Respiratory parameters and acute kidney injury in acute respiratory distress syndrome: a causal inference study
Introduction
Acute kidney injury (AKI) is common in critically ill patients and is associated with poor outcomes (1). Several risk factors are associated with AKI in critically ill patients including hypovolemia, sepsis, comorbidities and nephrotoxic drug exposure (2,3). Increasing evidence also suggests a deleterious interaction between lung and kidney dysfunctions. AKI is now recognized as the most common organ dysfunction in acute respiratory distress syndrome (ARDS) patients, thus prolonging the duration of MV (MV) and affecting mortality (4-7). Several studies had evaluated the association between respiratory failure and AKI, including an increase in AKI odds-ratio associated with MV in critically ill patients (8). Additionally, in a large study, it was demonstrated that both MV and ARDS were independently associated with subsequent occurrence of AKI (4).
Several experimental studies in humans and animals have explored the pathophysiological mechanisms of lung-kidney interactions, suggesting some pathways to explain the deleterious effects of ARDS/MV on renal function (9-11). First, positive-pressure ventilation may reduce cardiac output and increase central venous pressure, thereby diminishing renal blood flow, free water clearance, or the glomerular filtration rate (12). Additionally, both hypoxemia and hypercapnia have been associated with increased renal resistance even in critically ill patients exposed to moderate levels of hypoxemia (10). Finally, emerging data suggest that ventilator-induced lung injury may not only affect the lung but may also lead to further systemic inflammation via the release of inflammatory cytokines (13).
Despite a body of evidence suggesting clinical and physiological interactions between ARDS and AKI, there are scarce data about respiratory and MV-related parameters effecting renal function in ARDS patients. A meta-analysis (8), including studies with different levels of tidal volume and PEEP, found no association between ventilator parameters and AKI. However, the included studies were not specifically designed to determine whether MV has an effect on renal function; therefore, a large diversity in such studies, study designs and different definitions of AKI were combined. Moreover, in some cases, it was not possible to determine from the published material definitively whether AKI was present at the start of MV or developed after MV was initiated. Another retrospective study (14), including patients with and without ARDS, found no significant association; however, this study evaluated several correlated variables in the same multivariate model, potentially leading to over-adjustment and a bias to including false cofounders.
The primary objective of this study was to assess the respirator-related parameters associated with subsequent AKI in mechanically ventilated patients with ARDS. Since MV has many correlated and mathematically-linked parameters, we chose a causal diagram approach (15,16) to better select the confounding variable and to evaluate if there are ventilatory and/or hemodynamic mediators between MV and AKI association.
Methods
Multiparameter intelligent monitoring in intensive care III database and data collection
The Multiparameter Intelligent Monitoring in Intensive Care (MIMIC-III) project (17), maintained by the Massachusetts Institute of Technology Laboratory for Computational Physiology, contains data on patients hospitalized in an ICU at Beth Israel Deaconess Medical Center from 2001 to 2012. The database is freely available, so that any researcher who accepts the data use agreement and has attended “protecting human subjects training” can apply for permission to access the data (see Supplemental File). This study was approved by the institutional review boards of Massachusetts Institute of Technology and Beth Israel Deaconess Medical Center and was granted a waiver of informed consent.
We included all patients 15 years and older who received ventilation for at least 48 hours on their first interval of MV and had ARDS [defined as a PaO2/FiO2 ratio <300 mmHg in the absence of cardiogenic pulmonary edema (18)] in the first 48 h of MV. Patients with cardiogenic pulmonary edema were identified using ICU admission diagnosis and/or trough a Natural Language Processing (NLP) algorithm that searched in discharge summaries those whom were admitted due cardiogenic pulmonary edema or those with a left ventricular ejection fraction (LVEF) less than 40%. We performed a validation of the NLP algorithm through a formal examination of the discharge summaries of 100 random patients and a concordance of 98%. Patients were excluded from all analyses if they lacked hospital admission information, if they underwent ventilation with any modes other than volume control ventilation (VCV) for the first 48 h of MV, or if they had no plateau pressure (PPLAT) or tidal volume (Vt) measured in the first 48 h of MV. We also had to exclude patients who had missing height measurements, as we could not calculate the predicted body weight and, consequently, could not correct tidal volume by bodyweight. Patients with known end-stage renal disease (ESRD), previous renal transplantation, those who underwent renal replacement therapy (RRT) before or up to day 1 of MV initiation, and those with serum creatinine (SCr) >4 mg/dL at MV initiation were also excluded.
Data collection
All data were extracted from the MIMIC-III database (v1.4) and included demographic information (e.g., age, gender, height), sepsis diagnosis as described by Angus et al. (19), Elixhauser comorbidity index (20), SCr at MV initiation and disease severity score assessed by the simplified acute physiology score (SAPS II). We calculated predicted body weight using the patient’s height and sex (21). Additionally, arterial blood gas analyses in the first 48 h of MV were evaluated and the worst PaO2, PaCO2 and PaO2/FiO2 ratio (when PEEP was at least 5 cmH2O) were selected. We collected daily SCr up to day 7 after MV initiation, urine output, need for vasoactive drugs, exposure to nephrotoxic drugs (amphotericin, vancomycin, aminoglycoside – median daily doses are shown in Table S1), fluid balance before MV initiation and need of RRT.
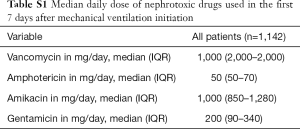
Full table
Exposure variables
The exposure variables were the time-weighted PEEP, ΔP, plateau pressure (PPlat) and tidal volume (Vt) corrected for predicted body weight [calculated using the patient’s height and sex (21)] in the first 48 h of MV. The values were calculated by averaging the available values at each min during the first 48 h of MV. ΔP was calculated from PPlat-PEEP. The min by min value was obtained by carrying each observation forward until the next observation was recorded (22). The variable with the least available values was PPlat (median 8, IQR 5–9) in the first 48 h of MV. Additionally, we hypothesized that parameters of ARDS severity were related to AKI and we also investigated the worst PaO2, PaCO2, and PaO2/FiO2 ratio in the first 48 h and time-weighted the average respiratory system compliance (Crs). We calculated static compliance from VT and ΔP (VT/ΔP). The time-weighted averages for both variables were calculated by averaging the min by min values as already described. The other collected variables were included as confounders in predicting AKI (baseline SCr, age, gender, sepsis, SAPS II, need for vasoactive drugs, fluid balance before day 1 of MV and exposure to nephrotoxic drugs). Additional analyses were performed in 2 nested subcohorts with available central venous pressure (CVP) and mean pulmonary artery pressure (PAP). In these patients, time-weighted CVP, mean PAP and mean systemic arterial pressure (SAP) were calculated as specified above to evaluate if hemodynamic parameters that could be modified by MV would act as mediator in the causal association between exposure and outcomes.
Outcomes
Severe AKI was defined according to the Kidney Disease Improving Global Outcome (KDIGO) criteria (23) stage II or III using SCr and urine output criteria. Briefly, SCr levels were used to classify AKI stage, since our objective was to evaluate the effects of ARDS and MV on renal function, the SCr before or after 12 h of MV initiation was used as baseline renal function. We classified patients based on the KDIGO maximum stage achieved during the first 7 days after MV. Patients who received RRT during this period were considered as AKI stage 3. Urine output was assessed every hour. We used the urine output criterion for patients with at least 12 consecutive hours of valid output measurements. In patients with insufficient urine output measurements, only the SCr criterion was applied. Additionally, 28-day mortality was evaluated to validate our data collection and our model. We expected to find an independent association between ΔP and 28-day mortality in these patients.
Directed acyclic graph
Since MV parameters have many correlated variables and mathematical linking (for example, Crs is calculated from VT and ΔP; PPlat is the sum of ΔP and PEEP), to avoid over-adjustment of the model and permitting the exploration of the total and mediated effect of each exposure variables, we performed a directed acyclic graph (DAG) using the dagitty tool - www.dagitty.net (24). Briefly, a causal diagram is a graphical tool that enables the visualization of the relationships between the exposure of interest, the outcome being studied, and all other variables that are associated in some way with at least two other variables in the diagram. DAG is a special form of causal diagram that does not contain any directed cycles. A comprehensive review can be found in recent reviews (15,16).
In our DAG construction (Figure 1), we had the following assumptions: (I) because we studied only the first 48 h of MV, we assumed that all respiratory and blood gas variables could be influenced by lung disease severity, here represented by Crs (i.e., PaO2, PaCO2, PEEP, Vt, PaO2/FiO2 and ΔP could be, at least partly, be determined by Crs); (II) all other clinical variables (age, gender, Elixhauser comorbidity index, fluid balance before MV, baseline renal function, SAPS II, nephrotoxic drugs, vasoactive drugs and sepsis) were each one independently considered confounders of all considered exposures in the causal association with severe AKI. This assumption is based on previous knowledge (for example, presence of sepsis can be associated with both respiratory and renal failure) or in the absence of data supporting the exclusion of any possible association, i.e., it is impossible to exclude the association of nephrotoxic drugs and a higher PEEP, even a third unmeasured confounder, like severe lung infection, for example. Additionally, (III) if possible, if relationships between two variables were bidirectional, they were modified according to evaluated exposures, making them real confounders. These interactions are represented in Figure 1 as connectors without arrow. Finally, (IV) all respiratory related variables, except for PaO2/FiO2 ratio, were considered potential causes of severe AKI (see Supplemental file). The variables included in the adjustment of each exposure are described in the Table S2.
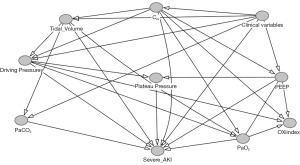
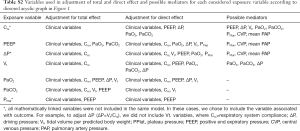
Full table
Statistical analysis
Normality of the data was assessed, and are reported as the mean and standard deviation (SD) or median and IQR (25th–75th percentiles), when appropriate. Baseline characteristics were compared using a 2-sample t-test or Mann-Whitney test for continuous variables, whereas dichotomous variables were assessed with χ2 test or Fisher’s exact test. Simple correlations between continuous variables were analyzed using Spearman’s rank correlation coefficient. Non-normal distributions were natural log-transformed for additional analysis. First, we evaluated the total effect of each exposure on severe AKI using multivariable logistic regression. If there was any significant causal association between the exposure and severe AKI, we evaluated possible mediators to determine if such an association was, at least partly, mediated. In the mediation analyses, we used conditional mean models for nested counterfactuals outcomes with an imputation-based approach. We used R program 3.5 to run analyses using the Medflex package (25).
Results
Population characteristics
The MIMIC-III database contains the records of 46,520 patients, of which 38,605 were aged ≥15 years at the time of admission. Of the 5,226 patients who received MV for a minimum of 48 h, 3,147 received modes of ventilation other than VCV in the first 48 h of MV, had cardiogenic pulmonary edema or a LVEF less than 40% and were excluded. In addition, 108 patients were excluded because there were no arterial blood gas analyses available in the first 48 h of MV. Of the remaining 2,028 patients, 1,750 had the criteria diagnosis of ARDS. Additionally, 453 patients were missing a height measurement, 67 patients had a SCr at MV initiation greater than 4 mg/dL and 31 other patients lacking MV parameters (see Figure 2) were excluded. After all exclusions, 1,142 patients were included in the final analyses. Characteristics of these patients according to severe AKI development are shown in Table 1.
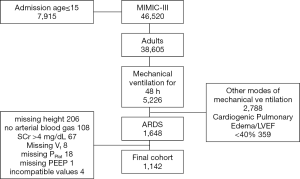
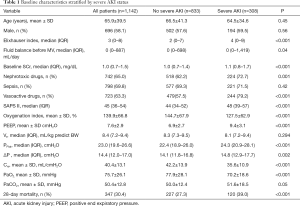
Full table
Causal association between respiratory-related parameters and severe AKI
Using our DAG assumptions (Figure 1), we adjusted respiratory-related exposures to different real confounders to determine if there was a causal association between considered exposures and severe AKI. As stated in the methods, all non-respiratory clinical variables were used in adjustment of all exposures. A complete list of adjusted variables for each evaluated exposure is shown in Table S2.
After evaluating all respiratory-related exposure variables (Table 2), low Crs and high PEEP levels had significant causal association with severe AKI. Each 5-mL/cmH2O increase in Crs reduced the chance of severe AKI by 10% (OR 0.90, 95% CI: 0.84–0.94), and each 1-cmH2O increase in PEEP increased the chance of severe AKI by 5% (OR 1.05, 95% CI: 1.03–1.10), as shown in Figure 3. Additionally, low PaO2 had trends in causal association with severe AKI, as shown in Table 2. Although ΔP has recently been associated with mortality in ARDS patients, even using a parsimonious model considering our assumptions, ΔP was not associated with severe AKI. Additionally, PPlat and Vt had no significant causal association with severe AKI.
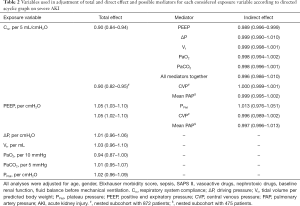
Full table
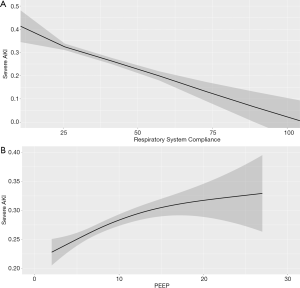
Direct and indirect effects of Crs and PEEP on severe AKI
To further explore the associations between respiratory-related variables and severe AKI, we explored if the causal association between low Crs and high PEEP on severe AKI had any mediators. According to our assumed DAG, effects of Crs on severe AKI could be, at least partly, mediated by PEEP, Vt, PPlat, ΔP, PaO2 and/or PaCO2. From these, we could identify only PEEP as having a significant mediation effect on the causal association between Crs and severe AKI. Although significant, the indirect effect through PEEP was minimal. Moreover, even including all possible mediators in the same analyses, the large effect of Crs was direct, as shown in Table 2. We also examined whether the PPlat mediated the effects of PEEP, but we could not identify any significant mediation.
In two nested subcohorts, we further tested if CVP (n=872) or mean PAP (n=475) could mediated the causal association between low Crs and high PEEP on severe AKI. We did not identify any significant mediation of these hemodynamic parameters on the causal association between Crs and severe AKI—see Table 2.
DAG validation and sensitivity analyses
Because our findings rely upon DAG assumptions, we performed additional analyses. First, we sought to replicate the recently demonstrated causal association between ΔP and mortality using our suggested DAG. Although ΔP had no causal association with severe AKI, no other respiratory-related variable, except ΔP, was independently associated with 28-day mortality, confirming data and precision from a previous study by Amato et al. (26), as shown in Figure S1. Additionally, because ΔP is highly associated with mortality and mathematically linked with Crs, we considered an alternative pathway: after adjusting for other clinical variables and Vt, we tested the effects of ΔP on severe AKI with Crs as a potential mediator. There was no significant direct effect of ΔP on severe AKI and almost all effects from ΔP were mediated by low Crs, as shown in Figure S2.
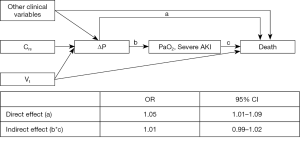
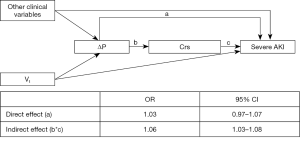
Additionally, we performed two additional sensitivity analyses to determine whether Crs and PEEP maintained their significant causal association with outcomes. In the first analysis, because high Vt can over distend lungs and interfere with Crs, we used only data with a Vt <8 mL/kg of predicted body weight. In the second analysis, RRT was considered as an alternative outcome. However, we could not detect a significant mediation of PEEP on the causal association between Crs and the need for RRT, and all other results were very similar, as shown in Tables S3 and S4.
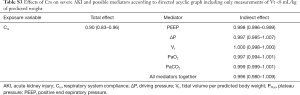
Full table
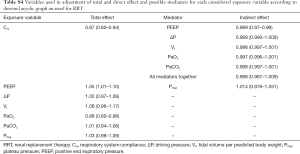
Full table
Traditional approach
Finally, we performed several models of logistic regression not following the DAG assumptions. Different models were built to evaluate if any other variable mathematically linked to Crs conferred predictive additional information, although adding when ΔP attenuated the Crs odds ratio, the only mechanical-related variables associated with severe AKI remained PEEP and Crs, as shown in Table S5.
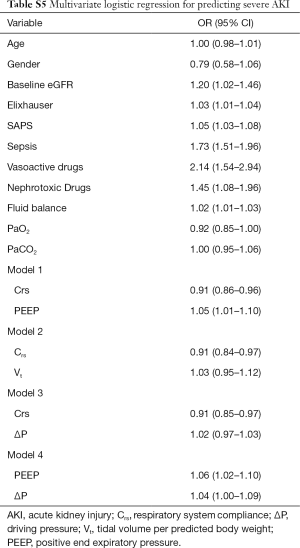
Full table
Discussion
In the present study, we evaluated respiratory-related variables in ARDS patients and their causal association with severe AKI. To perform this analysis, first we created a DAG with the assumption that all other respiratory variables suffer interference from lung disease severity, represented herein by Crs. Under these assumptions, we could identify that Crs and PEEP had a causal association with severe AKI. Moreover, a causal association between Crs and severe AKI was not mediated by any mechanical or blood gas variable, except by PEEP to a small degree.
Over-adjustment bias or unnecessary adjustment has recently drawn the attention of the research community, mainly in observational studies (27). Because there are many interrelationships between respiratory variables, including all variables in the same model cannot be appropriate. To overcome this problem, we chose a causal inference approach. To perform this, the first, and probably most important step, is to construct a DAG. As reported by recent guidelines (16), there is some difficulty in deciding what causal sequence of events do or do not exist. We tried to follow a temporal and comprehensive sequence. First, severe AKI is a later event, so it was considered a consequence of any possible causal association. Second, because we studied the first 48 h of MV, we assumed that pulmonary characteristics (Crs) would influence mechanical ventilation parameters and blood gas parameters. Finally, oxygenation index, although a recognized marker of ARDS severity, was not included as a confounder in any causal analysis. This is because the oxygenation index is (I) a consequence of lung disease/mechanical ventilator parameters and (II) PaO2 would mediate any possible causal association with severe AKI, as shown in Figure 1. Using this approach, it is clear that, for example, PEEP is a component of PPlat; thus, PPlat must be treated as a mediator, rather than a confounding variable, of the association between PEEP and severe AKI.
The first main finding of the present paper is that Crs had a causal association with severe AKI, and that this causal association is not mediated by any mechanical or blood gas parameters. This finding reinforces the cross-talking theory between organs, where a worsening in lung function can lead to worsening AKI. Our study suggests a causal association between CRS and severe AKI, but we cannot exclude a bidirectional pathway between renal and lung injuries. For example, it is possible that severe AKI implies in worsening Crs and, subsequently, in a vicious cycle. From a practical viewpoint, our finding suggest that interventions aimed to reduce AKI in ARDS patients must focus mainly in avoiding further lung injury and, except for PEEP as discussed below, other isolated mechanical ventilator parameters changes, including ΔP, will probably be inefficient in reducing AKI incidence in this population. In other words, an MV approach to ameliorate the renal outcome in ARDS patients will have a great chance to be effective if it ameliorates Crs first.
The other important finding was the causal association between PEEP and severe AKI. Although this is a highly plausible association (high intrathoracic pressure can lead to significant hemodynamic changes and PEEP is the pressure applied during the greater part of the respiratory cycle) (28), we could not demonstrate any significant mediation of hemodynamic parameters between high PEEP and severe AKI. In a meta-analysis, van den Akker (8) did not find any association between PEEP or Vt and AKI. However, as stated above in the cited study, the definition of AKI was not standardized, and information about renal status before MV was lacking in some of these studies. In another study, Lombardi et al. (14) found a univariate association between PEEP and AKI. However, after adjusting for more than 20 other variables, including peak pressure, which is in part determined by PEEP, no significant association remained. In this case, as stated above, peak pressure would be a possible mediator of PEEP effect and not a real confounder, and we interpreted it as an over-adjustment bias.
Our study has several limitations. First, it is difficult to establish causality in observational studies and in this paper we chose to explore causal associations. Second, our DAG has some assumptions with limited validity, but we think our primary objective (avoiding over-adjustment bias) was achieved, disclosing important causal associations that must be considered in future studies. Additionally, we performed several additional analyses that support our main finding. Third, our study is a retrospective one and data collection was carried out from 2001 to 2012, so medical practices were performed at the physician’s discretion, which implies in the lack of standardization in some controversial or changing issues—for example, target Vt, RRT indications and others. In addition, since we did not have data about inspiratory time, we could not evaluate mean airway pressure as another exposure. Finally, we selected patients under volume-controlled mode, limiting our findings to pressure and assisted modes.
In conclusion, using a model that confirms ΔP has a causal association with mortality in ARDS patients, our results show that Crs and PEEP are the only respiratory-related variables with a direct causal association on severe AKI. Because severe AKI is main related to lung injury severity, this study suggests that approaches reducing Vt and/or ΔP in ARDS can have limited effect on renal protection
Supplementary
MIMIC-III description and data collection
The database includes basic admission and demographic information (of which not all was used in the present study), as well as vital signs, laboratory and radiology results, medications, discharge diagnoses, nursing notes, physician discharge summaries and dates of death. The MIMIC-III database contains patients from five ICU types: medical ICU (MICU), surgical ICU (SICU), cardiac ICU (CICU), cardiac surgery recovery unit (CSRU), and neonatal ICU (NICU). Other clinical data were added to the database, including pharmacy, provider order entry records, admission and death records, discharge summaries, and 9th Revision (ICD-9) codes.
All data were extracted from the MIMIC-III database (v1.4) and included demographic information (e.g., age, gender, height), sepsis diagnosis as described by Angus et al. (19), Elixhauser comorbidity index (20), SCr at MV initiation and disease severity score assessed by the simplified acute physiology score (SAPS I). We chose SAPS I because it was recently used in both articles evaluating driving pressure (ΔP) as a risk factor for mortality (22,26). Additionally, arterial blood gas analyses in the first 48 h of MV were evaluated and the worst PaO2, PaCO2 and PaO2//FiO2 ratio (when PEEP was at least 5 cmH2O) were selected. We collected daily SCr up to day 7 after MV initiation, urine output, need for vasoactive drugs, exposure to nephrotoxic drugs (amphotericin, vancomycin, aminoglycoside), fluid balance before VM initiation and need of RRT.
Directed acyclic graph construction
In our directed acyclic graph (DAG) we had the following assumptions (I) because we studied only the first 48 h of MV, we assumed that all respiratory and blood gas variables could be influenced by lung disease severity, here represented by Crs (i.e., PaO2, PaCO2, PEEP, Vt, PaO2//FiO2 and ΔP could be, at least partly, be determined by Crs); (II) all other clinical variables (age, gender, Elixhauser comorbidity index, fluid balance before MV, baseline renal function, SAPS, nephrotoxic drugs, vasoactive drugs and sepsis) were considered confounders of all considered exposures in the causal association with severe AKI. This assumption is based on previous knowledge (for example, presence of sepsis can be associated with both respiratory and renal failure) or in the absence of data supporting the exclusion of any possible association, i.e., it is impossible to exclude the association of nephrotoxic drugs and a higher PEEP, even a third unmeasured confounder, like severe lung infection, for example. Additionally, (III) if possible, if relationships between two variables were bidirectional, they were modified according to evaluated exposures, making them real confounders. These interactions are represented in Figure 1 as connectors without arrow. Finally, (IV) all respiratory related variables, except for PaO2/FiO2 ratio, were considered potential causes of severe AKI. The variables included in the adjustment of each exposure are described in the Table S1.
Acknowledgments
Funding: AB Libório received a grant from Conselho Nacional de Desenvolvimento Científico e Tecnológico (number: 307875/2018-0).
Footnote
Conflicts of Interest: The authors have no conflicts of interest to declare.
Ethical Statement: The authors are accountable for all aspects of the work in ensuring that questions related to the accuracy or integrity of any part of the work are appropriately investigated and resolved. This study was approved by the institutional review boards of Massachusetts Institute of Technology and Beth Israel Deaconess Medical Center and was granted a waiver of informed consent.
References
- Hoste EAJ, Kellum JA, Selby NM, et al. Global epidemiology and outcomes of acute kidney injury. Nat Rev Nephrol 2018;14:607-25. [Crossref] [PubMed]
- Bellomo R, Kellum JA, Ronco C, et al. Acute kidney injury in sepsis. Intensive Care Med 2017;43:816-28. [Crossref] [PubMed]
- Vanmassenhove J, Kielstein J, Jörres A, et al. Management of patients at risk of acute kidney injury. Lancet 2017;389:2139-51. [Crossref] [PubMed]
- Darmon M, Clec’h C, Adrie C, et al. Acute respiratory distress syndrome and risk of AKI among critically ill patients. Clin J Am Soc Nephrol 2014;9:1347-53. [Crossref] [PubMed]
- Seeley EJ. Updates in the management of acute lung injury: a focus on the overlap between AKI and ARDS. Adv Chronic Kidney Dis 2013;20:14-20. [Crossref] [PubMed]
- Ranieri VM, Giunta F, Suter PM, et al. Mechanical ventilation as a mediator of multisystem organ failure in acute respiratory distress syndrome. JAMA 2000;284:43-4. [Crossref] [PubMed]
- Vieira JM, Castro I, Curvello-Neto A, et al. Effect of acute kidney injury on weaning from mechanical ventilation in critically ill patients. Crit Care Med 2007;35:184-91. [Crossref] [PubMed]
- van den Akker JP, Egal M, Groeneveld AB. Invasive mechanical ventilation as a risk factor for acute kidney injury in the critically ill: a systematic review and meta-analysis. Crit Care 2013;17:R98. [Crossref] [PubMed]
- Murdaugh HV Jr. Sieker Ho, Manfredi F. Effect of altered intrathoracic pressure on renal hemodynamics, electrolyte excretion and water clearance. J Clin Invest 1959;38:834-42. [Crossref] [PubMed]
- Darmon M, Schortgen F, Leon R, et al. Impact of mild hypoxemia on renal function and renal resistive index during mechanical ventilation. Intensive Care Med 2009;35:1031-8. [Crossref] [PubMed]
- Jacob LP, Chazalet JJA, Payen DM, et al. Renal hemodynamic and functional effect of PEEP ventilation in human renal transplantations. Am J Respir Crit Care Med 1995;152:103-7. [Crossref] [PubMed]
- Darmon M, Legrand M, Terzi N. Understanding the kidney during acute respiratory failure. Intensive Care Med 2017;43:1144-7. [Crossref] [PubMed]
- Han S, Mallampalli RK. The Acute Respiratory Distress Syndrome: From Mechanism to Translation. J Immunol 2015;194:855-60. [Crossref] [PubMed]
- Lombardi R, Nin N, Penũelas O, et al. Acute kidney injury in mechanically ventilated patients: The risk factor profile depends on the timing of AKI onset. Shock 2017;48:411-7. [Crossref] [PubMed]
- Suttorp MM, Siegerink B, Jager KJ, Zoccali C, Dekker FW. Graphical presentation of confounding in directed acyclic graphs. Nephrol Dial Transplant 2015;30:1418-23. [Crossref] [PubMed]
- Staplin N, Herrington WG, Judge PK, et al. Use of Causal Diagrams to Inform the Design and Interpretation of Observational Studies: An Example from the Study of Heart and Renal Protection (SHARP). Clin J Am Soc Nephrol 2017;12:546-52. [Crossref] [PubMed]
- Johnson AEW, Pollard TJ, Shen L, et al. MIMIC-III, a freely accessible critical care database. Sci Data 2016;3:160035. [Crossref] [PubMed]
- ARDS Definition Task Force, Ranieri VM, Rubenfeld GD, et al. Acute respiratory distress syndrome: the Berlin Definition. JAMA 2012;307:2526-33. [PubMed]
- Angus DC, Linde-Zwirble WT, Lidicker J, et al. Epidemiology of severe sepsis in the United States: Analysis of incidence, outcome, and associated costs of care. Crit Care Med 2001;29:1303-10. [Crossref] [PubMed]
- Elixhauser A, Steiner C, Harris DR, et al. Comorbidity Measures for Use with Administrative Data. Med Care 1998;36:8-27. [Crossref] [PubMed]
- Acute Respiratory Distress Syndrome Network., Brower RG, Matthay MA, et al. Ventilation with lower tidal volumes as compared with traditional tidal volumes for acute lung injury and the acute respiratory distress syndrome. N Engl J Med 2000;342:1301-8. [Crossref] [PubMed]
- Schmidt MFS, Amaral ACKB, Fan E, et al. Driving Pressure and Hospital Mortality in Patients Without ARDS: A Cohort Study. Chest 2018;153:46-54. [Crossref] [PubMed]
- Kidney Disease: Improving Global Outcomes (KDIGO) Acute Kidney Injury Work Group. KDIGO Clinical Practice Guideline for Acute Kidney Injury. Kidney Int Suppl 2012;2:1-138.
- Textor J, van der Zander B, Gilthorpe MS, et al. Robust causal inference using directed acyclic graphs: The R package “dagitty.” Int J Epidemiol 2016;45:1887-94. [PubMed]
- Steen J, Loeys T, Moerkerke B, et al. medflex: An R Package for Flexible Mediation Analysis using Natural Effect Models. J Stat Softw 2017. [Crossref]
- Amato MBP, Maureen D, Meadle O. Driving pressure and survival in the ARDS. New Engl J Med 2015;372:747-55. [Crossref] [PubMed]
- Schisterman EF, Cole SR, Platf RW. Overadjustment bias and unnecessary adjustment in epidemiologic studies. Epidemiology 2009;20:488-95. [Crossref] [PubMed]
- Koyner JL, Murray PT. Mechanical ventilation and lung-kidney interactions. Clin J Am Soc Nephrol 2008;3:562-70. [Crossref] [PubMed]