Tumor mutational and indel burden: a systematic pan-cancer evaluation as prognostic biomarkers
Introduction
Cancer originates from gene mutations (1). Particular gene mutations can be both predictive to treatment response and prognostic for survival (2,3). The total number of mutations is named tumor mutational burden (TMB), which was defined as the number of non-synonymous somatic, coding, base substitution, and indel mutations per megabase (Mb) of genome examined (4). Nowadays, whole-exome sequencing is considered the gold standard to determine the TMB, and estimating TMB by targeted next-generation sequencing (NGS) panels has been promoted as a simpler and cheaper option (5).
The adaptive immune system detects and identifies tumors by non-self neoantigens that arise as the result of somatic mutations. Thus, it is reasonable to hypothesize that the number of non-synonymous somatic mutations in a tumor, i.e. TMB, may affect the odds of generating immunogenic peptides and thereby influence immune checkpoint inhibitors (ICIs) response in patients (6). Therefore, in the booming era of immuno-oncology, TMB has been widely studied as the predictor of response to ICIs (4,7,8). Its predictive function has been tested retrospectively or prospectively in melanoma, non-small-cell lung cancer, small cell lung cancer and bladder cancer (8-11). What’s more, Turajlic and colleagues found that frameshift indels had a more significant association with ICIs response than TMB across three separate melanoma cohorts, indicating that indel burden might be a better biomarker to ICIs (12). However, for clinical application, TMB has yet to address several pitfalls in order to become a reliable predictive biomarker to ICIs. Before establishing the ability of TMB to predict ICI response and survival benefit, its general prognostic impact on overall survival (OS) should be clarified (13), which has never been investigated systematically due to the lack of matched and high-quality data.
The recently completed The Cancer Genome Atlas (TCGA) project provides matched molecular and clinical data (14), which makes it possible to systematically analyze the survival impact of TMB and indel burden. Therefore, we conducted this pan-cancer analysis to evaluate the general prognostic impact of TMB and indel burden in patients newly diagnosed with cancer.
Methods
Patients
For a decade, the TCGA program collected clinicopathologic annotation data together with multi-platform molecular profiles of more than 11,000 human tumors across 33 different cancer types (15). A standardized dataset named the TCGA Pan-Cancer Clinical Data Resource was developed by Liu and colleagues (15) (https://doi.org/10.1016/j.cell.2018.02.052), from which the curated and filtered clinical and survival outcome data with high quality were obtained. Curated and filtered somatic mutation data, which could be readily used for pan-cancer analysis, were obtained from the Multi-Center Mutation Calling in Multiple Cancers (MC3) project (https://gdc.cancer.gov/about-data/publications/mc3-2017), which generated a comprehensive encyclopedia of somatic mutation calls from the TCGA data by Ellrott and colleagues (16). We retrieved all records from 11,160 patients. The exclusion criteria were as follows: (I) patients with incomplete survival data and follow-up information; (II) patients without somatic mutation information.
Within these 33 cancer types, 10 cancer types (diffuse large B-cell lymphoma, pheochromocytoma and paraganglioma, testicular germ cell tumors, thymoma, breast invasive carcinoma, kidney chromophobe, lower grade glioma, prostate adenocarcinoma, rectum adenocarcinoma and thyroid carcinoma) were excluded because the number of death events was too small for OS analysis, as determined by Liu et al. (15). For glioblastoma multiforme, acute myeloid leukemia and sarcoma, no stage information or other crucial confounding factors were available in the TCGA Pan-Cancer Clinical Data Resource, which might substantially compromise the reliability of the prognostic analysis of TMB; thus these three cancer types were also not included in this pan-cancer analysis. Eventually, adrenocortical carcinoma (ACC), bladder urothelial carcinoma (BLCA), cervical squamous cell carcinoma and endocervical adenocarcinoma (CESC), cholangiocarcinoma (CHOL), colon adenocarcinoma (COAD), esophageal carcinoma (ESCA), head and neck squamous cell carcinoma (HNSC), kidney renal clear cell carcinoma (KIRC), kidney renal papillary cell carcinoma (KIRP), liver hepatocellular carcinoma (LIHC), lung adenocarcinoma (LUAD), lung squamous cell carcinoma (LUSC), mesothelioma (MESO), ovarian serous cystadenocarcinoma (OV), pancreatic adenocarcinoma (PAAD), skin cutaneous melanoma (SKCM), stomach adenocarcinoma (STAD), uterine corpus endometrial carcinoma (UCEC), uterine carcinosarcoma (UCS) and uveal melanoma (UVM) were all included in the current analysis.
Variables
Examined clinicopathological variables included age, sex, race, stage, and year of initial pathologic diagnosis. Race was divided into white, black, and other ethnicity. Age and year of initial pathologic diagnosis were both retained as continuous variables. The AJCC staging system was adopted for most cancer types, except for CESC, OV, UCEC, and UCS, for which clinical stages were adopted.
TMB and indel burden
We used the uniform somatic called variants determined by TCGA MC3 project, which were comprehensively curated from detection using seven methods (MuTect, MuSE, VarScan2, Radia, Pindel, Somatic Sniper, Indelocator). TMB was defined as the number of non-synonymous somatic, coding, base substitution, and indel mutations per megabase (Mb) of genome examined (4), while indel burden consisted of frameshift small insertions and deletions, which created a novel open reading frame and could produce a large quantity of neoantigenic peptides highly distinct from self (17). We used 38 Mb as the estimate of the exome size (18). For the two FDA-authorized or approved panels, the MSK-IMPACT panel and the FoundationOne CDx panel, the coding region captured covers 1.22 Mb and 1.1 Mb, respectively (18,19). The indel burden was calculated as the absolute frameshift indel count per case.
Statistical analysis
The primary outcome of this study was OS. OS was defined as the time from diagnosis to the date of death. Patients who were still alive at the follow-up cut-off date were treated as censored observations. Although retrospective analyses have showed the predictive function of high TMB for a better response to ICIs, the optimal cutpoint to define high TMB varied among studies (20). Therefore, for each cancer type, we varied the threshold of TMB from the 50th to 90th percentiles, and selected the one that yielded the highest statistical significance level to define the TMB-high (TMB > threshold) and TMB-low (TMB ≤ threshold) subsets (21). Associations between TMB and patient demographic characteristics were assessed using Pearson χ2 or Fisher’s exact test for categorical data and the Wilcoxon rank sum test for ordinal and continuous data. Inverse probability of treatment weight (IPTW)-based analysis was adopted to evaluate the impact of TMB on OS. Propensity score of TMB was generated from a multivariable logistic regression model for the association between baseline covariates and TMB. The logistic model was constructed via stepwise variable selection, with a threshold of P<0.20 required for initial inclusion and P<0.10 required to remain in the model. On the basis of the propensity score, IPTW was calculated for each patient (22). The impact of TMB on OS was then evaluated using univariate analysis based on the Kaplan-Meier estimator and Cox regression weighted by IPTW (22). Similar analyses were performed to evaluate the impact of indel burden on OS. The nominal level of significance was set at 0.05. Statistical analyses were performed using R v. 3.5.1 (http://www.r-project.org).
Results
Figure 1 depicted the design of this pan-cancer analysis. We obtained curated and filtered somatic mutation data from the Multi-Center Mutation Calling in Multiple Cancers (MC3) project (16), and clinical and survival outcome data from the TCGA Pan-Cancer Clinical Data Resource (15). In total, 6,035 eligible patients from 20 different cancer types were enrolled in this study. The TMB across 20 cancer types are presented in Figure 2A. The median TMB ranged from 0.34 mutations per megabase (mut/Mb) to 13.09 mut/Mb, with SKCM having the highest median TMB while UVM had the lowest. The median number of indels ranged from 0 to 9, UVM still had the lowest median number of indels, but LUSC other than SKCM had the highest (Figure 2B). The baseline patient characteristics for each cancer type are shown in Table 1.
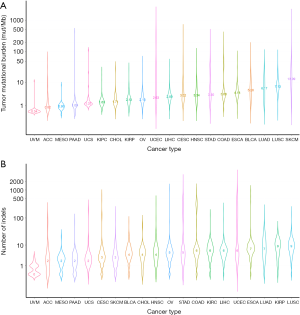
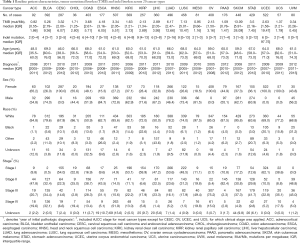
Full table
For each cancer type, we varied the threshold of TMB from the 50th to 90th percentiles, and the one that yielded the highest statistical significance level was selected (21) (Tables S1-S10). As shown in Tables S1-S10, clinicopathologic factors were not equally distributed between TMB-high and TMB-low patients across different cancer types. And TMB-high patients tended to be older, with statistically significant differences observed in eight out of 20 cancer types.

Full table
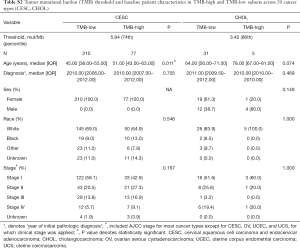
Full table
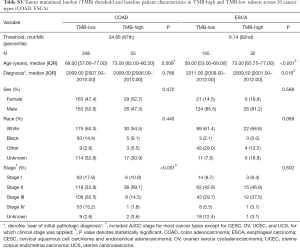
Full table
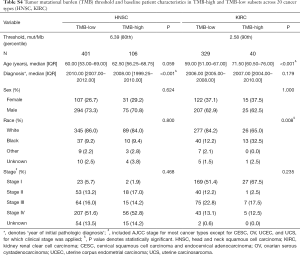
Full table
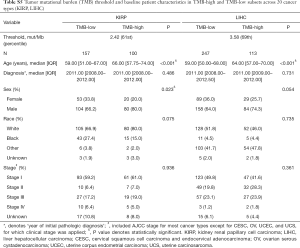
Full table
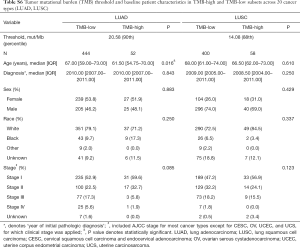
Full table
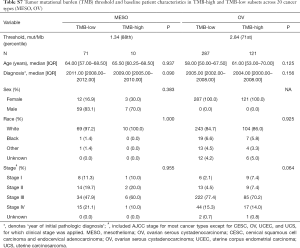
Full table
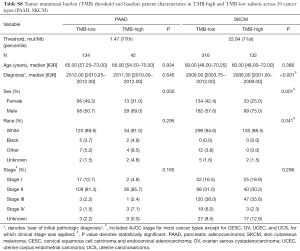
Full table
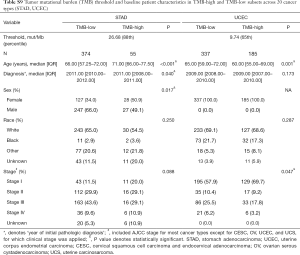
Full table
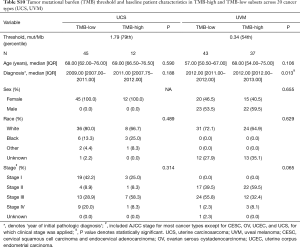
Full table
To mitigate against potential bias caused by unbalanced variables between the two subsets, we employed the IPTW method for bias adjustment. After being weighted by IPTW, we found that the impact of TMB on OS was different across cancers. These 20 cancer types could be classified into three distinct groups, namely the TMB-Worse, TMB-Better and TMB-Similar groups. Eight cancer types, ACC, CHOL, COAD, ESCA, KIRC, LIHC, MESO and PAAD were included in the TMB-Worse group, in which patients with high TMB had a poorer prognosis compared with those with low TMB (Figure 3A,B,C,D,E,F,G,H, all P<0.05). However, in the TMB-Better group, which included BLCA, KIRP, STAD, CESC, OV and UCEC, high TMB was a statistically significant prognostic indicator of decreased mortality (Figure 3I,J,K,L,M,N, all P<0.05). In the TMB-Similar group, TMB did not have a significant impact on OS (Figure 3O,P,Q,R,S,T, all P>0.05).
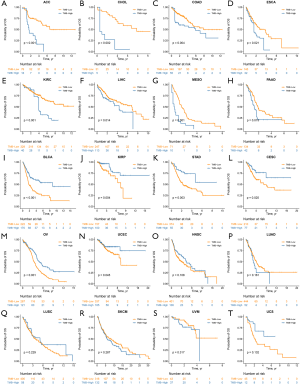
Hazard ratios (HRs) based on IPTW-weighted Cox proportional hazards models were shown in Figure 4A. In the TMB-Worse group, CHOL had the highest HR =6.10 [95% confidence interval (CI), 1.91–19.46, P=0.002], followed by ACC, MESO, KIRC, COAD, ESCA, PAAD and LIHC. In the TMB-Better group, KIRP had the lowest HR =0.34 (95% CI, 0.16–0.70, P=0.004), followed by STAD, BLCA, CESC, OV and UCEC. Trends toward better outcome of TMB-low patients were observed in some cancer types of the TMB-Similar group such as LUAD, SKCM and UVM.
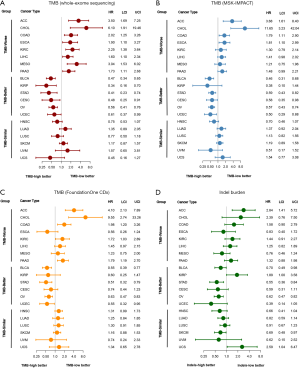
When the analysis was limited to data from only those genes included in the FDA-authorized MSK-IMPACT panel (Figure 4B) or the FDA-approved FoundationOne CDx panel (Figure 4C) used in routine clinical practice, TMB remained significantly associated with OS in the majority of cancer types in the TMB-Worse and TMB-Better groups, with only numerical survival differences captured in several cancer types.
Indel burden also had divergent prognostic impact in different cancer types, but differences from that of TMB existed, with six out of 20 cancer types in which the survival impact was significant (Figure 4D). Notably, indel burden significantly affected OS in two out of six cancer types in the TMB-Similar group. In UCS, patients with high indel burden had significantly worse prognosis than those with low indel burden, while in SKCM, high indel burden were prognostic of better survival (Figure S1).
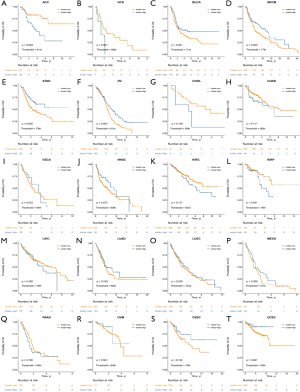
Discussion
In this pan-cancer analysis, we found that both TMB and indel burden had divergent survival impacts in different cancer types. In ACC, CHOL, COAD, ESCA, KIRC, LIHC, MESO and PAAD, high TMB was strongly associated with inferior survival; whereas in BLCA, KIRP, STAD, CESC, OV and UCEC, patients with high TMB had superior prognosis compared with those with low TMB. In the other six cancer types, HNSC, LUAD, LUSC, SKCM, UVM and UCS, TMB did not significantly impact the survival of patients newly diagnosed with cancer. Interestingly, in two out of these six cancer types, indel burden significantly impacted OS, although it did not impact OS in all the other 14 cancer types as TMB did.
From palliative chemotherapy to neoadjuvant chemotherapy, the extensive indications of ICIs have created a new era of immuno-oncology (23). Consequently, the predictive value of TMB and indel burden is considerable to help to identify patients who will derive the greatest therapeutic benefit (24). However, there appears to be groupthink to rush TMB for approval by the US FDA and widespread use in practice despite of several unsettled pitfalls, such as its general prognostic impact (13). Samstein and colleagues showed that “Tumor mutational load predicts survival after immunotherapy across multiple cancer types” (25). Meanwhile, as they evaluated the predictive function of TMB using single-arm data, they additionally investigated the general prognostic impact of TMB in several tumor entities, but failed to reveal significant survival differences between TMB-high and TMB-low patients in the majority of entities examined. However, the number of patients of each tumor entities in the non-ICI-treated cohort they studied was relatively small, and some entities were merged together, which might lack power to discover significant survival differences. What’s more, the recent announced results of Checkmate-227 investigating nivolumab plus ipilimumab versus chemotherapy failed to establish the predictive function of TMB (26). Therefore, we believe that it is of great importance to thoroughly investigate the general prognostic impact of TMB in patients newly diagnosed with cancer using the high-quality and matched data from TCGA. The results of this study showed that TMB has divergent survival impact in different cancer types, which should be seriously taken into account when establishing the predictive function of TMB to ICIs. For instance, for cancer types in the TMB-Better group, we should be cautious when we observed better survival in the TMB-high group than that in the TMB-low group in ICI-treated cohort, which might be only due to the general prognostic impact of TMB and unrelated to ICIs. Whereas for cancer types in the TMB-Worse and TMB-Similar group, if significant survival benefit was found in the TMB-high group versus TMB-low group, we could confidently claim the predictive function of TMB to ICIs. Our findings also suggest that an optimized genomic classifier incorporating TMB information is likely to improve prognostication, risk stratification, and management in the majority (14/20) of the examined cancer types.
Previous studies of the survival impact of TMB have focused on several specific cancer types. Li and colleagues have found that the MUC16 mutation was associated with high TMB and subsequently better prognosis in STAD, which is consistent with our finding (27). While in microsatellite stable metastatic colorectal cancer patients receiving first-line chemotherapy plus targeted therapy, TMB-high was found to be associated with favorable OS (28), which seemed to be in conflict with our results. We assumed that microsatellite stable metastatic colorectal cancer patients with high TMB might be more sensitive to chemotherapy plus targeted therapy, thus TMB-high should probably be a predictive biomarker to chemotherapy plus targeted therapy rather than a general prognostic factor, because Samstein et al. also found that TMB-high was associated with worse OS in patients with colorectal cancer (25). But still, further data are required to confirm this issue. On the other hand, in non-small-cell lung cancer, Owada-Ozaki and colleagues found that high TMB was associated with poor prognosis in patients with completely resected non-small cell lung cancer (29). In contrast, results from pooled analysis of the LB2 study suggested that high TMB was associated with better prognosis in patients with resected non-small-cell lung cancer (30). The population characteristics are similar in the aforementioned two studies except for race and sample size, which could not account for the conflicting conclusions. Our analysis showed that TMB was associated with prognosis neither in patients with LUSC nor in patients with LUAD, indicating that further investigations considering specific confounders such as histology and driver gene mutations are needed. In addition, Hwang and colleagues found that high TMB resulted in inferior OS in patients with neuroblastoma, a cancer type that has not been included in the TCGA (31). Except for the aforementioned cancer types, there is no study on the survival impact of TMB in patients with other cancer types. For the first time, our pan-cancer analysis provides key information and data to address these issues.
In addition to TMB, indel burden is also an emerging biomarker for ICIs treatment. Based on the hypothesis that frameshift indels might be an ideal source of tumor-derived neoantigens and so induce multiple neoantigen reactive T cells, Turajlic and colleagues further found that frameshift indels had more significant association with ICIs response than TMB (12). However, scarce data were available regarding the general prognostic impact of indel burden. Our results showed that indel burden also had divergent prognostic impact in different cancer types. Unlike TMB, indel burden only significantly impacted OS in six out of 20 cancer types. As demonstrated by Turajlic et al., the median proportion of indels (calculated as number of indels divided by total counts of TMB) in most of the cancer types were relatively low (<10%) (12). We also found that the range of the absolute indel counts were quite narrow in the majority of these 20 cancer types (Table 1). We speculated that the discriminative power of indel burden was limited consequently. However, indel burden significantly impacted OS in two out of six cancer types in the TMB-Similar group, suggesting a supplementary role of indel burden to TMB.
A high TMB representative of a complex genetic profile may be a hallmark of more aggressive and treatment-refractory disease (31), which may lead to worse prognosis for patients with high TMB in the TMB-Worse group. Increased TMB may also be associated with increased prevalence of resistance pathways (32). However, a high TMB was also proposed to reflect the presence of mutation-associated neoantigens, with consequent increased lymphocyte infiltration in the tumor microenvironment and better prognosis (33), which may explain why high TMB was associated with superior OS in the TMB-Better group. To sum up, high TMB is more like a double-edged sword, and which survival path it would lead to mainly depends on the interaction between tumor and microenvironment.
Routine determination of TMB through whole-exome sequencing is currently still clinically impractical (34). Determination of TMB using NGS panels is now more feasible as the commercialization of targeted NGS panels scales up (35,36). TMB determined by the FDA-authorized MSK-IMPACT panel or the FDA-approved FoundationOne CDx panel, was proven to be the predictor of response to ICIs in multiple cancer types (7,19,37). Hence, we limited the analysis to these two panels (18,38), and found that the prognostic value of TMB remained significant in the majority of the 14 cancer types in the TMB-Worse and TMB-Better groups. This suggests that TMB based on targeted NGS panel is of great clinical value. However, only numerical survival differences were captured in several cancer types, suggesting that gene panels tailored specifically for these cancer types may be necessary.
The present study has several limitations. Although TCGA provides us with high-quality data, the included confounding factors were limited as this is a pan-cancer analysis. The impact of unaccounted confounders, e.g., treatment information, on our results cannot be excluded and further validation from prospective studies is still needed. Due to the lack of inadequate survival information, we did not study the survival impact of TMB or indel burden in patients with several common cancer types, such as breast invasive carcinoma and rectal adenocarcinoma, which are pending investigation (15). Besides, in the TCGA cohort, advanced cancers are relatively underrepresented, which might lead to potential bias.
In conclusion, our pan-cancer analysis provides key information pertaining to the general prognostic impact of TMB and indel burden across 20 primary solid tumors. We found that TMB has divergent survival impacts in different cancer types, thus could be incorporated in prognostication and risk stratification. More importantly, the prognostic impact should be taken into account when establishing the predictive function of TMB to ICI treatment.
Acknowledgments
We would like to thank the staff members of the TCGA Research Network for their involvement in the TCGA Pan-Cancer Clinical Data Resource project and the TCGA MC3 project. We would also like to thank Mr. Christopher Lavender, the native language editor from Sun Yat-sen University Cancer Center, for his assistance in editing this manuscript.
Funding: This work was supported by grants from the National Key Research and Development Program of China (2017YFC1308900); the National Natural Science Foundation of China (81872011); the Guangzhou Health and Medical Collaborative Innovation Project (201704020220); the Sun Yat-sen University Clinical Research 5010 Program (2018014); and the Young Teacher Training Program of Sun Yat-sen University (17ykpy78).
Footnote
Conflicts of Interest: The authors have no conflicts of interest to declare.
Ethics Statement: The authors are accountable for all aspects of the work in ensuring that questions related to the accuracy or integrity of any part of the work are appropriately investigated and resolved. Ethical approval was waived because we used only publicly available data and materials in this study.
References
- Martincorena I, Campbell PJ. Somatic mutation in cancer and normal cells. Science 2015;349:1483-9. [Crossref] [PubMed]
- Sanz-Garcia E, Argiles G, Elez E, et al. BRAF mutant colorectal cancer: prognosis, treatment, and new perspectives. Ann Oncol 2017;28:2648-57. [Crossref] [PubMed]
- Allegra CJ, Rumble RB, Hamilton SR, et al. Extended RAS Gene Mutation Testing in Metastatic Colorectal Carcinoma to Predict Response to Anti-Epidermal Growth Factor Receptor Monoclonal Antibody Therapy: American Society of Clinical Oncology Provisional Clinical Opinion Update 2015. J Clin Oncol 2016;34:179-85. [Crossref] [PubMed]
- Yarchoan M, Hopkins A, Jaffee EM. Tumor Mutational Burden and Response Rate to PD-1 Inhibition. N Engl J Med 2017;377:2500-1. [Crossref] [PubMed]
- Wu HX, Wang ZX, Zhao Q, et al. Designing gene panels for tumor mutational burden estimation: the need to shift from 'correlation' to 'accuracy'. J Immunother Cancer 2019;7:206. [Crossref] [PubMed]
- Havel JJ, Chowell D, Chan TA. The evolving landscape of biomarkers for checkpoint inhibitor immunotherapy. Nat Rev Cancer 2019;19:133-50. [Crossref] [PubMed]
- Gandara DR, Paul SM, Kowanetz M, et al. Blood-based tumor mutational burden as a predictor of clinical benefit in non-small-cell lung cancer patients treated with atezolizumab. Nat Med 2018;24:1441-8. [Crossref] [PubMed]
- Hellmann MD, Ciuleanu TE, Pluzanski A, et al. Nivolumab plus Ipilimumab in Lung Cancer with a High Tumor Mutational Burden. N Engl J Med 2018;378:2093-104. [Crossref] [PubMed]
- Rizvi NA, Hellmann MD, Snyder A, et al. Cancer immunology. Mutational landscape determines sensitivity to PD-1 blockade in non-small cell lung cancer. Science 2015;348:124-8. [Crossref] [PubMed]
- Hugo W, Zaretsky JM, Sun L, et al. Genomic and Transcriptomic Features of Response to Anti-PD-1 Therapy in Metastatic Melanoma. Cell 2016;165:35-44. [Crossref] [PubMed]
- Rosenberg JE, Hoffman-Censits J, Powles T, et al. Atezolizumab in patients with locally advanced and metastatic urothelial carcinoma who have progressed following treatment with platinum-based chemotherapy: a single-arm, multicentre, phase 2 trial. Lancet 2016;387:1909-20. [Crossref] [PubMed]
- Turajlic S, Litchfield K, Xu H, et al. Insertion-and-deletion-derived tumour-specific neoantigens and the immunogenic phenotype: a pan-cancer analysis. Lancet Oncol 2017;18:1009-21. [Crossref] [PubMed]
- Addeo A, Banna GL, Weiss GJ. Tumor Mutation Burden-From Hopes to Doubts. JAMA Oncol 2019;5:934-5. [Crossref] [PubMed]
- The TCGA Legacy. Cell 2018;173:281-2. [Crossref] [PubMed]
- Liu J, Lichtenberg T, Hoadley KA, et al. An Integrated TCGA Pan-Cancer Clinical Data Resource to Drive High-Quality Survival Outcome Analytics. Cell 2018;173:400-16.e11. [Crossref] [PubMed]
- Ellrott K, Bailey MH, Saksena G, et al. Scalable Open Science Approach for Mutation Calling of Tumor Exomes Using Multiple Genomic Pipelines. Cell Syst 2018;6:271-81.e7. [Crossref] [PubMed]
- Linnebacher M, Gebert J, Rudy W, et al. Frameshift peptide-derived T-cell epitopes: a source of novel tumor-specific antigens. Int J Cancer 2001;93:6-11. [Crossref] [PubMed]
- Chalmers ZR, Connelly CF, Fabrizio D, et al. Analysis of 100,000 human cancer genomes reveals the landscape of tumor mutational burden. Genome Med 2017;9:34. [Crossref] [PubMed]
- Rizvi H, Sanchez-Vega F, La K, et al. Molecular Determinants of Response to Anti-Programmed Cell Death (PD)-1 and Anti-Programmed Death-Ligand 1 (PD-L1) Blockade in Patients With Non-Small-Cell Lung Cancer Profiled With Targeted Next-Generation Sequencing. J Clin Oncol 2018;36:633-41. [Crossref] [PubMed]
- Endris V, Buchhalter I, Allgauer M, et al. Measurement of tumor mutational burden (TMB) in routine molecular diagnostics: in silico and real-life analysis of three larger gene panels. Int J Cancer 2019;144:2303-12. [PubMed]
- Thomas A, Routh ED, Pullikuth A, et al. Tumor mutational burden is a determinant of immune-mediated survival in breast cancer. Oncoimmunology 2018;7:e1490854. [Crossref] [PubMed]
- Cole SR, Hernan MA. Constructing inverse probability weights for marginal structural models. Am J Epidemiol 2008;168:656-64. [Crossref] [PubMed]
- Constantinidou A, Alifieris C, Trafalis DT. Targeting Programmed Cell Death -1 (PD-1) and Ligand (PD-L1): A new era in cancer active immunotherapy. Pharmacol Ther 2019;194:84-106. [Crossref] [PubMed]
- Conway JR, Kofman E, Mo SS, et al. Genomics of response to immune checkpoint therapies for cancer: implications for precision medicine. Genome Med 2018;10:93. [Crossref] [PubMed]
- Samstein RM, Lee CH, Shoushtari AN. Tumor mutational load predicts survival after immunotherapy across multiple cancer types. Nat Genet 2019;51:202-6. [Crossref] [PubMed]
- Hellmann MD, Paz-Ares L, Bernabe Caro R, et al. Nivolumab plus Ipilimumab in Advanced Non-Small-Cell Lung Cancer. N Engl J Med 2019;381:2020-31. [Crossref] [PubMed]
- Li X, Pasche B, Zhang W, et al. Association of MUC16 Mutation With Tumor Mutation Load and Outcomes in Patients With Gastric Cancer. JAMA Oncol 2018;4:1691-8. [Crossref] [PubMed]
- Innocenti F, Ou FS, Qu X, et al. Mutational Analysis of Patients With Colorectal Cancer in CALGB/SWOG 80405 Identifies New Roles of Microsatellite Instability and Tumor Mutational Burden for Patient Outcome. J Clin Oncol 2019;37:1217-27. [Crossref] [PubMed]
- Owada-Ozaki Y, Muto S, Takagi H, et al. Prognostic Impact of Tumor Mutation Burden in Patients With Completely Resected Non-Small Cell Lung Cancer: Brief Report. J Thorac Oncol 2018;13:1217-21. [Crossref] [PubMed]
- Devarakonda S, Rotolo F, Tsao MS, et al. Tumor Mutation Burden as a Biomarker in Resected Non-Small-Cell Lung Cancer. J Clin Oncol 2018;36:2995-3006. [Crossref] [PubMed]
- Hwang WL, Wolfson RL, Niemierko A, et al. Clinical Impact of Tumor Mutational Burden in Neuroblastoma. J Natl Cancer Inst 2018. [Epub ahead of print]. [PubMed]
- Offin M, Rizvi H, Tenet M, et al. Tumor Mutation Burden and Efficacy of EGFR-Tyrosine Kinase Inhibitors in Patients with EGFR-Mutant Lung Cancers. Clin Cancer Res 2019;25:1063-9. [Crossref] [PubMed]
- Giannakis M, Mu XJ, Shukla SA, et al. Genomic Correlates of Immune-Cell Infiltrates in Colorectal Carcinoma. Cell Rep 2016;15:857-65. [Crossref] [PubMed]
- Chan TA, Yarchoan M, Jaffee E, et al. Development of tumor mutation burden as an immunotherapy biomarker: utility for the oncology clinic. Ann Oncol 2019;30:44-56. [Crossref] [PubMed]
- Hyman DM, Solit DB, Arcila ME, et al. Precision medicine at Memorial Sloan Kettering Cancer Center: clinical next-generation sequencing enabling next-generation targeted therapy trials. Drug Discov Today 2015;20:1422-8. [Crossref] [PubMed]
- Szustakowski JD, Green G, Geese WJ, et al. Evaluation of tumor mutation burden as a biomarker for immune checkpoint inhibitor efficacy: A calibration study of whole exome sequencing with FoundationOne®. Cancer Res 2018;78:abstr 5528.
- Goodman AM, Kato S, Bazhenova L, et al. Tumor Mutational Burden as an Independent Predictor of Response to Immunotherapy in Diverse Cancers. Mol Cancer Ther 2017;16:2598-608. [Crossref] [PubMed]
- Zehir A, Benayed R, Shah RH, et al. Mutational landscape of metastatic cancer revealed from prospective clinical sequencing of 10,000 patients. Nat Med 2017;23:703-13. [Crossref] [PubMed]