Prognostic nomogram integrated baseline serum lipids for patients with non-esophageal squamous cell carcinoma
Introduction
Esophageal cancer (EC) is one of common causes of cancer death worldwide (1). EC is the 5th leading cancer in incidence and is ranked 4th for cancer-related mortality in China (2). The prognosis of EC is extremely poor because of the inability to detect the disease at an early stage (3). The predominant histological subtype of EC is esophageal squamous cell carcinoma (ESCC), which accounts for more than 90% of all cases in China (4). Non-esophageal squamous cell carcinoma (non-ESCC) is a rare subtype of EC, which is a rare disease. In addition, studies investigating the prognostic risk factors of non-ESCC are limited. Therefore, studying the prognosis for non-ESCC patients becomes a health problem that needs prompt solutions.
The serum lipid profile including total cholesterol (TC), triglycerides (TG), high-density lipoprotein cholesterol (HDL-C), low-density lipoprotein cholesterol (LDL-C), apolipoproteinA-I (ApoAI), and apolipoprotein B (ApoB). Lipids, as key components of the cellular membrane, as well as the metabolites of organisms, play important roles in processes, including cellular energy storage, structural composition, and signal transduction (5,6). In previous studies, it has been shown that an abnormal lipid metabolism was strongly associated with an increased risk of several types of cancer risk (7-10).
Nomograms have been used as reliable and pragmatic prediction tools to obtain individual risk by integrating some important factors for estimating prognosis in the outcomes of cancers. In addition, nomograms have been proven to provide more precise prediction compared with traditional TNM staging systems (11). In this study, we adopted nomograms to investigate the prognostic values of the serum lipid profile on overall survival (OS) and disease-free survival (DFS) in patients with non-ESCC, In addition, we further visualized it as nomogram for more convenient clinical practice, and then compared the prediction accuracy between prognostic nomograms and traditional TNM staging systems.
Methods
Patients and study design
Here, we performed a retrospective study of non-ESCC patients. Patients were collected at the Sun Yat-sen University Cancer Center (SYSUCC), Guangzhou, China. between January 2006 and December 2016. This study was approved by the Clinical Research Ethics Committee of the Sun SYSUCC, and all patients provided written informed consent at the first visit to our center. Patients included in the analysis met the following criteria: (I) non-ESCC diagnosis confirmed by histopathology, no malignancies except for non-ESCC; (II) patients who did not undergo anti-tumor therapy; (III) no cardiovascular disease, diabetes, and chronic hepatitis; (IV) data were collected one week before treatment.
Clinical information was collected from medical charts and records before treatment at the SYSUCC. Patients were classified according to 7th edition of the AJCC TNM staging guidelines. Clinical characteristics included gender, age, family history, alcohol consumption history, cancer location, histological type, TNM stage, and treatment. The prognostic markers included TC, TG, HDL-C, LDL-C, APOAI, APOB, AI, THR, LHR, and body mass index (BMI). The AI was calculated by the following formula: (TC-HDL-C)/HDL-C (12). The definition of THR was the ratio of TC to HDL-C, and LHR was the ratio of LDL-C to HDL-C.
Clinical outcome assessment and patient follow-up
The patients were followed up via clinic visits and telephone interviews. OS was calculated from the date of the first non-ESCC diagnosis to the date of death due to cancer or by patient censoring on the date of the last follow-up. DFS was defined as the date of the first non-ESCC diagnosis to the date of the first relapse at any site, death due to cancer, or the date of the last follow-up visit. All patients were followed up until death or April 2018 (end of follow-up).
Statistics
Categorical variables were classified based on clinical findings. AI and BMI were transformed into categorical variables based on routine cut-off values in the clinical application. The best cut-off values of other continuous variables were determined by X-tile (13). OS and DFS were estimated by the Kaplan-Meier survival analysis and were compared by using the log-rank test. Variables with a P value of ≤0.1 in univariate analysis were subjected to Cox proportional analysis. The predictive accuracy of the independent prognostic factors was evaluated adopting time-dependent receiving operative characteristics (ROC) curve. According to the results of Cox proportional analysis, prognostic nomogram for predicting OS and DFS were established and the predictive accuracy was measured by Harrell’s concordance index (C-index). The larger the C-index, the more accurate prognostic prediction (14), and validated using 1,000 bootstrap re-samplings. Comparisons between the prognostic nomogram and traditional TNM staging systems were evaluated using the C-index and decision curve analysis (15). Statistical analyses were performed using SPSS software, version 19.0 (SPSS Inc., Chicago, IL, USA) and R 3.4.4 software (Institute of Statistics and Mathematics, Vienna, Austria). P values of <0.05 were considered statistically significant.
Results
Characteristics of all patients
According to our selection criteria, a total of 180 patients were included in the retrospective study. One hundred and forty (77.8%) patients were male, and 40 (22.2%) were female; the median age was 56.3 (range, 24–80) years. The median follow-up for OS and DFS was 18 and 15 months respectively. Baseline characteristics of non-ESCC patients and subgroups were shown in Table 1.
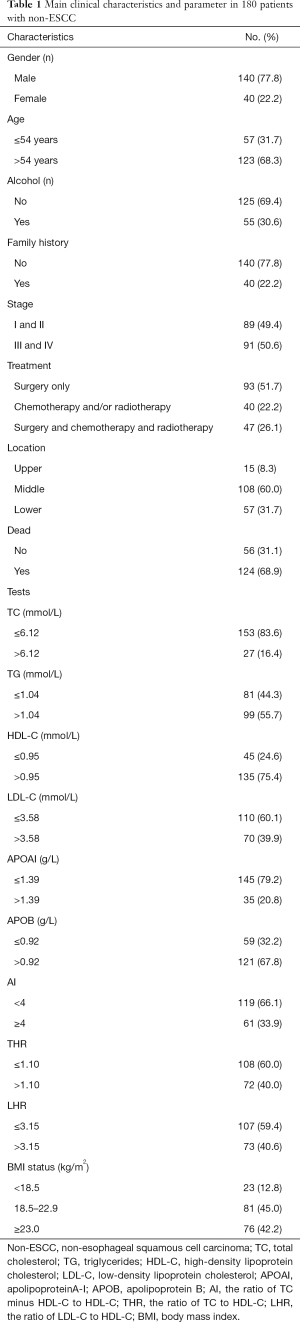
Full table
The prognostic factors impact on outcomes of non-ESCC
The results of univariate analysis and multivariate Cox hazards analysis were presented in Table 2. For OS, the univariate analysis demonstrated that age, cancer type, TNM stage, TC, TG, HDL-C, LDL-C, APOB, AI, THR, and LHR were associated with OS (P ≤0.1). In addition, multivariate Cox proportional analysis showed that cancer type [hazard ratio (HR) =0.62; 95% confidence interval (CI): 0.48–0.79; P<0.001], TNM stage (HR =2.00; 95% CI: 1.29–3.08; P=0.002), TC (HR =3.15; 95% CI: 1.63–6.10; P=0.001), and TG (HR =0.49; 95% CI: 0.29–0.83; P=0.009) were significant independent prognostic factors in non-ESCC patients. For DFS, the univariate analysis showed that cancer type, TNM stage, TC, TG, HDL-C, LDL-C, APOB, AI, THR, and LHR were associated with OS (P ≤0.1). But the multivariate analysis showed that only cancer type (HR =0.67; 95% CI: 0.53–0.85; P=0.001), TNM stage (HR =2.23, 95% CI: 1.47–3.39; P<0.001), TC (HR = 3.22, 95% CI: 1.71–6.06; P<0.001), TG (HR =0.48, 95% CI: 0.29–0.79; P=0.004), and LDL-C (HR =0.53, 95% CI: 0.28–0.99; P=0.045) were significantly independently associated with DFS. A forest plot was created to shows the hazard ratios and 95% confidence intervals for DFS and OS according to the Cox proportional hazards regression analysis (Figure 1). In line with these findings, the Kaplan-Meier curves for OS and DFS according to cancer type, TNM stage, TC, TG and LDL-C levels were significantly different, as confirmed by the log-rank test (Figure 2). Furthermore, the results of time-dependent ROC curve for OS showed that AUCs of cancer type and TG were higher than TNM stage (Figure 3A). For DFS, data showed that the AUCs of cancer type, TG, and LDL-C were higher than the TNM stage (Figure 3B).
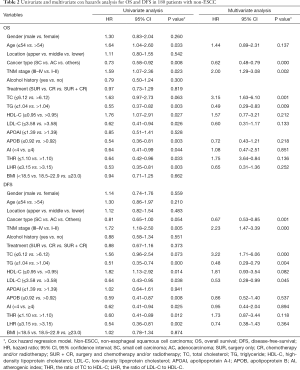
Full table
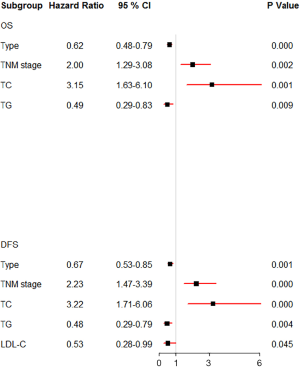
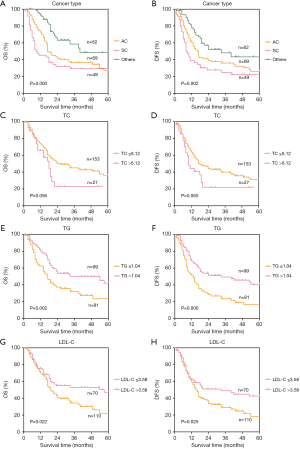
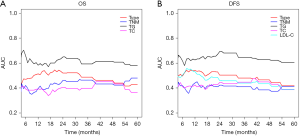
Prognostic nomograms for prediction of OS and DFS
The resulting variables from the Cox proportional analysis were used to build the prognostic nomograms for OS and DFS (Figure 4). The prognostic factors of nomogram for OS included four risk factors, including cancer type, TNM, TC, and TG. In addition, the nomogram for DFS included five risk factors (cancer type, TNM, TC, TG, and LDL-C). Each prognostic factor within the nomogram was assigned a point. By sum of the total points from all variables combined with the location at the total point scale allowed us to obtain the probabilities of the outcomes by drawing a vertical line towards the axis labeled “1-, 3- and 5-Year Overall Survival/Disease-Free Survival Probability”.
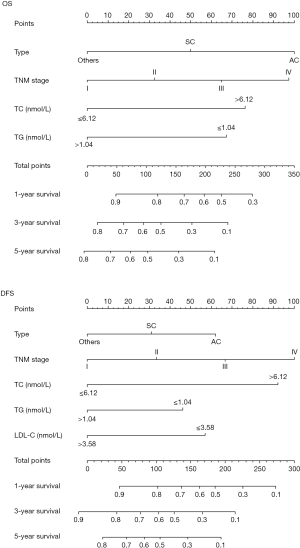
Comparison of predictive accuracy for OS and DFS between nomogram and staging systems
As shown in Table 3, our prognostic nomograms displayed better accuracy than TNM stage in predicting both OS and DFS in non-ESCC patients. The C-index of the nomograms for OS was 0.69 (95% CI: 0.64–0.74), which was significantly higher than that of the TNM stage (0.58; 95% CI: 0.53–0.64; P=0.005). For DFS, the C-index of the nomograms was 0.70 (95% CI: 0.64–0.75), which was also significantly higher than that of the TNM stage (0.60; 95% CI: 0.55–0.65; P=0.001). In addition, decision curve analysis showed that both the predictive accuracy of prognostic nomograms for OS and DFS were better than the TNM stage (Figure 5).
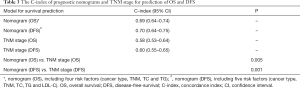
Full table
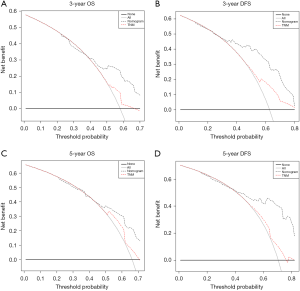
Discussion
In our study, we investigated the prognostic values of serum lipids and clinical characteristics in non-ESCC patients. Based on the results of Cox hazards analysis, we established nomograms predicting OS and DFS in non-ESCC patients, which showed better predictive accuracy than traditional TNM staging systems. By doing this, we could assess the prognosis risk of each patient, and provided individual tailored post-treatment. To our knowledge, this study was the first retrospective analysis to investigate the prognostic roles of pretreatment of serum lipids in non-ESCC patients.
The TNM stage was the most common tool used in prognostic and guiding treatment options of many cancers. However, this system had some controversies, because it was only based on the anatomical extent of cancers, which was not adequate for prognosis without taking into account other prognostic biomarkers (16,17). Moreover, in this study, we integrated TNM stage with baseline serum lipids to predict both OS and DFS in non-ESCC patients, which displayed better accuracy compared to the TNM stage.
Lipids were components of biological membranes, and played several important roles in energy storage and cellular signalling. Abnormal lipid metabolism can affect cell growth, proliferation, differentiation, and motility (18). Fatty acid synthase (FASN) played a crucial role in epithelial-mesenchymal transition (EMT), which had been shown to be closely related to cancer development and metastasis (19). In several studies, high expression of FASN had reported in breast cancer, prostate cancer, ovarian cancer, and colorectal cancer (20-22). In the present study, we confirmed that the serum lipids of TC, TG, and LDL-C were significantly associated with the mortality of non-ESCC patients. These results were similar with previous reports. Cholesterol played an important role in cellular structure and function, especially in the synthesis of steroid hormones, and an abnormal cholesterol biosynthesis might contribute to tumor growth and progression (23). Cholesterol had been shown to modulate several proteins implicated in key cellular signaling pathways to alter the cytoskeleton, cell polarity, and angiogenesis, thereby leading to malignant transformation (24-28). Several studies had reported that serum TC levels were related with the prognosis in lung cancer, breast cancer, prostate cancer, and colorectal cancer (29,30). Our results showed that non-ESCC patients with high preoperative serum TC levels (TC >6.12 mmol/L) had a shorter DFS and OS than the non-ESCC patients with serum TC levels ≤6.12 mmol/L). TG acted as an energy source for neoplastic cells, which could promote cell proliferation and tumor growth (31,32). Moreover, several studies reported that the levels of serum TG were associated with lung cancer, thyroid cancer, rectal cancer, breast cancer, non-Hodgkin’s lymphoma, and prostate cancer (9,33). Both DFS and OS of patients with TG levels ≤1.04 mmol/L were significantly shorter than the patients with TG >1.04 mmol/L. LDL and the LDL receptor (LDLR) were prognostic indexes for survival in patients with small cell lung cancer (34). Ox-LDL receptor 1 (OLR1) could activate nuclear factor-κB (NF-κB) target genes, leading to proliferation, migration, and inhibition of apoptosis and de novo lipogenesis genes (35). In our study, we found that LDL-C was an independent prognostic factor for DFS in non-ESCC patients. However, for OS, this phenomenon was not observed. Other prognostic factors, such as HDL-C, APOAI, APOB, AI, THR, and LHR were not independent prognostic factor based on the multivariate analysis, which maybe the because of the difference in tumor types, research population, and cut-off values leading to different results.
This study had several limitations. First, this study was a retrospective analysis, so there may be a potential source for selection bias. Second, this was a single-center study of a limited number of patients. Third, this study only focused on the prognostic values of serum lipids, other prognostic factors, such as inflammation-based prognostic markers (36-38), molecular biomarkers (39-41), and coagulation (42,43) were not included. Thus, future validation of our findings in a larger population across multiple centers was warranted.
Conclusions
Overall, we established predictive nomograms based on the pretreatment of serum lipids for OS and DFS in non-ESCC patients, which showed that the predictive accuracy was better than traditional TNM staging system. It could as practical tools for individualized prognostication in clinical medicine.
Acknowledgments
None.
Footnote
Conflicts of Interest: The authors have no conflicts of interest to declare.
Ethical Statement: The authors are accountable for all aspects of the work in ensuring that questions related to the accuracy or integrity of any part of the work are appropriately investigated and resolved. The ethics of study was approved by the Clinical Research Ethics Committee of the Sun Yat-sen University Cancer Center (No. GZR2015-015) and written informed consent was obtained from all patients.
References
- Siegel RL, Miller KD. PhD AJD. Cancer statistics, 2018. CA Cancer J Clin 2018;68:277-300.
- Chen W, Zheng R, Zeng H, et al. Annual report on status of cancer in China, 2011. Chin J Cancer Res 2015;27:2-12. [Crossref] [PubMed]
- Mccormack VA, Menya D, Munishi MO, et al. Informing etiologic research priorities for squamous cell esophageal cancer in Africa: A review of setting-specific exposures to known and putative risk factors. Int J Cancer 2017;140:259-71. [Crossref] [PubMed]
- Law S, Wong J. Changing disease burden and management issues for esophageal cancer in the Asia-Pacific region. J Gastroenterol Hepatol 2002;17:374-81. [Crossref] [PubMed]
- Grunt TW. Interacting Cancer Machineries: Cell Signaling, Lipid Metabolism, and Epigenetics. Trends Endocrinol Metab 2018;29:86-98. [Crossref] [PubMed]
- Beloribi-Djefaflia S, Vasseur S, Guillaumond F. Lipid metabolic reprogramming in cancer cells. Oncogenesis 2016;5:e189. [Crossref] [PubMed]
- Fiorenza AM, Branchi A, Sommariva D. Serum lipoprotein profile in patients with cancer. A comparison with non-cancer subjects. Int J Clin Lab Res 2000;30:141-5. [Crossref] [PubMed]
- Jiang J, Nilsson-Ehle P, Xu N. Influence of liver cancer on lipid and lipoprotein metabolism. Lipids Health Dis 2006;5:4. [Crossref] [PubMed]
- Li X, Tang H, Wang J, et al. The effect of preoperative serum triglycerides and high-density lipoprotein-cholesterol levels on the prognosis of breast cancer. Breast 2017;32:1-6. [Crossref] [PubMed]
- Jiang SS, Weng DS, Jiang L, et al. The clinical significance of preoperative serum cholesterol and high-density lipoprotein-cholesterol levels in hepatocellular carcinoma. J Cancer 2016;7:626-32. [Crossref] [PubMed]
- Chen S, Lai Y, He Z, et al. Establishment and validation of a predictive nomogram model for non-small cell lung cancer patients with chronic hepatitis B viral infection. J Transl Med 2018;16:116. [Crossref] [PubMed]
- Zhang M, Bi LF, Fang JH, et al. Beneficial effects of taurine on serum lipids in overweight or obese non-diabetic subjects. Amino Acids 2004;26:267-71. [Crossref] [PubMed]
- Camp RL, Dolledfilhart M, Rimm DL. X-tile: a new bio-informatics tool for biomarker assessment and outcome-based cut-point optimization. Clin Cancer Res 2004;10:7252-9. [Crossref] [PubMed]
- Harrell FE Jr, Lee KL, Califf RM, et al. Regression modelling strategies for improved prognostic prediction. Stat Med 1984;3:143-52. [Crossref] [PubMed]
- Vickers AJ, Elkin EB. Decision curve analysis: a novel method for evaluating prediction models. Med Decis Making 2006;26:565-74. [Crossref] [PubMed]
- Li J, Chen S, Peng S, et al. Prognostic nomogram for patients with Nasopharyngeal Carcinoma incorporating hematological biomarkers and clinical characteristics. Int J Biol Sci 2018;14:549-56. [Crossref] [PubMed]
- Tang LQ, Li CF, Li J, et al. Establishment and Validation of Prognostic Nomograms for Endemic Nasopharyngeal Carcinoma. J Natl Cancer Inst 2015;108:djv291. [Crossref] [PubMed]
- Santos CR, Schulze A. Lipid metabolism in cancer. FEBS J 2012;279:2610-23. [Crossref] [PubMed]
- Jiang L, Wang H, Li J, et al. Up-regulated FASN expression promotes transcoelomic metastasis of ovarian cancer cell through epithelial-mesenchymal transition. Int J Mol Sci 2014;15:11539-54. [Crossref] [PubMed]
- Menendez JA, Lupu R. Fatty acid synthase and the lipogenic phenotype in cancer pathogenesis. Nat Rev Cancer 2007;7:763-77. [Crossref] [PubMed]
- Milgraum LZ, Witters LA, Pasternack GR, et al. Enzymes of the fatty acid synthesis pathway are highly expressed in in situ breast carcinoma. Clin Cancer Res 1997;3:2115-20. [PubMed]
- Chavarro JE, Kenfield SA, Stampfer MJ, et al. Blood levels of saturated and monounsaturated fatty acids as markers of de novo lipogenesis and risk of prostate cancer. Am J Epidemiol 2013;178:1246-55. [Crossref] [PubMed]
- Murai T. Cholesterol lowering: role in cancer prevention and treatment. Biol Chem 2015;396:1-11. [Crossref] [PubMed]
- Lingwood D, Simons K. Lipid rafts as a membrane-organizing principle. Science 2010;327:46-50. [Crossref] [PubMed]
- Baumann P, Thiele W, Cremers N, et al. CD24 interacts with and promotes the activity of c-src within lipid rafts in breast cancer cells, thereby increasing integrin-dependent adhesion. Cell Mol Life Sci 2012;69:435-48. [Crossref] [PubMed]
- Lee BH, Taylor MG, Robinet P, et al. Dysregulation of cholesterol homeostasis in human prostate cancer through loss of ABCA1. Cancer Res 2013;73:1211-8. [Crossref] [PubMed]
- Michalaki V, Koutroulis G, Syrigos K, et al. Evaluation of serum lipids and high-density lipoprotein subfractions (HDL2, HDL3) in postmenopausal patients with breast cancer. Mol Cell Biochem 2005;268:19-24. [Crossref] [PubMed]
- Mandal CC, Rahman MM. Targeting Intracellular Cholesterol is a Novel Therapeutic Strategy for Cancer Treatment. J Cancer Sci Ther 2014;6:510-3. [Crossref] [PubMed]
- Li JR, Zhang Y, Zheng JL. Decreased pretreatment serum cholesterol level is related with poor prognosis in resectable non-small cell lung cancer. Int J Clin Exp Pathol 2015;8:11877-83. [PubMed]
- Muka T, Kraja B, Ruiter R, et al. Dietary polyunsaturated fatty acids intake modifies the positive association between serum total cholesterol and colorectal cancer risk: the Rotterdam Study. J Epidemiol Community Health 2016;70:881-7. [Crossref] [PubMed]
- McKeown-Eyssen G. Epidemiology of colorectal cancer revisited: are serum triglycerides and/or plasma glucose associated with risk? Cancer Epidemiol Biomarkers Prev 1994;3:687-95. [PubMed]
- Saavedra-García P, Nichols K, Mahmud Z, et al. Unravelling the role of fatty acid metabolism in cancer through the FOXO3-FOXM1 axis. Mol Cell Endocrinol 2018;462:82-92. [Crossref] [PubMed]
- Ulmer H, Borena W, Rapp K, et al. Serum triglyceride concentrations and cancer risk in a large cohort study in Austria. Br J Cancer 2009;101:1202-6. [Crossref] [PubMed]
- Zhou T, Zhan J, Fang W, et al. Serum low-density lipoprotein and low-density lipoprotein expression level at diagnosis are favorable prognostic factors in patients with small-cell lung cancer (SCLC). BMC cancer 2017;17:269. [Crossref] [PubMed]
- Khaidakov M, Mitra S, Kang BY, et al. Oxidized LDL receptor 1 (OLR1) as a possible link between obesity, dyslipidemia and cancer. PloS one 2011;6:e20277. [Crossref] [PubMed]
- Hirahara N, Tajima Y, Fujii Y, et al. A Novel Prognostic Scoring System Using Inflammatory Response Biomarkers for Esophageal Squamous Cell Carcinoma. World J Surg 2018;42:172-84. [Crossref] [PubMed]
- Reeh M, Ghadban T, Uzunoglu FG, et al. Hamburg-Glasgow classification: preoperative staging by combination of disseminated tumour load and systemic inflammation in oesophageal carcinoma. Br J Cancer 2017;117:612-8. [Crossref] [PubMed]
- Wang L, Wang C, Wang J, et al. A novel systemic immune-inflammation index predicts survival and quality of life of patients after curative resection for esophageal squamous cell carcinoma. J Cancer Res Clin Oncol 2017;143:2077-86. [Crossref] [PubMed]
- Kang K, Huang YH, Li HP, et al. Expression of UCA1 and MALAT1 long-chain non-coding RNAs in esophageal squamous cell carcinoma tissues is predictive of patient prognosis. Arch Med Sci 2018;14:752-9. [PubMed]
- Mao Y, Fu Z, Zhang Y, et al. A seven-lncRNA signature predicts overall survival in esophageal squamous cell carcinoma. Sci Rep 2018;8:8823. [Crossref] [PubMed]
- Jiang S, Zhang Q, Su Y, et al. Network-Based Differential Analysis to Identify Molecular Features of Tumorigenesis for Esophageal Squamous Carcinoma. Molecules 2018;23:E88. [Crossref] [PubMed]
- Tan Z, Zhang M, Han Q, et al. A novel blood tool of cancer prognosis in esophageal squamous cell carcinoma: the Fibrinogen/Albumin Ratio. J Cancer 2017;8:1025-9. [Crossref] [PubMed]
- Ghanim B, Hoda MA, Klikovits T, et al. Circulating fibrinogen is a prognostic and predictive biomarker in malignant pleural mesothelioma. Br J Cancer 2014;110:984-90. [Crossref] [PubMed]