Genetic landscape of prognostic value in pancreatic ductal adenocarcinoma microenvironment
Introduction
In developed countries, pancreatic cancer turns out to be the fourth leading cause of cancer-related death with a 5-year survival rate of less than 8.0%. In 2019, 56,770 people are predicted to be diagnosed with pancreatic cancer and 45,750 patients will die from the disease in United State (1,2). Although enormous efforts have been made to improve survival of pancreatic cancer patients, the treatment effect is still not obvious. Pancreatic ductal adenocarcinoma (PDAC) is considered as an intricate genetic disorder, therefore, it is crucial to identify genomic alterations in PDAC and its impacts on clinical prognosis.
Nowadays, lots of studies have profiled and analyzed various amounts of PDAC specimens to uncover molecular aberrations at different levels of DNA, RNA, protein and epigenetics by using The Cancer Genome Atlas (TCGA) Research Network (3-5). Recent whole genomes analyses of PDAC have revealed a complex mutational landscape: KRAS, TP53, SMAD4 and CDKN2A are the four most common mutated genes, of which KRAS mutations are near ubiquitous (6). Additionally, mutations of KRAS gene may predict a worse prognosis, which accounts for over 90% of PDAC (7,8).
The tumor microenvironment (TME) is the surroundings where cancer cells exist and absorb nutrients or interact with other ingredients. Besides the cancer cells, the TME is composed of surrounding blood vessels, the extracellular matrix, other non-tumor cells (for example, immune cells, dendritic cells, macrophages and fibroblasts) and also inflammatory mediators (9,10). On one hand, the mutational landscape of cancer cells, a direct reflection of cancer immunogenicity, can dictate the extent and phenotype of immune cell infiltration, and more generally influence the whole TME (11-13). On the other hand, TME also effect the gene expression of cancer cells, which is associated with the clinical prognosis (14-17). Immune and stromal cells, two major components of non-tumor cell populations in the TME, have been identified as prognostic assessment of tumor (18,19). Then, Yoshihara et al. designed an algorithm based on gene expression signatures to estimate the immune and stromal cells, as well as tumor purity, called Estimation of STromal and Immune cells in MAlignant Tumor tissues using Expression data (ESTIMATE) (20). Subsequently, ESTIMATE algorithm has been utilized to prostate cancer (21), cholangiocarcinoma (22), glioblastoma (23), lung cancer (24), breast cancer (25), salivary duct carcinoma (26), colon cancer (27) and many other malignancies, disposing the large data with effect, whereas the immune and/or stromal scores of PDAC in prognostic value has not been sufficiently investigated.
In this study, we focused on the immune and stromal cells that formed the major fraction of non-tumor components of PDAC tissues. By making use of both TCGA PDAC cohorts and immune/stromal scores based on ESTIMATE algorithm, a group of genes related to the microenvironment of PDAC was obtained for predicting prognosis or recurrence, and potential microenvironment associated functions were enriched in high immune/stromal environment.
Methods
Database
Level 3 gene expression profile for pancreatic cancer was downloaded from the TCGA data portal [TCGA pancreatic cancer (PAAD)] (https://xenabrowser.net/datapages/), RNA expression for pancreatic cancer using IlluminaHiSeq_RNASeqV2 (October 13, 2017). Gene mutation details about KRAS, TP53, SMAD4 and CDKN2A, along with data on clinical characteristics such as age, gender, TNM stage, histological type, survival and recurrence was also obtained from TCGA data portal. The downloaded database was applied by the ESTIMATE algorithm for calculating immune scores and stromal scores (https://bioinformatics.mdanderson.org/estimate/) (20). This study was approved by the Clinical Research Ethic Committee of Zhongshan Hospital, Fudan University (B2014-019).
Tumor IMmune Estimation Resource (TIMER)
The TIMER is a web-based open access resource for systematical analysis of immune infiltrations within various cancers (https://cistrome.shinyapps.io/timer/). All the PDAC cases from TCGA database were estimated for the abundances of six tumor-infiltrating cell populations including CD4+ T cells, CD8+ T cells, B cells, Macrophages, Neutrophils and Dendritic cells (DCs).
Differentially expressed genes (DEGs) identification
Package “limma” was used for data analysis (28). Fold change (FC) >3 and adj. P<0.05 were set as the cutoffs to identify the DEGs.
Volcano plots and heatmaps for clustering analysis
Volcano plots were generated with all related genes using package “gplots” in PDAC cases with FC >3 and adj. P<0.05. Package “gplots” were also used for generating heatmaps and clustering with DEGs.
Enrichment analysis of DEGs
The Database for Annotation, Visualization and Integrated Discovery (DAVID) (https://david.ncifcrf.gov/) (29) was an open-access tool for functional enrichment analysis of DEGs to identify gene oncology (GO) characteristics including biological processes (BP), molecular functions (MF), and cellular components (CC). Pathway enrichment analysis in reference to Kyoto Encyclopedia of Genes and Genomes (KEGG) pathways was also performed within the DAVID database. False discovery rate (FDR) <0.05 was used as the cut-off.
Protein-protein interaction (PPI) network construction
The PPI network was initially obtained from STRING database (30) and reconstructed via Cytoscape software (31). The minimum required interaction score was set up as 0.400. Further analysis was just applied for individual networks that owned 10 or more nodes, and networks were directly excluded from further study if they have less than 10 nodes. Molecular COmplex Detection (MCODE) was then manipulated to find topology-based clusters to locate regions with a high degree of connectivity.
Analysis
In this study, statistical analyses were performed with statistical software package R (version 3.3.4, http://www.r-project.org/). The Kaplan-Meier survival curves of overall survival (OS) and recurrence free survival (RFS) were generated by Graphpad Prism 7 program, and the Log-rank test for Kaplan-Meier survival curves was conducted to illustrate their relationships to gene mutations or expression levels of DEGs. All P value <0.05 made a significant difference statistically.
Results
Patients characteristics
The gene expression profiles, mutation data and clinical information of 179 pancreatic cancer patients with an initial pathologic diagnosis ranging from 2001 to 2013 were retrieved from the TCGA cohorts. Of them, 99 (55.3%) patients were male and 80 (44.7%) patients were female. Eighty-two patients (45.8%) were less than 65 years old. And 147 (82.1%) patients were pathologically diagnosed as PDAC, while 31 (17.3%) patients were other specific subtypes including neuroendocrine carcinoma, colloid carcinoma, undifferentiated carcinoma and so on. All other clinicopathological characteristics were displayed in Table S1. In the total cohort, 172 (96.1%) patients had complete and valid OS data and 152 (84.9%) patients had complete and valid RFS data. Among all PDAC patients, 146 (99.3%) patients had complete and detailed gene mutation data, 141 (95.9%) patients had complete and valid OS data (cohort OS), and 122 (83.0%) patients had complete and valid RFS data (cohort RFS) (Figure 1).
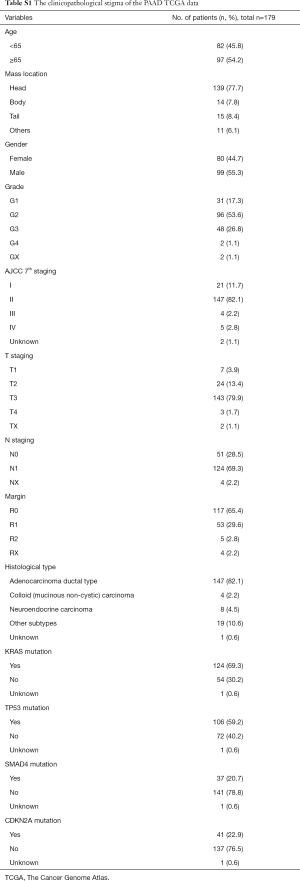
Full table
Immune scores and stromal scores are significantly associated with PDAC subtype
ESTIMATE algorithm-derived immune scores ranged from −1,559.87 to 3,037.78 (stromal scores: −1,843.32 to 2,179.19, Figure 2A,B). The average immune score of PDAC subtype was higher than that of other specific subtypes (Figure 2A, P<0.001), as well as stromal score (Figure 2B, P=0.007), which indicated that both scores were meaningfully correlated with PDAC.
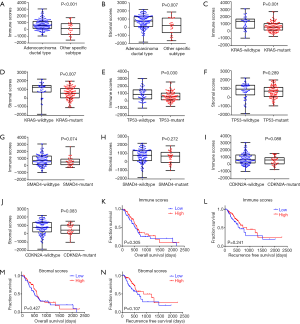
Based on the published four leading mutation gene in PDAC (KRAS, TP53, SMAD4 and CDKN2A), mutations of these genes are prognostic variables for worse survival rates in PDAC. Thus, the distribution of immune/stromal scores according to the status of these four gene mutations in PDAC cases was plotted. The results showed that KRAS mutant cases had significantly lower immune scores (P=0.001) and stromal scores (P=0.007), in concert with lower immune scores in TP53 mutant cases (P=0.030) (Figure 2C,D,E,F). However, no significant difference was found in SMAD4 and CDKN2A mutations (Figure 2G,H,I,J).
The PDAC cases were parted into high and low score groups to uncover the potential correlation of OS or RFS with immune/stromal scores. Kaplan-Meier survival curves showed that median OS and RFS of cases with the high immune scores was longer than those in the low score group (OS, Figure 2K, 596 vs. 518 d, P=0.305; RFS, Figure 2L, 872 vs. 593 d, P=0.241). Consistently, patients with higher stromal scores also had longer median OS and RFS, when compared to those with lower scores (OS, Figure 2M, 603 vs. 511 d, P=0.427; RFS, Figure 2N, 872 vs. 620 d, P=0.107), although they were not statistically significant.
Infiltration level of immune cells with immune scores and stromal scores in PDAC
With the estimation of TIMER, the infiltration levels of CD4+ T cells, CD8+ T cells, B cells, Macrophages, Neutrophils and DCs in PDAC cases were retrieved. In the cohort OS, the infiltration levels of CD4+ T cells, CD8+ T cells, B cells, Macrophages, Neutrophils and DCs in high score group of immune scores were higher than those in the low score group (Figure 3A, all P<0.001). However, the infiltration level of CD4+ T cells was significantly lower in the high stromal score group than that in the low stromal score group (Figure 3B, all P<0.001). Additionally, in the cohort RFS, the same findings were achieved based on stromal scores (Figure 3C, all P<0.001). Concomitantly, the infiltration levels of CD4+ T cells, CD8+ T cells, B cells, Neutrophils and DCs were significantly higher in the high immune score group (all P<0.001) except for macrophages (Figure 3D, P=0.113).
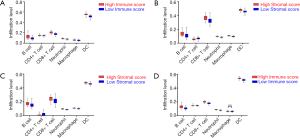
Comparison of gene expression profile in PDAC with immune/stromal scores
To find out the correlation of global gene expression profiles with immune/stromal scores, all PDAC cases retrieved from TCGA database were analyzed. Volcano plots in Figure 4A,B (heatmaps at http://fp.amegroups.cn/cms/7163e514a54a84ea9f6c0cb2c3e9502f/atm.2019.10.91-1.pdf and http://fp.amegroups.cn/cms/690049836e7ea409972c4702e67c884d/atm.2019.10.91-2.pdf) and Figure S1A,B (heatmaps at http://fp.amegroups.cn/cms/f09688e3ced2927a8f9ddcac36fc0b4d/atm.2019.10.91-3.pdf and http://fp.amegroups.cn/cms/e9c7cee39b6804d69f3d7615402ae928/atm.2019.10.91-4.pdf) displayed distinct gene expression profiles of cases between high and low immune/stromal score groups in the cohort OS/RFS. Within the cohort OS, in comparison to low immune score group, 317 upregulated genes and 9 downregulated genes were observed in the high score group (FC >3.0, P<0.05). Likewise, compared to low stromal score group, 227 upregulated genes and 17 downregulated genes were observed in the high score group (FC >3.0, P<0.05). Moreover, Venn diagrams (Figure 4C,D) revealed 124 upregulated genes were commonly found in both high score groups, as well as 6 downregulated genes. In the cohort RFS, 379 upregulated genes and 6 downregulated genes were observed in the high score group based on immune scores (FC >3.0, P<0.05), and 205 upregulated genes and 6 downregulated genes were found in the high stromal score group. In addition, Venn diagrams (Figure S1C,D) showed that 91 upregulated genes and 2 downregulated genes commonly appeared in both high score groups.
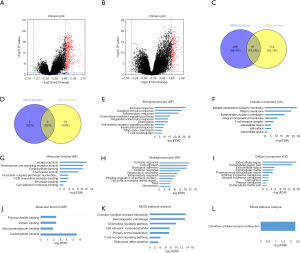
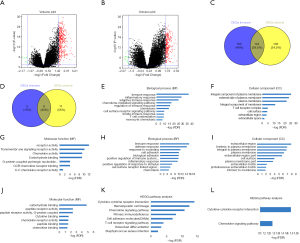
To reveal the underlying functional impacts of the DEGs, functional enrichment analysis of the DEGs were performed in high-immune/stromal score groups. Based on immune scores in the cohort OS, Top GO terms identified included immune, inflammatory and adaptive immune response, integral component of plasma membrane and T cell receptor complex, and receptor and chemokine activity (Figure 4E,F,G), while based on stromal scores, immune and defense response, extracellular region, and carbohydrate and cytokine binding were identified (Figure 4H,I,J). According to KEGG pathway analysis, the top one pathway with either immune scores or stromal scores was cytokine-cytokine receptor interaction (Figure 4K,L). However, based on immune/stromal scores in the cohort RFS, the top GO terms and KEGG pathways were displayed in Figure S1E,F,G,H,I,J,K,L.
Correlation of DEGs expression in OS and RFS
To investigate the potential impacts of individual DEGs on survival, Kaplan-Meier survival curves were plotted and analyzed for the PDAC cohort. Within the total 326 DEGs in the high-immune score group, 82 DEGs were verified to be significantly associated with better OS in log-rank tests (P<0.05), while 58 DEGs were confirmed as better OS predictors in the 244 DEGs in the high-stromal score group (P<0.05, Table S2). In addition, among the high-immune score group of 385 DEGs, 53 DEGs were verified to be significantly associated with longer RFS in log-rank tests (P<0.05), and in the high-stromal score group of 221 DEGs, 17 DEGs were associated with longer RFS (P<0.05, Table S3).

Full table
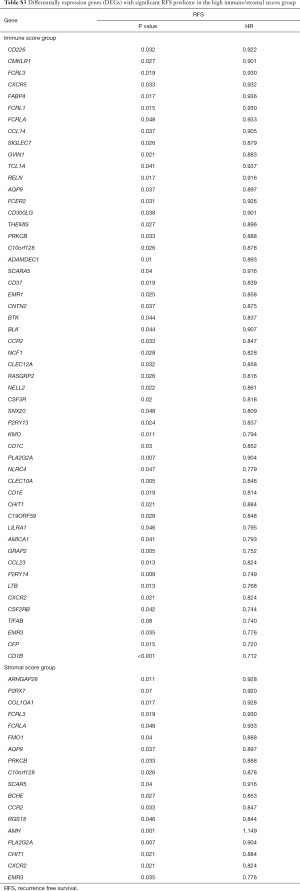
Full table
PPI of genes with prognostic value
PPI networks with the STRING and Cytoscape tool were administrated to better illustrate the interactions among the identified DEGs related to OS/RFS in high immune/stromal score groups. The network consisted of 8 modules including 146 nodes and 473 edges. The top two most significant modules were selected for further analysis (Figure 5). For the purpose of convenience of recognition, specific names were given to these two modules—CNR2 and CCL22 modules, respectively. In the CNR2 module (Figure 5A), the remarkable 11 nodes involved in 55 edges formed in the network. CNR2, CCR5, CXCR2, CCR4, P2RY13, PTGER3, and CCL19 were typically presented in this network, as they had the highest degree of connectivity with other members of the module. Meanwhile, in the CCL22 module (Figure 5B), CCL22, BTK, CD19, and CD1C had higher degree values.
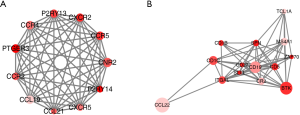
Functional enrichment analysis of genes with prognostic value
Functional enrichment analysis of these genes indicated robust relationship with immune response, which was consistent with PPI network analysis. In the enrichment analysis, a total of 9 GO terms of BP, 6 of CC and 2 of MF were found to be significant (FDR, or FDR <0.05, -log FDR >1.301). Top GO terms included immune and adaptive immune response (Figure 6A), plasma and integral of plasma membrane (Figure 6B), and chemokine receptor activity (Figure 6C). What’s more, altered transcriptional output in the pathways yielded from the KEGG analysis (Figure 6D) were associated with immune response.
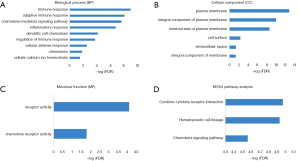
Discussion
In this study, we attempted to take advantage of samples in the TCGA database to obtain a list of genes that related to the PDAC microenvironment, which supported to predict prognosis or recurrence. Based on immune/stromal scores from the ESTIMATE algorithm, we found that both scores were higher in PDAC subtype. When considering to frequent gene mutation related to PDAC, significant increasing scores were found in KRAS and TP53 mutation. Although there was no statistical difference in high vs. low immune/stromal score groups, cases with higher immune/stromal scores may actually obtain a longer OS or RFS. As recently reported, higher immune cell score was validated to be associated with better prognosis of resected PDAC patients (32,33). So that we utilized TIMER, an open-access web server for comprehensive analysis of tumor-infiltrating immune cells, to characterize the landscape of immune infiltrations, which was crucial for the investigation and validation of immune scores and looked for the distribution of each immune components (34). Intriguingly, just CD4+ T cells showed an adverse trend in high vs. low immune/stromal score groups.
Then, by comparing global gene expression within the high vs. low immune (stromal) scores, 326 DEGs for cohort OS and 485 DEGs for cohort RFS were found to be involved in TME, totally enriching in immune, inflammatory and adaptive immune response, T cell receptor complex, and cytokine and chemokine activity, which further validated the essential role of immune regulations in the formation of PDAC microenvironment (35-37).
Next, OS and RFS of these DEGs were analyzed to identify a list of genes were associated with prognosis and recurrence in PDAC patients in terms of immune/stromal scores. Accordingly, 8 PPI network modules were depicted through STRING and Cytoscape tool, and all of which showed strong associations with immune response. From the PPI networks, CNR2 and CCL22 are highly interconnected nodes. CNR2 encodes the Cnr2 receptor, which is highly expressed in T and B lymphocytes, dendritic cells, natural killer cells, monocyte/macrophages, and neutrophils (38,39). Many studies verified CNR2 expressed highly in tumor-associated macrophages or various cancer cells and its expression was proved to be correlated with poor prognosis (40-43). However, CNR2 was also proved as an efficient prognostic regulator for some specific cancers in several researches, which had not previously been linked with PDAC prognosis (44-46). In our study, CNR2 was significantly proved to be associated with a better prognosis for PDAC patients. Likewise, CCL22, known as macrophage-derived chemokine, abundantly expressed in many malignancies was correlated with migration of regulatory T cells (47,48). It has been widely reported for its role in tumor-associated immunosuppression and could further predict reduced survival (47-50). It was strange that CCL22 expression in microenvironment displayed a positive role in survival of PDAC patients within our study, which may potentially facilitate other immune components except for regulatory T cells. However, Nakanishi et al. (51) previously reported that macrophage-derived CCL22 in human lung cancer was significantly correlated with lower risk of recurrence after tumor resection and longer RFS, which was consistent with our findings.
The study of tumor gene expression profiles contributes to the identification of molecular subtypes and the establishment of predictive prognostic models, enriching our understanding of the molecular pathways of tumorigenesis (6). In addition, in our previous research, we found the tumor infiltrating CD8+ T cells in PDAC were significantly associated with survival, while tumor infiltrating Tregs had the adverse role. Also, TGF-beta blockade combined with depletion of Treg or DC vaccine administration were verified to successfully inhibit PDAC growth (2,52). All our previous results showed PDAC microenvironment had a giant impact on tumor progression. Concomitantly, pancreatic cancer cell-intrinsic PD-1 was also identified and proved its role in tumor progression (53). Current progresses in cancer immunotherapy and the reduced cost of high-throughput techniques have led to extensive investigations of tumor immune cell communications by means of genomic tools. Thus, through our mining the PDAC microenvironment related genes with valuable prognostic roles, some novel microenvironment related gene panel may be established for survival or recurrence prediction. However, owing to the heterogeneity of the tumor genomes and the plasticity of the host immune system, the communication between tumor cells and immune infiltrations remains a testing research topic and further validations are preferred (54).
However, there were still some limitations in this study. First, all the data are retrieved from the public database and external validations will be required to verify our findings. Second, the significance of up- and down-regulated genes may not be the answers to what is really driving the aggressive biology of pancreatic cancer, so further mechanistic study of these genes are encouraged.
In conclusion, we have extracted a list of genes with prognostic value in PDAC microenvironment from functional enrichment analysis of TCGA database based on immune and stromal scores in our study. These genes can be useful to predict the prognosis of PDAC patients and show their potentials to be auxiliary prognostic biomarkers for PDAC. Additionally, further research on these genes may be valuable and helpful to understand the crosstalk between tumor and microenvironment, new immune evasion mechanisms and underlying novel therapeutic targets in an integrated manner.
Acknowledgments
Funding: This work was granted by Zhongshan Clinical Trial (2016ZSLC14), Clinical Science and Technology Innovation Project, Shenkang Hospital Development Center of Shanghai (SHDC12017X04) and Shanghai Pujiang Program (16PJD013).
Footnote
Conflicts of Interest: The authors have no conflicts of interest to declare.
Ethical Statement: The authors are accountable for all aspects of the work in ensuring that questions related to the accuracy or integrity of any part of the work are appropriately investigated and resolved. This study was approved by the Clinical Research Ethic Committee of Zhongshan Hospital, Fudan University (B2014-019).
References
- Siegel RL, Miller KD, Jemal A. Cancer statistics, 2019. CA Cancer J Clin 2019;69:7-34. [Crossref] [PubMed]
- Pu N, Zhao G, Yin H, et al. CD25 and TGF-beta blockade based on predictive integrated immune ratio inhibits tumor growth in pancreatic cancer. J Transl Med 2018;16:294. [Crossref] [PubMed]
- Ho J, Li X, Zhang L, et al. Translational genomics in pancreatic ductal adenocarcinoma: A review with re-analysis of TCGA dataset. Semin Cancer Biol 2019;55:70-7. [Crossref] [PubMed]
- Yan Y, Gao R, Trinh TLP, et al. Immunodeficiency in Pancreatic Adenocarcinoma with Diabetes Revealed by Comparative Genomics. Clin Cancer Res 2017;23:6363-73. [Crossref] [PubMed]
- Cancer Genome Atlas Research Network. Electronic address aadhe, Cancer Genome Atlas Research N. Integrated Genomic Characterization of Pancreatic Ductal Adenocarcinoma. Cancer Cell 2017;32:185-203.e13. [Crossref]
- Waddell N, Pajic M, Patch AM, et al. Whole genomes redefine the mutational landscape of pancreatic cancer. Nature 2015;518:495-501. [Crossref] [PubMed]
- Bournet B, Muscari F, Buscail C, et al. KRAS G12D Mutation Subtype Is A Prognostic Factor for Advanced Pancreatic Adenocarcinoma. Clin Transl Gastroenterol 2016;7:e157. [Crossref] [PubMed]
- Kwon MJ, Jeon JY, Park HR, et al. Low frequency of KRAS mutation in pancreatic ductal adenocarcinomas in Korean patients and its prognostic value. Pancreas 2015;44:484-92. [PubMed]
- Junttila MR, de Sauvage FJ. Influence of tumour micro-environment heterogeneity on therapeutic response. Nature 2013;501:346-54. [Crossref] [PubMed]
- Hanahan D, Weinberg RA. Hallmarks of Cancer: The Next Generation. Cell 2011;144:646-74. [Crossref] [PubMed]
- Petitprez F, Vano YA, Becht E, et al. Transcriptomic analysis of the tumor microenvironment to guide prognosis and immunotherapies. Cancer Immunol Immunother 2018;67:981-8. [Crossref] [PubMed]
- Li J, Byrne KT, Yan F, et al. Tumor Cell-Intrinsic Factors Underlie Heterogeneity of Immune Cell Infiltration and Response to Immunotherapy. Immunity 2018;49:178-93.e7. [Crossref] [PubMed]
- Binnewies M, Roberts EW, Kersten K, et al. Understanding the tumor immune microenvironment (TIME) for effective therapy. Nat Med 2018;24:541-50. [Crossref] [PubMed]
- Maley CC, Aktipis A, Graham TA, et al. Classifying the evolutionary and ecological features of neoplasms. Nat Rev Cancer 2017;17:605-19. [Crossref] [PubMed]
- Herrera M, Islam ABMMK, Herrera A, et al. Functional Heterogeneity of Cancer-Associated Fibroblasts from Human Colon Tumors Shows Specific Prognostic Gene Expression Signature. Clinical Cancer Research 2013;19:5914-26. [Crossref] [PubMed]
- Cooper LA, Gutman DA, Chisolm C, et al. The tumor microenvironment strongly impacts master transcriptional regulators and gene expression class of glioblastoma. Am J Pathol 2012;180:2108-19. [Crossref] [PubMed]
- Artacho-Cordón A, Artacho-Cordon F, Rios-Arrabal S, et al. Tumor microenvironment and breast cancer progression: a complex scenario. Cancer Biol Ther 2012;13:14-24. [Crossref] [PubMed]
- Efstathiou JA, Mouw KW, Gibb EA, et al. Impact of Immune and Stromal Infiltration on Outcomes Following Bladder-Sparing Trimodality Therapy for Muscle-Invasive Bladder Cancer. Eur Urol 2019;76:59-68. [Crossref] [PubMed]
- Mahajan UM, Langhoff E, Goni E, et al. Immune Cell and Stromal Signature Associated With Progression-Free Survival of Patients With Resected Pancreatic Ductal Adenocarcinoma. Gastroenterology 2018;155:1625-39.e2. [Crossref] [PubMed]
- Yoshihara K, Shahmoradgoli M, Martinez E, et al. Inferring tumour purity and stromal and immune cell admixture from expression data. Nat Commun 2013;4:2612. [Crossref] [PubMed]
- Fraser M, Sabelnykova VY, Yamaguchi TN, et al. Genomic hallmarks of localized, non-indolent prostate cancer. Nature 2017;541:359-64. [Crossref] [PubMed]
- Jusakul A, Cutcutache I, Yong CH, et al. Whole-Genome and Epigenomic Landscapes of Etiologically Distinct Subtypes of Cholangiocarcinoma. Cancer Discov 2017;7:1116-35. [Crossref] [PubMed]
- Jia D, Li S, Li D, et al. Mining TCGA database for genes of prognostic value in glioblastoma microenvironment. Aging (Albany NY) 2018;10:592-605. [Crossref] [PubMed]
- Karlsson A, Jonsson M, Lauss M, et al. Genome-wide DNA methylation analysis of lung carcinoma reveals one neuroendocrine and four adenocarcinoma epitypes associated with patient outcome. Clin Cancer Res 2014;20:6127-40. [Crossref] [PubMed]
- Abba MC, Gong T, Lu Y, et al. A Molecular Portrait of High-Grade Ductal Carcinoma In Situ. Cancer Res 2015;75:3980-90. [Crossref] [PubMed]
- Dalin MG, Desrichard A, Katabi N, et al. Comprehensive Molecular Characterization of Salivary Duct Carcinoma Reveals Actionable Targets and Similarity to Apocrine Breast Cancer. Clin Cancer Res 2016;22:4623-33. [Crossref] [PubMed]
- Alonso MH, Ausso S, Lopez-Doriga A, et al. Comprehensive analysis of copy number aberrations in microsatellite stable colon cancer in view of stromal component. Br J Cancer 2017;117:421-31. [Crossref] [PubMed]
- Ritchie ME, Phipson B, Wu D, et al. limma powers differential expression analyses for RNA-sequencing and microarray studies. Nucleic Acids Res 2015;43:e47. [Crossref] [PubMed]
- Huang W, Sherman BT, Lempicki RA. Systematic and integrative analysis of large gene lists using DAVID bioinformatics resources. Nat Protoc 2009;4:44-57. [Crossref] [PubMed]
- Szklarczyk D, Franceschini A, Wyder S, et al. STRING v10: protein-protein interaction networks, integrated over the tree of life. Nucleic Acids Res 2015;43:D447-52. [Crossref] [PubMed]
- Shannon P, Markiel A, Ozier O, et al. Cytoscape: A software environment for integrated models of biomolecular interaction networks. Genome Res 2003;13:2498-504. [Crossref] [PubMed]
- Tahkola K, Leppanen J, Ahtiainen M, et al. Immune cell score in pancreatic cancer-comparison of hotspot and whole-section techniques. Virchows Arch 2019. [Crossref] [PubMed]
- Tahkola K, Mecklin JP, Wirta EV, et al. High immune cell score predicts improved survival in pancreatic cancer. Virchows Arch 2018;472:653-65. [Crossref] [PubMed]
- Li T, Fan J, Wang B, et al. TIMER: A Web Server for Comprehensive Analysis of Tumor-Infiltrating Immune Cells. Cancer Res 2017;77:e108-10. [Crossref] [PubMed]
- Sherman MH, Yu RT, Tseng TW, et al. Stromal cues regulate the pancreatic cancer epigenome and metabolome. Proc Natl Acad Sci U S A 2017;114:1129-34. [Crossref] [PubMed]
- Chang JH, Jiang Y, Pillarisetty VG. Role of immune cells in pancreatic cancer from bench to clinical application: An updated review. Medicine (Baltimore) 2016;95:e5541. [Crossref] [PubMed]
- Feig C, Gopinathan A, Neesse A, et al. The pancreas cancer microenvironment. Clin Cancer Res 2012;18:4266-76. [Crossref] [PubMed]
- Rieder SA, Chauhan A, Singh U, et al. Cannabinoid-induced apoptosis in immune cells as a pathway to immunosuppression. Immunobiology 2010;215:598-605. [Crossref] [PubMed]
- Pandey R, Mousawy K, Nagarkatti M, et al. Endocannabinoids and immune regulation. Pharmacol Res 2009;60:85-92. [Crossref] [PubMed]
- Wang J, Xu YZ, Zhu LS, et al. Cannabinoid receptor 2 as a novel target for promotion of renal cell carcinoma prognosis and progression. J Cancer Res Clin Oncol 2018;144:39-52. [Crossref] [PubMed]
- Pérez-Gómez E, Andradas C, Blasco-Benito S, et al. Role of cannabinoid receptor CB2 in HER2 pro-oncogenic signaling in breast cancer. J Natl Cancer Inst 2015;107:djv077. [Crossref] [PubMed]
- Xiang W, Shi R, Kang X, et al. Monoacylglycerol lipase regulates cannabinoid receptor 2-dependent macrophage activation and cancer progression. Nat Commun 2018;9:2574. [Crossref] [PubMed]
- Martínez-Martínez E, Martin-Ruiz A, Martin P, et al. CB2 cannabinoid receptor activation promotes colon cancer progression via AKT/GSK3beta signaling pathway. Oncotarget 2016;7:68781-91. [Crossref] [PubMed]
- Elbaz M, Ahirwar D, Ravi J, et al. Novel role of cannabinoid receptor 2 in inhibiting EGF/EGFR and IGF-I/IGF-IR pathways in breast cancer. Oncotarget 2017;8:29668-78. [Crossref] [PubMed]
- Bettiga A, Aureli M, Colciago G, et al. Bladder cancer cell growth and motility implicate cannabinoid 2 receptor-mediated modifications of sphingolipids metabolism. Sci Rep 2017;7:42157. [Crossref] [PubMed]
- Ravi J, Elbaz M, Wani NA, et al. Cannabinoid receptor-2 agonist inhibits macrophage induced EMT in non-small cell lung cancer by downregulation of EGFR pathway. Mol Carcinog 2016;55:2063-76. [Crossref] [PubMed]
- Gobert M, Treilleux I, Bendriss-Vermare N, et al. Regulatory T Cells Recruited through CCL22/CCR4 Are Selectively Activated in Lymphoid Infiltrates Surrounding Primary Breast Tumors and Lead to an Adverse Clinical Outcome. Cancer Res 2009;69:2000-9. [Crossref] [PubMed]
- Curiel TJ, Coukos G, Zou L, et al. Specific recruitment of regulatory T cells in ovarian carcinoma fosters immune privilege and predicts reduced survival. Nat Med 2004;10:942-9. [Crossref] [PubMed]
- Wu S, He H, Liu H, et al. C-C motif chemokine 22 predicts postoperative prognosis and adjuvant chemotherapeutic benefits in patients with stage II/III gastric cancer. Oncoimmunology 2018;7:e1433517. [Crossref] [PubMed]
- Wiedemann GM, Knott MM, Vetter VK, et al. Cancer cell-derived IL-1alpha induces CCL22 and the recruitment of regulatory T cells. Oncoimmunology 2016;5:e1175794. [Crossref] [PubMed]
- Nakanishi T, Imaizumi K, Hasegawa Y, et al. Expression of macrophage-derived chemokine (MDC)/CCL22 in human lung cancer. Cancer Immunol Immunother 2006;55:1320-9. [Crossref] [PubMed]
- Pu N, Zhao G, Gao S, et al. Neutralizing TGF-beta promotes anti-tumor immunity of dendritic cells against pancreatic cancer by regulating T lymphocytes. Cent Eur J Immunol 2018;43:123-31. [Crossref] [PubMed]
- Pu N, Gao S, Yin H, et al. Cell-intrinsic PD-1 promotes proliferation in pancreatic cancer by targeting CYR61/CTGF via the hippo pathway. Cancer Lett 2019;460:42-53. [Crossref] [PubMed]
- Hackl H, Charoentong P, Finotello F, et al. Computational genomics tools for dissecting tumour-immune cell interactions. Nat Rev Genet 2016;17:441-58. [Crossref] [PubMed]