Variations in gut microbial profiles in ankylosing spondylitis: disease phenotype-related dysbiosis
Introduction
Spondyloarthritis (SpA) refers to a group of disease with overlapping clinical features and pathogenic mechanisms, yet with important clinical and outcome differences, including psoriatic arthritis (PsA), arthritis related to inflammatory bowel disease (IBD), reactive arthritis, juvenile idiopathic arthritis (JIA), and ankylosing spondylitis (AS) (1). The exact pathogenesis of SpA remains unknown, however, growing evidence implicated that SpA is the consequence of a complex interaction between genetic polymorphisms and environmental factors (2,3).
AS, a prototypic and best studied subtype of SpA, is characterised by the sacroiliitis and spondylitis, which may lead to bony ankylosis in its extreme (4,5). Human leukocyte antigen (HLA)-B27 is the dominant genetic risk factor for AS (6), and is present in as many as 90% of individuals with AS. Although it is almost necessary to have HLA-B27 for the development of AS, it is not sufficient. Less than 5% of HLA-B27 carriers are affected (7). Interestingly, in HLA-B27/β2-microglobulin-transgenic (β2m-Tg) rat, one of the most important animal model of SpA (8), animals remain healthy in an environment completely free of known microbes, while reintroduction of normal commensal gut bacteria is sufficient to trigger colitis and arthritis (9). More recently, HLA-B27/β2m-Tg rats were shown to have different gut microbiota with an increase in the abundance of Prevotella spp. and a decrease in the abundance of Rikenellaceae when compared with wild-type rats (10) and accompanied by perturbed mucosal immunity (11). In AS patients, as many as 40–60% of them were present with subclinical intestinal inflammation, and 5–10% of these cases will progress into clinical established IBD during their disease course (12). Increasing studies showed evidence for the link between gut dysbiosis and IBD. So, from the above perspectives, it is reasonable for us to consider that gut dysbiosis maybe one of important factors contributing to the pathogenesis of AS. A study of 10 AS patients and 9 healthy controls (HCs) by 16S ribosomal DNA sequencing analysis showed dysbiosis in terminal ileum biopsy specimens of AS patients (13). And a more recent study based on shotgun sequencing using gut microbial DNA from fecal samples of 211 Chinese individuals also shown alteration of gut microbiome in AS patients (14).
As we know, AS showed complicated clinical symptoms, comprising of axial and asymmetric peripheral joint inflammation and extra-articular manifestations, including uveitis, psoriasis, and IBD. Nearly 50% of AS patients have peripheral manifestation such as peripheral arthritis, enthesis or dactylitis, and these peripheral symptoms seem to contribute to higher level of disease activity in AS patients (15). Sulfasalazine (SASP) is a sulfa antimicrobial used to treat IBD in clinic for years (16), and also applied in AS patients who have concomitant peripheral manifestations (17,18). SASP showed no efficacy for AS patients with only axial joints involved (19). Of note, in rats with experimental colitis, SASP alleviated colitis through modulating the gut microbiome composition and function (20). This may suggest that gut microbiome might be associated with the distinguished efficacy of SASP in AS patients with different phenotype. However, no research has investigated the features of the gut microbiota in patients with different phenotypes of AS. And whether there are differences in gut microbiome between AS patients with only axial involved and concomitant peripheral joints involved remain to be determined.
In this study, we characterized the gut microbiota in fecal samples from AS patients using 16S ribosomal DNA sequencing and identified specific features of the gut microbiota showing association with phenotypes of AS. We also constructed a disease classifier for discriminating between HCs and different AS subgroups.
Methods
Participants
AS patients were recruited from outpatient Clinic of Rheumatology and Immunology Department of the Third Affiliated Hospital of Sun Yat-sen University from January to June 2017. All patients were older than 18 years old, and fulfilled the 1984 modified New York criteria for AS (21). All patients were free from conventional disease modifying anti-rheumatic drugs (such as SASP), corticosteroids, and biological agents for at least 3 months before sample collection. Demographic data, body mass index (BMI), clinical manifestation, HLA-B27 status, erythrocyte sedimentation rate (ESR), C-reactive protein (CRP), and therapeutic regimen and metrics for disease activity [i.e., ankylosing spondylitis disease activity score (ASDAS)] (22) were collected from patients by trained investigators. According to the 2009 ASAS classification criteria for SpA (23), patients with ever or currently peripheral manifestations including peripheral arthritis, and/or enthesitis, and/or dactylitis were classified as the peripheral AS (pAS) and others were classified into the axial AS (axAS) subgroup. HCs were enrolled among those took annual physical examination in the Third Affiliated Hospital of Sun Yat-sen University. They were never diagnosed with AS, IBD or any other autoimmune disease.
Individuals with the following disease were excluded from the study: cardiovascular disease, diabetes mellitus, cirrhosis and infection disease. Individuals taking antibiotic drugs or probiotic supplements within 2 months prior to sample collection were also excluded from the study.
All participants gave written informed consent and the study was approved by the ethics committee of the Third Affiliated Hospital of Sun Yat-sen University.
Samples collection and DNA extraction
Fresh stool samples were collected from participants and placed into sterile boxes and transported within ice boxes within 2 hours to the laboratory for further processing. Each stool sample was divided into 5 aliquots (200 mg) with Eppendorf tube and stored at –80 °C until DNA extraction. Microbial DNA was extracted with a fecal DNA extraction kit (Tiangen Biotech Co., Ltd., Beijing, China.) according to the manufacturer’s instruction. Finally, DNA was suspended in 100 mL AE buffer and stored at –80 °C for further analysis.
16S rRNA gene sequencing and data processing
The V4 region of 16S rRNA gene from each sample was amplified using dual-indexed V4-region primer (515F, 5'-GTGCCAGCMGCCGCGGTAA-3', and 806R 5'-GGACTACHVGGGTWTCTAAT-3') (24) with barcodes. All polymerase chain reaction (PCR) reactions were carried out with Phusion® High-Fidelity PCR Master Mix (New England Biolabs, Ipswich, MA, USA), and mixture PCR products were purified with Qiagen Gel Extraction Kit (Qiagen, Hilden, Germany). The library quality was assessed on the Qubit® 2.0 Fluorometer (Thermo Scientific, Waltham, MA, USA) and Agilent Bioanalyzer 2100 systems. Finally, the library was sequenced on an Illumina HiSeq2500 platform and 250 bp paired-end reads were generated.
Paired-end reads at 250 bp in length were merged using Flash (v1.2.7) (25), and then processed through a QIIME-based bioinformatics pipeline. Briefly, we conducted data filtration according to the QIIME (v1.9.1) (26) quality controlled process to reduce sequencing and PCR errors, aligned the resulting sequences to the SILVA 16S rRNA sequence database (27), and use UCHIME to remove any chimeric sequences (28,29). Sequences were clustered into operational taxonomic units (OTUs) at 97% similarity cutoff using Uparse (v7.0.1001) (30). All sequences were classified using a naive Bayesian classifier trained against the RDP training set (v14), and OTUs were assigned a classification based on which taxonomy had the majority consensus of sequences within a given OTU (31). Finally, we obtained 65,826 (55,691 to 75,470) sequences per sample for further analysis. To limit the effects of uneven sample, we rarefied the data set to 55,691 sequences per sample. Alpha diversity on Shannon and Simpson index and beta diversity on weighted and unweighted UniFrac were also calculated by QIIME (v1.9.1).
Statistical analysis
R (v3.4.4) was used to analyze the data. Mann-Whitney and chi-square tests were used to compare continuous and categorical demographics/clinical factors, respectively. Mann-Whitney was used to compared Shannon and Simpson index between groups. Distance matrices were assessed for similarity between groups using permutational multivariate analysis of variance (PERMANOVA) with vegan R package (v2.4.4). Principal coordinates analysis (PCoA) was used for visualization of the data present in the beta diversity distance matrix. Differences of gut microbiome were analysed using Kruskal-Wallis test for univariate comparison; and P value less than 0.05 following a false discovery rate (FDR) correction for multiple comparisons was considered statistically significant. To define more precisely the taxa that were driving the differentiation for the microbiota of the groups, we performed analysis using linear discriminant analysis (LDA) effect size (LEfSe, an algorithm for high-dimensional biomarker discovery which exploits LDA to robustly identify features statistically different among classes) (32) based on a web-based tool Microbiomeanalyst (a tool for comprehensive statistical, visual and meta-analysis of microbiome data) (33). Random forest algorithm and a stratified 10-fold cross-validation approach (34) were used to set up a gut microbiota-based model for predictive diagnosis of AS and its subtypes with random forest package (v3.4.4). Receiver operating characteristic (ROC) curve was plotted and the area under the curve (AUC) was used evaluate the performance of the random forest classifier.
Results
Population characteristics
A total of 60 individuals were enrolled in the current study, composed of 41 AS patients and 19 HCs (Table 1). AS patients and HCs were age, gender and BMI matched (P>0.05). Among AS patients, 20 patients were defined as axAS while 21 were defined as pAS. The mean age of the patients with axAS was 29.90±10.03 compared with 28.03±10.61 for patients with pAS (P>0.05). Patients with pAS had an earlier disease onset [18.0 (7.00) years] than patients with axAS [25.5 (11.75) years, P<0.01]. The two subgroups of patients had similar disease activity according to measurements such as CRP, ESR and Bath AS disease activity index (BASDAI).
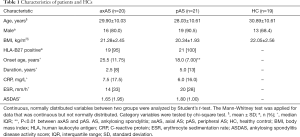
Full table
Profile of gut microbiota in AS
To assess the overall structure of the gut microbiota, the score plot of the principal coordinate analysis based on unweighted UniFrac distances was constructed, and the results showed that the structure and composition of the microbiota differed significantly between AS and HCs (Figure 1A). Measurement of within-sample diversity (alpha diversity) revealed no significant differences between the HC subjects and the patients with AS based on the Shannon and Simpson index (Figure 1B). That might indicate that the differences of gut microbiota between patients and HCs were majorly driven by the differential abundance of bacteria instead of the quantity of bacterial species.
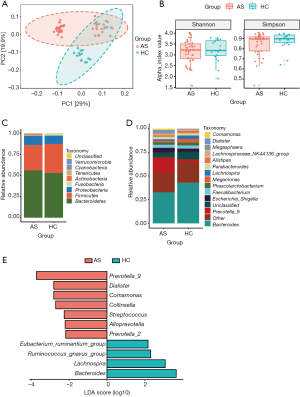
At phylum level, Bacteroidetes, Firmicutes, and Proteobacteria were the most common phyla identified in the two groups, contributing 97.46% and 97.51% of the gut bacteria in the AS group and HC group, respectively (Figure 1C). At the genus level, 133 genera were classified from the fecal bacteria. The predominant genera were defined as comprising greater than 1% of the total gut bacteria. Nineteen predominant genera were detected in the AS group, and 15 genera were detected in the HC group, including 13 genera found in both groups (Figure 1D, Table S1). These predominant genera accounted for 81.39% and 77.53% of the total sequences from AS group and HC group, respectively. Bacteroides was the most predominant genera in both groups, but was significantly decreased in the patients with AS. In addition, the Prevotella_9 was the second predominant genera accounting for 16.12% of total sequences in AS group, while it only accounting for 2.86% in HC group. Average composition of bacterial community at the phylum and genus levels were shown in Figure 1, respectively.
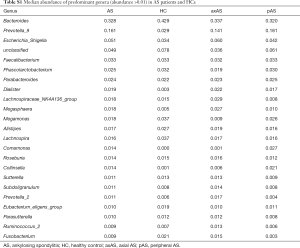
Full table
Compared with the HC group, at phylum level, AS patients were identified with significantly higher abundance of Actinobacteria (FDR =0.003, Figure S1A), and lower abundance of Tenericutes and Verrucomicrobia (FDR =0.007 and 0.003, respectively, Figure S1A). At genus level, 33 genera belong to 5 phyla, showed significant difference between AS patients and HCs (all FDR <0.05, Figure S1B, Table S2).
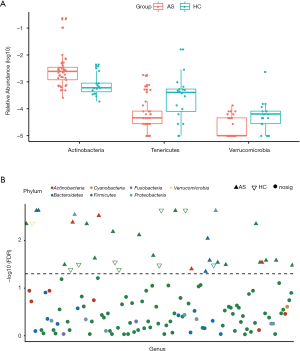
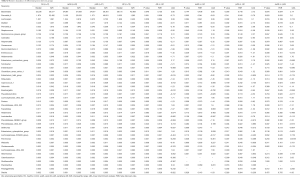
Full table
Further to identified more specific bacterial taxa associated with AS patients, we compared gut microbiota between patients and HCs using LEfSe analysis. We found that 7 genera including Prevotella_9, Dialister, Comamonas, Collinsella, Streptococcus, Alloprevotella and Prevotella_2 were significantly enriched in AS patients, while 4 genera including Eubacterium_ruminantium_group, Ruminococcus_gnavus_group, Lachnospira and Bacteroides were significantly enriched in HC (Figure 1E).
axAS and pAS specific microbial signature
To better understand the association of clinical phenotype and gut microbiota composition, we performed additional subgroup analyses. Compared with HCs, no significant difference was shown in either axAS or pAS in both Shannon and Simpson index (Figure 2A). Similarly, no difference was found between axAS and pAS patients in alpha diversity (Figure 2A). The PCoA based on the unweighted UniFrac distance analysis showed that patients with axAS or pAS were obvious separated from HCs (P=0.032 and P=0.016, respectively, Figure 2B), but no obvious separation was found between axAS and pAS (P=0.657, Figure 2B).
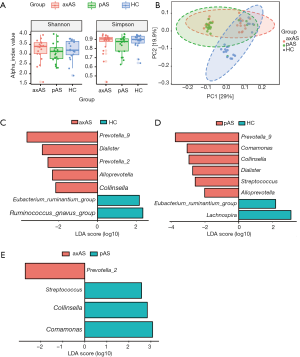
When come to the analysis of phylotypes, we found that Tenericutes showed significant lower abundance in both axAS and pAS subgroups in comparison to HC. While Actinobacteria only showed significant difference between pAS patients and HC, and Verrucomicrobia only showed significant difference between axAS patients and HC (Figure S2A). No phyla showed significant difference between patients with axAS and pAS. At genus level, 9 genera were identified with significantly different abundance between axAS and HC (FDR <0.05, Figure S2B, Table S2), while 31 genera were identified between pAS and HC (FDR <0.05, Figure S2C, Table S2). Thirteen genera were identified different between axAS and pAS (P<0.05), but only 1 genus still showed significant difference after correcting P with FDR (Table S2). Gut microbiome composition of pAS patients seemed to be more obviously deviated from HC, for more differential genera were identified in comparison to HC than that in axAS patients.
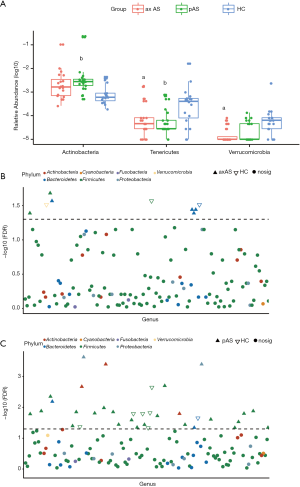
Also, we used LEfSe analysis to identified specific taxa that were associated with the subgroups of AS patients. Alloprevotella, Prevotella_9, Collinsella, Dialister and Eubacterium_ruminantium_group were identified as discriminative bacteria in both axAS and pAS patients when compared to those in HCs. However, differences of Prevotella_2 and Ruminococcus_gnavus_group were identified only in comparison axAS with HCs; differences of Streptococcus, Comamonas, and Lachnospira were identified only in comparison pAS with HCs. As expected, discriminative bacteria were identified between axAS and pAS subgroups. Between the two AS subgroups, Prevotella_2 was enriched in axAS patients, while Comamonas, Streptococcus and Collinsella were enriched in pAS patients (Figure 2C,D,E).
Microbiota-based predictive model for classification of AS
To determine whether the gut bacterial taxa can be regarded as identification biomarkers for distinguishing AS patients with different phenotype from HCs and from each other, random forest model was constructed to classify AS patients based on taxa at genus level. We carried out 10-fold cross-validation to evaluated the importance of indicator bacterial genera. The cross-validation error curve became stable when the 8 most relevant genera were used. Thus, we defined these 8 genera as biomarker taxa, including Alloprevotella, Acidominococcus, Holdemanella, Allisonella, Dialister, Collinsella, Streptococcus and Comamonas (Figure 3A). All these 8 genera were identified with significantly different abundance between AS patients and HCs with the above mentioned LEfSe analysis. We could accurately differentiate AS patients from HCs using this model, as the value of the AUC the ROC curve was up to 0.950. No difference was found when the predictive model was applied only to distinguish axAS patients or pAS patients from HCs (AUC =0.958 and 0.925, respectively; P=0.85; Figure 3B). However, we obtained poor performance when discriminating between axAS and pAS using the same method to construct a model based on the gut microbiota (data not shown).
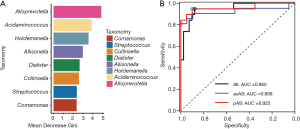
Discussion
In this study, we have demonstrated a clear distinction in microbiome profile between AS patients and healthy people, and for the first time to reveal the association with the clinical phenotypes of AS. Differential characteristics of gut microbiota could be used to accurately distinguish AS patients from healthy people.
Findings on species richness of gut microbiota in AS patients reported by different studies were inconsistent. We observed no significant difference in alpha diversity indexes between AS patients and HCs. Costello et al. (13) reported a higher diversity while Breban et al. (35) reported a decreased diversity in SpA patients. Despite this, our data showed consistent findings that composition of gut microbiota in AS patients was distinct from that in healthy people. This suggests that alteration of richness of species may not be the dominant feature in AS patients.
In the current study, 11 genera were identified to be associated with AS, including the enrichment of Prevotella and Dialister and the depletion of Bacteroides, which were also observed in the previous studies. For instance, Wen et al. found an increased abundance of Prevotella and decreased abundance of Bacteroide in AS patients (14) based on shotgun sequencing using gut microbial DNA from fecal samples of 211 Chinese individuals also shown alteration of gut microbiome in AS patients. And Tito et al. (36) reported an association of carriage of Dialister with disease activity in a study of 27 SpA patients (i.e., not necessarily AS) and 15 HCs using 16S rRNA profiling in ileal or colonic mucosal biopsies. In our study, we observed a decrease abundance of Ruminococcus_gnavus in AS patients, especially in patients with axAS. However, Breban et al. reported that SpA patients possess a decrease abundance in Ruminococcus_gnavus (35). Ruminococcus_gnavus was reported with an association with IBD in previous study (37). The different finding between our study and the Breban’s remains an open question because that there is difference in gut inflammation status between the two study cohorts. Comamonas, a possible pathogen that may cause intestinal infection such as appendicitis (38), showed a higher abundance in AS patients.
When come to the difference between axAS and pAS patients, overall construction of gut microbiome and alpha diversity were found similarly. In addition, a gut microbiota-based model shows low accuracy to classified the two subgroups of patients. Despite that, we found that Prevotella_2 was enriched in axAS patients, while Comamonas, Streptococcus and Collinsella were more enriched in pAS patients. Of note, these 4 genera show significant difference not only between AS patients and HC, but also between patients with axAS and pAS. Comamonas, Streptococcus and Collinsella are opportunistic pathogens that might trigger the proinflammatory factors and induce chronic inflammation. Patients with pAS were more common to suffer uveitis, psoriasis and IBD, which were also reported to be associated with gut dysbiosis. So, we suppose that SASP, a sulfa antimicrobial, may work by targeting these unfavorable bacteria. However, it needs to go further study.
During the last decade, AS has been considered as a subset of the broader entity referred to as which also includes non-radiographic axial SpA (axSpA). In the meantime, classification criteria for axSpA have been established with the intention of improving the sensitivity for an early diagnosis of AS and reducing diagnostic delay (39). However, the duration for diagnostic delay has not markedly improved (40) and there remains a high prevalence of undiagnosed axSpA in patients with chronic low back pain (41). One method to solve this problem is to find out new specific diagnostic biomarkers. Our study formulated a gut-microbial-based classification model including 8 genera with an accuracy of 0.95 for classification AS from healthy people. Wen et al. also reported a classifier with 35 microbial gene markers with an accuracy of 0.96. This suggested that the gut microbiota biomarkers may be helpful for improving the early diagnosis for AS. Yang et al. found Rifaximin (a gastrointestinal selective antibiotic) can reduce the inflammation activity by changing the gut microbiota composition with increased Bacteroidetes/Firmicutes phylum ratio, as well as selectively promoted some probiotic populations. This implicated that gut microbiota might not only be useful for diagnosis but also novel treatment target for AS. But it needs further study. A longitudinal study of gut microbiome in a multicenter and larger cohort with AS could be the next research step.
Anti tumor necrosis factor (TNF)-alpha biology and SASP, recommended for treatment of AS and IBD, were reported with an effect on alteration of gut microbiome in patients with IBD. In order to reduce the confounding influence as possible, we set up exclusion criteria to rule out patients who using anti TNF-alpha biology and SASP within 3 months before sample collection. In spite of this, we don’t know whether application of nonsteroidal anti-inflammatory drugs (NSAIDs) is driving the difference seen between AS patients and HCs for few of AS patient take any NSAIDs within 3 months before sample collection. This will require further study, such as the analysis of newly diagnosed and treatment naïve AS patients. Detailed dietary questionnaires have not been implemented in our study. None of the patients enrolled were following extreme dietary regimens such as strict vegan or vegetarian diets.
In conclusion, our study revealed specific alterations in the gut microbiomes in patients with different phenotypes of AS, and the classification model based on gut microbial features might provide a new direction for future clinical diagnosis. Further, discovery of the associated microbes of AS in the gut microbiome may help us to seek more treatments for this disease.
Acknowledgments
We gratefully acknowledge Professor Xuegong Zhang (MOE Key Laboratory of Bioinformatics and Bioinformatics Division, TNLIST/Department of Automation, Tsinghua, University), and Dr. Hao Zheng for the suggestion on data analysis.
Funding: This study was supported by The National Key Technology R&D Program of China (2014BA107B05), Major Program of Health Medical Collaborate Innovation of Guangzhou (201604020013), and General Program of National Natural Science Foundation of China (81571595).
Footnote
Conflicts of Interest: The authors have no conflicts of interest to declare.
Ethical Statement: The authors are accountable for all aspects of the work in ensuring that questions related to the accuracy or integrity of any part of the work are appropriately investigated and resolved. All participants gave written informed consent and the study was approved by the ethics committee of the Third Affiliated Hospital of Sun Yat-sen University (200740).
References
- Dougados M, Baeten D. Spondyloarthritis. Lancet 2011;377:2127-37. [Crossref] [PubMed]
- Brown MA, Kennedy LG, MacGregor AJ, et al. Susceptibility to ankylosing spondylitis in twins: the role of genes, HLA, and the environment. Arthritis Rheum 1997;40:1823-8. [Crossref] [PubMed]
- Manasson J, Shen N, Garcia Ferrer HR, et al. Gut microbiota perturbations in reactive arthritis and postinfectious spondyloarthritis. Arthritis Rheumatol 2018;70:242-54. [Crossref] [PubMed]
- Braun J, Sieper J. Ankylosing spondylitis. Lancet 2007;369:1379-90. [Crossref] [PubMed]
- Taurog JD, Chhabra A, Colbert RA. Ankylosing spondylitis and axial spondyloarthritis. N Engl J Med 2016;374:2563-74. [Crossref] [PubMed]
- Brewerton DA, Hart FD, Nicholls A, et al. Ankylosing spondylitis and HL-A 27. Lancet 1973;1:904-7. [Crossref] [PubMed]
- Yang M, Xu M, Pan X, et al. Epidemiological comparison of clinical manifestations according to HLA-B*27 carrier status of Chinese ankylosing spondylitis patients. Tissue Antigens 2013;82:338-43. [Crossref] [PubMed]
- Hammer RE, Maika SD, Richardson JA, et al. Spontaneous inflammatory disease in transgenic rats expressing HLA-B27 and human beta 2m: an animal model of HLA-B27-associated human disorders. Cell 1990;63:1099-112. [Crossref] [PubMed]
- Taurog JD, Richardson JA, Croft JT, et al. The germfree state prevents development of gut and joint inflammatory disease in HLA-B27 transgenic rats. J Exp Med 1994;180:2359-64. [Crossref] [PubMed]
- Lin P, Bach M, Asquith M, et al. HLA-B27 and human beta2-microglobulin affect the gut microbiota of transgenic rats. PLoS One 2014;9:e105684. [Crossref] [PubMed]
- Asquith MJ, Stauffer P, Davin S, et al. Perturbed mucosal immunity and dysbiosis accompany clinical disease in a rat model of spondyloarthritis. Arthritis Rheumatol 2016;68:2151-62. [Crossref] [PubMed]
- Crane AM, Bradbury L, van Heel DA, et al. Role of NOD2 variants in spondylarthritis. Arthritis Rheum 2002;46:1629-33. [Crossref] [PubMed]
- Costello ME, Ciccia F, Willner D, et al. Brief report: intestinal dysbiosis in ankylosing spondylitis. Arthritis Rheumatol 2015;67:686-91. [Crossref] [PubMed]
- Wen C, Zheng Z, Shao T, et al. Quantitative metagenomics reveals unique gut microbiome biomarkers in ankylosing spondylitis. Genome Biol 2017;18:142. [Crossref] [PubMed]
- de Winter JJ, Paramarta JE, de Jong HM, et al. Peripheral disease contributes significantly to the level of disease activity in axial spondyloarthritis. RMD Open 2019;5:e000802. [Crossref] [PubMed]
- Sutherland L, Macdonald JK. Oral 5-aminosalicylic acid for maintenance of remission in ulcerative colitis. Cochrane Database Syst Rev 2006.CD000544. [PubMed]
- Braun J, van den Berg R, Baraliakos X, et al. 2010 update of the ASAS/EULAR recommendations for the management of ankylosing spondylitis. Ann Rheum Dis 2011;70:896-904. [Crossref] [PubMed]
- van der Heijde D, Ramiro S, Landewe R, et al. 2016 update of the ASAS-EULAR management recommendations for axial spondyloarthritis. Ann Rheum Dis 2017;76:978-91. [Crossref] [PubMed]
- Chen J, Lin S, Liu C. Sulfasalazine for ankylosing spondylitis. Cochrane Database Syst Rev 2014.CD004800. [PubMed]
- Zheng H, Chen M, Li Y, et al. Modulation of gut microbiome composition and function in experimental colitis treated with sulfasalazine. Front Microbiol 2017;8:1703. [Crossref] [PubMed]
- van der Linden S, Valkenburg HA, Cats A. Evaluation of diagnostic criteria for ankylosing spondylitis. A proposal for modification of the New York criteria. Arthritis Rheum 1984;27:361-8. [Crossref] [PubMed]
- Machado PM, Landewe R, Heijde DV, et al. Ankylosing Spondylitis Disease Activity Score (ASDAS): 2018 update of the nomenclature for disease activity states. Ann Rheum Dis 2018;77:1539-40. [PubMed]
- Rudwaleit M, van der Heijde D, Landewe R, et al. The Assessment of SpondyloArthritis International Society classification criteria for peripheral spondyloarthritis and for spondyloarthritis in general. Ann Rheum Dis 2011;70:25-31. [Crossref] [PubMed]
- Walters W, Hyde ER, Berg-Lyons D, et al. Improved bacterial 16S rRNA gene (V4 and V4-5) and fungal internal transcribed spacer marker gene primers for microbial community surveys. mSystems 2015. [Crossref] [PubMed]
- Magoč T, Salzberg SL. FLASH: fast length adjustment of short reads to improve genome assemblies. Bioinformatics 2011;27:2957-63. [Crossref] [PubMed]
- Caporaso JG, Kuczynski J, Stombaugh J, et al. QIIME allows analysis of high-throughput community sequencing data. Nat Methods 2010;7:335-6. [Crossref] [PubMed]
- Quast C, Pruesse E, Yilmaz P, et al. The SILVA ribosomal RNA gene database project: improved data processing and web-based tools. Nucleic Acids Res 2013;41:D590-6. [Crossref] [PubMed]
- Edgar RC, Haas BJ, Clemente JC, et al. UCHIME improves sensitivity and speed of chimera detection. Bioinformatics 2011;27:2194-200. [Crossref] [PubMed]
- Haas BJ, Gevers D, Earl AM, et al. Chimeric 16S rRNA sequence formation and detection in Sanger and 454-pyrosequenced PCR amplicons. Genome Res 2011;21:494-504. [Crossref] [PubMed]
- Edgar RC. UPARSE: highly accurate OTU sequences from microbial amplicon reads. Nat Methods 2013;10:996-8. [Crossref] [PubMed]
- Wang Q, Garrity GM, Tiedje JM, et al. Naive Bayesian classifier for rapid assignment of rRNA sequences into the new bacterial taxonomy. Appl Environ Microbiol 2007;73:5261-7. [Crossref] [PubMed]
- Segata N, Izard J, Waldron L, et al. Metagenomic biomarker discovery and explanation. Genome Biol 2011;12:R60. [Crossref] [PubMed]
- Dhariwal A, Chong J, Habib S, et al. MicrobiomeAnalyst: a web-based tool for comprehensive statistical, visual and meta-analysis of microbiome data. Nucleic Acids Res 2017;45:W180-8. [Crossref] [PubMed]
- Zheng H, Wu H, Li J, et al. CpGIMethPred: computational model for predicting methylation status of CpG islands in human genome. BMC Med Genomics. 2013;6 Suppl 1:S13. [Crossref] [PubMed]
- Breban M, Tap J, Leboime A, et al. Faecal microbiota study reveals specific dysbiosis in spondyloarthritis. Ann Rheum Dis 2017;76:1614-22. [Crossref] [PubMed]
- Tito RY, Cypers H, Joossens M, et al. Brief report: dialister as a microbial marker of disease activity in spondyloarthritis. Arthritis Rheumatol 2017;69:114-21. [Crossref] [PubMed]
- Hall AB, Yassour M, Sauk J, et al. A novel Ruminococcus gnavus clade enriched in inflammatory bowel disease patients. Genome Med 2017;9:103. [Crossref] [PubMed]
- Bayhan Gİ, Tanır G, Karaman I, et al. Comamonas testosteroni: an unusual bacteria associated with acute appendicitis. Balkan Med J 2013;30:447-8. [Crossref] [PubMed]
- Rudwaleit M, Landewe R, van der Heijde D, et al. The development of Assessment of SpondyloArthritis international Society classification criteria for axial spondyloarthritis (part I): classification of paper patients by expert opinion including uncertainty appraisal. Ann Rheum Dis 2009;68:770-6. [Crossref] [PubMed]
- Sykes MP, Doll H, Sengupta R, et al. Delay to diagnosis in axial spondyloarthritis: are we improving in the UK? Rheumatology (Oxford) 2015;54:2283-4. [PubMed]
- Tant L, Delmotte N, Van den Enden M, et al. High Prevalence of Undiagnosed Axial Spondyloarthritis in Patients with Chronic Low Back Pain Consulting Non-Rheumatologist Specialists in Belgium: SUSPECT Study. Rheumatol Ther 2017;4:121-32. [Crossref] [PubMed]