Net benefit of routine urine parameters for urinary tract infection screening: a decision curve analysis
Introduction
Urinary tract infection (UTI) is common in clinical practice and urine culture is the “gold standard” for its diagnosis (1). However, urine culture has its intrinsic disadvantages. First, it is time- and labor-consuming and does not facilitate early diagnosis. Second, a large portion of specimens sent for urine culture are negative and thus increase the workload of the clinical microbiology laboratory. Therefore, developing UTI screening tools to decrease the rates of unnecessary urine culture is of great value. However, it should be kept in mind that the screening tools are typical “double-edged sword”, although it can decrease the rates of unnecessary urine culture, some UTI patients may be missed. Therefore, it is necessary to evaluate whether the screening tool is of net benefit.
Routine urine analysis has been widely used in UTI screening for a long time. A previous meta-analysis indicated that white blood cells (WBCs) and bacteria, which are measured by automated urine analyzer, are useful tools for UTI screening (2). The sensitivity and specificity for WBCs in the previous meta-analysis were 0.87 and 0.67, respectively. For urine bacteria, the sensitivity and specificity were 0.92 and 0.60, respectively (2). Additionally, two previous studies also indicated that urine nitrite is of value for UTI diagnosis (3,4). However, to the best of our knowledge, this type of research has only reported the sensitivity, specificity and area under receiver operating characteristic (ROC) curve (AUC). These metrics, although they have been widely used in screening or diagnostic trials, only reflect the predictive accuracy of an index test and do not incorporate information on consequences. Additionally, it also remains unclear whether these tests, when used together, can improve the predictive accuracy and net benefit for UTI.
In this study, we investigated the predictive accuracy of a combined model incorporating WBCs, bacterial and nitrite using the logistic regression model, net reclassification improvement (NRI) and integrated discrimination improvement (IDI). Additionally, the net benefit of the model and the single test was analyzed using decision curve analysis (DCA).
Methods
Data collection
This is a retrospective observational study using the data stored in the laboratory information system (LIS) of our institution. All urine specimens sent to the clinical microbiology laboratory for culture between January 2017 and April 2017 were eligible. The exclusion criteria were the following: (I) specimens without routine urine analysis within 24 hours before or after urine culture; (II) contaminated specimens, defined as more than three types of bacteria identified. Of the remaining eligible cases, we extracted the following data from the LIS: age, sex, urine culture results, urine WBCs, bacteria and nitrite. Urine WBCs and bacteria were detected with Sysmex UF-1000i automated urine sediment analyzer. UTI was defined as more than 105 cfu/mL bacterial growth, as previously described (5).
This study was approved by the ethics committee of our institution (No. KY2018026) and the study was performed in accordance with the Declaration of Helsinki. Informed consent was waived because the retrospective design of this study.
Sample size estimation
According to our experience, the prevalence of UTI is approximately 15% in the urine specimens sent to clinical microbiology laboratory for culture. The alpha, AUC of ROC and its 95% confidence interval (95% CI) width were set at 0.05, 0.80 and 0.10, respectively. The calculated sample size was 780, including 117 UTI specimens from UTI patients. Sample estimation was performed with PASS 15 (NCSS Statistical Software, Kaysville, Utah, USA).
Statistical analysis
Because all continuous variables were not normally distributed (Kolmogorov-Smirnov test), they were expressed as median and interquartile and compared with the Mann-Whitney test. Categorical variables were expressed as absolute number and compared with the chi-square test. The ROC curve analysis was used to estimate the screening performance of WBCs and bacteria for UTI. We used the logistic regression analysis to incorporate WBCs, bacteria and nitrite into a model and estimated the screening performance of the model using ROC curve analysis. The threshold with maximum Youden index was chosen as the optimal threshold and the corresponding sensitivity and specificity were calculated. NRI and IDI were used to estimate whether the model, by incorporating WBCs, bacteria and nitrite, provides added values beyond a single test (6). DCA was used to estimate the net benefit of WBCs, bacteria and the model (7). All analyses were performed with Sigmaplot 12.0 (Systat Software Inc., San Jose, CA, USA), SPSS 18.0 (SPSS, Inc., Chicago, IL, USA) and R (https://www.r-project.org/, version 3.5.0). The packages used with R included PredictABLE (8) and rmda (9). A P value less than 0.05 was defined as statistically significant.
Results
Characteristics of the subjects
Between January 2017 and April 2017, 1,525 urine specimens were sent to our clinical microbiology laboratory for culture. Among them, 140 specimens were contaminated, and 569 specimens did not have results of urine flow cytometry. Finally, 927 specimens with 156 UTIs were included in the present study. The characteristics of these specimens are summarized in Table 1.
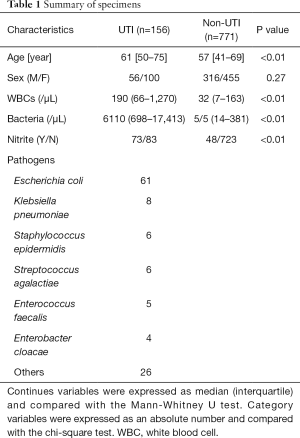
Full table
Assessment the performance of WBCs and bacteria for UTI screening using ROC curve analysis, NRI and IDI
Figure 1 is a ROC curve depicting the screening accuracy of WBCs and bacteria for UTI. The AUCs (95% CI) of WBCs and bacteria were 0.729 (95% CI: 0.690–0.769) and 0.836 (95% CI: 0.800–0.872), respectively. Next, we used logistic regression analysis to incorporate WBCs, bacteria and nitrite into a model. The coefficients and odds ratios (ORs) of WBCs, bacteria and nitrite are listed in Table 2.
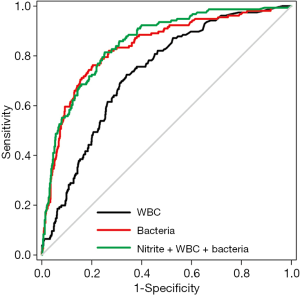
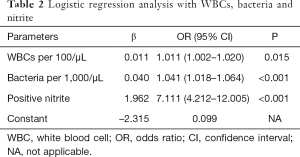
Full table
The AUCs, optimal sensitivities and specificities of WBCs, bacteria and the model are listed in Table 3. The AUC for this model was 0.851 (95% CI: 0.820–0.883), which was significantly higher than that of WBCs (P<0.001), but not significantly higher than that of bacteria (P=0.531).
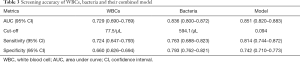
Full table
Considering that the AUC of bacteria was significantly higher than that of WBCs, we further analyzed whether WBCs and nitrite could provide added value in addition to bacteria. Using IDI and NRI, we found that nitrite and WBCs could significantly improve the predictive accuracy of bacteria, with a continuous NRI of 0.642 (95% CI: 0.484–0.800; P<0.001) and an IDI of 0.070 (95% CI: 0.042–0.098; P<0.001).
Assessment of the clinical efficiency of WBCs and bacteria for UTI screening using DCA
Figure 2 is a decision curve depicting the clinical efficiency of WBCs, bacteria and their combination (the model) for screening UTI. Notably, the clinical efficiency of their combination was higher than that of both bacteria and WBCs alone. The net benefit of bacteria and the model was closed 0.08; at a threshold of 0.30, the net benefit of WBCs, bacteria and the model were 0.0025, 0.0419 and 0.0627, respectively (Table 4). Using the net benefit formula (7), we calculated that the number of false positive results per 100 patients decreased, with WBCs, bacteria and the model being approximately 1, 10 and 15, respectively, with no decrease in the number of patients with UTI.
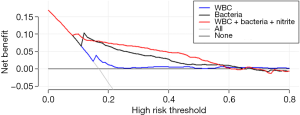
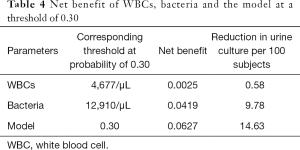
Full table
Discussion
In this study, we found that urine WBCs, bacteria and nitrite were useful screening tools for UTI screening. Using DCA, we found that bacteria had a high net benefit for UTI screening while the net benefit introduced by WBCs was low. Furthermore, combined use of WBCs, bacteria and nitrite had a higher net benefit than using bacteria alone.
Similar to a previous meta-analysis (2), we found that both WBCs and bacteria had a higher screening performance for UTI screening, and the screening performance of bacteria was significantly higher than that of WBCs. However, compared with previous studies, our study had two strengths. First, we found that combined use of WBCs, bacteria and nitrite improved the efficiency of UTI screening. The logistic regression model incorporating WBCs, bacteria and nitrite had a significantly higher AUC than that of WBCs. Furthermore, the results of IDI and NRI indicated that combined use of WBCs, nitrite and bacteria had a higher screening efficiency than the single parameter alone. Second, previous studies only calculated the AUC, optimal thresholds, and corresponding sensitivity and specificity of routine urine parameters for UTI screening. However, these metrics only concern the accuracy of an index test, and do not incorporate information on clinical consequence (7). In this study, we used DCA to estimate the net benefit of these parameters. Unlike traditional ROC curve analysis, DCA uses “treat all” and “treat none” as reference and can calculate the net benefit of an index test. We noted that the decision curve of WBCs nearly overlapped with the reference line (“treat all” and “treat none”), indicating that it has limited value in UTI screening, although its AUC was 0.729. By contrast, the decision curve of bacteria was notably above the reference line, indicating that introducing urine bacteria into the UTI screening procedure has a high net benefit. Furthermore, we noted that the decision curve of the model incorporating bacteria, WBCs and nitrite was above that of bacteria, indicating that combined use of WBCs, bacteria and nitrite has a significantly higher net benefit than that of a single one.
Our findings had some clinical implications. Because WBCs, bacteria and nitrite are usually tested together to screen UTI in clinical practice, the coefficients (Table 2) of these parameters can be easily incorporated into the LIS and thus the probability of UTI can be easily calculated. Listing this probability into their laboratory reports may help clinicians better manage suspected UTI subjects.
Our study has two limitations. First, this is a single center study with a small sample size. The coefficients of WBCs, bacteria and nitrite in a logistic regression model may be affected by the prevalence of the UTI and the disease spectrum in the target population. Second, this is a retrospective study based on the specimens sent to the clinical microbiology laboratory, and no inclusion and exclusion criteria were defined on symptoms and signs. Therefore, the representativeness of the studied cohort may be negatively affected. Our results need to be validated by other centers. Despite these limitations, our study did analyze the screening performance of routine urinary parameters using DCA and thus can provide a new insight into UTI screening. Further prospective, multicenter studies with larger sample sizes are needed to validate the findings of this study.
Conclusions
In conclusion, our study indicates that urine bacteria had a high net benefit for UTI screening, while WBCs had limited value. Furthermore, combined use of bacteria, WBCs and nitrite had a higher net benefit than any single one of these parameters alone.
Acknowledgments
We thank Mr. John Ayric Gray in AME publishing company for editing this manuscript.
Funding: None.
Footnote
Provenance and Peer Review: This article was commissioned by the Guest Editors (Zhi-De Hu, Bing Gu) for the series “Advances in laboratory tests for infectious diseases” published in Annals of Translational Medicine. The article was sent for external peer review organized by the Guest Editors and the editorial office.
Conflicts of Interest: All authors have completed the ICMJE uniform disclosure form (available at http://dx.doi.org/10.21037/atm.2019.09.52). The series “Advances in Laboratory Tests for Infectious Diseases” was commissioned by the editorial office without any funding or sponsorship. ZDH served as the unpaid Guest Editor of the series and serves as an unpaid Executive Editor of Annals of Translational Medicine from Apr 2020 to Mar 2025. The other authors have no other conflicts of interest to declare.
Ethical Statement: The authors are accountable for all aspects of the work in ensuring that questions related to the accuracy or integrity of any part of the work are appropriately investigated and resolved. This study was approved by the ethics committee of our institution (No. KY2018026) and the study was performed in accordance with the Declaration of Helsinki. Informed consent was waived because the retrospective design of this study.
Open Access Statement: This is an Open Access article distributed in accordance with the Creative Commons Attribution-NonCommercial-NoDerivs 4.0 International License (CC BY-NC-ND 4.0), which permits the non-commercial replication and distribution of the article with the strict proviso that no changes or edits are made and the original work is properly cited (including links to both the formal publication through the relevant DOI and the license). See: https://creativecommons.org/licenses/by-nc-nd/4.0/.
References
- Gupta K, Grigoryan L, Trautner B. Urinary tract infection. Ann Intern Med 2017;167:ITC49-64. [Crossref] [PubMed]
- Shang YJ, Wang QQ, Zhang JR, et al. Systematic review and meta-analysis of flow cytometry in urinary tract infection screening. Clin Chim Acta 2013;424:90-5. [Crossref] [PubMed]
- Mori R, Yonemoto N, Fitzgerald A, et al. Diagnostic performance of urine dipstick testing in children with suspected UTI: a systematic review of relationship with age and comparison with microscopy. Acta Paediatr 2010;99:581-4. [Crossref] [PubMed]
- Devillé WL, Yzermans JC, van Duijn NP, et al. The urine dipstick test useful to rule out infections. A meta-analysis of the accuracy. BMC Urol 2004;4:4. [Crossref] [PubMed]
- De Rosa R, Grosso S, Lorenzi G, et al. Evaluation of the new Sysmex UF-5000 fluorescence flow cytometry analyser for ruling out bacterial urinary tract infection and for prediction of Gram negative bacteria in urine cultures. Clin Chim Acta 2018;484:171-8. [Crossref] [PubMed]
- Pencina MJ, D'Agostino RB Sr, D'Agostino RB Jr, et al. Evaluating the added predictive ability of a new marker: from area under the ROC curve to reclassification and beyond. Stat Med 2008;27:157-72; discussion 207-12. [Crossref] [PubMed]
- Vickers AJ, Elkin EB. Decision curve analysis: a novel method for evaluating prediction models. Med Decis Making 2006;26:565-74. [Crossref] [PubMed]
- Kundu S, Aulchenko YS, van Duijn CM, et al. PredictABEL: an R package for the assessment of risk prediction models. Eur J Epidemiol 2011;26:261-4. [Crossref] [PubMed]
- Zhang Z, Rousson V, Lee WC, et al. Decision curve analysis: a technical note. Ann Transl Med 2018;6:308. [Crossref] [PubMed]