Psychological resilience negatively correlates with resting-state brain network flexibility in young healthy adults: a dynamic functional magnetic resonance imaging study
Introduction
Psychological resilience is an important personality character that is defined as one’ ability to positively cope with stress, adversity, and negative events in life (1-3). A decrease in psychological resilience has been found to be associated with many common psychiatric diseases, such as the schizophrenia (4), bipolar disorder (5), major depressive disorder (6), and posttraumatic stress disorder (7). Investigating the neural correlates of psychological resilience, therefore, may provide important clinical implications for understanding the pathological mechanisms underlying these diseases.
The application of functional neuroimaging techniques in the past decades, such as the functional magnetic resonance imaging (fMRI) and electroencephalography (EEG), has provided promising and non-invasive approaches to characterize the intrinsic functional organization of human brain in different spatial/temporal scales (8-10). In recent years, there have also been some efforts to link the psychological resilience to brain functions using fMRI or EEG in various populations such as the normal adults (11,12), fire-fighters (13) and posttraumatic stress disorder patients (7). For example, using fMRI, it has been reported that the brain activity during rest as measured by regional homogeneity (ReHo) in the dorsal anterior cingulate cortex negatively predicted psychological resilience in healthy young adults (11). However, the number of studies which have reported a potential association between altered brain functions and changes in psychological resilience is still very limited, and the neural substrates of psychological resilience remain largely unclear compared with some other personal traits (12).
One of the reasons why the findings in previous studies regarding the relationship between brain functions and psychological resilience were limited, as suggested by some recent work (12,14), may be that they only focus on the “static” features of brain. Generally, most conventional functional neuroimaging studies were performed based on the assumption that the features of brain functions never change over time. It has been newly proved that, however, the functional organization of brain fluctuates even during rest, and much important information about the brain may be lost when using conventional static analysis methods (15,16). Therefore, to characterize the “dynamic” features of human brain has become an emerging topic in recent functional neuroimaging studies (17,18). One of these novel features, for example, is the brain network flexibility which is defined by all brain regions’ average rate of switching between different modules in the framework of dynamic network model (19,20). Such flexibility of dynamic brain network has been suggested to be an important fundamental feature of brain function (21), and has been reported to be associated with learning (20), cognition (22,23), emotion (24), as well as multiple mental diseases such as the major depressive disorder (25) and schizophrenia (26). Importantly, in a most recent study, a lower flexibility of EEG source-space brain network was found to be related with a higher psychological resilience in healthy adults, while no significant relationships were found between the psychological resilience and conventional static brain network metrics (12). These findings highlighted the importance of the dynamic reconfiguration of functional brain network, as measured by network flexibility, in the neural substrates of psychological resilience (12).
There are some limitations, however, to the above findings in recent EEG-based dynamic brain network study. Firstly, they only focused on the brain network flexibility at a temporal scale of milliseconds, and whether a negative relationship between brain network flexibility and psychological resilience exists in other temporal scales (e.g., a scale of seconds) remains unknown to our knowledge. Secondly, the EEG-based approaches were limited by relatively low spatial resolution and inability to satisfactorily capture the regional alterations of brain activity, especially for the deep brain areas such as subcortical structures (27). These limitations could be partly overcome by verifying the results in fMRI-based brain networks, since the fMRI has a larger temporal scale (typically a scale of seconds), a much higher spatial resolution and a greater ability to measure deep brain structures compared with EEG (27). However, whether the fMRI-based brain network flexibility is also related to psychological resilience remains unknown to our knowledge.
For the above concerns, the present study aimed to investigate the possible relationship between psychological resilience and brain network flexibility, which is a novel and important dynamic feature of the brain functional organization, using the resting-state fMRI approaches for the first time. To reach this goal, a group of healthy adults were recruited and their psychological resilience was quantified by a widely-used and validated scale (28); resting-state fMRI data were scanned from each subject and the brain network flexibility was calculated following previous publications (21-25,29); the psychological resilience score was then correlated with brain network flexibility at both global and regional levels. In addition, the association between psychological resilience and the modularity of conventional static brain network was also tested for comparison. It was anticipated that the results would provide important complementary information to previous findings in EEG-based studies, and further improve our understanding of the neural mechanisms underlying psychological resilience.
Methods
Participants
Using the Structured Clinical Interview for DSM-IV, Non-patient Version (SCID-I/NP) (30), a total of 48 healthy adult participants were recruited from the Second Xiangya Hospital in Changsha, China based on the following inclusion criteria: (I) 18–35 years of age and had been educated for at least 9 years; (II) right-handed, Han Chinese; (III) had no history or family history of any psychiatric disorder; (IV) had no history of other severe disorders or any contraindication to fMRI scanning. All participants completed the Information (WAIS-I) and Digit Symbol (WAIS-DS) subtests of the Wechsler Adult Intelligence Scale (WAIS) (31) to assess their cognitive functions (4,32). The study was approved by the Ethics Committee of Second Xiangya Hospital, and written informed consent was obtained from all subjects.
Since fMRI data of seven participants were excluded from the analysis because of poor quality (see later), the final analyzed sample included 41 subjects [mean age: 22.39±2.66 (SD); gender: 22 males/19 females; mean years of education: 14.63±1.83 (SD); mean WAIS-I score: 22.01±4.73 (SD); mean WAIS-DS score: 90.10 ± 13.87 (SD)].
Measures of psychological resilience
To measure psychological resilience, all participants completed the Chinese version of Connor-Davidson Resilience Scale (CD-RISC) (28), which is a validated and widely-used scale for psychological resilience (4,33,34). Briefly, it is a 25-item self-rating scale with the scores of each single item ranging from 0 to 4, and the score of the full scale ranging from 0 to 100. A higher CD-RISC score then indicates a higher psychological resilience (28). The mean CD-RISC score of all participants in the final analyzed sample (including 41 subjects) was 70.56±12.29 (SD).
Imaging data acquisition and preprocessing
Resting-state fMRI and T1-weighted structural images were acquired from each subject using a 3.0 T Philips MRI scanner. The fMRI images were obtained by gradient echo-planar imaging sequence (repetition time/echo time = 2,000/30 ms; slice number =36; thickness/gap =4.0/0 mm; field of view =240×240 mm2; acquisition matrix =64×64; flip angle =90°; number of time points =250), and the T1-weighted images were obtained by three-dimensional fast spoiled gradient recalled sequence (repetition time/echo time =7.5/3.7 ms; slice number =180; thickness/gap =1.0/0 mm; field of view =240×240 mm2; acquisition matrix 256×200; flip angle =8°).
The imaging data preprocessing was performed using the standard pipeline provided by the DPARSF software (35,36). Briefly, it included removing the first 10 volumes, slice-timing, head motion realignment, brain tissue segmentation, spatial normalization, temporal filtering (0.01–0.10 Hz), and regressing out signals from the white matter and cerebrospinal fluid as well as the Friston-24 head motion parameters (37). Moreover, two and five subjects were excluded from the analysis for excessive head motion [as defined by mean framewise-displacement (FD) (38) >0.2 mm] and poor image quality (as determined by a manual checking), respectively.
Flexibility of dynamic brain network
After preprocessing, the brain network flexibility of each subject was calculated following some previous publications (21-25,29), which was summarized as follows (and also see Figure 1):
- Dynamic network construction: a total of 90 brain regions of interest (ROIs) were firstly defined by the Automated Anatomical Labeling (AAL) atlas (39). The mean time series of each ROI was extracted and then divided into T=12 non-overlapping time windows of 20 time points (40 s) each (Figure 1A). Such a window length was chosen because it was suggested to be able to produce relatively robust results, and has been widely used in the fMRI studies investigating brain network flexibility (18,24,25). For each of the 12 windows, a 90×90 connectivity matrix was calculated by the Pearson correlation coefficients, to evaluate the functional connectivity between each pair of ROIs. As the result, these time-ordered matrices formed a dynamic brain network G = (Gt)t = 1, 2, 3, …, 12, where Gt is the tth connectivity matrix representing the “snapshot” of brain functional organization within the tth time window (Figure 1B).
- Dynamic community structure detection: to calculate the flexibility of obtained dynamic networks, a dynamic community detection method as described by Mucha et al. (40) was implemented with an open-source Matlab code package (https://github.com/GenLouvain/GenLouvain) (41). Briefly, the dynamic community structures were identified by maximizing a Louvain-like modularity function (Q) as
,
where the two free parameters γ and ω were both set at a default value of 1 (24,29); Aijs is the correlation coefficient between ROIs i and j in the sth connectivity matrix; kis and ms are the node degree of ROI i and the sum degree of all ROIs in the sth connectivity matrix, respectively; δ(Mis, Mjs) and δ(Mis, Mjr) equal to 1 when the corresponding ROIs belong to the same module, and equal to 0 when not (21,40). Before community detection, all negative correlation values in the connection matrices were set to zeros as in previous studies, since the interpretation of negative correlations is still debating (21,22). As the final output of community detection, a time-dependent community assignment matrix was acquired for each subject (Figure 1C). - Network flexibility calculation: the brain network flexibility was then calculated at both global and regional levels, based on the above acquired dynamic community assignment matrices. Briefly, the flexibility of a ROI i (fi) was defined by its frequency of changing the community as fi = ni/N, where ni is the number of times it changed its community assignment, and N is the maximum possible number of changes (equaled to T − 1 = 11 here) (22,25). Such calculations were performed using the Network Community Toolbox (http://commdetect.weebly.com). Since individual runs of the community detection algorithms could produce slightly different results, the procedures of community detection and flexibility calculation were run for 100 times, and the final values of flexibility were obtained by averaging these 100 runs (12,22,25). The obtained flexibility for each ROI ranged from 0 to 1, with a higher value indicating a higher frequency of changing its community affiliation (Figure 1D). After that, flexibility of the whole brain network was obtained by averaging all the 90 ROIs.
- Flexibility of individual subnetworks: based on previous research (42,43), all ROIs from the AAL atlas can be assigned into 9 predefined subnetworks including the visual, auditory, default-mode, salience, sensorimotor, frontoparietal, cingulo-opercular, attention and subcortical subnetworks (see Table S1 for assignments). Therefore, we further calculated the flexibility at subnetwork level, by averaging all the ROIs belonging to each subnetwork.
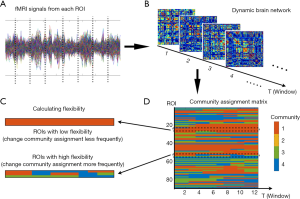
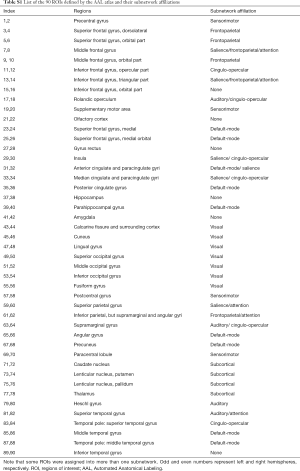
Full table
Modularity of static brain network
For comparative purposes, we also calculated a validated and widely used metric related to conventional static network modular structures, the modularity (44-46) for each subject. In brief, static weighted brain networks were constructed using signals of the whole fMRI scan following common procedures (47,48); similar with the dynamic networks, connections between each pair of ROIs were estimated by Pearson correlations and all negative correlations were set to zeros. The calculations were performed using the Brain Connectivity Toolbox (https://sites.google.com/site/bctnet). More detailed information about the definition of modularity in static brain networks can be found a previous publication (49).
Correlations
The association between psychological resilience (as measured by the CD-RISC score) and brain network flexibility was assessed using the partial Pearson correlation adjusted for age, sex, years of education and head motion (as measured by mean FD). This was performed at all the global, subnetwork and ROI levels. At global level, the CD-RISC score was correlated with flexibility of the whole brain network. At subnetwork and ROI levels, the CD-RISC score was correlated with flexibility of each of the 9 subnetworks as well as each of the 90 ROIs, with false discovery rate (FDR) corrections (50) applied for multiple tests across the multiple subnetworks/ROIs. Significance was set at corrected P<0.5. Here, the ROIs with a trends of correlation [as defined by a higher threshold of corrected P<0.1 (51)] were also reported. For comparative purposes, the modularity of static brain network was correlated with CD-RISC score using the same above partial correlation, too. The results were visualized using the BrainNet Viewer (52).
Follow-up analyses
We further performed several follow-up analyses to see if our results were affected by some other factors. Firstly, the correlation analyses between CD-RISC score and brain network flexibility were repeated using a different parcellation scheme based on the Power atlas with 264 ROIs (43,53), also at all the global, subnetwork (see Table S2 for ROI assignments) and ROI levels. Secondly, the analyses were repeated with the global signal regression (GSR) performed, which is a still controversial (54,55) option in fMRI data preprocessing and was not performed in our primary analyses, to see its possible effects. Lastly, since cognitive function has been suggested to be an important factor associated with both psychological resilience (4,56) and brain network flexibility (23), we correlated the WAIS-I/WAIS-DS scores and CD-RISC score/brain network flexibility and when a significant correlations was observed, we further performed a partial correlation between CD-RISC score and brain network flexibility with the WAIS-I/WAIS-DS score as an additional covariate.

Full table
Results
Correlations
As shown in Figure 2, a significant negative correlation was found between the CD-RISC score and brain network flexibility at the global level (r=−0.533, P=0.001). At the level of subnetworks, the CD-RISC score was significantly negatively correlated with flexibility of the visual subnetwork (r=−0.576, FDR-corrected P=0.002, Figure 3), but not with other subnetworks (all FDR-corrected P>0.05, Figure 3). At the ROI level, the CD-RISC score was significantly negatively correlated with flexibilities of the right superior frontal gyrus (medial orbital) (r=−0.508, FDR-corrected P=0.030), right lingual gyrus (r=−0.509, FDR-corrected P=0.030), left middle occipital gyrus (r=−0.617, FDR-corrected P=0.004), left inferior occipital gyrus (r=−0.525, FDR-corrected P=0.030) and right inferior occipital gyrus (r=−0.499, FDR-corrected P=0.030), but not with other ROIs (all FDR-corrected P>0.05). Trends of negative correlations (FDR-corrected P<0.1) were also found between the CD-RISC score and a number of ROIs belonging to the default-mode and visual subnetworks (Figure 4 and Table S3). There was no significant correlation between the CD-RISC score and modularity of static brain network (r=0.320, P=0.054, Figure 2).
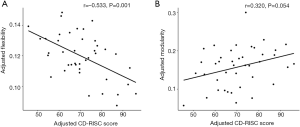
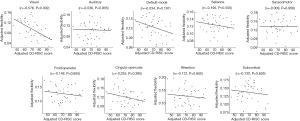
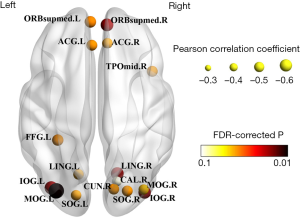
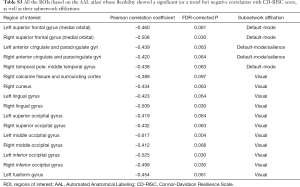
Full table
Follow-up analyses
When repeating the analyses with a different parcellation scheme based on the Power atlas, or with the GSR performed in data preprocessing, negative correlations between the CD-RISC score and global brain network flexibility remained significant (both P<0.05, Figure 5). At the subnetwork level, similarly, negative correlations remained significant between the CD-RISC score and flexibility of the visual subnetwork (both FDR-corrected P<0.05, Figure 5), while no significant correlations were found for any other subnetwork (FDR-corrected P>0.05). At the level of individual ROIs, however, no results remained significant after corrections for multiple tests (all FDR-corrected P>0.05).
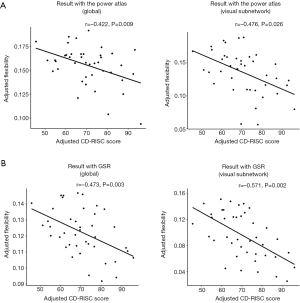
The WAIS-I score was significantly positively correlated with CD-RISC score (r=0.440, P=0.004), but not with brain network flexibility (r=−0.007, P=0.966); no significant correlations were found for the WAIS-DS score (all P>0.05). The partial correlation between CD-RISC score and brain network flexibility remained significant when including the WAIS-I score as an additional covariate (r=−0.526, P=0.001).
Discussion
To our knowledge, this study explored the possible relationship between psychological resilience and brain network dynamics in healthy adults using the resting-state fMRI approaches for the first time. In specific, we examined an important feature of the brain network called flexibility, which quantifies the dynamic variations of its modular community structures over time. Generally, our results revealed that psychological resilience is strongly negatively associated with the brain network flexibility at all the global, subnetwork and regional levels. Meanwhile, the modularity of static brain network did not reveal significant correlation with psychological resilience. These results may allow us to better understand the neural substrates of psychological resilience.
In the present study, we found that the participants’ psychological resilience scores were significantly negatively correlated with the flexibility of their brain networks (Figure 2). A higher flexibility refers to a higher frequency of switching between different functional modules for all brain regions (21,25). Therefore, our result indicates that a temporally less stable functional brain organization may be related to lower psychological resilience. Interestingly, similar results were obtained in a recent study using EEG, which reported a negative correlation between psychological resilience and the flexibility of EEG source-space brain network across multiple frequency bands (12). Considering such previous findings together with our results, it can be concluded that the negative relationship between brain network flexibility and psychological resilience may exist across a wide range of temporal scales from milliseconds to seconds. Our results thus not only reinforce the newly hypothesis that the brain network dynamics play crucial roles in psychological resilience (12), but also can be meaningful for better understanding the multi-scale topological nature of human brain (57).
A dynamic neural community structure has been suggested to be necessary for the brain system to adapt to changing environmental demands (26,58). However, the biological significance of an excessive fluctuation of functional brain structures during rest remains not completely clear (59). One hypothesis is that an excessively increased flexibility may indicate a under-constrained brain network with overloaded information (21,26). Such a under-constrained and “overloaded” brain network may be more likely to fail to adapt to changing demands following external stress factors (12). These may partly interpret why brains of the subjects with a decreased psychological resilience are more flexible during rest than resilient ones, since psychological resilience refers to a capacity to adapt to external stresses and changes (12,60). It is noteworthy that in the present study, we found that the dynamic but not static brain network metrics related to modularity structures could reveal this association between psychological resilience and brain functions. Our results thus highlighted the critical importance of studying the brain functional dynamics in research on psychological resilience.
At the subnetwork level, we found that psychological resilience was significantly negatively correlated with flexibility of the visual subnetwork (Figure 3). Moreover, significant (or trends for) negative correlations were found between psychological resilience and flexibilities of a number of visual and default-mode areas at the ROI level (Figure 4 and Table S3). Based on the hypothesis that increased brain network flexibility may indicate increased information load (21), an increased flexibility of the visual system may be related to a lower ability to sufficiently filter unwanted sensory information (61). It may be associated with the deficits in processing sensory information which have been reported in low-resilient subjects (62). Another point we consider is that since the visual system has been proved to be one of the most “inflexible” part of the brain compared than other sensory systems (24), its flexibility may be most sensitive to changes in the information load. The default-mode areas, on the other hand, are known to mediate one’s self-referential and internally-directed processing (63,64). Furthermore, increased temporal fluctuations of the default-mode subnetwork have been suggested to be associated with increased frequencies of spontaneous, internally-oriented thoughts such as mind-wandering in healthy adults (65,66) and negative ruminations in depressive subjects (59,67). An increased flexibility of the default-mode areas may then be reflective of an exaggerated focus on one’s negative internalized experience in the low-resilient subjects (14). Our results, therefore, may suggest the importance of the visual and default-mode areas in the relationship between psychological resilience and brain network dynamics. In fact, our findings and hypothesis are supported by the previous study applying EEG, which also reported that flexibilities of several visual regions were negatively correlated with psychological resilience in healthy adults (12); and another recent study conducted in children and adolescents, which found that participants with higher psychological resilience are characterized by shorter duration with the default-mode subnetwork activated over time (14).
It is noteworthy that in our analyses, all correlations were performed controlling for age, sex, education and head motion. Moreover, the correlation between psychological resilience score and brain network flexibility remained significant when additionally controlling for cognitive performance. The main results were robust to changes in the parcellation scheme or data preprocessing options, too (Figure 5). Therefore, our findings are more likely to be associated with the neural mechanisms underlying psychological resilience, but not driven by other factors such as age, sex, cognition, head motion or data preprocessing scheme.
There are several limitations and future research directions to be noted. Firstly, although we found a negative association between psychological resilience and brain network flexibility, the causality between them remains unclear based on the current findings. Secondly, the current sample size is relatively small. Future studies should verify our results in a bigger sample in order to increase the reliability and statistical power (68), and to test if differential patterns would exist across different subpopulations [e.g., male and female participants (69)]. Thirdly, we only investigated the relationship between psychological resilience and brain network dynamics during rest, and future studies may explore such relationship under specific tasks to further improve our knowledge on it. Fourthly, while we only applied the fMRI approaches, an integrated EEG-fMRI study or a functional-structural coupling neuroimaging study (70) may provide more insights into the neurol basis of psychological resilience. Lastly and importantly, confirming our findings in psychiatric populations who are known to have decreased psychological resilience (4-7), rather than only in healthy populations, may provide much more important clinical implications for these findings.
Conclusions
In summary, in the present study, we explored the neural correlates of psychological resilience in healthy adults by investigating its relationship with brain network flexibility, a fundamental dynamic feature of brain network defined by switching frequency of its modular community structures. Our results revealed that psychological resilience is significantly negatively correlated with brain network flexibility, but not with modularity of conventional static network, at both global and regional levels. Specially, those regions showing significant correlations were mainly located in the visual and default-mode areas. The results suggest that excessive fluctuations of the brain functional organization during rest may be indicative of a lower psychological resilience, and the visual and default-mode systems may play crucial roles in such relationship. These findings may provide important implications for improving our understanding of the neural mechanisms underlying individual differences in psychological resilience and related psychiatric disorders.
Acknowledgments
Funding: This study was supported by the China Precision Medicine Initiative (grant number 2016YFC0906300) and the National Natural Science Foundation of China (grant numbers 81561168021 and 81671335).
Footnote
Conflicts of Interest: The authors have no conflicts of interest to declare.
Ethical Statement: The authors are accountable for all aspects of the work in ensuring that questions related to the accuracy or integrity of any part of the work are appropriately investigated and resolved. The study was approved by the Ethics Committee of Second Xiangya Hospital, and written informed consent was obtained from all subjects.
References
- Martin A, Njoroge W. Resilience and vulnerability: Adaptation in the context of childhood adversities. Am J Psychiatry 2005;162:1553-a-4. [Crossref]
- Fletcher D, Sarkar M. Psychological resilience: A review and critique of definitions, concepts, and theory. Eur Psychol 2013;18:12-23. [Crossref]
- Southwick SM, Charney DS. The science of resilience: implications for the prevention and treatment of depression. Science 2012;338:79-82. [Crossref] [PubMed]
- Deng M, Pan Y, Zhou L, et al. Resilience and cognitive function in patients with schizophrenia and bipolar disorder, and healthy controls. Front Psychiatry 2018;9:279. [Crossref] [PubMed]
- Choi JW, Cha B, Jang J, et al. Resilience and impulsivity in euthymic patients with bipolar disorder. J Affect Disord 2015;170:172-7. [Crossref] [PubMed]
- Kesebir S, Gündoğar D, Küçüksubaşı Y, et al. The relation between affective temperament and resilience in depression: a controlled study. J Affect Disord 2013;148:352-6. [Crossref] [PubMed]
- New AS, Fan J, Murrough JW, et al. A functional magnetic resonance imaging study of deliberate emotion regulation in resilience and posttraumatic stress disorder. Biol Psychiatry 2009;66:656-64. [Crossref] [PubMed]
- Power JD, Schlaggar BL, Petersen SE. Studying brain organization via spontaneous fMRI signal. Neuron 2014;84:681-96. [Crossref] [PubMed]
- Wang H, Li L, Wu T, et al. Increased cerebellar activation after repetitive transcranial magnetic stimulation over the primary motor cortex in patients with multiple system atrophy. Ann Transl Med 2016;4:103. [Crossref] [PubMed]
- Hassan M, Chaton L, Benquet P, et al. Functional connectivity disruptions correlate with cognitive phenotypes in Parkinson's disease. Neuroimage Clin 2017;14:591-601. [Crossref] [PubMed]
- Kong F, Wang X, Hu S, et al. Neural correlates of psychological resilience and their relation to life satisfaction in a sample of healthy young adults. Neuroimage 2015;123:165-72. [Crossref] [PubMed]
- Paban V, Modolo J, Mheich A, et al. Psychological resilience correlates with EEG source-space brain network flexibility. Netw Neurosci 2019;3:539-50. [Crossref] [PubMed]
- Reynaud E, Guedj E, Souville M, et al. Relationship between emotional experience and resilience: An fMRI study in fire-fighters. Neuropsychologia 2013;51:845-9. [Crossref] [PubMed]
- Iadipaolo AS, Marusak HA, Paulisin SM, et al. Distinct neural correlates of trait resilience within core neurocognitive networks in at-risk children and adolescents. Neuroimage Clin 2018;20:24-34. [Crossref] [PubMed]
- Chang C, Glover GH. Time–frequency dynamics of resting-state brain connectivity measured with fMRI. Neuroimage 2010;50:81-98. [Crossref] [PubMed]
- Hutchison RM, Womelsdorf T, Gati JS, et al. Resting-state networks show dynamic functional connectivity in awake humans and anesthetized macaques. Hum Brain Mapp 2013;34:2154-77. [Crossref] [PubMed]
- Preti MG, Bolton TA, Van De Ville D. The dynamic functional connectome: state-of-the-art and perspectives. Neuroimage 2017;160:41-54. [Crossref] [PubMed]
- Hutchison RM, Womelsdorf T, Allen EA, et al. Dynamic functional connectivity: promise, issues, and interpretations. Neuroimage 2013;80:360-78. [Crossref] [PubMed]
- Sizemore AE, Bassett DS. Dynamic graph metrics: Tutorial, toolbox, and tale. NeuroImage 2018;180:417-27. [Crossref] [PubMed]
- Bassett DS, Wymbs NF, Porter MA, et al. Dynamic reconfiguration of human brain networks during learning. Proc Natl Acad Sci U S A 2011;108:7641-6. [Crossref] [PubMed]
- Pedersen M, Zalesky A, Omidvarnia A, et al. Multilayer network switching rate predicts brain performance. Proc Natl Acad Sci U S A 2018;115:13376-81. [Crossref] [PubMed]
- Braun U, Schäfer A, Walter H, et al. Dynamic reconfiguration of frontal brain networks during executive cognition in humans. Proc Natl Acad Sci U S A 2015;112:11678-83. [Crossref] [PubMed]
- He L, Zhuang K, Li Y, et al. Brain flexibility associated with need for cognition contributes to creative achievement. Psychophysiology 2019;56:e13464. [Crossref] [PubMed]
- Betzel RF, Satterthwaite TD, Gold JI, et al. Positive affect, surprise, and fatigue are correlates of network flexibility. Sci Rep 2017;7:520. [Crossref] [PubMed]
- Zheng H, Li F, Bo Q, et al. The dynamic characteristics of the anterior cingulate cortex in resting-state fMRI of patients with depression. J Affect Disord 2018;227:391-7. [Crossref] [PubMed]
- Braun U, Schäfer A, Bassett DS, et al. Dynamic brain network reconfiguration as a potential schizophrenia genetic risk mechanism modulated by NMDA receptor function. Proc Natl Acad Sci U S A 2016;113:12568-73. [Crossref] [PubMed]
- Ritter P, Villringer A. simultaneous EEG–fMRI. Neurosci Biobehav Rev 2006;30:823-38. [Crossref] [PubMed]
- Connor KM, Davidson JR. Development of a new resilience scale: The Connor-Davidson resilience scale (CD-RISC). Depress Anxiety 2003;18:76-82. [Crossref] [PubMed]
- Harlalka V, Bapi RS, Vinod P, et al. Atypical flexibility in dynamic functional connectivity quantifies the severity in autism spectrum disorder. Front Hum Neurosci 2019;13:6. [Crossref] [PubMed]
- First MB, Spitzer R, Gibbon M, et al. Structured clinical interview for DSM-IV clinical version (SCID-I/CV). Washington, DC: American Psychiatric Press; 1997.
- Gong YX. Revision of Wechsler's Adult Intelligence Scale in China. Acta Psychologica Sinica 1983;15:121-9.
- Long Y, Ouyang X, Liu Z, et al. Associations among suicidal ideation, white matter integrity and cognitive deficit in first-episode schizophrenia. Front Psychiatry 2018;9:391. [Crossref] [PubMed]
- Campbell-Sills L, Stein MB. Psychometric analysis and refinement of the connor–davidson resilience scale (CD-RISC): Validation of a 10-item measure of resilience. J Trauma Stress 2007;20:1019-28. [Crossref] [PubMed]
- Yu X, Zhang J. Factor analysis and psychometric evaluation of the Connor-Davidson Resilience Scale (CD-RISC) with Chinese people. Soc Behav Pers 2007;35:19-30. [Crossref]
- Yan C, Zang Y. DPARSF: a MATLAB toolbox for "pipeline" data analysis of resting-state fMRI. Front Syst Neurosci 2010;4:13. [PubMed]
- Yan CG, Wang XD, Zuo XN, et al. DPABI: data processing & analysis for (resting-state) brain imaging. Neuroinformatics 2016;14:339-51. [Crossref] [PubMed]
- Friston KJ, Williams S, Howard R, et al. Movement-related effects in fMRI time-series. Magn Reson Med 1996;35:346-55. [Crossref] [PubMed]
- Jenkinson M, Bannister P, Brady M, et al. Improved optimization for the robust and accurate linear registration and motion correction of brain images. Neuroimage 2002;17:825-41. [Crossref] [PubMed]
- Tzourio-Mazoyer N, Landeau B, Papathanassiou D, et al. Automated anatomical labeling of activations in SPM using a macroscopic anatomical parcellation of the MNI MRI single-subject brain. Neuroimage 2002;15:273-89. [Crossref] [PubMed]
- Mucha PJ, Richardson T, Macon K, et al. Community structure in time-dependent, multiscale, and multiplex networks. Science 2010;328:876-8. [Crossref] [PubMed]
- Jeub LG, Bazzi M, Jutla IS, et al. A generalized Louvain method for community detection implemented in MATLAB. Available online: (2011-2019).https://github.com/GenLouvain/GenLouvain
- Cao H, Chung Y, McEwen SC, et al. Progressive reconfiguration of resting-state brain networks as psychosis develops: Preliminary results from the North American Prodrome Longitudinal Study (NAPLS) consortium. Schizophr Res 2019. [Epub ahead of print]. [Crossref] [PubMed]
- Power JD, Cohen AL, Nelson SM, et al. Functional network organization of the human brain. Neuron 2011;72:665-78. [Crossref] [PubMed]
- Braun U, Plichta MM, Esslinger C, et al. Test–retest reliability of resting-state connectivity network characteristics using fMRI and graph theoretical measures. Neuroimage 2012;59:1404-12. [Crossref] [PubMed]
- Cao H, Plichta MM, Schäfer A, et al. Test–retest reliability of fMRI-based graph theoretical properties during working memory, emotion processing, and resting state. Neuroimage 2014;84:888-900. [Crossref] [PubMed]
- Tao J, Jiang X, Wang X, et al. Disrupted control-related functional brain networks in drug-naive children with attention-deficit/hyperactivity disorder. Front Psychiatry 2017;8:246. [Crossref] [PubMed]
- Cao H, Bertolino A, Walter H, et al. Altered functional subnetwork during emotional face processing: a potential intermediate phenotype for schizophrenia. JAMA Psychiatry 2016;73:598-605. [Crossref] [PubMed]
- Wen X, Zhang H, Li G, et al. First-year development of modules and hubs in infant brain functional networks. Neuroimage 2019;185:222-35. [Crossref] [PubMed]
- Rubinov M, Sporns O. Complex network measures of brain connectivity: uses and interpretations. Neuroimage 2010;52:1059-69. [Crossref] [PubMed]
- Benjamini Y, Hochberg Y. Controlling the false discovery rate: a practical and powerful approach to multiple testing. J R Stat Soc Series B Stat Methodol 1995;57:289-300.
- Liu C, Tardif T, Wu H, et al. The representation of category typicality in the frontal cortex and its cross-linguistic variations. Brain Lang 2013;127:415-27. [Crossref] [PubMed]
- Xia M, Wang J, He Y. BrainNet Viewer: a network visualization tool for human brain connectomics. PLoS One 2013;8:e68910. [Crossref] [PubMed]
- Mohr H, Wolfensteller U, Betzel RF, et al. Integration and segregation of large-scale brain networks during short-term task automatization. Nat Commun 2016;7:13217. [Crossref] [PubMed]
- Murphy K, Fox MD. Towards a consensus regarding global signal regression for resting state functional connectivity MRI. Neuroimage 2017;154:169-73. [Crossref] [PubMed]
- Lydon-Staley DM, Ciric R, Satterthwaite TD, et al. Evaluation of confound regression strategies for the mitigation of micromovement artifact in studies of dynamic resting-state functional connectivity and multilayer network modularity. Netw Neurosci 2019;3:427-54. [Crossref] [PubMed]
- Simeon D, Yehuda R, Cunill R, et al. Factors associated with resilience in healthy adults. Psychoneuroendocrinology 2007;32:1149-52. [Crossref] [PubMed]
- Betzel RF, Bassett DS. Multi-scale brain networks. Neuroimage 2017;160:73-83. [Crossref] [PubMed]
- Kashtan N, Alon U. Spontaneous evolution of modularity and network motifs. Proc Natl Acad Sci U S A 2005;102:13773-8. [Crossref] [PubMed]
- Wise T, Marwood L, Perkins A, et al. Instability of default mode network connectivity in major depression: a two-sample confirmation study. Transl Psychiatry 2017;7:e1105. [Crossref] [PubMed]
- Bhamra R, Dani S, Burnard K. Resilience: the concept, a literature review and future directions. Int J Prod Res 2011;49:5375-93. [Crossref]
- Hong LE, Summerfelt A, Mitchell BD, et al. Sensory gating endophenotype based on its neural oscillatory pattern and heritability estimate. Arch Gen Psychiatry 2008;65:1008-16. [Crossref] [PubMed]
- Haase L, Stewart JL, Youssef B, et al. When the brain does not adequately feel the body: links between low resilience and interoception. Biol Psychol 2016;113:37-45. [Crossref] [PubMed]
- Whitfield-Gabrieli S, Ford JM. Default mode network activity and connectivity in psychopathology. Annu Rev Clin Psychol 2012;8:49-76. [Crossref] [PubMed]
- Chen YC, Zhang H, Kong Y, et al. Alterations of the default mode network and cognitive impairment in patients with unilateral chronic tinnitus. Quant Imaging Med Surg 2018;8:1020. [Crossref] [PubMed]
- Kucyi A, Davis KD. Dynamic functional connectivity of the default mode network tracks daydreaming. Neuroimage 2014;100:471-80. [Crossref] [PubMed]
- Zabelina DL, Andrews-Hanna JR. Dynamic network interactions supporting internally-oriented cognition. Curr Opin Neurobiol 2016;40:86-93. [Crossref] [PubMed]
- Kaiser RH, Whitfield-Gabrieli S, Dillon DG, et al. Dynamic resting-state functional connectivity in major depression. Neuropsychopharmacology 2016;41:1822. [Crossref] [PubMed]
- Cao H, McEwen SC, Forsyth JK, et al. Toward leveraging human connectomic data in large consortia: generalizability of fmri-based brain graphs across sites, sessions, and paradigms. Cereb Cortex 2019;29:1263-79. [Crossref] [PubMed]
- Xin J, Zhang XY, Tang Y, et al. Brain Differences Between Men and Women: Evidence From Deep Learning. Front Neurosci 2019;13:185. [Crossref] [PubMed]
- Yang J, Pu W, Ouyang X, et al. Abnormal Connectivity Within Anterior Cortical Midline Structures in Bipolar Disorder: Evidence From Integrated MRI and Functional MRI. Front Psychiatry 2019;10:788. [Crossref] [PubMed]