A predictive analysis approach for paediatric and adult high-grade glioma: miRNAs and network insight
Introduction
Glioma is a solid tumour with a high incidence rate. Furthermore, high-grade glioma (HGG) is considered to be one of the most malignant gliomas due to its strong degree of differentiation, invasion and migration. HGG exhibits much heterogeneity (1) between adults and children, as reflected in many aspects. For example, tumours in children involve the entire brain, including the cerebellum, brainstem, and diencephalon, whereas more than 75% of adults are mostly affected in the supratentorial compartment (2). Regarding treatment, surgery with radiotherapy and chemotherapy is the standard treatment for HGG patients. Diagnosis methods mainly depend on imaging techniques, including computed tomography (CT), perfusion magnetic resonance imaging, radioisotope scanning (γ-ray brain imaging), ultrasound cerebrospinal fluid (CSF) examination and postoperative pathological analysis of craniotomy. Despite the heterogeneity in HGG, finding clear differences between adults and children for treatment and diagnostics is difficult (3). Moreover, the special location of the tumour makes surgery and diagnosis inconvenient. Overall, differentially expressed factors in children and adults have profound significance in clinical applications for HGG. In this study, we focused on common molecules expressed in HGG between children and adults.
MicroRNAs comprise a family of small non-coding RNA molecules of 18–25 nucleotides that participate in post-transcriptional gene regulation. Much data show that miRNA signatures can refine glioblastoma classification, differentiate GBM subclasses, as well as provide regulatory links regarding disrupted signalling pathways (4). It has been shown that miRNAs are integrally involved in the development and progression of HGG. Indeed, they are essential regulators of many key pathways, such as Wnt signalling, and they have been implicated in tumour pathogenesis. Because miRNAs have been shown to play crucial roles in HGG progression, invasion, tumour growth, and therapy responses, it is very likely that some miRNAs may be useful biomarkers for patients with brain tumours. Long non-coding RNA (lncRNA) is a type of RNA more than 200 nucleotides in length that does not have the ability to a encode protein. The functions of lncRNAs include chromatin remodelling, transcriptional control, and post-transcriptional processing. Abnormal expression of lncRNAs is related to the progression of glioma, and their role in cancer is usually achieved via the regulation of miRNA. For example, upregulation of lncRNA HOXA-AS3 (5) promotes tumour progression of glioma and predicts a poor prognosis. By inhibiting miR-29a, lncRNA H19 regulates the angiogenesis of gliomas and the biological behaviour of endothelial cells associated with gliomas. High expression of LINC00152 is associated with tumour grade and poor prognosis in patients with HGG (6). These findings highlight the important role of lncRNAs in gliomas and may provide a potential therapeutic target for HGG. Additionally, differential expression of some molecules in GBM fields, such as IDH1 and MGMT, has been observed, with significant mutations in adults with HGG but not in children. Histone H3 is frequently mutated in children with HGG but rarely in adults with HGG (7,8). Studies have also reported that expression of EZH2 (9), WEE1 (10) and other genes is significantly higher in both adults and children with HGG. All of the above studies support that in HGG, various mutations may be unique and/or shared between adults and children.
Based on this clinical phenomenon, we comprehensively analysed the molecular expression similarities and differences between the two populations from the perspective of bioinformatics. Although there are some reports on differences between adult and paediatric gliomas, there is no systematic summary and analysis of molecular expression occurring in HGG or the possible significance of differences between adults and children (11), including differences in genes, miRNAs and lncRNAs. Moreover, differences in the clinical diagnosis and treatment (12) have not yet been considered, and expression differences between adults and children and the possible significance of these differences in HGG diagnosis have not been clearly explained in detail. In this study, we analysed and integrated sequencing data obtained from the Gene Expression Omnibus (GEO) database, prepared a detailed list and found differences in miRNAs and genes between adults and children, as well as the differences in lncRNAs among adults. Ultimately, 14 genes, 2 miRNAs, and lncRNAs that were upregulated and 17 genes, 8 miRNAs, and 8 lncRNAs that were downregulated were found to be involved in the same changes between adults and children. Different genes and miRNAs between adults and children were independently analysed; we examined the independent biological processes in which they are involved and the significance of differences. These hallmark findings extend our knowledge of HGG in children and adults. Here, we discuss possible roles in tumour biology and the current understanding of how these findings may translate into new diagnostic approaches.
Methods
Microarray analysis
Three types of chips, including gene, miRNA, and lncRNA chips, were used. Microarray data were analysed using GEO2R software (https://www.ncbi.nlm.nih.gov/geo/geo2r/). Set the two groups as HGG group and normal group, put the corresponding samples into the group for comparison analysis, For all experimental groups, differential expression was identified by a significance level of P<0.05 or |logFC| ≥2. For details, see Table 1.
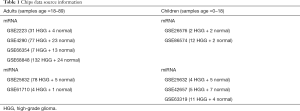
Full table
Functional enrichment analysis
Gene function annotation was performed using Database for Annotation, Visualization and Integrated Discovery v.6.8 (DAVID v.6.8) to complement Kyoto Encyclopedia of Genes and Genomes (KEGG) pathway and Gene Ontology (GO) analyses. GO analysis was limited to biological process terms; organization and visualization were achieved using the ClueGO and CluePedia plugin of the Cytoscape (v.3.5.1) platform. GO terms were connected based on overlap of shared genes and grouped by functional similarity.
LncRNA-microRNA-gene interaction network
To further elucidate correlations between genes, microRNA and lncRNA, potential interaction analyses were conducted. We obtained chips data from the GEO database and target genes from online software miRSystem (http://mirsystem.cgm.ntu.edu.tw/index.php), a database that integrates seven well-known miRNA target gene prediction programs: DIANA, miRanda, miRBridge, PicTar, PITA, rna22, and TargetScan. the prediction condition use Hit=3. We also obtained target lncRNAs from DIANA-LncBase V.2 (http://carolina.imis.athena-innovation.gr/diana_tools/web/index.php?r=lncbasev2%2Findex). Finally, we used Cytoscape to visualize relationships among genes, microRNAs and lncRNAs. To further elucidate correlations between genes, microRNAs and lncRNAs, maps were drawn network construction and analysis with Cytoscape. Information on protein-protein interactions (PPIs) was derived from Search Tool for the Retrieval of Interacting Genes/Proteins (https://string-db.org/). Only experimentally validated interactions with a score ≥0.4 were used.
Data download from TCGA and survival analysis in R
We utilized TCGA data for verification and R for data analysis. Log in to the TCGA database (https://www.cancer.gov/about-nci/organization/ccg/research/structural-genomics/tcga) online website, enter the “Launch Data Portal” interface, and download the four files “MANIFEST, Cart, metadata, clinical”. Using the CMD command under the windows system download the “MANIFEST” file or use download software gdc-transfer tool, download the mRNA and miRNA expression profile data of 517 samples of GBM. From TCGA download the original transcript files and clinical information files containing genes. Then, Perl script was used to analyse the original data. Next download the R package “edgeR” at website https://bioconductor.org/biocLite.R. The “edge R” package was employed to standardize expression data; clinical data. Use the perl script to parse out the sample survival time information in the “clinical” file. In the R system, use the “hash” package to integrate the survival time with the expression data to form the basic data for survival analysis. Then use the R package “survival” to get a PDF file of the survival curve. The clinical survival time was related to the expression value, and P<0.05 was considered statistically significant.
Hub genes and miRNAs expression differences in cancerous and paracancerous tissues
We obtained data from TCGA, use the perl script to integrate all the data in one file and convert the gene name in the form of TCGA to the ensembl website (http://asia.ensembl.org/index.html). The conventional gene name form in the downloaded “Homo_sapiens.GRCh38.93.chr.gtf.gz” file. Under the R system, “edgeR” was used to decompose the mRNA/miRNA files with differential up/down regulation and a corrected expression profile file “normalizeExp”. The “normalizeExp” file is a file necessary for our analysis of expression differences and survival curve statistics. After identifying hub genes, scatter graphs were generated using Graphpad Prism7.
Statistical analysis
Statistical analysis of the data was performed using GraphPad Prism 7.0 software. Data are presented as the mean ± SEM. Statistical comparisons among multiple groups were performed using ANOVA with the Holms-Sidak post hoc test; P<0.05 was considered statistically significant. Differences between two groups were assessed using Student’s t-test. Hub gene validation was performed using the t-test.
Results
Chip analyses
We screened 6 mRNA chips, including those for adults and children, as well as 5 miRNA chips and 1 lncRNA chip under certain conditions. Then take intersection which classified according to the up-down regulation of the expression. The classified miRNAs that were upregulated based on adult-to-child differences were used to predict target genes and then intersected with downregulated genes in the chips, and vice versa. The downregulated genes were used to cross-validate the target genes predicted by upregulated miRNAs in children and adults. These genes were then evaluated by GO, KEGG, and PPI network analyses, and hub genes were obtained. the most statistically significant core genes as well as miRNAs were verified in TCGA. The lncRNAs predicted by the most upregulated miRNAs were compared with downregulated lncRNAs in the lncRNA chip. Conversely, lncRNAs predicted by downregulated core miRNAs were compared with the lncRNAs upregulated in the lncRNA chip (see the flow Figure 1).
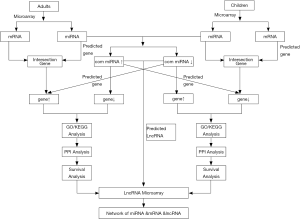
Differential analysis of mRNA and miRNA expression profiles between adults and children
We used the GEO2R tool in the GEO database to screen for differentially expressed genes and miRNAs between HGG and normal tissues (Table 1). After screening these chips, we found 5,287 genes and 20 miRNAs that were differentially expressed in adults; 6,022 genes and 47 miRNAs were differentially expressed in children. We used a heatmap to visualize miRNA expression in adults (Figure 2A) and then intersected all the miRNA chips data from adults which were classified according to logFC value is positive or negative. The resut shows 7 upregulated miRNAs and 13 downregulated miRNAs (Figure 2B). The results from the adult chips showed that 228 upregulated genes and 627 downregulated genes. Similarly, we visualized miRNAs in children using heatmap (Figure 2C). And the results from the chips for children revealed 268 upregulated genes and 458 downregulated genes (Figure 2D) and 9 upregulated miRNAs and 38 downregulated miRNAs (Tables 2-4).
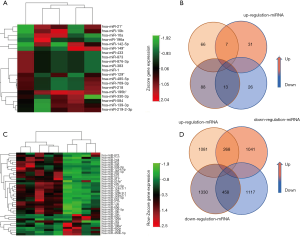
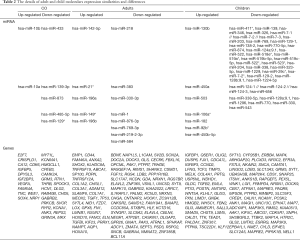
Full table
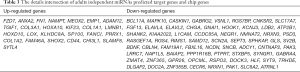
Full table
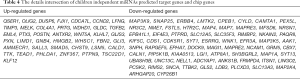
Full table
Prediction of miRNA target genes and relationships with genes on the chips
According to the competing endogenous RNA (ceRNA) hypothesis, we predicted the target genes of differential miRNAs using the online software miRSystem. First, we obtained intersecting elements using the upregulated miRNAs to predict target genes with downregulated genes based on chip data. We also used downregulated miRNAs to predict target genes with upregulated genes from the complete intersecting chip data. This method was applied equally to adults and children for data processing. The intersection of the data for the adults and children was used to obtain the intersection again. Next, we used co-up- or co-downregulated microRNAs based on the data for both the adults and children to predict target genes. Finally, to obtain genes involved in HGG in both adults and children, we took the second and third times of the intersection. The same approach was used to identify individual components for adults and children with HGG. Eventually, we obtained miRNAs and their target genes that were differentially expressed according to chip data for both adults and children, miRNAs with their target genes that were differentially expressed only according to the adult data, and the target genes of miRNAs with child-specific differences. It is worth noting that these target genes were all present in mRNA chips that we previously screened. There were 22 common terms between the upregulated miRNAs and predicted genes and the downregulated genes from the microarray. Correspondingly, there were 13 common terms between the downregulated miRNAs and predicted genes and the upregulated expression genes from the microarray (Figure 3A). We obtained 5 upregulated and 6 downregulated miRNAs and 26 upregulated and 68 downregulated independent adult networks (Figure 3B) and one independent child network (Figure 3C). Details are shown in Tables 2-4.
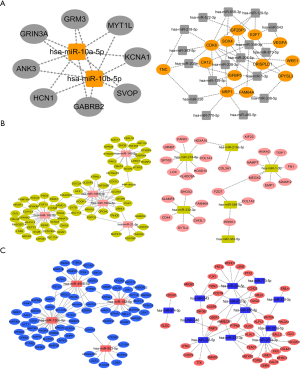
Enrichment analysis of biological processes for the three groups
Through a complex process of identifying intersections, we obtained common differences for 21 genes, including 13 upregulated and 8 downregulated genes, between adults and children. This step was repeated, and we found children-specific differences in 111 genes (41 upregulated and 70 downregulated) and adult-specific differences in 94 genes (26 upregulated and 68 downregulated). We sorted and grouped these genes based on expression values (plus or minus) with the plug-in ClueGO+CluePedia in Cytoscape 3.5.1 for biological process analysis. The results showed the co-upregulated genes to be mainly associated with axon extension, positive regulation of cell migration related to neovascularization, positive regulation of cell attachment and assembly, positive regulation of cell matrix adhesion, and regulation of retinal ganglion cell axon induction. The common downregulated genes were mainly concentrated in regulation of neuronal action potential, cardiac action potential regulation and cell reaction to magnesium ions (Figure 4A,B). The child-specific upregulated genes are related to active regulation of ossification, response to transformed growth factors, regulation of osteoblast differentiation, embryonic limb morphology, and transformation of growth factor receptor signalling pathways.
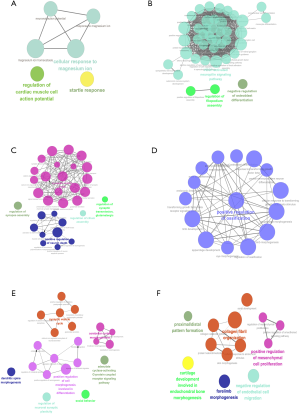
The child-specific downregulated genes are mainly involved in regulating synaptic assembly, activating JUN kinase activity, positive regulation of neuron death, and synaptic vesicular circulation (Figure 4C,D). The adult-specific upregulated genes are mainly involved in negative regulation of endothelial cell migration, positive regulation of mesenchymal cell proliferation and positive regulation of smooth signalling pathway. The adult-specific downregulated genes are associated with in adenosine cell activation of the G protein-coupled receptor signalling pathway, social behaviour, regulation of synaptic plasticity and dendritic spine morphology (Figure 4E,F).
PPI and KEGG pathway enrichment analyses for the three groups
Based on the prediction results, HGG differs between adults and children. Downregulated genes are mainly involved in the neuroactive ligand-receptor interaction, cocaine addiction, and nicotine addiction. The main genes that are predicted to participate in neuroactive ligand-receptor interactions are GABRB2, GRIN3A, and GRM3. The cocaine addiction pathway is mainly regulated by GABRB2, GRM3 and GABRB2, and GRIN3A is enriched in the nicotine addiction pathway. These co-upregulated genes are mainly regulated by CDK6, SOX4 and VEGFA, which play a role in the pathway “microRNAs in cancer (Figure 5A). The co-downregulated genes regulated by highly expressed miRNAs in adult HGG are related to the synapse, synapse part and cell junction. Representative genes are ATP2B1, BAIAP2, BDNF, and CBLN4. We also found co-upregulated genes enriched in adult HGG to be CD44, COL1A1, COL1A2, COL3A1, FN1, and HOXD10, which participate in the ECM-receptor interaction, focal adhesion, protein digestion and absorption, proteoglycans in cancer, platelet activation and the PI3K-Akt signalling pathway. And pathway “microRNAs in cancer” was found for adult HGG, involving three genes: CD44, HOXD10 and KIF23. This result is different from the above pathway “microRNAs in cancer” (Figure 5B). The particular genes regulated by low-expressed miRNAs among paediatric HGG are mainly involved in the synaptic vesicle cycle pathway, including SNAP25, SYT1, and UNC13C. One finding that attracted our attention was that CCND2, LIMD1, TEAD1, TGFB2, and WNT5A associated with highly expressed genes mediating Hippo signalling were only identified in paediatric HGG (Figure 5C).
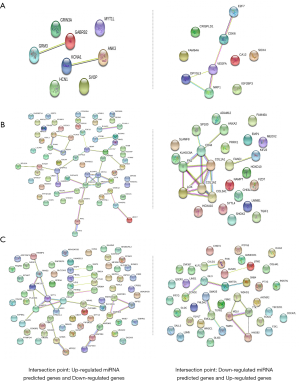
Validation of hub genes in both children and adults in The Cancer Genome Atlas (TCGA) database
We verified in the TCGA database the genes identified by gene enrichment results of the KEGG pathway analysis for both adults and children. The Perl script language was used to group the core genes and miRNAs into survival curve and integration of expression profile data. After validation by TCGA, we identified a total of 3 to present statistically significant miRNAs with the support of large-sample data. We obtained 2 increased miRNAs, miR-10a and miR-10b, and only 1 miRNA, miR-139, to be decreases (Figure 6A). Next, we used the expression data downloaded from TCGA to plot the differences between the expression levels of the three miRNAs in the disease group and the normal group, presenting a statistically significant pattern of differences that matched our screening results (Figure 6B). Similarly, we performed survival and differential expression analyses of key genes. Consistent with the trend of a shorter survival time with lower expression, comparison with normal samples revealed three downregulated genes: GABRB2, GRM3, and GRIN3A (Figure 6C,D). In contrast, consistent with the trend of a shorter survival time with higher expression, comparison with normal samples revealed three genes upregulated in glioma: CDK6, SOX4, and VEGFA. VEGFA is recognized as crucial for angiogenesis in a variety of tumours (13,14) (Figure 6E,F). These findings provide a new understanding of the commonalities between adults and children with regard to HGG.
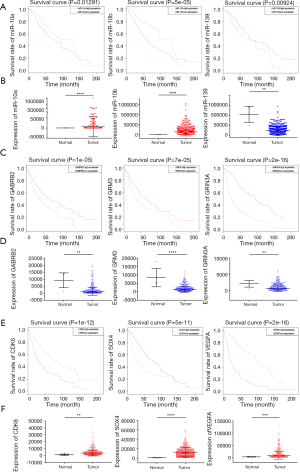
LncRNA-miRNA-mRNA network between adults and children
We analysed the chip GSE104267 with GEO2R under the conditions P<0.05 and |logFC| ≥1 and used differentially expressed key miRNAs common to both adults and children to predict target lncRNAs through the DIANA TOOL LncBase Predicted v.2. We obtained intersection prediction results and chip differences for lncRNAs. The network diagram consisted of the following: miR-10a, miR-10b, and miR-139 were identified as core miRNAs; the target lncRNAs included 9 downregulated (SNHG15, THTPA, SNHG10, SNHG14, SNHG22, LINC01006, MAG12-AS3, ACO13354.2, SLNFL1-AS1) and 4 upregulated (AC005154.6, LINC00271, LINC00969, LINC-PINT) lncRNAs. Here, we return to the intersection in the third step of the target genes predicted by miRNA and the differentially expressed genes in chips, which genes are predicted from the core miRNA. The genes included 7 downregulated (SOVP, ANK3, MYT1L, GABRB2, GRM3, HCN1, KCNA1) and 3 upregulated (CDK6, CA12, NRP1) genes. These molecules are very likely to be differentially expressed during the occurrence and development of high-level gliomas in adults and children based on our series of analyses. In this way we extended the possible molecules to complete the construction of the network. These molecules will play an important role in the diagnosis of glioma or in the differentiation of glioma between adults and children (Figure 7).
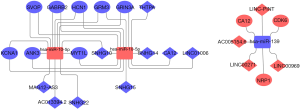
Discussion
HGGs comprise 15% to 20% of all childhood tumours of the central nervous system (CNS), and 70% to 90% of patients die within 2 years of diagnosis (15). As there are a limited number of effective methods to treat HGG, it is essential to explore the underlying molecular mechanism of HGG. Furthermore, an improved understanding of paediatric HGG is essential to identify relevant therapeutic targets. The incidence rates (16), detailed anatomic location, and pathologic spectrum of gliomas differ between children and adults, suggesting that the representation of progenitor and mature cell types, as well as the microenvironment, within the developing brain may influence the disease process. Nonetheless, the same disease occurs in both populations, and there must be some common aspects with regard to pathogenicity and anatomical location. As such, the two groups should not be analysed separately. In the present study, we examined both separately and together the two cohorts and summarized the miRNA-mRNA-lncRNA network based on expression patterns to identify differential molecules with the same trend in both children and adults with HGG, offering a broad perspective for HGG patients. miR-10a and miR-10b, members of the miR-10 family, are located on the long arm of human chromosome 17. These two molecules are well known in many species to play important regulatory roles in the developmental process of the HOX gene cluster (17). The miR-10 family has received increasing attention because of its high degree of conservation in evolution and structure. To date, hundreds of reports have confirmed that these two miRNAs can induce cancer cell proliferation in the development of malignant cancer (18,19) and participate in classic cancer pathways, such as PI3K/AKT/Wnt/β-catenin/mTOR signalling (20), in combination with target genes such as HOXD10, HOXB1, HOXB3 or noncoding RNA (17). Because this family of miRNAs is stable in the blood, it is often used as a biomarker for the diagnosis and prognosis of several diseases, including cervical cancer (21), gastric cancer (22), colorectal cancer, breast cancer, acute myeloid and leukaemia, among others. However, this family has less frequently been discussed in glioblastoma. However, their involvement in the induction of cancer cell proliferation in glioblastoma is a fact (23), and other studies have demonstrated their involvement in brain damage, ischaemia, endocrine functions and other nervous system functions. Therefore, the miR-10 family has high potential to serve as a diagnostic marker for HGG.
miR-10a, which is located at 17q21.32, upstream of HOXB4, adopts a hairpin of 28 bases (-UACCCUGUAGAUCCGAAUUUGUG-). This sequence shows consistency in a variety of mammals (17). Genome-wide profiling and identification of differentially expressed miRNAs between glioblastoma and normal neural stem cells has revealed significantly high expression of miR-10a in miRNAs (23). The same results were also found in a study on microRNAs in glioblastoma multiforme by Karsy et al. (24). Based on miRNA expression profiles of brain tumours from paediatric patients of different ages, this high expression appeared only in HGG. miR-10a exhibits moderate to weak expression in low-grade gliomas (LGGs), which provides fairly consistent evidence for our network. Nonetheless, miR-10a can drive downstream target gene expression to promote the development of cancer. The low EphA8 expression reported in HGG is caused by the binding of miR-10a to the 3’-untranslated region (UTR) of EphA8, resulting in increased expression of E-cadherin and induction of the epithelial-mesenchymal transition (EMT) to increase HGG invasion and migration (25). In addition, miR-10a can bind to the lncRNA ZFAS1 to induce the development of renal cancer (26). This reason for cancer promotion in a variety of cancers is most likely due to the fact that miR-10a can stimulate global protein translation during amino acid starvation by binding to the 5’UTR region of mRNA encoding ribosomal proteins. miR-10a promotes cell proliferation in humans and also inhibits proliferation and induces apoptosis in ovarian granulosa cells. Acting as a sponge, miR-10a targets the circular RNA (circRNA) ITCH to repress the proliferation of human epithelial ovarian cancer cells and promote apoptosis (27).
The conserved miRNA miR-10b (-UACCCUGUAGAACCGAAUUUGUG-) differs from miR-10a by only a change from one uracil to adenine (14). It is located upstream in promoter P2 of the gene HOXD4. Pre-miR-10b is the result of the cleavage of HOXD4 by the Drosha enzyme (28). It has been reported to be an important biomarker for the survival, staging treatment, and prognosis of more than a dozen malignant tumours, providing a large amount of information for patients with metastatic cancer. Our research was based on similarities between children and adults, and we noted that Iacomino et al. found that children with early obesity exhibit statistically significant downregulation of miR-10b (29). We sought to determine whether this affects the sensitivity of miR-10b as a diagnostic marker for HGG in children, In another study we found negative answer based on 120 patients indicated that expression of miR-10b in HGG was much higher than that in LGG (30,31). Many studies on miR-10b have focused on changes occurring in drug resistance during treatment of tumours (32), providing information on the growth state of cancerous tissues. Cancer cells that have developed resistance are more capable of growth and have a strong ability to invade and migrate, resulting in changes in miR-10b levels. Similar to miR-10a, miR-10b also binds to target genes or noncoding RNAs to regulate EMT in a variety of tumours (33), yet miR-10b is more capable of inducing gene methylation in tumours as well as regulation. Indeed, miR-10b has been extensively studied and shown to regulate glioma cancer stem cells and proliferation. Analysis of miR-10b expression in human glioma and glioma cell lines revealed correlations with increasing glioma grade and tumour multifocality identified on magnetic resonance imaging (34). Therefore, we consider that miR-10b can be used for HGG diagnosis in children and adults.
miR-139 (-GTGTCTCCAGTGTGGCTCGGAGGCTGGAGA-) is a miRNA frequently associated with the initiation and development of tumours in peripheral blood; it is located on human chromosome11q13.4. A large number of reports have found a significant negative correlation between miR-139 and tumours (35,36). The latest study showed that 325 samples in the Chinese Glioma Genome Atlas database exhibited increased expression of the RELB proto-oncogene (37); miR-139-3p and miR-139-5p expression was negatively correlated with RELB, consistent with our analysis of miR-139-3p. Many reports have also shown that miR-139-3p acts as a marker (38) for liver cancer, glioma, and osteosarcoma and also a marker for the diagnosis or prognosis of ovarian cancer, demonstrating that miR-139-3p has properties of a marker. Furthermore, some studies have confirmed that miR-139-3p is involved in the inhibition of glioblastoma growth and migration by targeting MDA-9/NIN1/RPNI2 (39,40) in glioblastoma, and other reports have shown that downregulation of miR-139-3p by the regulation of the lncRNA TP73-AS1 enhances in glioblastoma (41). The circRNA hsa_circ_0000592 interacts with miR-139-3p in gastric cancer (42), and their binding is involved in the biological process of tumour development. Catanzaro et al. found that miR-139-5p regulates the proliferation of supratentorial LGGs via the PI3K/AKT/mTORC1 signalling pathway, confirming the role of miR-139 in childhood gliomas (43). Overall, miR-139 may be used as a biomarker to distinguish HGG between children and adults.
In similar studies (44), the detailed information of the chips in the GEO database to find the tumor or disease-related chips we want to study, and perform a preliminary screening through the GEO 2R. According to the theory that the miRNA can play a sponge adsorption role in the website, the expression of the miRNA and the gene are reversely intersected, and the core genes in the result are analyzed by GO/KEGG/PPI analysis. Database verification through a larger number of databases, such as TCGA/Oncomine/CGGA, or experimental verification, is one of the mainstream methods for biomarker screening of markers. With the development of bioinformatics and the enrichment of databases, there will be new computing methods or online computing websites for researchers to richer algorithms to find HGG markers. In addition, according to research by Medarova et al. (45). A non-invasive imaging method is used to provide excellent information magnetic resonance imaging (MRI) from deep tissue in the body. Contrast agents have been found to have two functions through MRI, enabling bimodal preclinical imaging for cancer diagnosis. In addition, it can also be used as a treatment platform. This can be achieved by functionalizing the use of therapeutic materials superparamagnetic iron oxide nanoparticles to build a multifunctional contrast agent based on bimodal imaging and therapeutic capabilities, which can provide information about disease conditions and regulate oncogenes through bimodal non-invasive imaging Contrast agents (miRNA and/or mRNA) may have a significant impact on cancer. The synthesis and application of oligonucleotide-functionalized therapeutic magnetic nanoparticles have verified tumor uptake of contrast agents by non-invasive MRI and in vivo fluorescence imaging. The therapeutic oligonucleotide component can be a double-stranded siRNA molecule for silencing mRNA causing disease, or it can be an antisense oligonucleotide to inhibit miRNA over-expressed in cancer-tumor. This study provides a promising premise for the diagnosis and treatment for HGG of our screened markers.
Conclusions
Although gliomas have been analysed numerous times and there are many differences in the molecular expression of gliomas between children and adults, our study is the first to explore common molecular expression profiles in HGG between children and adults. The miRNA-mRNA-lncRNA network may be useful for the diagnosis, treatment and prognosis of HGG. We performed a large number of GEO database analyses and found data and opinions supporting our theory in TCGA’s large sample database and individual research reports. Our reliable network provides a possible direction for further exploration of potential coding/noncoding RNA biomarkers in HGG. However, the true sensitivity and specificity of these molecules as potential markers of HGG remain to be verified. With the continuous improvements in bioinformatics, we present a reminder that common circRNAs in children and adults should be addressed, which will be the direction of our next efforts.
Acknowledgments
Funding: The National Natural Science Foundation of China (Grant No. 81502676) and the Heilongjiang Provincial Postdoctoral Science Foundation (approval No. LBH-Z14157) provide support for Dr. Wu Liande and eFund (WLD-QN110). Li Jieyuan is a postdoctoral researcher at Televie. Open topic for the Key Laboratory of Functional and Clinical Transformation of Fujian Medical College (JNYLC1809).
Footnote
Conflicts of Interest: The authors have no conflicts of interest to declare.
Ethical Statement: The authors are accountable for all aspects of the work in ensuring that questions related to the accuracy or integrity of any part of the work are appropriately investigated and resolved. Comply with all international, national and/or institutional animal care and use guidelines. Comply with the ethical standards of the institution and/or the National Research Council, as well as the 1964 Helsinki Declaration and its subsequent amendments or similar ethical standards. Informed consent was obtained from all individual participants included in the study.
Open Access Statement: This is an Open Access article distributed in accordance with the Creative Commons Attribution-NonCommercial-NoDerivs 4.0 International License (CC BY-NC-ND 4.0), which permits the non-commercial replication and distribution of the article with the strict proviso that no changes or edits are made and the original work is properly cited (including links to both the formal publication through the relevant DOI and the license). See: https://creativecommons.org/licenses/by-nc-nd/4.0/.
References
- Greenall SA, Lim YC, Mitchell CB, et al. Cyclin-dependent kinase 7 is a therapeutic target in high-grade glioma. Oncogenesis 2017;6:e336. [Crossref] [PubMed]
- Baker SJ, Ellison DW, Gutmann DH. Pediatric gliomas as neurodevelopmental disorders. Glia 2016;64:879-95. [PubMed]
- Petersen CT, Krenciute G. Next Generation CAR T Cells for the Immunotherapy of High-Grade Glioma. Front Oncol 2019;9:69. [Crossref] [PubMed]
- Barciszewska AM. MicroRNAs as efficient biomarkers in high-grade gliomas. Folia Neuropathol 2016;54:369-74. [Crossref] [PubMed]
- Wu F, Zhang C, Cai J, et al. Upregulation of long noncoding RNA HOXA-AS3 promotes tumor progression and predicts poor prognosis in glioma. Oncotarget 2017;8:53110-23. [PubMed]
- Wang W, Wu F, Zhao Z, et al. Long noncoding RNA LINC00152 is a potential prognostic biomarker in patients with high-grade glioma. CNS Neurosci Ther 2018;24:957-66. [Crossref] [PubMed]
- Castel D, Philippe C, Kergrohen T, et al. Transcriptomic and epigenetic profiling of 'diffuse midline gliomas, H3 K27M-mutant' discriminate two subgroups based on the type of histone H3 mutated and not supratentorial or infratentorial location. Acta Neuropathol Commun 2018;6:117. [Crossref] [PubMed]
- Duchatel RJ, Jackson ER, Alvaro F, et al. Signal Transduction in Diffuse Intrinsic Pontine Glioma. Proteomics 2019;19:e1800479. [Crossref] [PubMed]
- Karlowee V, Amatya VJ, Takayasu T, et al. Immunostaining of Increased Expression of Enhancer of Zeste Homolog 2 (EZH2) in Diffuse Midline Glioma H3K27M-Mutant Patients with Poor Survival. Pathobiology 2019;86:152-61. [Crossref] [PubMed]
- Mueller S, Hashizume R, Yang X, et al. Targeting Wee1 for the treatment of pediatric high-grade gliomas. Neuro Oncol 2014;16:352-60. [Crossref] [PubMed]
- Muhammed A, Gaber MS, Elbeltagy M, et al. Risk stratification of pediatric high-grade glioma: a newly proposed prognostic score. Childs Nerv Syst 2019;35:2355-62. [Crossref] [PubMed]
- Carceller F, Jerome NP, Fowkes LA, et al. Post-radiotherapy apparent diffusion coefficient (ADC) in children and young adults with high-grade gliomas and diffuse intrinsic pontine gliomas. Pediatr Hematol Oncol 2019;36:103-12. [Crossref] [PubMed]
- Ferrara N, Adamis AP. Ten years of anti-vascular endothelial growth factor therapy. Nat Rev Drug Discov 2016;15:385-403. [Crossref] [PubMed]
- Greenberger S, Boscolo E, Adini I, et al. Corticosteroid suppression of VEGF-A in infantile hemangioma-derived stem cells. N Engl J Med 2010;362:1005-13. [Crossref] [PubMed]
- Broniscer A, Gajjar A. Supratentorial high-grade astrocytoma and diffuse brainstem glioma: two challenges for the pediatric oncologist. Oncologist 2004;9:197-206. [Crossref] [PubMed]
- Crocetti E, Trama A, Stiller C, et al. Epidemiology of glial and non-glial brain tumours in Europe. Eur J Cancer 2012;48:1532-42. [Crossref] [PubMed]
- Lund AH. miR-10 in development and cancer. Cell Death Differ 2010;17:209-14. [Crossref] [PubMed]
- Kim J, Siverly AN, Chen D, et al. Ablation of miR-10b Suppresses Oncogene-Induced Mammary Tumorigenesis and Metastasis and Reactivates Tumor-Suppressive Pathways. Cancer Res 2016;76:6424-35. [Crossref] [PubMed]
- Tu J, Cheung HH, Lu G, et al. MicroRNA-10a promotes granulosa cells tumor development via PTEN-AKT/Wnt regulatory axis. Cell Death Dis 2018;9:1076. [Crossref] [PubMed]
- Wang J, Wang A, He H, et al. Trametenolic acid B protects against cerebral ischemia and reperfusion injury through modulation of microRNA-10a and PI3K/Akt/mTOR signaling pathways. Biomed Pharmacother 2019;112:108692. [Crossref] [PubMed]
- Pardini B, De Maria D, Francavilla A, et al. MicroRNAs as markers of progression in cervical cancer: a systematic review. BMC Cancer 2018;18:696. [Crossref] [PubMed]
- Pereira AL, Magalhaes L, Moreira FC, et al. Epigenetic Field Cancerization in Gastric Cancer: microRNAs as Promising Biomarkers. J Cancer 2019;10:1560-9. [Crossref] [PubMed]
- Cheng JQ, Lang M-F, Yang S, et al. Genome-wide profiling identified a set of miRNAs that are differentially expressed in glioblastoma stem cells and normal neural stem cells. PLoS One 2012;7:e36248. [Crossref] [PubMed]
- Karsy M, Arslan E, Moy F. Current Progress on Understanding MicroRNAs in Glioblastoma Multiforme. Genes Cancer 2012;3:3-15. [Crossref] [PubMed]
- Yan Y, Wang Q, Yan XL, et al. miR-10a controls glioma migration and invasion through regulating epithelial–mesenchymal transition via EphA8. FEBS Lett 2015;589:756-65. [Crossref] [PubMed]
- Dong D, Mu Z, Wei N, et al. Long non-coding RNA ZFAS1 promotes proliferation and metastasis of clear cell renal cell carcinoma via targeting miR-10a/SKA1 pathway. Biomed Pharmacother 2019;111:917-25. [Crossref] [PubMed]
- Luo L, Gao YQ, Sun XF. Circular RNA ITCH suppresses proliferation and promotes apoptosis in human epithelial ovarian cancer cells by sponging miR-10a-alpha. Eur Rev Med Pharmacol Sci 2018;22:8119-26. [PubMed]
- Phua SL, Sivakamasundari V, Shao Y, et al. Nuclear accumulation of an uncapped RNA produced by Drosha cleavage of a transcript encoding miR-10b and HOXD4. PLoS One 2011;6:e25689. [Crossref] [PubMed]
- Iacomino G, Russo P, Marena P, et al. Circulating microRNAs are associated with early childhood obesity: results of the I.Family Study. Genes Nutr 2019;14:2. [Crossref] [PubMed]
- Tantawy M, Elzayat MG, Yehia D, et al. Identification of microRNA signature in different pediatric brain tumors. Genet Mol Biol 2018;41:27-34. [Crossref] [PubMed]
- Visani M, de Biase D, Marucci G, et al. Expression of 19 microRNAs in glioblastoma and comparison with other brain neoplasia of grades I-III. Mol Oncol 2014;8:417-30. [Crossref] [PubMed]
- Port M, Glaesener S, Ruf C, et al. Micro-RNA expression in cisplatin resistant germ cell tumor cell lines. Mol Cancer 2011;10:52. [Crossref] [PubMed]
- Liu S, Wang L, Li Y, et al. Long non-coding RNA CHRF promotes proliferation and mesenchymal transition (EMT) in prostate cancer cell line PC3 requiring up-regulating microRNA-10b. Biol Chem 2019. [Epub ahead of print]. [PubMed]
- Sasayama T, Nishihara M, Kondoh T, et al. MicroRNA-10b is overexpressed in malignant glioma and associated with tumor invasive factors, uPAR and RhoC. 2009;125:1407-13.
- Gu J, Chen Y, Huang H, et al. Gene module based regulator inference identifying miR-139 as a tumor suppressor in colorectal cancer. Mol Biosyst 2014;10:3249-54. [Crossref] [PubMed]
- Zhang Y, Shen WL, Shi ML, et al. Involvement of aberrant miR-139/Jun feedback loop in human gastric cancer. Biochim Biophys Acta 2015;1853:481-8. [Crossref] [PubMed]
- Zeng F, Wang K, Huang R, et al. RELB: A novel prognostic marker for glioblastoma as identified by population-based analysis. Oncol Lett 2019;18:386-94. [PubMed]
- Min L, Zhu S, Chen L, et al. Evaluation of circulating small extracellular vesicles derived miRNAs as biomarkers of early colon cancer: a comparison with plasma total miRNAs. J Extracell Vesicles 2019;8:1643670. [Crossref] [PubMed]
- Shi L, Yuan Y, Li HY. MicroRNA-139-3p suppresses growth and metastasis of glioblastoma via inhibition of NIN1/RPNI2 binding protein 1 homolog. Eur Rev Med Pharmacol Sci 2019;23:4264-74. [PubMed]
- Tian W, Wu W, Li X, et al. MiRNA-139-3p inhibits the proliferation, invasion, and migration of human glioma cells by targeting MDA-9/syntenin. Biochem Biophys Res Commun 2019;508:295-301. [Crossref] [PubMed]
- Xia Z, Yang X, Wu S, et al. LncRNA TP73-AS1 down-regulates miR-139-3p to promote retinoblastoma cell proliferation. Biosci Rep 2019. [Crossref] [PubMed]
- Liang M, Liu Z, Lin H, et al. High-throughput sequencing reveals circular RNA hsa_circ_0000592 as a novel player in the carcinogenesis of gastric carcinoma. Biosci Rep 2019. [Crossref] [PubMed]
- Catanzaro G, Besharat ZM, Miele E, et al. The miR-139-5p regulates proliferation of supratentorial paediatric low-grade gliomas by targeting the PI3K/AKT/mTORC1 signalling. Neuropathol Appl Neurobiol 2018;44:687-706. [Crossref] [PubMed]
- Huang GZ, Wu QQ, Zheng ZN, et al. Identification of Candidate Biomarkers and Analysis of Prognostic Values in Oral Squamous Cell Carcinoma. Front Oncol 2019;9:1054. [Crossref] [PubMed]
- Medarova Z, Balcioglu M, Yigit MV. Controlling RNA Expression in Cancer Using Iron Oxide Nanoparticles Detectable by MRI and In Vivo Optical Imaging. Methods Mol Biol 2016;1372:163-79. [Crossref] [PubMed]