Single-cell transcriptomics reveals the alteration of peripheral blood mononuclear cells driven by sepsis
Introduction
Sepsis is considered as a serious systemic inflammatory response syndrome caused by a dysregulated host response to infection (1). Sepsis can progress to multiple organs (brain, lung, liver, and so on) dysfunction and septic shock, which is one of the most common causes of death in intensive care units (ICU) (2,3). The causes of sepsis are complex and not understood clearly, generally speaking, sepsis is recognized to involve early activation of pro- and anti-inflammatory responses, but the detailed processes of immunity and inflammation are still poorly understood (1,4,5).
Peripheral blood mononuclear cells (PBMCs) are defined as any blood cell isolated from peripheral blood with a round nucleus, including monocytes, dendritic cells, and lymphocytes (6). As the core part of the immune system, PBMCs are the key drivers of the immune responses, involving many diseases (7-9). The genes about inflammatory response were downregulated, while genes involved in apoptosis were increased in monocytes of sepsis (10). It was shown that the immature neutrophils could differentiate into monocytic cells in the circulation during sepsis (11). Moreover, the major histocompatibility antigen HLA-DR was reduced in septic monocytes and as a potential therapeutic target for sepsis (12-14). Myeloid-derived suppressor cells (MDSCs) could suppress the immune response and protective in septic animal models (15,16). In a 28-day clinical retrospective study, the count of lymphocyte was higher in survivors than non-survivors and increased secondary infective development, associated with 28-day survival independently (17). Though, these descriptions of immune cells of peripheral blood and sepsis are partial and defective.
Single-cell transcriptomics sequencing has developed rapidly and been applied to various disciplines in recent years, and the method makes it possible to accurately characterize cell types and associated gene expression profiles in a mixture (18-20). In this current study, we creatively profiled biological characteristics of PBMCs comprehensively in sepsis by single-cell transcriptomics, the alterations of monocytes were observed emphatically, the function and biological characteristics varied greatly in different subsets of monocytes, expression of some representative genes was high-associated with septic indicators in clusters of monocytes driven by sepsis.
Methods
Isolation of PBMCs
Peripheral blood (about 10 mL) was collected in the heparin anticoagulant according to a standard manner, then transferred to a 50 mL centrifuge tube and diluted with 20 mL PBS. Fifteen mL Ficoll-Hypaque Solution (Meijingbio, China) was added to the tube and mixed gently. One thousand and five hundred rpm/min, centrifuge for 30 minutes, and transfer PBMC cell layer to another tube. Add enough PBS and mix thoroughly, centrifuge for 5 minutes at 1,800 rpm/min, then abandon supernatant. All the above experiments were performed at 4 degrees Celsius.
Single-cell RNA-sequencing (scRNA-seq)
All samples were uniformly resuscitated and washed twice by PBS (with 0.1% BSA), then the cells were 1 ml stain buffer (with 0.1% BSA) and counted. The viability of cells in all samples assessed >80%. Cells for all samples were labeled with sample tags (BD Human Single-Cell Multiplexing Kit, USA), counted and multiplexed, ready for single-cell capture. Single-cell capture and cDNA synthesis were performed by the BD Rhapsody Single-Cell Analysis System (BD, USA), according to the manufacturer’s recommendations. Libraries were sequenced on multiple runs of Illumina Next-Seq.
Unsupervised clustering of cells and t-distributed Stochastic Neighbor Embedding (tSNE)-visualization
With the data of SC-sequencing, unsupervised clustering of cells was performed with R (Seurat package version 2.2). Genes were filtered out if these expressed in less than two cells. Cells with >200 genes and <10% of mitochondrial genes were further processed. Then, Seurat arithmetic was used to calculate the variation coefficient of genes. Based on the first 1,500 highest alterable genes, principle part analysis (PCA) was used to perform dimensionality reduction of all data. A k-nearest neighbor graph was executed with Euclidean distances in the space of the first 10 significant principal components. The Louvain Modularity optimization algorithm clustered the cells in the picture, and then the results of unsupervised clustering were visualized by using tSNE project. Cells with high expression of hemoglobin were abandoned.
Identification of marker genes and cell-type annotation
Differential expression of every cluster was calculated by using the ‘bimod’ test as implemented in Seurat Find Markers function. Genes were found as marker genes when these were with a log2 average differential expression 0.585 and P<0.05. Canonical markers of known cell types annotated cell clusters.
Hallmark signal pathway analysis
The hallmark gene sets were downloaded from the Molecular Signature Database, which was publicly available. The activity of the signal pathway for each cell was scored by using gene set variation analysis (GSVA) methods follow a standardized strategy. The difference of pathway in two groups was calculated using a t-test. P value <0.05 was considered significantly different.
Statistical analysis of population shifts
The percent composition of cell types altered significantly in samples was evaluated using the Benjamin-Hochberg correction and nonparametric Kruskal-Wallis with Dunn’s multiple comparisons test.
Clinical data statistics
Every donor (including 4 normal controls and 7 patients with sepsis) was treated as a separate analytical unit, all clinical results shown in this paper were presented as mean ± SEM, and then differential analysis was performed with t-test by using GraphPad Prism 7. It was considered a significant difference when P value <0.05.
Results
Single-cell transcriptome profiling of PBMCs comparing control and sepsis
To characterize the cell types and gene expression profiles of PBMCs in sepsis, the PBMCs were isolated from 7 septic patients (SP1-SP7) and 4 controls (2 donors combined into 1 sample, total 2 samples for sequencing, CT1-CT2), and then theses samples were analyzed by single-cell RNA sequencing technology (BD Rhapsody) (Figure 1A). The total numbers of recovered cells were 26,674, including 3,183 for controls and 23,491 for septic patients, with the normal range of median gene (Figure 1B). Based on expression profiles, PBMCs were clustered for 9 cellular compositions shown by t distributed stochastic neighbor embedding (tSNE); every cell fraction was represented by bar plot (Figure 1C). All cells were also differentiated by the disease status (control and sepsis) to show the alterations of cell clusters driven by sepsis (Figure 1D). We identified 6 major cell types, including: B cells (MS4A1+), DC cells (CD11b+), Monocyte (CD68+), natural killer cells (CD3D− and NKG7+), natural killer T cells (CD56+), T cells (CD3D+) (Figure 1E). In a word, the PBMCs from sepsis and controls were profiled comprehensively by single-cell transcriptomes, the consists of cells that were defined for 6 clusters which were not library-specific.
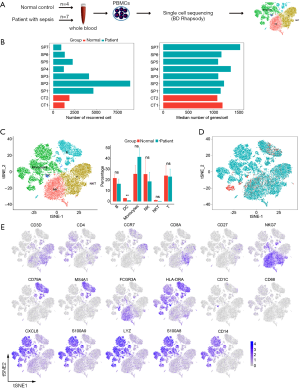
The alterations of T cell subsets in sepsis-related transcriptomic signatures
T cells were a huge cell type and seemed to be able to be clustered into more subsets (Figure 1C). To further understand the specific population shift of T cells, we extracted all the T cells from control and sepsis by using the Seurat R package. T cells were clustered into 2 subsets (cytotoxic T and helper T) (Figure 2A). There was no significant difference between normal control and sepsis in the expression distribution of representative selected genes (Figure 2B). Compared the top differential genes between controls and patients, we found RPS26, HLA-C, RBM38 etc. were down-regulated and TXNIP, PPDPF, PINM2 et al. were highly expressed in sepsis relative to control, shown a broader alteration in gene expression induced by sepsis (Figure 2C). To evaluate the distinctive biological properties between normal and septic T cells, we performed GSVA to analyze the hallmark signal pathway. Sepsis driven T cell was different from normal in representative pathways, for example, contrasting T cells from sepsis versus control, both estrogen response, p53 pathway and apoptosis were enriched, but IL-6 pathway was significantly inhibited (Figure 2D). Together, T cells could be further clustered into cytotoxic T and helper T whose population was nearly identical in normal, and sepsis, the gene expression and signal pathway of T cell of sepsis were different from normal.
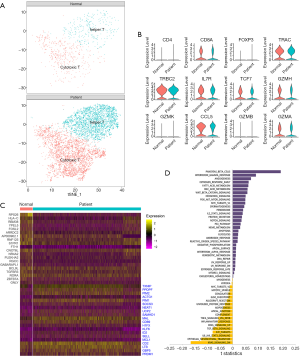
Sepsis drove the alterations of monocyte subsets in single-cell transcriptomics level
We further clustered monocyte into 6 subsets, and then assessed the septic influence on monocyte. Compared with control, the expansion of 5 monocyte subsets was significant in sepsis, including CD14+NEAT1+SELL+ monocyte, CD14+NEAT1+CCR1 monocyte, NEAT1+CD163+ monocyte, CCL3L1+ monocyte, CD16+ monocyte, while the alteration in cluster distribution of macrophages was obvious without remarkable quantity variance in control and sepsis (Figure 3A). CD14, S100A8, and S100A9 were abundantly expressed in both normal and sepsis-driven monocytes, showing a classical profile of monocyte in transcriptional level (Figure 3B). Also, HLA-DRB1 and HLA-DRA were significantly down-regulated in sepsis-driven monocyte compared with normal, which hinted the immune failure of monocytes (Figure 3B,C). Inflammation-associated proteins were acutely activated and increased in septic monocytes, such as CCL3, IL1B, CCL4, NLRP3 (Figure 3D). Similarly, inflammation-associated pathways (IL-6 signal pathway and complement response etc.) were enhanced in monocytes from sepsis. Meanwhile, the apoptosis of monocytes was activated, and oxidative phosphorylation was inhibited distinctly (Figure 3D). Interestingly, the androgen response was also obviously decreased (Figure 3D).
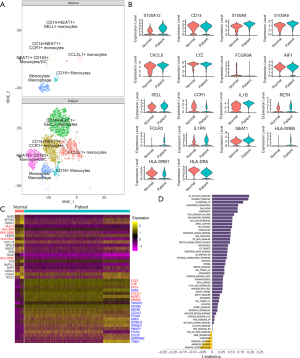
The monocytes from sepsis were clustered into 6 subsets, and most of those were proliferated mightily (Figures 3A,4A). It was worth studying in detail the alterations of each subset in all samples individually. The proportion of cells in the 6 clusters fluctuated wildly in sepsis (Figure 4B). In contrast to other subsets, both cluster 8 (monocytes/macrophage) and cluster 11 (CD16+ monocytes) were striking. We respectively compared the significant gene signatures of cluster 8 and cluster 11 between normal and sepsis, to assess their distinct biological properties driven by sepsis (Figure 4C,D). It was worth noting that both HLA-DQB1 and HLA-DRA were down-regulated in a total of 7 sepsis compared with control (Figure 4C). Here, CCL family (CCL4L, CCL3, CCL4, CCL3L) was increased in sepsis compared with normal, but the difference of their expression level in 7 sepsis patients was prominent (Figure 4D). Besides, the expression of TNF was significantly up-regulated in patients related to control, which was highly consistent in all sepsis samples (Figure 4D). Hierarchical clustering analysis revealed that there were significant differences in representative signaling pathways among 6 subsets of sepsis-driven monocytes, for example, cluster 5 was activated while both cluster 3 and cluster 7 were inhibited mightily in most of the typical pathways (Figure 4E).
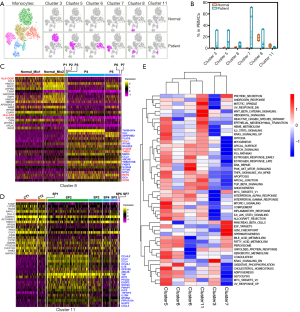
In summary, the monocytes were further clustered into 6 subsets, which proliferated mightily and were altered in biological properties driven by sepsis.
The correlation between septic indexes and clusters of monocytes
To study the association between septic indexes and monocytes, we performed a Spearman’s rank correlation analysis. The proportion of cluster 7 and cluster 11 in PBMCs was significantly positively correlated with NEWS Score first day in ICU and PaO2/FiO2 first day in ICU, respectively (Figure 5A). Moreover, it was proved in detail the correlation between clinical indicators and the expression of representative genes in clusters of septic monocytes (Figure 5B).
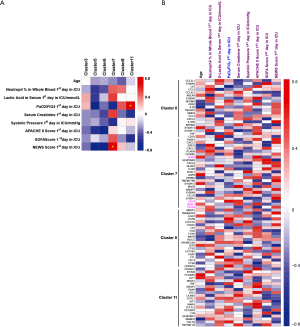
Discussion
In this current paper, we collected PBMCs from donors and sepsis patients, and then analyzed the alterations of PBMCs in function and composition driven by sepsis by single-cell transcriptomic sequencing based on BD Rhapsody. PBMCs were divided for 6 kinds of cell types, including B cell, DC, monocytes, NK cell, NKT cell, and T cell. We further analyzed the alteration of T cells and monocytes driven by sepsis. Notably, monocytes could be clustered into 6 subsets in a detailed manner (CD14+NEAT1+SELL+ monocyte, CD14+NEAT1+CCR1+ monocyte, NEAT1+CD163+ monocyte, CCL3L1+ monocyte, macrophage, CD16+ monocyte), most of them were proliferated in sepsis. The biological properties (population migration, genes expression, signal pathways) of T cell and monocytes were showed, most of which were associated with immunity and inflammation. Finally, we found that the expression of some representative genes in clusters of monocytes was highly associated with septic clinical indexes.
Sepsis is a critical condition caused by infection with unknown etiology and rapid progression, which is one of the deadliest diseases in ICU (1-3). PBMCs are the general term of mononuclear cells in peripheral blood, as an important component of the immune system, are widely involved in inflammation and immune processes caused by various causes (7-9). Sepsis can alter the biological characteristics of PBMCs, and the imbalance of PBMCs homeostasis is also the main cause of sepsis (1). The population of the T cells does not fluctuate wildly in the septic process (21), which was verified (Figure 1C). Immunosuppression is the noble cause of death in septic patients, and T lymphocyte exhaustion is its main characteristic (22). We observed that the p53 pathway and apoptosis were activated in septic T cells; in addition, as an inhibitor for proliferation and differentiation of T cell, estrogen response was tempestuously enriched driven by sepsis (23), all the evidence above pointed to subsequent T cell exhaustion. Immunosuppression of monocytes is a noble cause of the development of sepsis, which was suggested by HLA-DR reduction (12-14). We found down-regulation of HLA-DR was detected in both total monocytes (Figure 3C) and cluster 8 (Figure 4C) in sepsis compared with control, but this phenomenon could not be repeated in cluster 11 of monocytes (Figure 4D). The six clusters of monocytes shown great heterogeneity in cell proliferation and the signaling pathway is driven by sepsis (Figure 4B,E), which suggested that the function and biological characteristics varied greatly in different subsets of monocytes. More attention should be paid to the heterogeneity of immune cells in the future research and treatment of sepsis.
We further analyzed the correlation between the representative genes profile in clusters of monocytes and clinical indicators of patients. The expression of some genes was high-associated with septic clinical indexes, which suggested the possibility of finding a new target for diagnosing or treating sepsis by single-cell sequencing. This idea is novel and requires much further research.
Unexpectedly, LncRNA, such as NEAT1 (Figure 3B), MALAT1, and LINC01871, were covered in Monocytes, DC, and other cells (cluster 7, defined as NEAT1+CD163+ monocytes/DC; cluster 17, NEAT1+CD1c+DC). High expressed NEAT1 in sepsis was sporadically reported (24,25) suggested a diagnostic biomarker of sepsis and involved NF-κB proinflammation signal pathway. In this study, we showed a raised NEAT1 at least in four clusters of mononuclear cells especially Monocytes and DC, implies a positive function in differing of monocytes. Firstly, we confirmed the primary sub-group expressed NEAT1 are monocytes and differed cells derived monocytes using single-cell transcriptome sequencing technology. And a potential role of NEAT1 in sepsis was explained via correlation analysis with clinic indexes like PaO2/FiO2, Serum Creatinine and APACHE II Score etc. in cluster 7. Deeply cogitation about NEAT1 mechanism in monocytes differing via spongy reacted with mRNAs or co-forced with proteins will be essential next work.
In conclusion, though this article was not deeply researched, our single-cell data uncovered detailed biological properties of PBMCs from septic patients in terms of gene profile and signal pathway signatures, providing insights about etiology and potential treatment of sepsis.
Acknowledgments
Funding: GDPH Scientific Research Funds for Leading Medical Talents and Distinguished Young Scholars in Guangdong Province (KJ012019452).
Footnote
Conflicts of Interest: The authors have no conflicts of interest to declare.
Ethical Statement: The authors are accountable for all aspects of the work in ensuring that questions related to the accuracy or integrity of any part of the work are appropriately investigated and resolved.
References
- Singer M, Deutschman CS, Seymour CW, et al. The Third International Consensus Definitions for Sepsis and Septic Shock (Sepsis-3). JAMA 2016;315:801-10. [Crossref] [PubMed]
- Shahreyar M, Fahhoum R, Akinseye O, et al. Severe sepsis and cardiac arrhythmias. Ann Transl Med 2018;6:6. [Crossref] [PubMed]
- Fleischmann C, Scherag A, Adhikari NK, et al. Assessment of Global Incidence and Mortality of Hospital-treated Sepsis. Current Estimates and Limitations. Am J Respir Crit Care Med 2016;193:259-72. [Crossref] [PubMed]
- Angus DC, van der Poll T. Severe sepsis and septic shock. N Engl J Med 2013;369:840-51. [Crossref] [PubMed]
- Wiersinga WJ, Leopold SJ, Cranendonk DR, et al. Host innate immune responses to sepsis. Virulence 2014;5:36-44. [Crossref] [PubMed]
- Kleiveland CR. Peripheral Blood Mononuclear Cells. In: Verhoeckx K, Cotter P, López-Expósito I, et al., editors. The Impact of Food Bioactives on Health: in vitro and ex vivo models. Cham (CH): Springer; 2015.
- Hu YQ, Liu P, Mu ZL, et al. Aryl hydrocarbon receptor expression in serum, peripheral blood ononuclear cells, and skin lesions of patients with atopic dermatitis and its correlation with disease severity. Chin Med J (Engl) 2020;133:148-53. [Crossref] [PubMed]
- Shen G, Sun S, Huang J, et al. Dynamic changes of T cell receptor repertoires in patients with hepatitis B virus-related acute-on-chronic liver failure. Hepatol Int 2020;14:47-56. [Crossref] [PubMed]
- Luo T, Zheng F, Wang K, et al. A single-cell map for the transcriptomic signatures of peripheral blood mononuclear cells in end-stage renal disease. Nephrol Dial Transplant 2019. [Epub ahead of print]. [Crossref] [PubMed]
- Tang BM, McLean AS, Dawes IW, et al. Gene-expression profiling of peripheral blood mononuclear cells in sepsis. Crit Care Med 2009;37:882-8. [Crossref] [PubMed]
- Köffel R, Meshcheryakova A, Warszawska J, et al. Monocytic cell differentiation from band-stage neutrophils under inflammatory conditions via MKK6 activation. Blood 2014;124:2713-24. [Crossref] [PubMed]
- Venet F, Lukaszewicz AC, Payen D, et al. Monitoring the immune response in sepsis: a rational approach to administration of immunoadjuvant therapies. Curr Opin Immunol 2013;25:477-83. [Crossref] [PubMed]
- Lukaszewicz AC, Grienay M, Resche-Rigon M, et al. Monocytic HLA-DR expression in intensive care patients: Interes for prognosis and secondary infection prediction. Crit Care Med 2009;37:2746-52. [PubMed]
- Döcke WD, Randow F, Syrbe U, et al. Monocyte deactivation in septic patients: restoration by IFN-gamma treatment. Nat Med 1997;3:678-81. [Crossref] [PubMed]
- Ostrand-Rosenberg S, Sinha P. Myeloid-derived suppressor cells: linking inflammation and cancer. J Immunol 2009;182:4499-506. [Crossref] [PubMed]
- Derive M, Bouazza Y, Alauzet C, et al. Myeloid-derived suppressor cells control icrobial sepsis. Intensive Care Med 2012;38:1040-9. [Crossref] [PubMed]
- Drewry AM, Samra N, Skrupky LP, et al. Persistent lymphopenia after diagnosis of sepsis predicts mortality. Shock 2014;42:383-91. [Crossref] [PubMed]
- Papalexi E, Satija R. Single-cell RNA sequencing to explore immune cell heterogeneity. Nat Rev Immunol 2018;18:35-45. [Crossref] [PubMed]
- Krenkel O, Hundertmark J, Ritz TP, et al. Single Cell RNA Sequencing Identifies Subsets of Hepatic Stellate Cells and Myofibroblasts in Liver Fibrosis. Cells 2019. [Crossref] [PubMed]
- Magen A, Nie J, Ciucci T, et al. Single-Cell Profiling Defines Transcriptomic Signatures Specific to Tumor-Reactive versus Virus-Responsive CD4+ T Cells. Cell Rep 2019;29:3019-32.e6. [Crossref] [PubMed]
- Delano MJ, Ward PA. The immune system’s role in sepsis progression, resolution, and long-term outcome. Immunol Rev 2016;274:330-53. [Crossref] [PubMed]
- Shin J, Jin M. Potential Immunotherapeutics for Immunosuppression in Sepsis. Biomol Ther (Seoul) 2017;25:569-77. [Crossref] [PubMed]
- Tai P, Wang J, Jin H, et al. Induction of regulatory T cells by physiological level estrogen. J Cell Physiol 2008;214:456-64. [Crossref] [PubMed]
- Huang S, Qian K, Zhu Y, et al. Diagnostic Value of the lncRNA NEAT1 in Peripheral Blood Mononuclear Cells of Patients with sepsis. Dis Markers 2017;2017:7962836. [Crossref] [PubMed]
- Chen Y, Qiu J, Chen B, et al. Long non-coding RNA NEAT1 plays an important role in sepsis-induced acute kidney injury by targeting miR-204 and modulating the NF-κB pathway. Int Immunopharmacol 2018;59:252-60. [Crossref] [PubMed]