Therefore, for each test lncRNA, we could obtain a rank list. Taking all rank lists of all known LUAD lncRNAs together, we could calculate the ratio of the known LUAD lncRNAs which ranked in top n%. The receiver operator characteristic (ROC) curve could also be plotted, and the area under this curve (AUC) could be calculated according to the above results. The ROC curve plots the true-positive rate (TPR) versus the false-positive rate (FPR). For evaluating rankings of LUAD-lncRNA predictions, here, ROC curves could be interpreted as a plot of the frequency of the known LUAD lncRNAs above the threshold versus the frequency of the known LUAD lncRNAs below the threshold, where the threshold is a specific position in the ranking (35,36).
Clinical tissues
All lung cancer tissues and their corresponding adjacent tissues were collected from surgical resections of human lung cancer samples at the Shengjing Hospital of China Medical University. Informed consent was obtained from all patients, and the research method was approved by the Ethics Committee of China Medical University. All experimental procedures were carried out according to the World Medical Association Declaration of Helsinki and by the guidelines and regulations approved by the China Medical University. All tissue samples were immediately frozen and preserved in liquid nitrogen until used.
Cell culture
Normal 16HBE bronchial epithelial cells and LUAD cells including SK-Lu-1, A549, and H1299 were obtained from the Cell Bank of the Chinese Academy of Sciences (Shanghai, China), and cultured in Dulbecco’s modified Eagle’s medium (Invitrogen, Carlsbad, CA, USA), supplemented with 10% fetal bovine serum (FBS; Invitrogen), 50 U/mL penicillin, and 50 µg/mL streptomycin (Invitrogen). Cells were maintained at 37 °C in a humidified incubator at 5% CO2.
siRNA transfection
The sequence of the MAPKAPK5-AS1 siRNA was AAGCUAGAAUGGGACCCCAGGUU, and that of the control siRNA was CGUACGCGGAAUACUUCGAUU. All siRNA oligos were synthesized by GenePharma, Shanghai, China.
For transfection, A549 cells were seeded for 24 h and subsequently transfected with MAPKAPK5-AS1 siRNA or control siRNA using Lipofectamine 2000 (Invitrogen) with serum-free medium according to the experiment being performed. Following transfection (5 h), cells were transferred to complete medium, and cell lysates were harvested 48 h after transfection.
RNA extraction and qRT-PCR
Total RNA was extracted using TRIzol reagent (Invitrogen) according to manufacturer protocol and transcribed into cDNA using Superscriptase II (Invitrogen). Real-time PCR was performed using Power SYBR Green PCR Master Mix (Life Technologies; Thermo Fisher Scientific, Waltham, MA, USA). The following primer sets were used for real-time PCR: MAPKAPK5-AS1-F 5'-CCCTAAGACACGCCGCATAC-3'; MAPKAPK5-AS1-R 5'-GGGTTGACCTCCACAGATCC-3'; GAPDH-F: 5'-AGCCTCCCGCTTCGCTCTCT-3'; GAPDH-R 5'-GCGCCCAATACGACCAAATCCGT-3'.
GAPDH was used for normalization.
Colony formation assay
A549 cells were transfected with MAPKAPK5-AS1 siRNA or control siRNA. Twenty-four hours after transfection, 800 cells were counted and seeded in 6 cm dishes. After 10 days, colonies were stained with 0.1% crystal violet in 20% methanol for 15 min. The samples were photographed, and the numbers of visible colonies were counted.
Cell counting Kit-8 (CCK-8) cell viability assay
A549 cells were transfected with MAPKAPK5-AS1 siRNA or control siRNA, and 24 h later, cells were transferred into 96-well plates at a density of 2×103 cells/well. Cell viability was assessed by CCK-8 assay (Dojindo, Tokyo, Japan) at days 0, 1, and 3.
Ki-67 immunofluorescence staining
A549 cells were seeded on coverslips and transfected with MAPKAPK5-AS1 siRNA or control siRNA. After 48 h, cells were fixed and incubated with Ki-67 antibody (Cell Signaling Technology, Danvers, MA, USA) for 1 h and then incubated with Alexa-488 at room temperature for 20 min. Cells were then counterstained with DAPI to detect the cell nuclei. All coverslips were mounted using prolong® diamond antifade mountant (ABI, USA.)
Acridine orange/ethidium bromide (AO/EB) fluorescence staining
A549 cells in the exponential growth phase were cultivated on sterile coverslips for 24 h, followed by transfection with MAPKAPK5-AS1 siRNA or control siRNA and incubation with AO/EB mixing solution for 5 min (Solarbio, Beijing, China). Cell morphological changes were examined by fluorescence microscopy (200×), and the percentage of apoptotic cells was calculated using the following formula: apoptotic rate (%) = number of apoptotic cells/total number of cells counted.
Statistical analysis
Data were obtained from ≥3 independent experiments. Data are presented as mean ± SD. Statistical comparisons among multiple groups were performed by analysis of variance (ANOVA) followed by Tukey’s multiple comparison test. Statistical values were calculated using the SPSS 19.0 software and illustrated using the GraphPad Prism 5.0. Differences with a value of P<0.05 were regarded as statistically significant.
Results
Construction of the LUAD ceRNA network
To construct the LUAD ceRNA network, interactions between miRNAs and target genes/lncRNAs were filtered. Interactions between miRNAs and target genes were obtained from TarBase and mirTarBase with experimentally supported datasets. After the elimination of redundancy, 43,497 validated non-redundant human miRNA-target pairs were obtained. For miRNA-lncRNA interactions, interactions predicted by more than two programs, including TargetScan, mi-Randa, PITA, and RNAhybrid, were considered as candidates. These candidate interactions were also filtered by experimental interactions in starBase and DIANA-LncBase. A total of 314,729 miRNA-lncRNA interactions were retained for further analysis. The negative expression regulatory relationships between miRNAs-genes/lncRNAs were also identified with PCC less than -0.1 and correlated adjusted P values of less than 0.01. Finally, we constructed a LUAD ceRNA network that included 1,229 interactions (244 miRNA-gene interactions and 985 miRNA-lncRNA interactions) between 214 lncRNAs, 116 miRNAs, and 214 genes (Figure 1). The degrees of all nodes are ranges from 1 to 87, following a power-law distribution (y=0.711x–0.53), with R-squared being 0.791 and a correlation value being 0.924 (Figure S1).
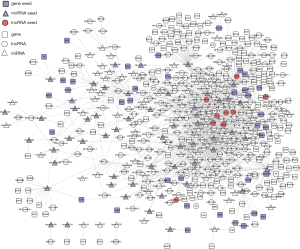
Performance of the RWR method to predict LUAD
To examine the performance of RWR in predicting LUAD, LOOCV method was performed. In each run of cross-validation, one known LUAD lncRNA, having being removed from the seed nodes, was considered as the test lncRNA. The seed nodes were defined as the known LUAD genes, miRNAs, and other known LUAD lncRNAs. Candidate lncRNAs were defined as all of the lncRNAs in the ceRNA network, excluding the seed lncRNAs. Next, we performed the RWR method and obtained scores of all of the candidate lncRNAs. The top-ranked lncRNAs were predicted to be related to LUAD. Next, ROC analysis was carried out to evaluate the overall performance by plotting the true positive rate versus the false positive rate at various threshold settings. There was one parameter, α, in the RWR method, which represented the restart probability. To test the effects of α, different values were assigned to α, and LOOCV analysis was performed. The resulting AUC values varied from 0.831 to 0.837 (Figure 2). So, α was taken as 0.3 for further analyses.
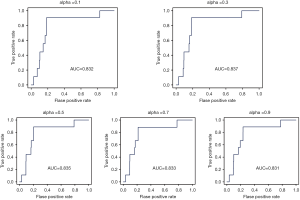
Investigating the robustness
We next assessed the robustness of the PROFANCY method after perturbation of the ceRNA network. After removing edges from 10% to 40%, we calculated the AUC value in each of these incomplete networks. We found that our method had strong resistance against the incompleteness of a network; the AUC value only had a slight decline (AUC=0.816) when deleting 10% edges (Table S1). Even when we deleted 30% edges, our method could keep a relatively high AUC value over 0.65.
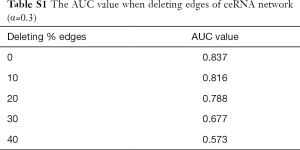
Full table
Predicting novel LUAD-related lncRNAs
We obtained 9 lncRNA seeds, 55 miRNA seeds, and 37 genes as seed nodes (see the Materials and Methods). The other 250 lncRNAs in the LUAD-specific ceRNA network were considered as candidates. Then, the RWR method was performed to predict LUAD-related lncRNAs. Table 1 lists the top 5 ranked lncRNAs related to LUAD. SNHG12, which had a four-fold changed expression in LUAD compared with normal tissue, was ranked first by our method. Recently, SNHG12 has been identified as a key regulator and validated by RT-PCR in LUAD tissues (37). SNHG6 ranked fifth and has been reported to serve as a prognostic marker for breast cancer combined with primary lung cancer (38).
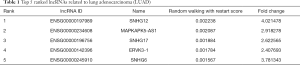
Full table
To further investigate the functional mechanism of predicted LUAD-related lncRNAs, a sub-network of SNHG12 and its first two neighbors were extracted from the ceRNA network. There were 147 nodes and 204 interactions in the SNHG12 related sub-network, which contained 36 seeds (13 miRNA seeds, 9 lncRNA seeds, and 14 gene seeds) (Figure 3A). Next, SNHG12 related gene neighbors were used to perform pathway enrichment analysis. The PI3K-Akt signaling pathway was significantly enriched (Figure 3B), suggesting that SNHG12 may be involved in this pathway and may play an essential role in LUAD.
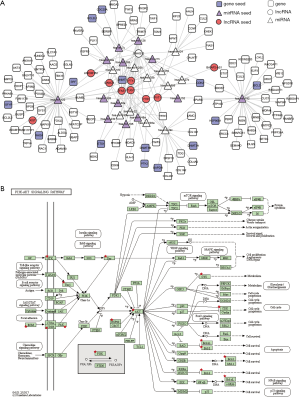
Similarly, the sub-network of SNHG6 contained 126 nodes and 129 interactions, among which there were 25 seeds (12 miRNA seeds and 13 gene seeds) (Figure 4A). SNHG6-related gene neighbors were significantly enriched in several cancer-related pathways, including the PI3K-Akt signaling pathway, the p53 signaling pathway, and the cell cycle pathway (Figure 4B).
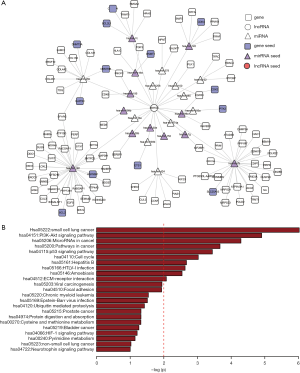
Although MAPKAPK5-AS1 has not been previously found to be associated with lung cancer, MAPKAPK5-AS1 was found to be significantly associated with overall survival with hepatocellular carcinoma and anaplastic gliomas (39,40). Thus, to validate this, we first analyzed its expression both in LUAD tissue samples and in LUAD cell lines. Compared with adjacent tissues, MAPKAPK5-AS1 expression was approximately seven-fold higher in lung cancer tissue (Figure 5A). Consistently, MAPKAPK5-AS1 was also overexpressed in all three LUAD cell lines (SK-lu-1, A549 and H1299) compared with human bronchial epithelioid cells (16HBE) (Figure 5B). Furthermore, we analyzed the function of MAPKAPK5-AS1 in LUAD in A549 cells. After silencing of MAPKAPK5-AS1 by siRNA transfection, a colony formation assay showed that fewer colonies were counted (Figure 5C).
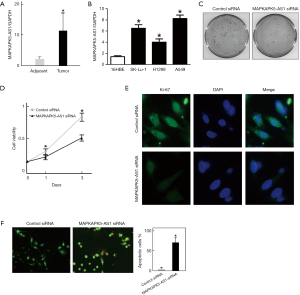
Additionally, the growth of A549 cells was significantly suppressed, which was observed by CCK-8 assay following MAPKAPK5-AS1 downregulation (Figure 5D). Moreover, immunofluorescence staining of Ki-67, a proliferation marker, revealed that the proliferation capability of A549 was dramatically reduced (Figure 5E). Finally, AO/EB staining showed an increased proportion of apoptotic cells in the A549 cells depleted of MAPKAPK5-AS1 (Figure 5F). All our results indicate that MAPKAPK5-AS1 plays a vital role in controlling A549 cell growth and survival.
Discussion
In the present study, we constructed a lung-cancer-specific ceRNA network to predict novel LUAD-associated lncRNAs. First, both miRNA-target gene/lncRNA interactions in multiple databases and negative expression correlations between miRNA-target genes were used to validate miRNA-target gene/lncRNA interactions. Then, the lung-cancer-specific ceRNA network was constructed with 544 nodes (214 lncRNAs, 116 miRNAs, and 214 genes) and 1,229 edges (244 miRNA-gene interactions and 985 miRNA-lncRNA interactions). The RWR method was performed to predict lung-cancer-associated risk lncRNAs. The LOOCV approach was used, and the prediction power achieved an AUC of over 0.83. Our method identified several novel lung-cancer-related lncRNAs, and some of these had previously been reported in the literature. Furthermore, we experimentally verified the role of MAPKAPK5-AS1 in lung cancer cell growth survival.
The success of this study could be attributed to two factors. First, the interactions we used to construct the lung-cancer-specific ceRNA network were supported by both experimental and computational approaches. Negative regulation of expression between miRNAs and their targets was also considered. In this way, it was possible to characterize the regulatory relationships in lung cancer. Second, different from methods which only consider direct interactions to other disease genes or the shortest-path distance to known disease genes, our use of the RWR method not only considered the direct and shortest-path interactions but also took the global structure of the interactome into account. In this study, we prioritized candidate lncRNAs based on their global similarity to known seeds, including known LUAD-related lncRNAs, miRNAs, and mRNAs, thereby capturing all topological information for the lung-cancer-specific ceRNA network to guarantee both accuracy and robustness.
Conclusions
In summary, we identified novel lncRNAs involved in lung cancer by random walking on a lung-cancer-specific ceRNA network. The role of MAPKAPK5-AS1 in LUAD was validated by siRNA transfection, a colony formation assay revealed fewer colonies, and a CCK-8 assay revealed significantly suppressed growth of A549 cells. Moreover, immunofluorescence staining of Ki-67 and AO/EB staining revealed the reduced proliferation capability and increased proportion of apoptotic cells of A549 following MAPKAPK5-AS1 downregulation. This lncRNA may serve as a biomarker for the diagnosis, treatment, and prognosis of lung cancer.
Acknowledgments
None.
Footnote
Conflicts of Interest: The authors have no conflicts of interest to declare.
Ethical Statement: The authors are accountable for all aspects of the work in ensuring that questions related to the accuracy or integrity of any part of the work are appropriately investigated and resolved. Informed consent was obtained from all patients, and the research method was approved by the Ethics Committee of China Medical University. All experimental procedures were carried out according to the World Medical Association Declaration of Helsinki and by the guidelines and regulations approved by the China Medical University.
References
- Guo X, Zhang Y, Zheng L, et al. Global characterization of T cells in non-small-cell lung cancer by single-cell sequencing. Nat Med 2018;24:978-85. [Crossref] [PubMed]
- Sun Y, Zhang R, Jiang Z, et al. Identifying candidate agents for lung adenocarcinoma by walking the human interactome. Onco Targets Ther 2016;9:5439-50. [Crossref] [PubMed]
- Mitiushkina NV, Tiurin VI, Iyevleva AG, et al. Variability in lung cancer response to ALK inhibitors cannot be explained by the diversity of ALK fusion variants. Biochimie 2018;154:19-24. [Crossref] [PubMed]
- Humphrey LL, Deffebach M, Pappas M, et al. Screening for lung cancer with low-dose computed tomography: a systematic review to update the US Preventive services task force recommendation. Ann Intern Med 2013;159:411-20. [Crossref] [PubMed]
- Nanavaty P, Alvarez MS, Alberts WM. Lung cancer screening: advantages, controversies, and applications. Cancer Control 2014;21:9-14. [Crossref] [PubMed]
- Perkel JM. Visiting "noncodarnia". BioTechniques 2013;54:301-303-4. [Crossref] [PubMed]
- Mercer TR, Dinger ME, Mattick JS. Long non-coding RNAs: insights into functions. Nat Rev Genet 2009;10:155-9. [Crossref] [PubMed]
- Qiu M, Xu Y, Wang J, et al. A novel lncRNA, LUADT1, promotes lung adenocarcinoma proliferation via the epigenetic suppression of p27. Cell Death Dis 2015;6:e1858. [Crossref] [PubMed]
- Wu Y, Liu H, Shi X, et al. The long non-coding RNA HNF1A-AS1 regulates proliferation and metastasis in lung adenocarcinoma. Oncotarget 2015;6:9160-72. [PubMed]
- Cabili MN, Trapnell C, Goff L, et al. Integrative annotation of human large intergenic noncoding RNAs reveals global properties and specific subclasses. Genes Dev 2011;25:1915-27. [Crossref] [PubMed]
- Yan X, Hu Z, Feng Y, et al. Comprehensive Genomic Characterization of Long Non-coding RNAs across Human Cancers. Cancer Cell 2015;28:529-40. [Crossref] [PubMed]
- Salmena L, Poliseno L, Tay Y, Kats L, Pandolfi PP. A ceRNA hypothesis: the Rosetta Stone of a hidden RNA language? Cell 2011;146:353-8. [Crossref] [PubMed]
- Tay Y, Rinn J, Pandolfi PP. The multilayered complexity of ceRNA crosstalk and competition. Nature 2014;505:344-52. [Crossref] [PubMed]
- Lai K, Jia S, Yu S, et al. Genome-wide analysis of aberrantly expressed lncRNAs and miRNAs with associated co-expression and ceRNA networks in beta-thalassemia and hereditary persistence of fetal hemoglobin. Oncotarget 2017;8:49931-43. [Crossref] [PubMed]
- Wang P, Ning S, Zhang Y, et al. Identification of lncRNA-associated competing triplets reveals global patterns and prognostic markers for cancer. Nucleic Acids Res 2015;43:3478-89. [Crossref] [PubMed]
- Xiong DD, Li ZY, Liang L, et al. The LncRNA NEAT1 Accelerates Lung Adenocarcinoma Deterioration and Binds to Mir-193a-3p as a Competitive Endogenous RNA. Cell Physiol Biochem 2018;48:905-18. [Crossref] [PubMed]
- Cai Y, Sun Z, Jia H, et al. Rpph1 Upregulates CDC42 Expression and Promotes Hippocampal Neuron Dendritic Spine Formation by Competing with miR-330-5p. Front Mol Neurosci 2017;10:27. [Crossref] [PubMed]
- Wang H, Niu L, Jiang S, et al. Comprehensive analysis of aberrantly expressed profiles of lncRNAs and miRNAs with associated ceRNA network in muscle-invasive bladder cancer. Oncotarget 2016;7:86174-85. [Crossref] [PubMed]
- Yao Q, Xu Y, Yang H, et al. Global Prioritization of Disease Candidate Metabolites Based on a Multi-omics Composite Network. Sci Rep 2015;5:17201. [Crossref] [PubMed]
- Yao Q, Wu L, Li J, et al. Global Prioritizing Disease Candidate lncRNAs via a Multi-level Composite Network. Sci Rep 2017;7:39516. [Crossref] [PubMed]
- Li Y, Patra JC. Genome-wide inferring gene-phenotype relationship by walking on the heterogeneous network. Bioinformatics 2010;26:1219-24. [Crossref] [PubMed]
- Harrow J, Frankish A, Gonzalez JM, et al. GENCODE: the reference human genome annotation for The ENCODE Project. Genome Res 2012;22:1760-74. [Crossref] [PubMed]
- Wang W, Lou W, Ding B, et al. A novel mRNA-miRNA-lncRNA competing endogenous RNA triple sub-network associated with prognosis of pancreatic cancer. Aging 2019;11:2610-27. [Crossref] [PubMed]
- Vlachos IS, Paraskevopoulou MD, Karagkouni D, et al. DIANA-TarBase v7.0: indexing more than half a million experimentally supported miRNA:mRNA interactions. Nucleic Acids Res 2015;43:D153-9. [Crossref] [PubMed]
- Chou CH, Chang NW, Shrestha S, et al. miRTarBase 2016: updates to the experimentally validated miRNA-target interactions database. Nucleic Acids Res 2016;44:D239-47. [Crossref] [PubMed]
- Lewis BP, Burge CB, Bartel DP. Conserved seed pairing, often flanked by adenosines, indicates that thousands of human genes are microRNA targets. Cell 2005;120:15-20. [Crossref] [PubMed]
- Betel D, Koppal A, Agius P, et al. Comprehensive modeling of microRNA targets predicts functional non-conserved and non-canonical sites. Genome Biol 2010;11:R90. [Crossref] [PubMed]
- Kertesz M, Iovino N, Unnerstall U, et al. The role of site accessibility in microRNA target recognition. Nat Genet 2007;39:1278-84. [Crossref] [PubMed]
- Rehmsmeier M, Steffen P, Hochsmann M, et al. Fast and effective prediction of microRNA/target duplexes. RNA 2004;10:1507-17. [Crossref] [PubMed]
- Li JH, Liu S, Zhou H, et al. starBase v2.0: decoding miRNA-ceRNA, miRNA-ncRNA and protein-RNA interaction networks from large-scale CLIP-Seq data. Nucleic Acids Res 2014;42:D92-7. [Crossref] [PubMed]
- Paraskevopoulou MD, Georgakilas G, Kostoulas N, et al. DIANA-LncBase: experimentally verified and computationally predicted microRNA targets on long non-coding RNAs. Nucleic Acids Res 2013;41:D239-45. [Crossref] [PubMed]
- Mok SR, Mohan S, Grewal N, et al. A genetic database can be utilized to identify potential biomarkers for biphenotypic hepatocellular carcinoma-cholangiocarcinoma. J Gastrointest Oncol 2016;7:570-9. [Crossref] [PubMed]
- Jiang Q, Wang Y, Hao Y, et al. miR2Disease: a manually curated database for microRNA deregulation in human disease. Nucleic Acids Res 2009;37:D98-104. [Crossref] [PubMed]
- Ning S, Zhang J, Wang P, et al. Lnc2Cancer: a manually curated database of experimentally supported lncRNAs associated with various human cancers. Nucleic Acids Res 2016;44:D980-5. [Crossref] [PubMed]
- Köhler S, Bauer S, Horn D, et al. Walking the interactome for prioritization of candidate disease genes. Am J Hum Genet 2008;82:949-58. [Crossref] [PubMed]
- Aerts S, Lambrechts D, Maity S, et al. Gene prioritization through genomic data fusion. Nat Biotechnol 2006;24:537-44. [Crossref] [PubMed]
- Zhu TG, Xiao X, Wei Q, et al. Revealing potential long non-coding RNA biomarkers in lung adenocarcinoma using long non-coding RNA-mediated competitive endogenous RNA network. Braz J Med Biol Res 2017;50:e6297. [Crossref] [PubMed]
- Ding X, Zhang Y, Yang H, et al. Long non-coding RNAs may serve as biomarkers in breast cancer combined with primary lung cancer. Oncotarget 2017;8:58210-21. [PubMed]
- Zhang J, Fan D, Jian Z, et al. Cancer Specific Long Noncoding RNAs Show Differential Expression Patterns and Competing Endogenous RNA Potential in Hepatocellular Carcinoma. PLoS One 2015;10:e0141042. [Crossref] [PubMed]
- Li N, Wang C, Zhang P, et al. Emodin inhibits pancreatic cancer EMT and invasion by upregulating microRNA1271. Mol Med Rep 2018;18:3366-74. [PubMed]