CMTTdb: the cancer molecular targeted therapy database
Introduction
Cancer has emerged as one of the most prominent causes of death worldwide. According to the Global Cancer Statistics, cancer incidence and mortality rates have been continuing to rise worldwide in the past decade (1). It represents an enormous economic and social burden to society. Until now, the surgical resection and/or traditional chemotherapy by using cytotoxic drugs is still the major choice for cancer treatment (2). The cytotoxic drugs block carcinogenesis and tumor growth by interfering with rapidly dividing cells (3). Most of the cytotoxic drugs, however, present limited therapy efficiency and severe side effects in clinical use. Thus, it is critical to develop more effective and less harmful treatment methods which lead to better patient care.
In recent years, the quick advance of molecular targeted therapy has shed light on cancer treatment. Molecular targeted therapy agents block genes that are upregulated, overexpressed, or mutated specifically in cancer cells, thus minimizing toxicities while improving treatment efficacy (4). Since the emergence of the most successful molecular targeted therapeutic agent imatinib, which is used for treatment of chronic myeloid leukemia, much more attention has been drew on this area (5). There has been an explosion in the number of molecular targeted agents, as well as the accompanying biomarkers that can be used for selection of specific cancer patients (6). These advances have set the stage for precision oncology, which based on the idea that cancer treatment and prognosis could be remarkably improved if the choice of therapy could be guided by identification of specific genomic alterations in tumor cells (7). However, these agent-target relationships regarding to different cancer types are buried in thousands of published studies. It is still a challenging task to translate this clinical trial data into a systematically annotated and documented database for ease of interpretation.
Until now, several databases have been developed pointing to this problem. The Therapeutic Target Database, for instance, provides information about the known therapeutic targets, as well as the corresponding drugs directed at each of these targets described in literature (8). The Drug Bank is a resource that combines detailed drug data with comprehensive target information (9). The SuperTarget database provides the drug-target interactions and also the side-effects information of the drugs (10). Several other databases such as GtoPdb (11), CARLSBAD (12), BindingDB (13), TiPs (14), SIDER (15), focus on different aspects of the molecular targeted therapies, such as gene structure, chromosomal location, 3D-structure, activator, functions, biological pathways and side effects of the targets. However, none of these resources provides the detailed information about clinical trials of the therapeutic agents.
Here we provide a newly established knowledge repository: The Cancer Molecular-Targeted Therapy database (CMTTdb). This database integrates the anticancer therapy agents, targets and the associated clinical trial data from peer-reviewed publications. It particularly focuses on the key information for the randomized clinical trials (RCTs), such as patient selection criteria, tumor conditions, therapy modes (monotherapy or combined therapy), clinical efficacy evaluation parameters, such as maximal tolerated dose (MTD), dose-limiting toxicity (DLT), objective response rate (ORR), disease control rate (DCR), progression free survival (PFS), overall survival (OS), and drug-related adverse events. CMTTdb now contains data of 1,088 clinical trials for 165 therapeutic agents against 80 targets, which covered 15 major cancer types (95 molecular subtypes, and 56 histological or cytological subtypes). All the information in CMTTdb is freely available to the public domain through http://www.biosino.org/CMTTdb. Our database will be a valuable source for molecular-targeted cancer therapies and their application modes in practice, and also a bridge for the gap between bench and clinical application in the era of precision medicine.
Methods
We conducted a systematic searching from public databases and related literatures to collect information on clinical trials of therapy agents: literature collection was performed in PubMed by using keywords pertaining to molecular-targeted cancer therapies, including “targeted cancer therapy”, “targeted agents”, “targeted anticancer agents”, “targeted cancer treatment”, “precision cancer treatment”, “precision oncology”, “precision medicine”, “kinase inhibitors” and “monoclonal antibodies in oncology”. The search results were limited to those published before Dec. 2018. All the literatures were manually reviewed for data quality control. We refined the searching results to those performed in solid tumors from adult patients. Clinical trials that not related to solid tumors or malignancies for children were excluded. For the completeness of the database, we then made a complementary search for those well-known multicenter clinical trials. Specifically, we focused on the first authors or the corresponding authors of the literature and searched for all related clinical trials of solid tumor-targeted therapies in which they had participated.
Results
Content of the database
Through these efforts, we collected information about 1,088 clinical trials with 165 agents and 80 targets, covering 56 types of the solid tumors. For the propose of easy to access, all the tumor types were further classified into different categories according to the primary site of the tumor, molecular subtypes, cancer stage and metastatic site. For the therapeutic modes of the clinical trials, two different categories were identified: monotherapy and combinatory therapy (Figure 1A), the latter can be further classified into 10 subclasses according to the combination modalities, such as bi-targeted therapy, targeted therapy plus chemotherapy, targeted therapy plus chemo-radiation therapy or targeted therapy plus radiation therapy, etc. Among these, combination of targeted therapy and chemotherapy present most (58%) from combinatory therapy modes, and followed by the bi-targeted therapy (Figure 1B).
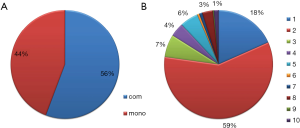
We then calculated occurrence frequency of certain target form different clinical trials among different cancer types, we found that the distribution of targets in different kinds of cancer are quite different, for instance, For tumors of brain and nervous system, “vascular endothelial growth factor receptor” has the highest occurrence frequency, while the target of “Receptor protein tyrosine kinase erbB2” presents height in breast cancer (Figure 2). This indicates that different cancer types may bear very different driver genes. Here we selected eleven targets which present height occurrence frequency across all the clinical trials and calculated the percentage of trials with successful results among different kinds of cancer (Table 1). The head and neck cancer, for example, 64 out of 83 clinical trials present effective results in regard to the targeted gene “epidermal growth factor receptor”, while 32 demonstrated positive among 44 trials for colorectal cancer, etc. This indicated that different clinical benefit could be obtained for different cancer types even if the same target was used. Thus, novel biomarkers and therapeutic target remains to be identified for more effective targeted therapy to patients.
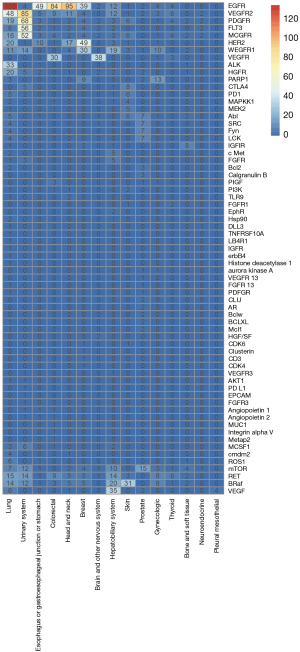
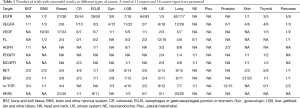
Full table
Database construction
CMTTdb was implemented as a relational database using MySQL. Five major tables were used to store data. Web interface was implemented using PHP language, and Apache was used as the HTTP server.
Implementation
CMTTdb provides a user-friendly interface allowing easy access to the data. The “Search” page permits users to perform a quick search in the database. Key words searching by therapy agent name, target name and cancer types are supported. This searching tool helps users to locate specific clinical trials information of interest rapidly and accurately. For example, a medicinal chemist or a pharmacologist may be particularly interested in the specific therapy agents, such as “imatinib”, which related to clinical trials for any cancer type, he may select “Agent” of the pull-down list, and type “imatinib” in the searching box, then a short list of targets and cancer types for those trials will be presented. In addition, users could get an overview of the database for the agent, target and cancer type with a unique database ID from the “Browse” page.
Detailed information of a specific clinical trial can be accessed via the hyperlink of database entry. This page consists of three parts: Summary, Clinical Trail Design, and Trail Results. In the “Summary” section, brief description and the reference of the trail was presented. Whereas for the “Trial Design” section, basic information about clinical trials are listed, which include NCT number, synonyms of the agent, target, cancer type and subtype, therapy type (monotherapy or combinatorial therapy), combination mode, patients feature, biomarkers and biomarker analysis, sample number, etc. The “Trial Results” section mainly includes parameters reflecting clinical efficacy, such as MDT, DLT, ORR, DCR, PFS, OS, drug-related adverse events and the clinical conclusions. We also provide a page for downloading of all the information in the database (Figure 3).
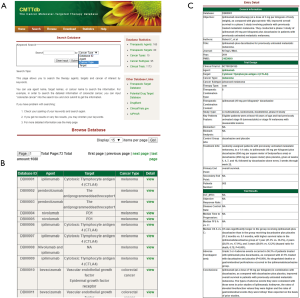
Discussion
With the advent of the era of precision medicine, investment has rapidly increased to search the optimal agent or the best treatment modalities for cancer therapy. Cancer targeted medicine has demonstrated great superiority over traditional therapies, especially the more efficacies and less toxicity (16). At present, a total of 43 different molecular-targeted anticancer agents have been approved by the US Food and Drug Administration (7). It could be expected much more prevalent application of the molecular targeted therapies in the future. The CMTTdb was thus established to utilize the public trial resources and in order to provide a tool to facilitate the clinical use in future.
One of the advantages of the CMTTdb is it particularly emphasis the clinical application potential of the randomized clinical trial data. Some important indices that critical for clinical practice are presented in the database. For instance, the patients’ inclusion criteria for the trial could help to evaluate whether some therapy methods are suitable to particular type of patient. The therapy efficiency and safety evaluation parameters could help to choose the best strategies for the patient. Particularly, this database includes the treatment modalities of the trails: whether it is a monotherapy or combinatorial therapy with other treatment methods. The detailed information about combination type was also indicated if combinatorial therapy was used. The bi-targeted therapy, for instance, indicated a combinatorial approach with another therapy agent, or combinatorial approach with traditional chemotherapy, radiation therapy, etc. Until now, a total of 519 monotherapy trails and 654 combinatorial therapy trails were included in this current version of database, which constitute about 44% and 56% of the total approaches, respectively. For the combinatorial therapy trails, the dual bi-targeted therapy (18%) and combination with chemotherapy (59%) constitute the most part. Although there are still controversies about whether the combined utilization of different types of drugs could increase the likelihood of toxic effects, combinatorial therapies are still widely used in most cases, due to that resistance to single-agent targeted therapy frequently arises and such therapy lacks sufficient efficacy for several indications (17). As such, it would be extremely useful for oncologists to have convenient access to such information on different molecular-targeted cancer therapies and their combinatory modes to evaluate their novel application in clinical practice for treatment of solid tumors.
It has been known that both the “blocking efficacy” of the agents and mutation frequency of driver gene in different kinds of cancer could impact the final effect of the trials (18). On one hand, trials with different drugs targets the same mutations in certain kinds of cancer may have different response rates and survival time. For instance, Studies showed that short impulse targeting of the mitogen-activated protein kinase (MAPK) promote cell proliferation, while longer and continuous MAPK targeting could inhibit cell growth (19). On the other hand, the plasticity and heterogeneity of the tumor may lead to in different results for trails from different cancer using same targeted agents. Furthermore, cancer cells could evolve persistently and new mutations may accumulate from primary tumor overtime, which will result in drug resistance and failure of treatment. Thus, combination with more agent or other therapy methods is necessary to achieve durable control of tumor growth. The well documented information for clinical trials, as well as agents and targets in this database will be valuable source for identifying appropriate combinations of therapies to deal with resistance to treatments.
Conclusions
With the rapid progress of molecular targeted therapy research and its clinical application, large amount of clinical trial data of the targeted agents for treatment of different types of cancer have published by public resources. CMTTdb provides a comprehensive collection of these resources together and link them into clinical use. We hope this database could be a valuable source for scientists and clinicians who have been striving to find the optimal agent or the best treatment modalities for cancer therapy, thus be beneficial to the growing number of cancer patients throughout the disease trajectory. This database will be updated periodically to incorporate new data as this field is advancing rapidly.
Acknowledgments
Funding: This work was supported by International Science and Technology Cooperation Projects (2016YFE0107100 and 2015DFA30650), CAMS Innovation Fund for Medical Science (CIFMS) (2017-I2M-4-003 and 2018-I2M-3-001), Beijing Natural Science Foundation (L172055 and 7192158), the Fundamental Research Funds for the Central Universities (3332018032), National Ten-thousand Talent Program and Youth Innovation Promotion Association of the Chinese Academy of Sciences (No. 2016252), the Youth foundation of the national natural science foundation of China (Grant No. 81902620), the Youth foundation of the national natural science foundation of China (Grant No. 81902353).
Footnote
Conflicts of Interest: The authors have no conflicts of interest to declare.
Ethical Statement: The authors are accountable for all aspects of the work in ensuring that questions related to the accuracy or integrity of any part of the work are appropriately investigated and resolved. Ethics approval and consent to participate are not applicable as a ‘database’ article, no data is newly generated, only data available in public literatures was collected.
References
- Torre LA, Siegel RL, Ward EM, et al. Global Cancer Incidence and Mortality Rates and Trends--An Update. Cancer Epidemiol Biomarkers Prev 2016;25:16-27. [Crossref] [PubMed]
- Miura K. The day will come to treat HCC in a drugstore? HepatoBiliary Surg Nutr 2017;6:420-3. [Crossref] [PubMed]
- Malhotra V, Perry MC. Classical chemotherapy: mechanisms, toxicities and the therapeutic window. Cancer Biol Ther 2003;2:S2-4. [Crossref] [PubMed]
- Huang M, Shen A, Ding J, et al. Molecularly targeted cancer therapy: some lessons from the past decade. Trends Pharmacol Sci 2014;35:41-50. [Crossref] [PubMed]
- Smith BD. Imatinib for chronic myeloid leukemia: the impact of its effectiveness and long-term side effects. J Natl Cancer Inst 2011;103:527-9. [Crossref] [PubMed]
- DiMasi JA, Grabowski HG. Economics of new oncology drug development. J Clin Oncol 2007;25:209-16. [Crossref] [PubMed]
- Gharwan H, Groninger H. Kinase inhibitors and monoclonal antibodies in oncology: clinical implications. Nat Rev Clin Oncol 2016;13:209-27. [Crossref] [PubMed]
- Li YH, Yu CY, Li XX, et al. Therapeutic target database update 2018: enriched resource for facilitating bench-to-clinic research of targeted therapeutics. Nucleic Acids Res 2018;46:D1121-7. [PubMed]
- Wishart DS, Feunang YD, Guo AC, et al. DrugBank 5.0: a major update to the DrugBank database for 2018. Nucleic Acids Res 2018;46:D1074-82. [Crossref] [PubMed]
- Hecker N, Ahmed J, von Eichborn J, et al. SuperTarget goes quantitative: update on drug-target interactions. Nucleic Acids Res 2012;40:D1113-7. [Crossref] [PubMed]
- Southan C, Sharman JL, Benson HE, et al. The IUPHAR/BPS Guide to PHARMACOLOGY in 2016: towards curated quantitative interactions between 1300 protein targets and 6000 ligands. Nucleic Acids Res 2016;44:D1054-68. [Crossref] [PubMed]
- Mathias SL, Hines-Kay J, Yang JJ, et al. The CARLSBAD database: a confederated database of chemical bioactivities. Database (Oxford) 2013;2013:bat044. [Crossref] [PubMed]
- Gilson MK, Liu T, Baitaluk M, et al. BindingDB in 2015: A public database for medicinal chemistry, computational chemistry and systems pharmacology. Nucleic Acids Res 2016;44:D1045-53. [Crossref] [PubMed]
- Lepore R, Tramontano A, Via A. TiPs: a database of therapeutic targets in pathogens and associated tools. Bioinformatics 2013;29:1821-2. [Crossref] [PubMed]
- Kuhn M, Letunic I, Jensen LJ, et al. The SIDER database of drugs and side effects. Nucleic Acids Res 2016;44:D1075-9. [Crossref] [PubMed]
- Salgado R, Moore H, Martens JWM, et al. Steps forward for cancer precision medicine. Nat Rev Drug Discov 2018;17:1-2. [Crossref] [PubMed]
- Ascierto PA, Marincola FM, Atkins MB. What's new in melanoma? Combination! J Transl Med 2015;13:213. [Crossref] [PubMed]
- Ghosh S, Banerjee S. Whole-genome mutational analysis: cause and effect of noncoding and structural mutations in liver cancer. HepatoBiliary Surg Nutr 2017;6:57-9. [Crossref] [PubMed]
- Marshall CJ. Specificity of receptor tyrosine kinase signaling: transient versus sustained extracellular signal-regulated kinase activation. Cell 1995;80:179-85. [Crossref] [PubMed]