The application of single-cell sequencing technology in the diagnosis and treatment of hepatocellular carcinoma
Introduction
Induced by carcinogenic factors, the genetic materials are impaired, causing the abnormal alteration of molecules regulating cell growth and proliferation, and eventually disorganized cell proliferation and clonal transformation into tumors. As a disease closely related to genetic content, tumor cells exhibit a combination of cytogenetic and epigenetic aberration, which are considered as the main determinant of cancer at the levels of symptoms and genetics (1).
The tumor heterogeneity, discovered and proposed by Fidler in 1977, is an important characteristic of malignant tumors (2). The tumor heterogeneity may manifest as the differences in pathological types and degrees of differentiation in the clinic; the differences in genome, transcriptome and epigenome at the molecular basis; the differences in invasiveness, growth rates and immune evasion of tumor cells for each individual patient; and the differences in morbidity, mortality, and sensitivities to treatment for different countries, ethnicity, races, genders, etc. All the factors above contribute to the great challenges during the long process of tumor treatment.
Primary liver cancer is the malignant tumor leading to second most neoplastic death worldwide. HCC, the major type of liver cancer, usually occurs alongside chronic liver diseases and the progression rate depends on the complex interactions between genetic and environmental factors (3). Recent studies have revealed that a large quantity of genetic mutations exists in the cellular genome of HCC, and the patterns of mutations are associated with their epidemiological backgrounds. The pathogenesis of HCC involves somatic cell mutation, hepatitis B virus (HBV) DNA integration, and the abnormal activation of telomerase transcription. The key oncogenes and critical pathways are involved in the genesis of the tumor as well. Complex pathogenesis leads to a variety of pathological types and the different sensitivities to treatment, which renders the significantly different prognosis among patients (4). This genomic and pathological inter-individual complexity has given HCC great heterogeneity, which has posed a great challenge to individualized and gene-based treatment of HCC patients.
The role of copy number variation (CNV) in diseases can be determined by traditional genome analysis, but among the neoplastic cell populations within which genetic heterogeneity is very common (5), some information relating to the reconstruction of cell evolutionary lineages might be omitted (6). Furthermore, the origin of a particular mutation can’t be pinpointed therefore unable to elucidate the progress of tumors.
In the past, individual cells with identical phenotypes were regarded as the same functional unit of tissues or organs, but the complex heterogeneities among the cells have been observed through deep sequencing of single-cells (7). At present, single-cell sequencing technology has been able to detect the genome, transcriptome, epigenetics, and other information of a single-cell at different regulatory levels, thereby achieve the information of complex cellular heterogeneity in cancer research. Even though single-cell sequencing technology has made a great leap in the last decade, its applications in HCC are still facing great limitations. This review article aims to give a brief introduction of single-cell sequencing technology, its applications in HCC research, and challenges and limitations that impede the single-cell research of HCC.
Single-cell sequencing technology
Sample preservation
Compared with traditional sequencing, a unique feature of single-cell sequencing is the requirement of obtaining single cells in good status. Common single-cell sequencing technologies were originally designed to use freshly isolated cells, which may avoid the effects of ischemia and hypoxia on tissue and cell status after dissociated from the body. However, due to the limitations of the required infrastructure or specialized equipment during the actual operation, it may be difficult to process the samples in real time. At the same time, simultaneous processing after collecting multiple samples at different times can avoid technical batch effects (8).
Conventional preservation procedures, such as freezing, can cause cell membrane crystallization and disruption, thereby hindering subsequent single cell preparation. However, when preserved with the cryoprotectant dimethyl-sulfoxide at −80 °C or in liquid nitrogen, the cellular structures and integrity of RNA molecules from cell lines or primary tissues can be maintained after months (9). In addition, methanol fixation combined with droplet-based single-cell methods, can also be used to stabilize and preserve isolated cells for weeks while ensuring the accuracy of single-cell RNA sequencing data (10). Sample preservation methods allow the location and time of sampling independent of subsequent processing steps, make complex experimental designs possible and expand the range of accessible specimens.
Isolation of single cells
Single-cell isolation from samples begins with transforming tissues into live cell suspension, especially when managing complex solid tissues mechanic or enzymatic decomposition is a necessary step. Meanwhile, cell viability should be maintained to avoid deviating to specific subgroups (11). The next step is to sort the cells, while this step can be skipped when the rigorous screening of target cells is not mandatory. Eventually, the single cell is directed into the individual reaction chamber for lysis and further processing.
The common techniques for single-cell isolation include micromanipulation, laser capture microdissection, fluorescence-activated cell sorting (FACS), magnetic-activated cell sorting (MACS) and microfluidic control. These techniques have their respective advantages, disadvantages and application fields.
Micromanipulation and laser capture microdissection are low-throughput methods. The basis of micromanipulation is the visual inspection of cell morphology and staining characteristics, and the single cells are separated manually under a microscope. It is simple, low-cost and suitable for suspended cells, despite that identifying cells under a microscope is prone to errors. Optical tweezers is a separation technique combining microscopic spectroscopy and optical trap, and enforced by FACS and microfluidic technology, therefore it can achieve high-throughput automatic separation and cell sorting, as well as avoid DNA contamination (12).
Laser capture microdissection can be used to retrieve cells from fixed tissues or frozen sections. Unlike enzymatic separation techniques, the advantage of laser capture microdissection lies in retaining the information of the spatial location of cells sampled in tissues. The disadvantage is low-throughput and manual operation (13). Moreover, it is easy to damage the cell integrity during collection, and it is impossible to obtain the majority of target cell cytoplasm without mixing components of adjacent cells. Therefore, it is neither suitable for transcriptome analysis (14), nor an ideal method for single-cell isolation (11).
Single-cell isolation by FACS and MACS is based on specific cell characteristics of fluorescent labeling, and specific antigen with magnetic beads binding to cell surface antigens, respectively (15). The main advantage of FACS based on flow cytometry sorting is that it can select preferential and non-preferential isolation, and automatically isolate single cells with high accuracy and throughput (16). However, FACS requires a large number of suspended cells as starting material, which may affect the production of low-abundance cell subpopulations. Furthermore, the high-speed flow in the machine may cause damage to cells, so attention should be paid to the viability of the cells collected. Compared with FACS, MACS has the advantage of requiring less equipment and less time, but it lacks the sensitivity and cell-specificity provided by fluorescent labeling.
Microfluidic technology is a novel single-cell separation technique that fills a microfluidic chip with a controlled liquid flow of micron-diameter channels to isolate different cells. These single cells can be processed and lysed in the specified order for downstream single-cell sequencing (17). Microfluidic technology is expected to become the mainstream technology for future single-cell separation (18).
The process of cell sorting is related to the purpose of the experiment. For experiments that require unbiased access to the sample cells, all cells need to be captured during the separation process, and microfluidic and droplet-based sorting techniques can be used. However, for most experiments, it is necessary to make a biased selection of cells, enrich or exclude some cells, and commonly use FACS and MACS with specific markers.
Single-cell genome sequencing
The major challenge single-cell sequencing technique confronts is that the DNA or RNA content of a single-cell is far below the minimum requirement of the sequencing platform. Moreover, considering there are only 2 copies of DNA molecule in each cell comparing to thousands of copies of most RNA molecules, single-cell genome sequencing is more challenging than transcriptome sequencing (19). Therefore, amplification of whole-genome nucleotides is required before sequencing. According to the purposes of genome sequencing and transcriptome sequencing, it is classified into whole genome amplification (WGA) and whole transcriptome amplification (WTA).
The most common WGA techniques include PCR-based technology based on thermal cycling, such as degenerative-oligonucleotide-PCR (DOP-PCR), non-PCR-based technology based on isothermal reaction, such as multiple-displacement-amplification (MDA), multiple annealing-and looping-based amplification cycles (MALBAC) and linear amplification via transposon Insertion (LIANTI) (Table 1).
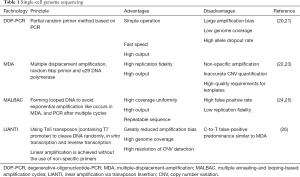
Full table
The primers adopted in DOP-PCR technology contain 6 bp random sequences at the 3’ end, which can bind to the genomic DNA strand randomly, and then amplify the whole genome (20). Because of the exponential amplification feature of PCR, the variation among different sequences in the genome are augmented, resulting in low coverage of the amplified genome and inhomogeneous amplification, which may lead to the loss of information on single nucleotide variation (SNV) and CNV. Due to these defects, DOP-PCR is not optimal for single-cell sequencing. WGA methods with lower amplification deviation and higher fidelity are needed. MDA technique uses a random hexamer primer to react with φ29 DNA polymerase. This polymerase has a strong strand-displacement property and can generate 50–100 kb DNA fragments by amplification under the isothermal conditions. Meanwhile, φ29 DNA polymerase has high replication fidelity, due to its 3’–5’ exonuclease activity and proofreading activity (22). Compared with DOP-PCR technology, MDA technology has higher fidelity and relatively lower amplification bias. However, amplification by MDA is still exponential, so the sequence preference of PCR reaction cannot be avoided, which results in uneven genome coverage and inaccurate CNV quantification. Amplification bias may result in the failure to detect both alleles when genotyping SNV in a diploid human genome from a single cell, resulting in mistaking heterozygous loci as homozygotes (24).
MALBAC technique adopts a quasi-linear amplification process that reduces the sequence preference of the exponential amplification. The amplification primers have 27 nucleotide sequences in common and 8 interchangeable nucleotides which could be homogenously hybridized with templates at 0 °C. At 65 °C, DNA polymerase with chain displacement activity is used to generate semi-proliferators of variable length (0.5 to 1.5 kb), which are then detached from the templates at 94 °C. The amplification of semi-proliferators produces complete amplifiers with complementary terminals. The temperature is recycled to 58 °C to allow the formation of intact amplicons and prevents further amplification and cross hybridization. After 5 pre-amplification cycles, the full amplicon is exponentially amplified by PCR to generate the DNA in micrograms required for next generation sequencing. In PCR, oligonucleotides with 27 common nucleotide sequences are used as primers (25). Sequence preference of MALBAC is repeatable among different cells, and CNV analysis can be performed after the standardization of reference cells. However, since the fidelity of polymerase used in MALBAC is not as ideal as φ29, the false positive rate is higher when detecting SNV. By adjusting the protocol, the false positive rates might be decreased by the way of using a high-fidelity enzyme or a thermostable DNA polymerase with strong proofreading activity (24).
The above techniques are unable to completely avoid the problem of amplification bias, while LIANTI, which uses linear amplification in the genome amplification process, can avoid it. LIANTI combines Tn5 transposition and T7 in vitro transcription (IVT). Tn5 transposons containing T7 promoters randomly insert into single cell genome, and then random DNA fragments undergo IVT conducted by T7 promoter to obtain plentiful linear amplification transcripts. After reverse transcription, the final amplified product is obtained, followed by normal library sequencing. The whole process greatly enhances the stability of amplification because it does not perform exponential amplification. In addition, this technique increases the spatial resolution of the measured copy number by three orders of magnitude, but the C-T mutation is still inevitable due to the deamination of cytosine caused by the cell lysis operation, which can be corrected by comparison with the sequence of the same type of cells (26).
Single-cell transcriptome sequencing
Single-cell transcriptome sequencing includes the following steps: single cell isolation, RNA extraction, reverse transcription, cDNA library construction, and finally sequencing. Similar to genome sequencing, the key is the whole transcriptome amplification of WTA, including the reverse transcription from RNA to cDNA, and the amplification of the cDNA. In order to truly present the single-cell transcriptome, it is critical to reversely transcribe all the RNA into cDNA for linear amplification. However, the PCR amplification of cDNA introduces variability and diversity, especially for the medium- and low-abundance transcript products (27). Therefore, single-cell WTA methods should be focused on reverse transcription of full-length DNA and eliminating amplification bias.
In single-cell sequencing, minimizing procedures and developing single-tube reactions are usually used to avoid partial material loss. The synthesis of cDNA and the selection of polyadenylation RNA types can be performed by reverse transcription. To achieve this, oligo-dT is contained in primers for reverse transcription in almost all the reported methods. With differences in primer components other than oligo-dT, principles and applications vary with different methods (Table 2).
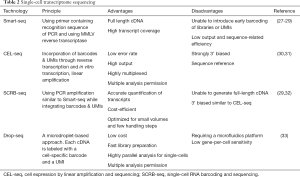
Full table
Smart-seq is an efficient and robust single-cell RNA sequencing method. First, each cell is dissolved in low osmotic solution, and then reverse transcription was initiated using primers containing oligo-dT and PCR downstream amplification sequences. Several untemplated C nucleotides are extended at the 3’ end by MMLV reverse transcriptase. Then introduce primers containing three G nucleotides and PCR upstream amplification sequences. Add conventional primers for PCR, followed by purifying the product for sequencing. Amplified cDNA is used to build a standard Illumina sequencing library, and finally each sequencing library is sequenced on the Illumina platform. Typically, >20 million unique sequencing reads may be generated (27). Smart-seq generates full-length cDNA without knowing the sequence of the mRNA. The technique of Smart-seq and its optimized versions of Smart-seq2 (28), STRT (34), SC3-seq (35) are PCR-based and the amplification is nonlinear. The amplification efficiency is correlated to the sequence. Smart-seq2 introduces template-switching oligonucleotides (TSOs), and adds ISPCR primers at the 3’ end of the cDNA to improve sequencing quality, sensitivity and accuracy, eliminating the need for purification steps (28). The main disadvantage of both Smart-seq is that the generation of full-length cDNA libraries results in the inability to integrate barcodes and UMIs early, thus resulting in lower output (29).
The advantage of linear amplification of cell expression by linear amplification and sequencing (CEL-seq) and massively parallel single-cell RNA-sequencing (MARS-seq) is the low error rate, nonetheless, the sequence preference exists just like PCR. The CEL-seq uses a primer, designed with an anchored polyT, a unique barcode, the 5’ Illumina sequencing adaptor, and a T7 promoter, to reverse transcribe RNA from each cell in a single tube. After cDNA is synthesized, the cDNA is purified and linearly amplified by IVT to form ssRNA by the T7 promoter. Reverse transcription of RNA is conducted by random primers with one end of the sequencing adaptor, and obtain cDNAs with known sequencing adaptors at both ends, through which the library can be completed (30). The need for an additional cycle of reverse transcription of amplified RNA may result in an additional 3’ end coverage deviation (31).
Single-cell RNA barcoding and sequencing (SCRB-seq) uses PCR amplification, which is similar to Smart-seq, but SCRB-seq integrates specific barcodes to distinguish the source of amplified molecules, and quantifies more accurately of transcripts for small volumes. SCRB-seq has been optimized with small volumes and few handling steps. Moreover, SCRB-seq cannot generate full-length cDNA, but enriches RNA 3’ terminal like CEL-seq (32).
Drop-seq uses a microfluidic device to encapsulate the cells and microbeads with DNA barcodes into the droplets. The cells release mRNA in the droplets, hybridize with the primers, and release the single-cell transcriptomes attached to microparticles (STAMPs) after the droplets rupture, amplifying and sequencing analysis. Drop-seq can process thousands of single cells at the same time, and at a low cost (33). inDROP and Drop-seq are fundamentally similar, but with fewer cells per treatment and a higher percentage of captured cells, which is more advantageous for analyzing very small tissue samples (36).
Single-cell epigenetic sequencing
Besides genome and transcriptome, cellular functions also depend on their epigenetic status, including DNA methylation, hydroxymethylation, histone modification, regulation of non-coding RNA, chromatin configuration and chromatin binding structural and regulatory proteins. The difference in the purpose of single-cell epigenetic sequencing determines the choice of technologies with different principles and applications (Table 3).
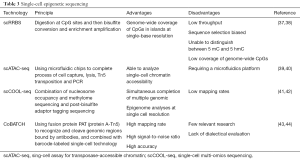
Full table
DNA methylation plays an important role in the regulation of gene expression, and its occurrence near the transcription start site is associated with the decreased expression level of the target gene. The high-throughput DNA methylation sequencing method is based on bisulfite salt and has been used to analyze the genome-wide methylation in single-cell. Single-cell Reduced representation bisulfite sequencing (scRRBS) is a modified protocol from RRBS, which integrates all the experimental procedures into a single-tube reaction prior to PCR amplification. This feature may reduce DNA loss and detect 40% of the CpG sites in a single cell (37). Comparing to single-cell bisulfite sequencing (scBS), scRRBS provides a wider coverage of the CpG islands despite its less coverage of CpG sites. But neither of the techniques is able to distinguish methylation from hydroxymethylation. Both of these two techniques have the disadvantages of low throughput and low coverage (38), therefore a combination of both should be collected.
Assay for transposase-accessible chromatin (ATAC-seq) has been gradually applied in epigenetic histology in recent years; sequencing adapters can be inserted into accessible regions of the genome by transposase Tn5 to label the regulatory regions (39). Moreover, by integrating the ATAC-seq method and single-cell platform C1 of Fluidigm, and using the microfluidic chip to complete the experimental procedures of capture, cleavage, transposition, PCR, etc. scATAC-seq has been established as a research method for single-cell chromatin accessibility analysis (40).
Single-cell multi-omics sequencing (scCOOL-seq) combines nucleosome occupancy and methylome sequencing (NOMe-seq) and post-bisulfite adaptor tagging sequencing (PBAT-seq) to achieve the simultaneous analysis of chromatin state, nucleosome positioning, DNA methylation, CNV and ploidy from the same single cell (41). scCOOL-seq greatly enhances the ability to analyze complex relationships between different layers of genetic and epigenetic regulations within a single cell.
Whole genome profiles of histone modification and DNA binding proteins can be obtained by chromatin immunoprecipitation followed by sequencing (CHIP-seq) (45). Single-cell chromatin immunocleavage sequencing (scCHIC-seq) achieves single-cell level analysis (43), but low throughput, increased sequencing costs, and extremely lower mapping rate, while CoBATCH utilizes the fusion protein PAT (Protein A-Tn5) to recognize and cleave specific genomic regions bound by antibodies, and combined with barcode labeling, high-throughput single-cell capture is achieved (44). Compared with the previous CHIP technologies, it is easier to operate, has a higher signal-to-noise ratio, accuracy and quality.
Application of single-cell sequencing in HCC
Heterogeneity analysis of the HCC cells
The most significant advantage of single-cell sequencing technology compared with traditional sequencing technology lies in the analysis of intercellular heterogeneity.
To simultaneously analyze the mechanism of mutual regulation among the single-cell genome, transcriptome and methylated DNA group, these three factors are integrated into single-cell trio sequencing technology (scTrio-seq), which can be accomplished in the same cell. It was used to analyze 25 cancer cells derived from tissue samples of HCC.
The relationship between promoter DNA methylation and gene expression in individual cells was investigated by using scTrio-seq data. It was found that promoter DNA methylation was negatively correlated with the similarity of corresponding gene expression levels in each HepG2 cell. This negative correlation indicates that expression of the corresponding gene in HepG2 cells is inhibited by a high DNA methylation pattern in the promoter. In addition, DNA methylation in the non-promoter region of the genome was positively correlated with gene expression. Furthermore, when it was moved to the 3’ end of the genome, the positive correlation increased, indicating that DNA methylation in the genome promoted the transcription of these genes.
After analyzing the HCC cells based on CNV patterns, it was found that the DNA copy number, DNA methylation and RNA expression levels of the two cell subpopulations were different. The cell subpopulation occupying a smaller portion of the tumor tissue had more copy gain, allowing itself to express more invasive cell markers, and have a higher chance to escape from immune surveillance (46).
This research is a successful attempt to perform single-cell sequencing on HCC cells, while there are evident limitations. Twenty-five HCC cells were isolated, however, there were no adjacent peritumor tissues (APTs) cells for comparison, and the differential manifestation of DNA methylation, gene expression and CNV patterns are what of real importance for HCC research. Meanwhile, only a limited number of 25 HCC cells were isolated from the numerous cells in HCC tissue, but they are not necessarily representative.
Reconstruction of HCC cell lineage
Identifying multiple samples from tumors by single-cell sequencing may provide the information about the evolutionary pathways, and at the same time, based on the comparison between the mutations of different samples, it is possible to find out how key mutations accumulate and evolve in the lineage to form heterogeneous tumors (47).
In a previous study, 96 tumor cells (30–36 cells per patient) and 15 normal hepatocytes (5 cells per patient) were collected from 3 male patients with HBV-related HCC. These three patients presented morphological features of visible gross portal vein tumor thrombus, multifocal tumors, and lobular multiple nodules, respectively (48).
Analysis of adjacent trees by CNV showed that HCC cells were significantly different from normal hepatocytes. Five HBV integration sites were identified by sequencing in cells of the patient with portal vein tumor thrombus. It was found that all the 5 integrations were present in each tumor cell and cells in the portal vein tumor thrombus, but not in normal cells. This indicated that the tumor originated from the monoclonal amplification of the HBV-integrated progenitor tumor cells. It also proved that portal vein tumor thrombus, as a feature of advanced cancer stage, originated from the tumor clone at the single-cell level.
In the patient with multifocal tumors, all the tumor cells from the two isolated lesions showed identical HBV integration sites, suggesting that the two lesions most likely originated from a single clone after early intrahepatic metastasis and obtaining sufficient growth advantage.
Unlike the two patients above, cells from the patient with lobular multiple nodules exhibited different CNV profiles. That reflected the polyclonal origin of the tumor and the mutagenic field effect of chronic hepatitis in the development of HCC, which resulted in an independent genetic lineage that barely shares any initial genetic changes (48).
Even if the number is still limited, not only HCC cells but also normal liver cells were isolated from three patients with different manifestations of HCC. ZNF717 was identified and verified as a tumor suppressor, however, it was poorly correlated with the other observations in the lineage of HCC.
The revelation of the immune microenvironment of HCC
In recent years, cancer immunotherapy has greatly changed the situation of cancer treatment (49). Although checkpoint blockade may leads to significant clinical response (50), it is inconsistent in different patients and types of cancer, while the immunotherapy treatment regimens for patients with HCC are limited and the clinical success rate is low (51). Therefore, it is important to obtain reliable predicting markers of therapeutic response. For example, an in-depth understanding of the mechanisms which cause increased CD8+ T cell depletion and Treg accumulation in cancer will provide better strategies for coordinating the immune system to eradicate cancer.
In a previous study, 5,063 T cells were isolated from peripheral blood, tumors and peritumor tissues of 6 patients with HCC, and 11 T cell subsets were screened by deep sequencing of single-cell transcriptome analysis. It was found that depleted CD8+ T cells and Treg cells were preferentially enriched in HCC, and there might be clonal amplification. By identifying the signaling genes of each T cell subset, it was found that the expression of the LAYN gene was up-regulated in activated CD8+ T cells and Treg cells (52).
In addition to exploring the immune microenvironment of HCC, single-cell sequencing can also be used to investigate the differences in immune status between HCC and its preceding diseases, such as chronic hepatitis B and steatohepatitis.
By comparing the CD8+ T cells isolated from the tissues of chronic hepatitis B and HCC, it was found that the latter had higher expression level of depletion markers, such as PD-1, TIM-3, LAG-3, and CTLA-4, which indicated that there were differences in cell phenotype and functional status between the two types of cells. The depletion level of CD8+ T cells in HCC was higher than that in chronic hepatitis B (53).
Obesity is an important risk factor of HCC, which is frequently observed to be accompanied by steatohepatitis. Abnormal accumulation of myeloid cells derived from bone marrow occurs in obesity-related steatohepatitis patients. To understand the mechanism, obesity model mice fed with “Western diet” was sacrificed to obtain myeloid cells from the liver and bone marrow, on which single-cell RNA-seq was performed. It was observed that monocyte derivatives were enriched in both the bone marrow and liver myeloid populations. Moreover, the liver myeloid cells were in a unique inflammatory status, where inflammatory calprotectin (S100A8/A9) was at a decreased level in macrophages and dendritic cells (54).
Detection of circulating tumor cells (CTC) of HCC
Most studies investigating CTC in HCC suggest direct associations between increased CTC counts and poor clinical outcomes (55,56). Considering the limited number of CTC in blood, the maximization of the detection rate of CTC can be tried by following the steps of CD45-negative enrichment, screening patients by imaging flow cytometry and performing scRNA seq. After single-cell transcriptome sequencing of the obtained CD45-negative cells, CTC cells with significantly different gene expression profiles from other clonal populations in the blood can be identified by comparing the variability of gene expression profiles, and bile acids and xenobiotic metabolism were the top-most enriched genomes in these cells. CD45-negative selection increases the ratio of CTC to non-CTC to approximately 1 CTC per 3,000 nucleated cells, and subsequent scRNA-seq is critical for accurate differentiation of CTCs using whole transcriptome data (57).
The application potential of single-cell sequencing in biomarker detection like CTC is undeniable, although this application has a higher requirement for accuracy, specificity and sensitivity, which points out the direction of further improvements.
Unraveling the structure and development of normal liver
Besides the tumor tissues, the physiological characteristics of normal livers should be better understood to establish a solid background for HCC research and treatment, in terms of both the structure and development.
A single-cell transcriptome sequencing of 8,444 cells in the human liver was conducted, and hepatocytes, endothelial cells, cholangiocytes, hepatic stellate cells (HSCs) and multiple sets of immune cells were sorted and clustered by using the t-SNE algorithm. The landscape of cells in the liver and signatures of specific cell subsets were elucidated, while there is still a potential of further analysis, such as cell lineage. A major limitation is an additional bias due to susceptibility of hepatocytes to mechanical forces in single-cell isolation, which weaken the strength of the landscape of liver resident cells and might be the reason why macrophage populations were studied more profoundly in this study (58).
Efforts have been made to unravel the development of liver parenchymal tissues with the help of single-cell RNA sequencing, which reveals the role of progenitor cells in the development of specific structures in the liver. Hepatobiliary hybrid progenitor (HHyP) cells in the fetus were identified to be distinct from hepatocytes and cholangiocytes and possess the gene signatures of both the hepatocytes and cholangiocytes lineages (59). There should be further attempts to find HHyP-related cells in adults or to verify the cell lineage in the mature hepatocytes and cholangiocyte populations.
Existing challenges and prospects
Single-cell sequencing technology point in a new direction for cancer research at the omics level. It provides research methods for analyzing the tumor heterogeneity, helps to improve the understanding of the pathogenesis of tumors, and enriches the methods of diagnosis and treatment in clinical practice. Single-cell sequencing technology is continuously improving, and it has been applied increasing frequently in cancer research. Cellular indexing of transcriptomes and epitopes by sequencing (CITE-seq), which combines highly multiplexed protein marker detection with unbiased transcriptome profiling for thousands of single cells (60), provides a large-scale and multi-dimensional analysis of single cells. The ability to refine the analysis of cell populations can be used to detect single tumor cells and multiple different immune cell pools that can infiltrate tumor tissue in tumor samples. Spatial transcriptomics enables spatial resolution and quantitative analysis of transcriptomes in tissue sections by placing histological sections on reverse transcription primers with position-specific barcodes (61). Hepatocytes operate in highly structured repeating anatomical units termed liver lobules (62). Obtaining the complete gene expression profile of a single hepatocyte at the lobule coordinates will help to model the liver’s response to various perturbations in detail (63), and help to understand the multiple components of tumor progression and therapy outcome (64), which may be the same in HCC.
There are still technical issues to be solved in single-cell sequencing technology, such as reliability and efficiency of single-cell separation, contamination avoidance of surrounding cells. Even though great challenges still existing, it could be expected that the reliability, efficiency, sensitivity and accuracy of single-cell sequencing will be improved with the development of relevant technologies.
With the help of single-cell sequencing technology, the role of specific types of cells in the development of tumors should be elucidated. For example, hepatic stellate cells (HSCs) known to differentiate to secrete collagen in the progression of liver fibrosis, has been proved to have great molecular heterogeneity that is functionally relevant to this disease, by using single-cell RNA-seq method (65).
When a single-cell sequencing method is widely applicable to different organs, tissues and cells, there should be higher-dimensional research into the cells that may have the same progenitor but different phenotypes and in different compartments of the body. Nowadays this idea has been realized in the research of the Natural killer (NK) cell subsets in blood and spleen (66). The liver, a vital organ in metabolism and immunity, should be taken into consideration as well.
In the future, single-cell sequencing has the potential to serve as a novel platform for research of biomedical research and a technological basis for individualized diagnosis and therapy, it is likely to be where advanced genomic technology and clinical medicine meet, in other words, a promising attempt of the idea of translational medicine.
Acknowledgments
Funding: This work is supported by grants from the Beijing Natural Science Foundation (L172055), the Beijing Municipal Science & Technology Commission research fund (Z171100000417004), WBE Liver Fibrosis Foundation (CFHPC 2020021) and Beijing Dongcheng District outstanding talent funding project.
Footnote
Conflicts of Interest: The authors have no conflicts of interest to declare.
Ethical Statement: The authors are accountable for all aspects of the work in ensuring that questions related to the accuracy or integrity of any part of the work are appropriately investigated and resolved.
References
- Lipinski KA, Barber LJ, Davies MN, et al. Cancer Evolution and the Limits of Predictability in Precision Cancer Medicine. Trends Cancer 2016;2:49-63. [Crossref] [PubMed]
- Fidler IJ, Kripke M. Metastasis results from preexisting variant cells within a malignant tumor. Science 1977;197:893-5. [Crossref] [PubMed]
- Wallace MC, Preen D, Jeffrey GP, et al. The evolving epidemiology of hepatocellular carcinoma: a global perspective. Expert Rev Gastroenterol Hepatol 2015;9:765-79. [Crossref] [PubMed]
- Shibata T, Aburatani H. Exploration of liver cancer genomes. Nat Rev Gastroenterol Hepatol 2014;11:340-9. [Crossref] [PubMed]
- Park SY, Gonen M, Kim HJ, et al. Cellular and genetic diversity in the progression of in situ human breast carcinomas to an invasive phenotype. J Clin Invest 2010;120:636-44. [Crossref] [PubMed]
- Navin N, Kendall J, Troge J, et al. Tumour evolution inferred by single-cell sequencing. Nature 2011;472:90-4. [Crossref] [PubMed]
- Eberwine J, Sul JY, Bartfai T, et al. The promise of single-cell sequencing. Nat Methods 2014;11:25-7. [Crossref] [PubMed]
- Lafzi A, Moutinho C, Picelli S, et al. Tutorial: guidelines for the experimental design of single-cell RNA sequencing studies. Nat Protoc 2018;13:2742-57. [Crossref] [PubMed]
- Guillaumet-Adkins A, Rodriguez-Esteban G, Mereu E, et al. Single-cell transcriptome conservation in cryopreserved cells and tissues. Genome Biol 2017;18:45. [Crossref] [PubMed]
- Alles J, Karaiskos N, Praktiknjo SD, et al. Cell fixation and preservation for droplet-based single-cell transcriptomics. BMC Biol 2017;15:44. [Crossref] [PubMed]
- Gawad C, Koh W, Quake SR. Single-cell genome sequencing: current state of the science. Nat Rev Genet 2016;17:175-88. [Crossref] [PubMed]
- Landry ZC, Giovanonni SJ, Quake SR, et al. Optofluidic cell selection from complex microbial communities for single-genome analysis. Methods Enzymol 2013;531:61-90. [Crossref] [PubMed]
- Shapiro E, Biezuner T, Linnarsson S. Single-cell sequencing-based technologies will revolutionize whole-organism science. Nat Rev Genet 2013;14:618-30. [Crossref] [PubMed]
- Espina V, Wulfkuhle JD, Calvert VS, et al. Laser-capture microdissection. Nat Protoc 2006;1:586-603. [Crossref] [PubMed]
- Sutermaster BA, Darling EM. Considerations for high-yield, high-throughput cell enrichment: fluorescence versus magnetic sorting. Sci Rep 2019;9:227. [Crossref] [PubMed]
- Dalerba P, Kalisky T, Sahoo D, et al. Single-cell dissection of transcriptional heterogeneity in human colon tumors. Nat Biotechnol 2011;29:1120-7. [Crossref] [PubMed]
- Kellogg RA, Gomez-Sjoberg R, Leyrat AA, et al. High-throughput microfluidic single-cell analysis pipeline for studies of signaling dynamics. Nat Protoc 2014;9:1713-26. [Crossref] [PubMed]
- Liang J, Cai W, Sun Z. Single-cell sequencing technologies: current and future. J Genet Genomics 2014;41:513-28. [Crossref] [PubMed]
- Wang Y, Navin NE. Advances and applications of single-cell sequencing technologies. Mol Cell 2015;58:598-609. [Crossref] [PubMed]
- Telenius Hk, Carter NP, Bebb CE, et al. Degenerate oligonucleotide-primed PCR: General amplification of target DNA by a single degenerate primer. Genomics 1992;13:718-25. [Crossref] [PubMed]
- Blagodatskikh KA, Kramarov VM, Barsova EV, et al. Improved DOP-PCR (iDOP-PCR): A robust and simple WGA method for efficient amplification of low copy number genomic DNA. PLoS One 2017;12:e0184507. [Crossref] [PubMed]
- Dean FB, Nelson JR, Giesler TL, et al. Rapid amplification of plasmid and phage DNA using Phi 29 DNA polymerase and multiply-primed rolling circle amplification. Genome Res 2001;11:1095-9. [Crossref] [PubMed]
- He F, Zhou W, Cai R, et al. Systematic assessment of the performance of whole-genome amplification for SNP/CNV detection and beta-thalassemia genotyping. J Hum Genet 2018;63:407-16. [Crossref] [PubMed]
- Lasken RS. Single-cell sequencing in its prime. Nat Biotechnol 2013;31:211-2. [Crossref] [PubMed]
- Zong C, Lu S, Chapman AR, et al. Genome-wide detection of single-nucleotide and copy-number variations of a single human cell. Science 2012;338:1622-6. [Crossref] [PubMed]
- Chen C, Xing D, Tan L, et al. Single-cell whole-genome analyses by Linear Amplification via Transposon Insertion (LIANTI). Science 2017;356:189-94. [Crossref] [PubMed]
- Ramsköld D, Luo S, Wang YC, et al. Full-length mRNA-Seq from single-cell levels of RNA and individual circulating tumor cells. Nat Biotechnol 2012;30:777-82. [Crossref] [PubMed]
- Picelli S, Bjorklund AK, Faridani OR, et al. Smart-seq2 for sensitive full-length transcriptome profiling in single cells. Nat Methods 2013;10:1096-8. [Crossref] [PubMed]
- Ziegenhain C, Vieth B, Parekh S, et al. Comparative Analysis of Single-Cell RNA Sequencing Methods. Mol Cell 2017;65:631-43.e4. [Crossref] [PubMed]
- Hashimshony T, Wagner F, Sher N, et al. CEL-Seq: single-cell RNA-Seq by multiplexed linear amplification. Cell Rep 2012;2:666-73. [Crossref] [PubMed]
- Kolodziejczyk AA, Kim JK, Svensson V, et al. The technology and biology of single-cell RNA sequencing. Mol Cell 2015;58:610-20. [Crossref] [PubMed]
- Soumillon M, Cacchiarelli D, Semrau S, et al. Characterization of directed differentiation by high-throughput single-cell RNA-Seq. bioRxiv 2014. [Crossref]
- Macosko EZ, Basu A, Satija R, et al. Highly Parallel Genome-wide Expression Profiling of Individual Cells Using Nanoliter Droplets. Cell 2015;161:1202-14. [Crossref] [PubMed]
- Islam S, Kjallquist U, Moliner A, et al. Highly multiplexed and strand-specific single-cell RNA 5' end sequencing. Nat Protoc 2012;7:813-28. [Crossref] [PubMed]
- Nakamura T, Yabuta Y, Okamoto I, et al. SC3-seq: a method for highly parallel and quantitative measurement of single-cell gene expression. Nucleic Acids Res 2015;43:e60. [Crossref] [PubMed]
- Klein AM, Mazutis L, Akartuna I, et al. Droplet barcoding for single-cell transcriptomics applied to embryonic stem cells. Cell 2015;161:1187-201. [Crossref] [PubMed]
- Guo H, Zhu P, Wu X, et al. Single-cell methylome landscapes of mouse embryonic stem cells and early embryos analyzed using reduced representation bisulfite sequencing. Genome Res 2013;23:2126-35. [Crossref] [PubMed]
- Guo H, Zhu P, Guo F, et al. Profiling DNA methylome landscapes of mammalian cells with single-cell reduced-representation bisulfite sequencing. Nat Protoc 2015;10:645-59. [Crossref] [PubMed]
- Buenrostro JD, Giresi PG, Zaba LC, et al. Transposition of native chromatin for fast and sensitive epigenomic profiling of open chromatin, DNA-binding proteins and nucleosome position. Nat Methods 2013;10:1213-8. [Crossref] [PubMed]
- Buenrostro JD, Wu B, Litzenburger UM, et al. Single-cell chromatin accessibility reveals principles of regulatory variation. Nature 2015;523:486-90. [Crossref] [PubMed]
- Guo F, Li L, Li J, et al. Single-cell multi-omics sequencing of mouse early embryos and embryonic stem cells. Cell Res 2017;27:967-88. [Crossref] [PubMed]
- Gu C, Liu S, Wu Q, et al. Integrative single-cell analysis of transcriptome, DNA methylome and chromatin accessibility in mouse oocytes. Cell Res 2019;29:110-23. [Crossref] [PubMed]
- Ku WL, Nakamura K, Gao W, et al. Single-cell chromatin immunocleavage sequencing (scChIC-seq) to profile histone modification. Nat Methods 2019;16:323-5. [Crossref] [PubMed]
- Wang Q, Xiong H, Ai S, et al. CoBATCH for High-Throughput Single-Cell Epigenomic Profiling. Mol Cell 2019;76:206-16.e7. [Crossref] [PubMed]
- Furey TS. ChIP-seq and beyond: new and improved methodologies to detect and characterize protein-DNA interactions. Nat Rev Genet 2012;13:840-52. [Crossref] [PubMed]
- Hou Y, Guo H, Cao C, et al. Single-cell triple omics sequencing reveals genetic, epigenetic, and transcriptomic heterogeneity in hepatocellular carcinomas. Cell Res 2016;26:304-19. [Crossref] [PubMed]
- Kim KI, Simon R. Using single cell sequencing data to model the evolutionary history of a tumor. BMC Bioinformatics 2014;15:27. [Crossref] [PubMed]
- Duan M, Hao J, Cui S, et al. Diverse modes of clonal evolution in HBV-related hepatocellular carcinoma revealed by single-cell genome sequencing. Cell Res 2018;28:359-73. [Crossref] [PubMed]
- Sharma P, Allison JP. Immune checkpoint targeting in cancer therapy: toward combination strategies with curative potential. Cell 2015;161:205-14. [Crossref] [PubMed]
- Topalian SL, Drake CG, Pardoll DM. Immune checkpoint blockade: a common denominator approach to cancer therapy. Cancer Cell 2015;27:450-61. [Crossref] [PubMed]
- Prieto J, Melero I, Sangro B. Immunological landscape and immunotherapy of hepatocellular carcinoma. Nat Rev Gastroenterol Hepatol 2015;12:681-700. [Crossref] [PubMed]
- Zheng C, Zheng L, Yoo JK, et al. Landscape of Infiltrating T Cells in Liver Cancer Revealed by Single-Cell Sequencing. Cell 2017;169:1342-56.e16. [Crossref] [PubMed]
- Wang X, He Q, Shen H, et al. Genetic and phenotypic difference in CD8+ T cell exhaustion between chronic hepatitis B infection and hepatocellular carcinoma. J Med Genet 2019;56:18-21. [Crossref] [PubMed]
- Krenkel O, Hundertmark J, Abdallah AT, et al. Myeloid cells in liver and bone marrow acquire a functionally distinct inflammatory phenotype during obesity-related steatohepatitis. Gut 2019. [Epub ahead of print]. [Crossref] [PubMed]
- Labgaa I, Villanueva A. Liquid biopsy in liver cancer. Discov Med 2015;19:263-73. [PubMed]
- Fan JL, Yang YF, Yuan CH, et al. Circulating Tumor Cells for Predicting the Prognostic of Patients with Hepatocellular Carcinoma: A Meta Analysis. Cell Physiol Biochem 2015;37:629-40. [Crossref] [PubMed]
- D'Avola D, Villacorta-Martin C, Martins-Filho SN, et al. High-density single cell mRNA sequencing to characterize circulating tumor cells in hepatocellular carcinoma. Sci Rep 2018;8:11570. [Crossref] [PubMed]
- MacParland SA, Liu JC, Ma XZ, et al. Single cell RNA sequencing of human liver reveals distinct intrahepatic macrophage populations. Nat Commun 2018;9:4383. [Crossref] [PubMed]
- Segal JM, Kent D, Wesche DJ, et al. Single cell analysis of human foetal liver captures the transcriptional profile of hepatobiliary hybrid progenitors. Nat Commun 2019;10:3350. [Crossref] [PubMed]
- Stoeckius M, Hafemeister C, Stephenson W, et al. Simultaneous epitope and transcriptome measurement in single cells. Nat Methods 2017;14:865-8. [Crossref] [PubMed]
- Ståhl PL, Salmen F, Vickovic S, et al. Visualization and analysis of gene expression in tissue sections by spatial transcriptomics. Science 2016;353:78-82. [Crossref] [PubMed]
- Ben-Moshe S, Itzkovitz S. Spatial heterogeneity in the mammalian liver. Nat Rev Gastroenterol Hepatol 2019;16:395-410. [Crossref] [PubMed]
- Moor AE, Itzkovitz S. Spatial transcriptomics: paving the way for tissue-level systems biology. Curr Opin Biotechnol 2017;46:126-33. [Crossref] [PubMed]
- Thrane K, Eriksson H, Maaskola J, et al. Spatially Resolved Transcriptomics Enables Dissection of Genetic Heterogeneity in Stage III Cutaneous Malignant Melanoma. Cancer Res 2018;78:5970-9. [PubMed]
- Krenkel O, Hundertmark J, Ritz TP, et al. Single Cell RNA Sequencing Identifies Subsets of Hepatic Stellate Cells and Myofibroblasts in Liver Fibrosis. Cells 2019. [Crossref] [PubMed]
- Crinier A, Milpied P, Escaliere B, et al. High-Dimensional Single-Cell Analysis Identifies Organ-Specific Signatures and Conserved NK Cell Subsets in Humans and Mice. Immunity 2018;49:971-86.e5. [Crossref] [PubMed]