A two-circular RNA signature of donor circFOXN2 and circNECTIN3 predicts early allograft dysfunction after liver transplantation
Introduction
Liver transplantation is considered to be the radical curative therapy for end-stage liver disease (1,2). Although perioperative management and surgical techniques have greatly improved, there are still some complications affecting the prognosis of recipients following liver transplantation. The incidence of early allograft dysfunction (EAD) following liver transplantation varies from 20% to 40% according to the liver transplantation centre (3-5). EAD significantly affects the survival rates of both grafts and recipients. It is important to achieve early prediction of EAD. Several studies have attempted to predict EAD based on clinical parameters, such as the recipient Model for End-stage Liver Disease (MELD) score or cold ischemia time (CIT) (6,7). In addition, microRNAs (miRNAs) and genes in donor liver tissues have been used to predict EAD (8,9).
Circular RNAs (circRNAs) are special endogenous RNAs with circular structures. The formation of circRNAs mainly goes through four spliceosome-dependent, intron-pairing-driven, lariat-driven and protein factor-associated circularization paths (10). CircRNAs play a key role in regulating gene expression by acting as a sponge for miRNAs (11). Due to the circular structure, circRNAs are often more stable than linear RNA molecules, which make the former a perfect candidate as predictive biomarkers. A previous study has shown that circRNAs have good predictive value for lung adenocarcinoma (12). Other researchers have also found the predictive value of circRNA in patients with active tuberculosis (13).
However, the expression profile and predictive value of graft circular RNAs in EAD are still unclear. In the present study, we aim to investigate the expression profile of circular RNAs in donor liver tissue and its predictive value for EAD after liver transplantation.
Methods
Study population and data collection
A total of 115 liver transplantation recipients between January 2015 and December 2017 at the First Affiliated Hospital, Zhejiang University School of Medicine, were included in this study. This study was approved by the hospital ethics committee and complied with the World Medical Association’s Declaration of Helsinki revised in 2013. Informed consent was obtained from all patients. The exclusion criteria included recipients younger than 18 years, recipients undergoing multiorgan transplantation or re-transplantation, and recipients with missing essential data for analysis. Donor liver tissue was collected and frozen in liquid nitrogen before storage. All specimens were stored at −80 °C.
EAD was defined by one or more of the following parameters in the revised criteria: (I) total bilirubin (TB) level ≥10 mg/dL on postoperative day 7; (II) international normalized ratio (INR) ≥1.6 on postoperative day 7; (III) alanine aminotransferase (ALT) or aspartate aminotransferase (AST) levels >3,000 IU/L within the first 7 postoperative days (3,14). The following clinical data were collected: recipient data including age, sex, preoperative MELD score, and operative time, and donor data including donor age, sex, body mass index (BMI), donor liver macrovesicular steatosis and CIT.
CircRNA sequencing
Total RNA was extracted from donor liver tissues using TRIzol reagent (Invitrogen) and treated with DNase I (DNA free kit, Ambion). The RiboMinus Eukaryote Kit (Qiagen) was used to remove ribosomal RNA, and then the samples were treated with RNase R (Epicenter). RNA sequencing (RNA-seq) libraries were prepared using the NEBNext® UltraTM RNA Library Prep Kit and sequenced using the Illumina HiSeq 3000 platform at RiboBio Co. Ltd., Guangzhou, China (15,16).
Quantitative real-time PCR (qRT-PCR) and Sanger sequencing
Total RNA was isolated from donor liver tissues using TRIzol reagent (Invitrogen). The concentration and purity of RNA were measured using NanoDrop ND-1000 (Thermo Fisher Scientific, Inc., USA). Reverse transcription and qPCR were carried out using a Geneseed® II First Strand cDNA Synthesis Kit and qPCR SYBR® Green Master Mix (Guangzhou Geneseed Biotech Co., China), respectively, according to the manufacturer’s instructions on an ABI 7500 Fast Real-Time PCR System (Applied Biosystems, CA, USA). The primers were synthesized from Geneseed Biotech Co. (Guangzhou, China). The data were analysed by the 2−ΔΔCT method (17). GAPDH was used as the internal control. The sequences of primers were listed in Table S1. The qRT-PCR products of circRNAs were inserted into the T vector and analysed using Sanger sequencing.
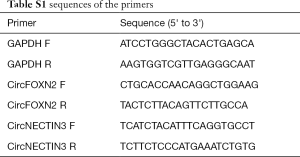
Full table
Functional analysis and ceRNA network construction
Kyoto Encyclopaedia of Genes and Genomes (KEGG, https://www.kegg.jp) and Gene Ontology (GO, http://geneontology.org) were employed to analyse the host genes of dysregulated circRNAs. Potential miRNAs targets of circRNAs were predicted using RNAhybrid and TargetScan. In addition, potential interactions between the miRNAs and genes were predicted with miRWalk and TargetScan. The circRNA-miRNA-mRNA network was constructed using Cytoscape.
Statistical analysis
Continuous parameters in the study were presented as the median and interquartile range or mean ± SEM. To compare different groups, Student’s t-test and the Mann-Whitney U test were performed where appropriate. Multivariate analysis included variables with a P value <0.05 using a binary logistic regression method. Youden Index was used to select the best cut-off value for EAD. P<0.05 was considered statistically significant. Statistical analyses were performed using GraphPad Prism version 7 (GraphPad Software, Inc. La Jolla, CA, USA) and SPSS version 21.0 (Chicago, IL, USA).
Results
Expression profiles of circRNAs in the EAD and non-EAD groups
As shown in the heat map (Figure 1A) and volcano plot (Figure 1B), 442 differentially expressed circRNAs were observed (fold change >2, P<0.05) in 3 pairs of donor liver tissue between the EAD and non-EAD groups. Among these circRNAs, 219 were downregulated in the EAD group, and 223 were upregulated. The chromosomal distribution of differentially expressed circRNAs is illustrated in Figure 1C. The top 3 Gene Ontology (GO) terms included binding, protein binding and catalytic activity among molecular functions; intracellular part, organelle and intracellular among cellular components; cellular process, metabolic process and organic substance metabolic process among biological processes (Figure 2A). The top 3 KEGG pathways related to host genes of the dysregulated circRNAs included arginine biosynthesis, tryptophan metabolism and glyoxylate and dicarboxylate metabolism (Figure 2B).
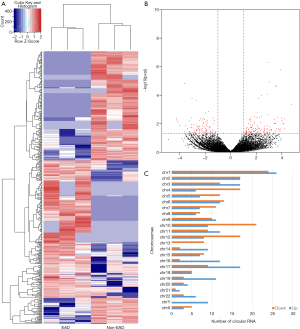
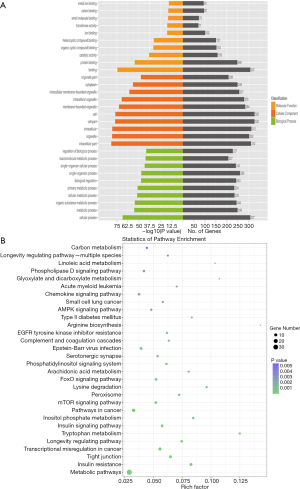
Significantly downregulated circFOXN2 and circNECTIN3 in EAD
According to the distribution and degree of difference based on raw data analysis, circFOXN2 and circNECTIN3 were selected for further research. Then, qRT-PCR was used to detect the expression levels of circFOXN2 and circNECTIN3 in 115 donor liver tissues. The backspliced junctions for circFOXN2 and circNECTIN3 in qRT-PCR products were confirmed by Sanger sequencing (Figure 3A). CircFOXN2 and circNECTIN3 were significantly downregulated in the EAD group (circFOXN2: P=0.038, Figure 3B; circNECTIN3: P=0.024, Figure 3C).
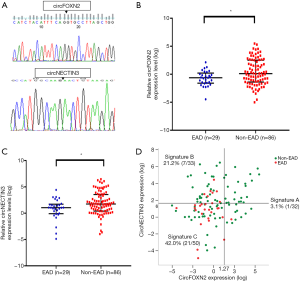
A two-circRNA signature combing donor circFOXN2 and circNECTIN3
According to the Youden index, the cut-off value was set as for 1.27 circFOXN2 (log) and 1.66 for circNECTIN3 (log). We further construct circular RNA signature (A, B and C) according to the cut-off value of circFOXN2 and circNECTIN3. Among the 115 recipients, 32 recipients with high circFOXN2 expression were classified as circular RNA signature A. Recipients with low circFOXN2 expression were categorized into circular RNA signature B (n=33, high circNECTIN3 expression) and C (n=50, low circNECTIN3 expression). The incidence rates of EAD in signature A, B and C were 3.1%, 21.2% and 42.0%, respectively (Figure 3D).
A novel predictive model for EAD
The results of univariate analysis of risk factors for EAD are demonstrated in Table 1. Preoperative MELD score (P=0.000), CIT (P=0.000) and circular RNA signature (P=0.000) were significantly different between the EAD and non-EAD groups. Furthermore, CIT and circular RNA signature were independent risk factors for EAD based on multivariate binary logistic regression analysis (P=0.000, P=0.013, respectively, Table 2). A novel model combing circFOXN2 and circNECTIN3 was constructed according to the independent risk factors. Predictive model score = −7.390 + 0.404*CIT + 2.318*(Signature B) + 3.111*(Signature C). As is shown in Figure 4, the novel model showed an improved predictive value with an AUC of 0.870 (95% CI: 0.797–0.942).
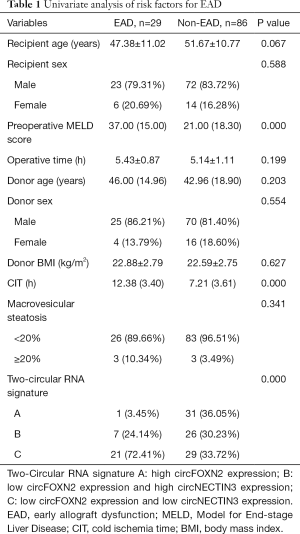
Full table
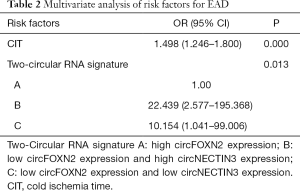
Full table
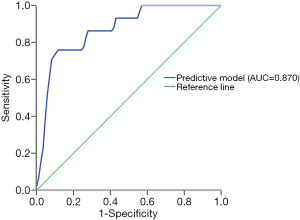
CircFOXN2/circNECTIN3-miR-135b-5p/miR-149-5p network construction
RNAhybrid and TargetScan were employed to predict the target miRNAs of circFOXN2 and circNECTIN3. To identify the potential targets related to EAD, we selected miRNAs associated with ischemia/reperfusion injury (IRI), as illustrated in the network. As is shown in the network, hsa-miR-135b-5p and hsa-miR-149-5p were the potential targets of both circFOXN2 and circNECTIN3. Subsequently, the target genes of the miRNAs were predicted by miRWalk and TargetScan. The circFOXN2/circNECTIN3-miRNA-mRNA network is shown in Figure 5.
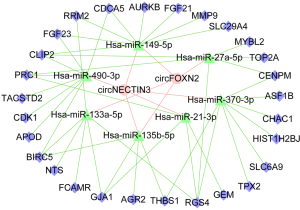
Discussion
EAD is a reversible injury following liver transplantation. Many factors contribute to the incidence of EAD, including graft IRI, hepatic steatosis, donor BMI, donor age and donor serum sodium level (6,18-20). Among these risk factors, graft IRI is generally considered one of the most important risk factors. Similar results have been found in the present study, showing that CIT was an independent risk factor for EAD. In addition to the clinical factors, Kurian et al. investigated the molecular mechanisms underlying EAD, defined relevant pathways (PPARα and NF-κB) and targets (such as CXCL1, IL1, TRAF6, TIPARP, and TNFRSF1B) associated with the incidence of EAD (9). CircRNAs are often more stable than linear RNAs, which makes circRNAs promising biomarkers and therapeutic targets (12). However, the expression profile and predictive value of circRNAs in EAD remain unknown.
Previous research found that donor biology plays an important role in tumour recurrence and new-onset diabetes mellitus after liver transplantation (21,22). Therefore, donor biology is associated with the outcomes after liver transplantation. In this study, graft circRNA profiles were established through circRNA sequencing. We further identified that circFOXN2 and circNECTIN3 were significantly downregulated in the EAD group. Furthermore, we developed circular RNA signature (A, B and C) according to the expression of circFOXN2 and circNECTIN3. We also constructed a novel model combing circular RNA signature to predict EAD with good performance. The present study indicates that donor biology plays an important role in the development of EAD.
Previous studies have attempted to predict EAD. Hoyer et al. developed an EAD prediction score based on clinical variables of donors (6). A nomogram was also constructed based on the clinical parameters of donors and recipients to predict individual incidence of EAD (7). In addition to clinical variables, donor biology has been used to predict EAD. Kurian et al. defined a gene expression signature in donor liver tissue with high predictive value for EAD (9). Additionally, a recent study found that miR-146b-5p can act as a biomarker of EAD (8). However, the role of stable circRNAs, which are perfect candidate predictive indicators, has not been elucidated before. The present study demonstrated the expression profile and predictive value of circRNAs in donor liver tissues.
In the present study, we selected miRNAs related to IRI and constructed a circFOXN2/circNECTIN3-miR-135b-5p/miR-149-5p network using database prediction. CircRNAs can act as miRNA sponges. MiRNAs are small, noncoding RNAs that play a pivotal role in the post-transcriptional regulation of gene expression. To explore the potential pathways related to EAD, we selected miRNAs associated with IRI to be construct a network. Many miRNAs, such as miR-21-3p and miR-490-3p, are involved in IRI (23,24). Regarding cardiac IRI, a recent study found that miR-149-5p could negatively regulate sphingosine-1-phosphate receptor 2 levels and improve the outcomes of transient middle cerebral artery occlusion in rats (25). Xie et al. also demonstrated that miR-135b-5p inhibition could protect cardiomyocytes from IRI in a mouse model through the JAK2/STAT3 pathway (26). Furthermore, Xing et al. found that miR-27a-5p was upregulated during hepatic IRI in mice (27). In addition, a previous study found that miR-133a-5p reversed the protective effect of propofol in a rat hepatic IRI model (28). Moreover, Li et al. illustrated that miR-370 levels were significantly increased in a mouse hepatic IRI model (29). Similar results have also been found in recent research (30). In the present study, ceRNA network analysis showed that circFOXN2 and circNECTIN3 regulated miR-135b-5p and miR-149-5p, which play important roles in IRI. Thus, the circFOXN2/circNECTIN3-miR-135b-5p/miR-149-5p network provides a novel avenue for exploring the incidence of EAD.
Nevertheless, this study had several limitations. First, this is a single-centre retrospective study. Second, the sample size was relatively small. Further prospective multicentre studies with large samples are needed to validate the results.
In conclusion, this study demonstrated the differential expression of 442 circRNAs between the EAD and non-EAD groups. The novel model combing donor circular RNA signature is effective at predicting EAD. Additionally, the circFOXN2/circNECTIN3-miRNA-mRNA network provides potential targets for predicting and treating EAD.
Acknowledgments
The authors acknowledge the support from Reform Program of Graduate Education for Professional Degree (Zhejiang University School of Medicine) and Academic Rising Star Program (Zhejiang University).
Funding: This study was supported by grants from National Natural Science Funds for Distinguished Young Scholar of China [Grant number: 81625003], National Natural Science Foundation of China (Grant number. 81570589, 81800578, 81702858, 81930016), National Science and Technology Major Project (Grant number: 2017ZX10203205), Changjiang Scholar Program of China to XX and Innovative Research Groups of National Natural Science Foundation of China (No. 81721091).
Footnote
Conflicts of Interest: The authors have no conflicts of interest to declare.
Ethical Statement: The authors are accountable for all aspects of the work in ensuring that questions related to the accuracy or integrity of any part of the work are appropriately investigated and resolved. This study was approved by the hospital ethics committee and complied with the Declaration of Helsinki. In addition, informed consent was obtained from all patients to allow the use of samples and clinical data for investigation. All collected samples were manipulated according to the protocols approved by the hospital ethics committee.
References
- Lee HW, Song GW, Lee SG, et al. Patient selection by tumor markers in liver transplantation for advanced hepatocellular carcinoma. Liver Transpl 2018;24:1243-51. [Crossref] [PubMed]
- Kim SH, Song GW, Hwang S, et al. Feasibility of ABO-incompatible adult living donor liver transplantation for acute-on-chronic liver failure. Hepatobiliary Pancreat Dis Int 2017;16:662-4. [Crossref] [PubMed]
- Olthoff KM, Kulik L, Samstein B, et al. Validation of a current definition of early allograft dysfunction in liver transplant recipients and analysis of risk factors. Liver Transpl 2010;16:943-9. [Crossref] [PubMed]
- Lee DD, Singh A, Burns JM, et al. Early allograft dysfunction in liver transplantation with donation after cardiac death donors results in inferior survival. Liver Transpl 2014;20:1447-53. [PubMed]
- Wadei HM, Lee DD, Croome KP, et al. Early Allograft Dysfunction After Liver Transplantation Is Associated With Short- and Long-Term Kidney Function Impairment. AM J Transplant 2016;16:850-9. [Crossref] [PubMed]
- Hoyer DP, Paul A, Gallinat A, et al. Donor information based prediction of early allograft dysfunction and outcome in liver transplantation. Liver Int 2015;35:156-63. [Crossref] [PubMed]
- Yang L, Xin EY, Liao B, et al. Development and Validation of a Nomogram for Predicting Incidence of Early Allograft Dysfunction Following Liver Transplantation. Transplant Proc 2017;49:1357-63. [Crossref] [PubMed]
- Li C, Zhao Q, Zhang W, et al. MicroRNA-146b-5p Identified in Porcine Liver Donation Model is Associated with Early Allograft Dysfunction in Human Liver Transplantation. Med Sci Monit 2017;23:5876-84. [Crossref] [PubMed]
- Kurian SM, Fouraschen SM, Langfelder P, et al. Genomic profiles and predictors of early allograft dysfunction after human liver transplantation. AM J Transplant 2015;15:1605-14. [Crossref] [PubMed]
- Liu J, Liu T, Wang X, et al. Circles reshaping the RNA world: from waste to treasure. Mol Cancer 2017;16:58. [Crossref] [PubMed]
- Han D, Li J, Wang H, et al. Circular RNA circMTO1 acts as the sponge of microRNA-9 to suppress hepatocellular carcinoma progression. Hepatology 2017;66:1151-64. [Crossref] [PubMed]
- Liu XX, Yang YE, Liu X, et al. A two-circular RNA signature as a noninvasive diagnostic biomarker for lung adenocarcinoma. J Transl Med 2019;17:50. [Crossref] [PubMed]
- Fu Y, Wang J, Qiao J, et al. Signature of circular RNAs in peripheral blood mononuclear cells from patients with active tuberculosis. J Cell Mol Med 2019;23:1917-25. [Crossref] [PubMed]
- Salvalaggio P, Afonso RC, Felga G, et al. A proposal to grade the severity of early allograft dysfunction after liver transplantation. Einstein (Sao Paulo) 2013;11:23-31. [Crossref] [PubMed]
- Zhang Y, Zhang XO, Chen T, et al. Circular intronic long noncoding RNAs. Mol Cell 2013;51:792-806. [Crossref] [PubMed]
- Yu J, Xu QG, Wang ZG, et al. Circular RNA cSMARCA5 inhibits growth and metastasis in hepatocellular carcinoma. J Hepatol 2018;68:1214-27. [Crossref] [PubMed]
- Jeck WR, Sharpless NE. Detecting and characterizing circular RNAs. Nat Biotechnol 2014;32:453-61. [Crossref] [PubMed]
- Ali JM, Davies SE, Brais RJ, et al. Analysis of ischemia/reperfusion injury in time-zero biopsies predicts liver allograft outcomes. Liver Transpl 2015;21:487-99. [Crossref] [PubMed]
- Lee DD, Croome KP, Shalev JA, et al. Early allograft dysfunction after liver transplantation: an intermediate outcome measure for targeted improvements. Ann Hepatol 2016;15:53-60. [Crossref] [PubMed]
- Totsuka E, Dodson F, Urakami A, et al. Influence of high donor serum sodium levels on early postoperative graft function in human liver transplantation: effect of correction of donor hypernatremia. Liver Transpl Surg 1999;5:421-8. [Crossref] [PubMed]
- Xu X, Ling Q, Wang J, et al. Donor miR-196a-2 polymorphism is associated with hepatocellular carcinoma recurrence after liver transplantation in a Han Chinese population. Int J Cancer 2016;138:620-9. [Crossref] [PubMed]
- Ling Q, Xie H, Lu D, et al. Association between donor and recipient TCF7L2 gene polymorphisms and the risk of new-onset diabetes mellitus after liver transplantation in a Han Chinese population. J Hepatol 2013;58:271-7. [Crossref] [PubMed]
- Jiang Y, Xie H, Tu W, et al. Exosomes secreted by HUVECs attenuate hypoxia/reoxygenation-induced apoptosis in neural cells by suppressing miR-21-3p. Am J Transl Res 2018;10:3529-41. [PubMed]
- Zhang C, Huang J, An W. Hepatic stimulator substance resists hepatic ischemia/reperfusion injury by regulating Drp1 translocation and activation. Hepatology 2017;66:1989-2001. [Crossref] [PubMed]
- Wan Y, Jin HJ, Zhu YY, et al. MicroRNA-149-5p regulates blood-brain barrier permeability after transient middle cerebral artery occlusion in rats by targeting S1PR2 of pericytes. FASEB J 2018;32:3133-48. [Crossref] [PubMed]
- Xie XJ, Fan DM, Xi K, et al. Suppression of microRNA-135b-5p protects against myocardial ischemia/reperfusion injury by activating JAK2/STAT3 signaling pathway in mice during sevoflurane anesthesia. Biosci Rep 2017. [Crossref] [PubMed]
- Xing Y, Li J, Li SP, et al. MiR-27a-5p regulates apoptosis of liver ischemia-reperfusion injury in mice by targeting Bach1. J Cell Biochem 2018;119:10376-83. [Crossref] [PubMed]
- Hao W, Zhao ZH, Meng QT, et al. Propofol protects against hepatic ischemia/reperfusion injury via miR-133a-5p regulating the expression of MAPK6. Cell Biol Int 2017;41:495-504. [Crossref] [PubMed]
- Li L, Li G, Yu C, et al. A role of microRNA-370 in hepatic ischaemia-reperfusion injury by targeting transforming growth factor-beta receptor II. Liver Int 2015;35:1124-32. [Crossref] [PubMed]
- Zhu J, Zhu F, Song W, et al. Altered miR-370 expression in hepatic ischemia-reperfusion injury correlates with the level of nuclear kappa B (NF-kappaB) related factors. Gene 2017;607:23-30. [Crossref] [PubMed]