Identification of HO-1 as a novel biomarker for graft acute cellular rejection and prognosis prediction after liver transplantation
Introduction
Liver transplantation (LT) has been the most effective and widely used treatment for patients with end-stage liver diseases and hepatocellular carcinoma (HCC) fulfilling the selecting criteria. With the improvements in surgical techniques, postoperative management, and immunosuppressive therapies, the long-term outcomes of LT have continued to improve over the last several decades, with 5- and 10-year survival reaching 70% and 60%, respectively (1,2). Despite the advances, acute cellular rejection (ACR) remains the most common and serious complication during early post-transplant period, occurring in 10–40% of patients and potentially leading to irreversible allograft failure (1). Meanwhile, increase use of immunosuppression due to ACR may result in inevitable complications, i.e., infections, metabolic disorders, nephrotoxicity, and ultimately malignancy (3). All the above, would substantially impair life quality, increase morbidity, and reduce long-term survival. Therefore, early identification of patients at risk of developing graft rejection is of paramount importance for saving the graft and increasing the long-term survival. To date, the diagnosis of ACR relies mainly on clinical manifestations and histopathological evidence. However, clinical symptoms like fever, abdominal pain, increasing ascites and laboratory abnormalities in ACR are usually insensitive and nonspecific, which can’t reflect the severity of ACR and support the early diagnosis. Liver biopsy remains the gold standard for ACR diagnosis (4), but its expense, inconvenience, susceptibility of sampling error and invasiveness with moderate to severe complications ultimately highlight the need for finding out non-invasive and reliable diagnostic biomarkers for ACR.
Proteomic analysis illuminates a better understanding of biological processes in both healthy and diseased conditions, which has been largely applied to investigate the mechanisms of rejection in organ transplantations (5). Isobaric tags for relative and absolute quantitation (iTRAQ), has become a superior mass-based quantitative proteomic technique by allowing simultaneous identification of protein profiles obtained from multiple and biologically complex samples (6). With the advantages of high-throughput, great accuracy and high sensitivity, iTRAQ has been widely used for systematically characterizing the unique proteomic profile and investigating the molecular mechanisms of human diseases (7). Combined with bioinformatics tools, the data obtained from iTRAQ can be further analyzed and successfully used for identification of novel diagnostic and prognostic biomarkers (8). For instance, Liu et al. comprehensively analyzed the proteome characteristics of chronic liver allograft dysfunction (CLAD) in rat models and discovered that targeting CXCL4 protected against the development of CLAD after LT by reducing liver fibrosis (9). However, the combination of iTRAQ-based quantitative proteomics and bioinformatics analysis has rarely been applied to the field of biomarker identification for ACR in LT.
To our knowledge, this is a novel study investigating preoperative protein profile changes and its influence on the prognosis of LT between ACR and non-rejection recipients. This study first aimed to comprehensively characterize the preoperative proteomic alterations among ACR patients using iTRAQ-based proteomics. Combined with bioinformatics analysis, novel potential biomarkers for ACR were identified and then the prognostic value of the identified biomarker were verified by a combination of tissue microarray (TMA) analysis and retrospective cohort study.
Methods
Patients and study design
This study design proceeded by two separate settings (Figure 1).
The training set was designed to investigate the proteome differences and to identify potential biomarkers between ACR and non-rejection group. The protein samples extracted from ACR (n=3) and non-rejection recipients (n=3) were analyzed by iTRAQ-based proteomics. Differentially expressed proteins (DEPs) were screened and the potential biomarkers for ACR were identified by comprehensive bioinformatics. For subjects, ACR were confirmed by histological findings according to the Banff criteria (10). All ACR episodes were resolved according to our previous reported protocol (11). Subjects included in non-rejection group were selected based on the following criteria: maintaining stable graft function and lacking rejection signs in the presence of immunosuppressive drugs (IS) for at least 6 months.
The validating set was performed to validate the prognostic value of the candidate biomarker [heme oxygenase-1 (HO-1)] identified in training set by a retrospective study based on a TMA constructed from two independent cohorts: ACR cohort (n=80) and non-rejection cohort (n=57) (Table 1). Survival analysis was performed to verify the predictive value of HO-1 for graft rejection. Subjects included in ACR cohort and non-rejection cohort followed the same standards as the training set.
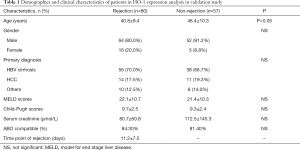
Full table
All subjects in this study were included between January 2012 and August 2013 from Department of Hepatobiliary and Pancreatic Surgery, First Affiliated Hospital, Zhejiang University. All subjects met the indications for LT. The pathological diagnosis of ACR of the subjects by needle core liver biopsy was confirmed independently by two experienced pathology experts. The study protocol was approved by the Ethics Committee of The First Affiliated Hospital, School of Medicine, Zhejiang University. No donor liver was from executed prisoners in this study. All recipients were informed with written informed consent for the tissue collection and biopsy.
iTRAQ-based quantitative proteomic study
The detailed protocols for iTRAQ protein identification were listed in Supplementary files.
The DEPs were identified based on following standards: the fold change of protein expression (rejection group vs. non-rejection group) was >1.2 for up-regulation and <0.83 for down-regulation, with P<0.05.
Gene ontology (GO) and pathway enrichment analysis
GO annotation, the international standardization of gene function classification system, can provide updating Ontologies, including molecular function, cellular component and biological process, to describe the biological characteristics of large genes and proteins in certain organism (12). In this work, GO enrichment analysis of all DEPs was performed using the Panther Classification System (http://www.pantherdb.org/) (13), compared to the whole human genome. Pathway enrichment analysis was implemented by PANTHER Pathways, which can classify the enriched pathways, providing important information about molecular interactions and reaction networks of the DEPs. P<0.05 was considered statistically significant.
Protein-protein interaction (PPI) network construction
The Search Tool for the Retrieval of Interacting Genes (STRING) database (http://string-db.org) (version 10.0) was used to analyze and visualize the PPI network of the DEPs (14). To exclude false positive interactions as possible, only DEPs with high confidence scores (combined score >0.7) were selected; the sources of interactions were based exclusively on databases and previous experimental results, while excluding other predictions from String (such as gene fusion and text mining). Then, the PPI network was reconstructed and visualized using Cytoscape software (version 3.4.0, http://cytoscape.org/) (15).
Module analysis of the PPI network
To find functional network modules or clusters from PPI network, the module analysis was performed using the Molecular Complex Detection (MCODE) in Cytoscape (16), with a connectivity degree cutoff =2. Then the significant modules with MCODE scores >4 and nodes >10 were selected. The pathway enrichment analysis of the significant modules was performed in Panther system as mentioned above, with P<0.05.
Tissue microarray and immunohistochemical (IHC) staining
The identified candidate protein (HO-1) was validated in two independent cohorts of 137 patients’ liver tissues using TMA constructed from formalin-fixed, paraffin embedded tissue blocks. To avoid bias of IHC interpretation, the samples were placed on the TMA blindly. Each sample was arranged in triplicate to avoid tissue loss and tissue heterogeneity. The detailed protocol of IHC staining and intensity scoring was listed in Supplementary Method.
Statistical analysis
Statistical analysis was conducted using SPSS 15.0 software (SPSS, Chicago, IL, USA). Fisher’s exact test was used for categorical variables analysis. χ2 test was used to analyze the immunohistochemical staining results and evaluate the correlation between HO-1 expression and the clinical outcomes. The overall survival was estimated using Kaplan-Meier method and the differences in survival between two groups were compared using the log-rank test. Multivariate analysis was performed using the Cox proportional hazards regression model. P<0.05 was considered statistically significant, with 95% confidence interval (CI).
Results
Demographic and clinical characteristics of patients
In the training set, both ACR and non-rejection group were matched for age, gender and primary diagnosis for protein identification. Details of the subjects referred to Table S1.
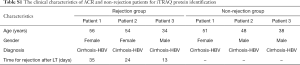
Full table
For the validating set, two cohorts of patients were included to validate HO-1 identified in the training set. The demographic and clinical characteristics of patients were summarized in Table 1. There were no differences in terms of gender, primary diagnosis, Meld scores, Child-Pugh scores, serum creatinine, or ABO compatible between two groups.
Proteomic analysis and identification of the DEPs
The basic information of the proteome profile identified by iTRAQ referred to Figure S1. A total of 287 proteins were identified as DEPs finally, including 173 (60.3%) up-regulated proteins and 114 (39.7%) down-regulated proteins among ACR vs. non-rejection group. The detailed information of the DEPs was show in Table S2.
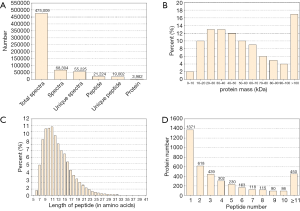

Full table
GO classification analysis of the DEPs
To identify the possible biological and functional properties of 287 DEPs, GO analysis was performed. The results showed different changes in biological characteristics occurred in ACR (Figure S2). In the biological process category, up-regulated DEPs were mainly enriched in biological regulation, signaling, immune system process, biological adhesion etc., while down-regulated DEPs were mainly enriched in metabolic process and catabolic process (Figure S2A). For molecular function, up-regulated DEPs were mainly observed in catalytic activity, nucleic acid binding and those terms in pathway regulation, while most down-regulated DEPs were more observed in catalytic/transferase/oxidoreductase activity (Figure S2B). In brief, up-regulated DEPs were mainly related to the regulation of allograft rejection while down-regulated DEPs may play a greater role in the impaired catabolic and metabolic functions during rejection. As immune system changes were important during ACR, the detailed information of the DEPs involved in this term was summarized in Table S3.
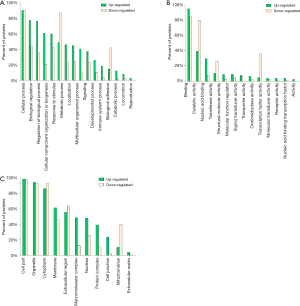
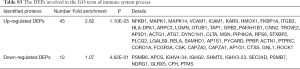
Full table
Pathway enrichment analysis
To further study the detailed molecular mechanisms of ACR, pathway enrichment analysis was performed. The enriched pathways significantly differed between up- and down-regulated DEPs (Figure 2). The mostly enriched pathways in up-regulated DEPs were integrin signaling pathway, Inflammation mediated by chemokine and cytokine signaling pathway, EGF receptor/FGF signaling pathway, T/B cell activation, etc. The down-regulated DEPs were mainly presented in Serine glycine biosynthesis and 5-hydroxytryptamine degradation. Consistent with the GO analysis, up-regulated DEPs were significantly enriched in pathways involved in immune system process and down-regulated DEPs were mainly involved in metabolic pathways.
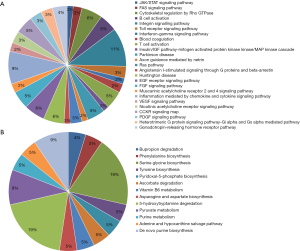
PPI network analysis of the DEPs
Next, 287 DEPs were summited into STRING database to visualized and analyze the PPI network. Through screening of high confidence interactions (scores >0.7), the PPI network was visualized in Figure 3. To better understand the relation between PPI network and protein expression level, the PPI network was reconstructed in Cytoscape (Figure S3). The up- and the down-regulated DEPs were clearly demarcated into 2 parts and connected by the interaction between HO-1 (gene symbol: HMOX1) and biliverdin reductase A (BVR) (gene symbol: BLVRA) in PPI network.
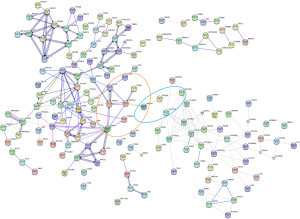
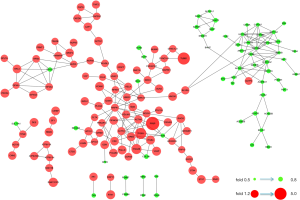
Module analysis and pathway enrichment
To identify functional modules from PPI network and find hub-proteins, the top 3 significant functional modules were obtained by module analysis (Figure S4). Except for the up-regulated BLVRA, all other proteins in module 1 (18 nodes, MCODE score =4.941) were down-regulated. On the contrary, the vast majority of the proteins in module 2 (30 nodes, MCODE score =4.138) were up-regulated. As for module 3 (10 nodes, MCODE score =4.000), only 40S ribosomal protein S23 (RPS23) was down-regulated.
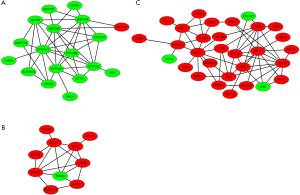
The module pathway enrichment was then analyzed (Table S4). The proteins in module 1 were significantly enriched in metabolic pathways. The proteins in module 2 were significantly enriched in Integrin signaling pathway, B/T cell activation, EGF receptor/FGF signaling pathway, Inflammation mediated by chemokine and cytokine signaling pathway, etc. No significant enrichment was found in module 3 (P>0.05).
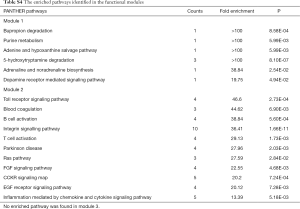
Full table
Module 2, with most proteins up-regulated, accounted for the majority of enrich pathways of 173 up-regulated DEPs (Table S4, Figure 2), which were mainly about regulation of inflammation and immune system process, suggesting that the activation of module 2 in allograft rejection; The enriched pathways in module 1, with most proteins down-regulated, were mainly involved in metabolic process, indicating the inhibition of module 1 during metabolic abnormalities in rejection. Notably, module 1 and module 2 were connected directly by HO-1-BLVRA interaction (Figures 3,S4). Pathway analysis showed that HIF-1 signaling pathway (including HMOX1, MAPK1, RELA, CUL2) and porphyrin and chlorophyll metabolism (including BLVRA, HMOX1, UGT1A9, UGT2B4) were enriched in the linking part (Figure 3). HO-1 was presented in both two enriched pathways in the connection part, indicating the potentially crucial role of HO-1 protein in the regulation of immunoreaction and metabolic function during rejection.
Identification of high HO-1 expression in ACR recipients by TMA analysis
According to the aforementioned results of GO, pathway, module analysis, HO-1 may serve as the key molecule in regulating allograft rejection. Therefore, HO-1 was selected as the candidate protein for further investigation.
To validate HO-1 expression level and its significance in ACR, HO-1 expression was detected by TMA in two independent cohorts, as described in Methods. The demographic and clinical characteristics of subjects in two cohorts were presented in Table 1.
Representative immunohistochemical staining for HO-1 in two cohorts was shown in Figure 4A. Statistical analysis of HO-1 expression in TMA showed that in consistent with proteomics quantification, HO-1 expression level was significantly higher in ACR group (n=80) than in non-rejection group (n=57) (Figure 4B, P<0.05).
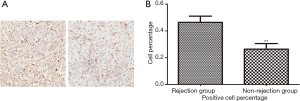
Identification of preoperative HO-1 expression as a potential prognostic factor for ACR
To investigate the association between preoperative HO-1 expression and clinical outcomes, the clinical data of 137 recipients in TMA analysis were reviewed retrospectively. 137 patients were divided into two groups according to HO-1 expression levels (Table 2). There was no difference in terms of age, gender and ABO compatible between high and low HO-1 expression group. Notably, significantly higher Child-Pugh and Meld scores were observed in high HO-1 expression group (P<0.01).
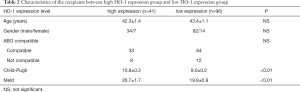
Full table
To further validate HO-1 expression level as a prognostic factor, overall survival rates were determined using the log-rank test with respect to HO-1 expression level. Kaplan-Meier survival curves based on HO-1 expression level showed that in a 5-year follow-up, high HO-1 expression group had significantly poorer overall survival rate compared with the low HO-1 expression group (P<0.05) (Figure 5).
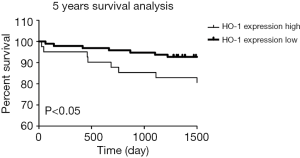
Moreover, multivariate analysis based on the Cox regression model was performed to confirm the pre-operative HO-1 expression as an independent prognostic factor. As showed in Table 3, Child-Pugh A was shown to be a protective prognostic factor while age >50 was an unfavourable one. Notably, HO-1 expression was significantly associated with overall survival of LT patients (OR 0.217, P=0.005), suggesting that high preoperative HO-1 expression may be used as an independent, unfavourable prognostic biomarker for predicting the development of post-transplant allograft ACR and recipient’s survival.
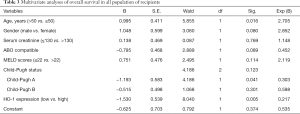
Full table
Discussion
In this study, we systematically compared and characterized the proteome differences of liver tissues between ACR and non-rejection group using iTRAQ-based comparative proteomics. Bioinformatics analysis successfully provided the distinguished molecular signature and critical signaling pathways during ACR. Further TMA analysis and retrospective cohort study showed that preoperative HO-1 expression was significantly higher in ACR patients than in non-rejection patients, which can independently predict the development of post-transplant ACR. HO-1 may serve as a potential biomarker for ACR prognosis.
The field of biomarker identification for liver rejection is an area of fast-growing interest in recent years. Many studies based on transcriptomics, proteomics and metabolomics have been performed in liver tissue, blood or urine to investigate the molecular signatures in rejection, aiming to find potential biomarkers (17-19). According to the previous studies, proteins related to inflammation are generally up-regulated in rejection graft due to the inflammatory response, while the down-regulated proteins are mainly associated with the disequilibrium of synthetic and metabolic homeostasis during rejection (20,21). As a result, the reported biomarkers are mainly pro-inflammatory and immunoregulatory cytokines, chemokines or other proteins related to inflammation, which showed an increased expression during ACR (21-24). Our differential proteomics study successfully filtered out 287 DEPs. These proteins, consistent with previously published studies, could obviously be divided into 2 distinct clusters: inflammation/immunoregulation-related proteins in up-regulated DEPs; functional proteins mainly involving in metabolic abnormalities in down-regulated DEPs (Table S2, Figure 2).
Further GO and pathway analyses systematically illustrated the visible differences of the molecular signatures between ACR and non-rejection group, reflecting the results of inflammatory reaction and functional abnormality in molecular level during ACR. In a proteomic study based on a CLAD rat model induced from ACR (9), Liu and colleagues found that the DEPs in blood were significantly enriched in B/T cell activation, Integrin signaling pathway, Chemokine signaling pathway, etc., presenting the high overlapping percentage of the enrich pathways with our study, indicating the crucial role of the activation of these pathways in inflammatory reaction during rejection. In another transcriptomic analysis conducted by Lozano et al. (25), the enriched pathways in differentially gene transcripts presented the similar pattern, showing enrichment of Integrin pathway, T/B-cell activation, etc. The high degree of similarity in functional pathway enrichment between different studies based on different strategies suggests the important roles of these pathways in the pathogenesis of ACR. Meanwhile, these studies confirm the reliability of our findings in the present work. However, the exact mechanisms underlying the activation of these pathways in ACR remain unclear.
Through module analysis, we identified three important functional modules, especially module 1 and module 2, which accounted for the major enriched pathways in DEPs. Notably, module 2 is relatively more activated, with overwhelming majority of proteins up-regulated, which is the exact opposite in module 1. Proteins in module 2, including NFKB1, MAPK1, MAPK14, RELA, HO-1, ICAM-1, VCAM-1, etc., conferring either pro- or anti-inflammatory effect in inflammation and immune response during ACR, have been reported to be potential biomarkers of liver rejection (18,21). The overexpression of these proteins suggests the excessive activation of the NF-κB and MAPK related inflammatory pathway. It may increase the expression of many inflammatory cytokines and chemokines in liver tissue or blood, which will be centrally involved in the activation of T cell immunity (26). Thus, preoperatively excessive activation of inflammatory pathways may exist in ACR patients and cause high levels of inflammatory cytokines in circulation, which may lead to allograft function abnormalities and post-transplantation rejection (27). For instance, the overexpression of ICAM-1 and VCAM-1 in serum or liver tissues, may facilitate the adhesion and extravasation of activated immune cells from the circulation into liver, thus magnifying the inflammation during ACR (18). Nonetheless, the exact mechanism underlying the up-regulation of these proteins at the point of ACR remains unknown and needs to be evaluated in further researches.
HO-1, the rate-limiting enzyme in heme catabolism, can catalyze heme to free iron, carbon monoxide (CO), and biliverdin (subsequently reduced to bilirubin by biliverdin reductase). Under various stressful stimuli like hypoxia, reactive oxygen species (ROS), and inflammation, HO-1 can be rapidly induced to confer cytoprotective functions through various biological processes, including antioxidant, maintaining microcirculation, preventing ischemia/reperfusion injury, anti-inflammation, immunoregulation, etc. (28). In LT setting, accumulating evidences have previously demonstrated up-regulated HO-1 expression during rejection, which may protect liver grafts against rejection and improve graft survival (29). In present study, HO-1’s critical role in PPI network suggested its importance in immunoregulation and liver metabolism during ACR. However, contrary to many aforementioned protective roles of HO-1 overexpression against rejection, our study showed relative overexpression of HO-1 in ACR subjects rather than non-rejection subjects and preoperatively exaggerated HO-1 expression significantly correlated with poorer 5-year overall survival. Though the exact mechanisms explaining this clinical observation remain unknown, we indeed found preoperative high HO-1 an independent adverse predictor for ACR instead of a protective one. More studies are needed to clarify this issue.
To our knowledge, this is the first study that confirms significant higher HO-1 expression in tissue level in ACR recipients compared to non-rejection recipients before transplantation. Similar observations have been made by several studies. Eisuke etc. observed significantly higher serum HO-1 levels in patients with more severe liver injury (30). In a study of 380 patients undergoing non-cardiac surgery, Zheng et al. reported preoperative serum HO-1 were significantly higher in patients with adverse cardiac events than in the controls, and higher preoperative HO-1 level was associated with the severity of postoperative adverse cardiac events (OR 1.30, P=0.002) (31). Contrary to our past views about HO-1’s protective roles, deliberately induced HO-1 overexpression may increase postoperative liver injury, as shown by aggravation of ALT level, cell necrosis and ferroptosis (32,33). In the donor setting, preoperative excessive HO-1 expression was also believed to be associated with postoperative rejection or early graft dysfunction. Using a lung transplantation model of rat, Bonnell et al. found that HO-1 expression levels progressively increased with time and with severity of ACR (34). In a prospective study, excessive preoperative HO-1 expression significantly correlated with post-transplant graft injury and poorer hepatobiliary function, which showed higher postoperative serum AST/ALT levels and worse bile excretion in high HO-1 expression recipients (35). Positive correlation between HO-1 expression and postoperative serum ALT levels seen in these studies suggested that HO-1 levels may partly reflect the severity of preoperative liver injury.
In this study, higher Child-Pugh scores and Meld scores before transplant were observed in recipients with high HO-1 expression (Table 2), indicating that patients in high HO-1 expression group presented poorer liver function. To be noted, better Child-Pugh status was found to be a favourable factor for LT prognosis in multivariate analyses (Table 3). There are studies reporting that worse MELD scores were associated postoperative graft dysfunction and poor prognosis (36,37). Therefore, we speculate that exaggerated HO-1 activity before transplant may reflect the excessive liver injuries in recipients, which can lead to more severe postoperative early graft dysfunction.
One explanation for the lack of graft protection seen with preoperative high HO-1 expression in our study is that apart from donor’s factor, pre-operative overall state of recipients can also provide important contributions to post-operative graft function. The recipients’ underlying illness and pre-operative condition may alter the levels of inflammatory and/or immunologically associated proteins in circulation. Preoperatively excessive activation of inflammatory pathways, as shown in our pathway analysis, may exist in ACR patients, causing high levels of inflammatory cytokines or immune injury-associated proteins in circulation (26,27), which, in return, may be detrimental to postoperative allograft function by aggravating inflammatory injuries in allograft and thus causing postoperative ACR. Theoretically, the more severe the pre-operative illness is, the more HO-1 may be induced to protect cells. Thus, it is quite reasonable to observe that pre-operative higher HO-1 expression was associated with higher rate of postoperative rejection and poorer survival.
Another possibility is that excessive HO-1 expression may aggravate the pre-operative illness instead of providing cytoprotection. Exaggerated HO-1 expression may sensitize cells to oxidative stress due to an accumulation of free iron, thereby resulting in a pro-oxidant condition and increasing postoperative oxidative injury (33,38). HO-1 overexpression may also aggravate the activation of macrophage, the main source of pro-inflammatory mediators in liver, thus increasing the releasing of ROS, TNF-α, TGF-β, etc. into circulation, which in return, may exacerbate postoperative immunoreaction and inflammation in the graft (39,40). However, we were unable to clarify the controversial effects of high HO-1 expression of either cytoprotection or increased cytotoxicity in grafts in this study. Further studies will be necessary to elucidate the clinical implications of HO-1 expression in detail.
In their serial studies, Nakamura etc. reported that post-transplant high HO-1 could regulate macrophage activation and sterile inflammation during I/R injury; and post- but not pre-transplant high HO-1 expression correlated with better hepatic function in human OLT (41,42). Notably, they also found that pre-transplant high and post-transplant low HO-1 expression trended with inferior overall survival (43). Post-transplant low macrophage HO-1 expression in human liver grafts correlated with a 40% reduced 3-year survival rates, indicating recipient HO-1 inducibility is essential for posttransplant hepatic HO-1 expression and its graft protective roles. Combining their results with our data, whether the combination of pre-transplant and post-transplant HO-1 expression will offer better predictive value deserves further researches.
There are several inherent limitations in this study. A limitation is the patients’ background. Different individual patients might have different genetic and pathological backgrounds. Though we matched the underlying diseases between ACR and non-rejection groups, the distinguished proteome profile and the HO-1 expression level between two groups may be affected by different individual background. Second, rejection is the result of the immune reactions between recipients and the donor liver. However, only the recipient’s HO-1 expression was examined and the influences of donor’s HO-1 expression were not investigated. In addition, whether combination of the recipient’s and donor’s HO-1 will offer better predictive value deserves further researches. The third limitation is the relatively small sample size, especially in training set. Though we included two cohorts in validating set to increase the sample volume and to guarantee the reliability of the research findings, HO-1’s predicting value needs to be confirmed in large multicenter prospective trials and further studies are also needed to investigate remaining potential biomarker candidates in DEPs list.
Conclusions
In summary, our study confirmed a list of 287 DEPs and systematically characterized the preoperative proteome differences in the episode of ACR, providing a set of potential targets for future investigation into the molecular mechanisms and biomarkers. Preoperative high HO-1 expression was validated in tissue level and was identified as a potential biomarker for predicting the development of post-transplant ACR and recipient’s survival. However, HO-1’s roles in ACR must be confirmed in larger cohort studies. Further studies could be performed to verify additional candidate biomarkers in the data set, which may eventually help develop more efficient diagnostic tools and treatment targets for ACR.
Supplementary
Protein preparation for iTRAQ analysis
Fresh liver tissues were collected from recipients’ explant liver intraoperatively and immediately preserved at −80 °C for further iTRAQ analysis. The fresh explant tissues were taken and fixed in 10% formalin for tissue microarray in validation set.
Liver tissues from three paired rejection and non-rejection recipients were respectively homogenized to powder in liquid nitrogen and then lysed with Lysis buffer A (7 M Urea, 2 M Thiourea, 4% CHAPS, 40 mM Tris-HCl, pH 8.5; 1mM PMSF and 2mM EDTA), followed by adding of 10 mM DTT 5 min later, sonication at 200 W for 15 min and then centrifugation at 4 °C, 30,000 ×g for 15 min. The supernatant was precipitated with chilled acetone (5× volume, containing 10% TCA) and incubated at −20 °C overnight. After centrifugation, the pellet was rinsed with chilled acetone three times and air dried. The pellet was dissolved in Lysis buffer B (7 M urea, 2 M thiourea, 4% NP40, 20 mM Tris-HCl, pH 8.0–8.5), sonicated at 200 W for 15 min and centrifuged at 4 °C, 30,000 ×g for 15 min. The supernatant was then transferred to the fresh tube, reduced using 10 mM DTT at 56 °C for 1 h, and alkylated with 55 mM IAM in the darkroom for 1 h. After being precipitated with chilled acetone (5× volume) at −20 °C for 2 h and centrifuged at 4 °C, 30,000 ×g for 15 min, the protein sample was air-dried and resuspended in 500 µL 0.5 M TEAB (Applied Biosystems, Milan, Italy), sonicated at 200 W for 15 min and centrifuged as describe above. Finally, the proteins in the supernatant were quantified using Bradford assay (Bio-Rad Laboratories, CA, USA) and stored at −80 °C for further analysis.
iTRAQ labeling and SCX fractionation
Pooled proteins (100 µg) from each sample solution were digested overnight with Trypsin Gold (Promega, Madison, WI, USA) with the ratio of trypsin: protein 1:30 at 37 °C, followed by a vacuum centrifugation for drying. Next, peptides were reconstituted in 0.5 M TEAB and processed with the iTRAQ-8plex kit (Applied Biosystems SCIEX, USA) following the manufacture’s protocol. Briefly, samples were labeled with the iTRAQ tags as follows: the rejection group samples were labeled with 118, 119 and 121 iTRAQ tags respectively, and the non-rejection group samples were labeled with 113, 115 and 117 iTRAQ tags (Figure 1). After incubation at room temperature for 2 h, the labeled samples were pooled together, dried by vacuum centrifugation, and subjected to fractionation. To avoid the labeling bias, two independent biological replicates were used and three independent replicate experiments were performed.
Strong cation exchange (SCX) chromatography was performed to clean and fractionate the labeled samples using LC-20AB HPLC Pump system (Shimadzu, Kyoto, Japan). Briefly, the iTRAQ-labeled peptide mixtures were reconstituted in 4 mL buffer A (25 mM NaH2PO4 in 25% ACN, pH 2.7) and then loaded onto a 4.6 mm × 250 mm Ultremex SCX column with 5 µm particles (Phenomenex). The peptides were eluted according to following procedures: flow rate 1 mL/min; a gradient of buffer A for 10 min, 5–60% buffer B (25 mM NaH2PO4, 1 M KCl in 25% ACN, pH 2.7) for 27 min, 60–100% buffer B for 1 min and maintenance at 100% for 1 min, equilibrium with buffer A for 10 min; monitoring the absorbance at 214 nm; fractions collected every 1 min. The eluted peptides were pooled into 20 fractions and desalted with a Strata X C18 column (Phenomenex) before vacuum-drying.
High-performance liquid chromatography-tandem mass spectrometry (HP LC-MS/MS)
The SCX fractions were first separated by nanoHPLC and then analyzed by tandem mass spectrometry (MS/MS). Briefly, each fraction was re-dissolved in buffer A (5% ACN, 0.1% FA), followed by being centrifuged at 20,000 ×g for 10 min. Using a LC-20AD nanoHPLC (Shimadzu, Kyoto, Japan), 10 µL of peptide sample was loaded onto a 2 cm C18 trap column with a flow rate of 8 µL/min for 3 min and was then gradiently eluted by buffer B (95% ACN, 0.1% FA) on a 10 cm analytical C18 column (inner diameter 75 µm) packed in-house with the following procedures: flow rate 0.3 µL/min, 5% buffer B for 1 min, a gradient from 5% to 35% buffer B for 35 min, a 5 min linear gradient to 60%, a 2 min linear gradient to 80% and maintenance for 4 min. Finally, the system returned to 5% buffer B in 1 min and was maintained at 5% for 10 min.
The LC eluted peptides were analyzed by TripleTOF 5600 System (AB SCIEX, Concord, ON) fitted with a Nanospray III source (AB SCIEX, Concord, ON) and a SilicaTip emitter (New Objectives, Woburn, MA). The key parameters of data acquisition included: ion spray voltage 2.5 kV, curtain gas 30psi, nebulizer gas 15psi, interface heater temperature 150 °C; resolving power (R.P.) of TOF MS scans: ≥ 30 kF WHM; information-dependent acquisition (IDA): survey scans 250 ms, charge state from 2+ to 5+, up to 30 MS/MS spectra acquired per cycle, minimum threshold 120 counts/s, total cycle time: fixed to 3.3 s; Q2 transmission 100 Da for 100%; four time bins summed for each scan, pulse frequency value 11 kHz, monitoring of the 40 GHz multichannel TDC detector with four-anode channel detect ion; collision-induced dissociation setting: sweeping collision energy 35±5 eV, iTRAQ adjust rolling collision energy for all precursor ions; dynamic exclusion: 1/2 of peak width (15 s).
Data analysis
Raw data files obtained from the iTRAQ were converted into MGF format files using Proteome Discoverer 1.2 (PD 1.2, Thermo). Mascot software (version 2.3.02, Matrix Science Inc., MA, USA) was used for proteins identification by searching against UniProt/SwissProt Homo sapiens database (2019_06, with 20,431 reviewed entries), with the false discovery rate (FDR) <1%. The key search parameters were set as follows: (I) type of search-MS/MS Ion search, charge states of peptides: +2 and +3; (II) the enzyme specificity of trypsin; (III) max missed cleavages: 1; (IV) the parent ion mass tolerance: 10 ppm, fragment ion mass tolerance: 0.5 Da; (V) the potential variable modifications: Gln > pyro-Glu (N-term Q), oxidation (M), deamidated (NQ); (VI) fixed modifications: carbamidomethyl (C), iTRAQ8plex (N-term), iTRAQ8plex (K). To reduce the probability of false peptide identification, only peptides with significance scores (≥20), FDR <1% and protein probability >99.0% were accepted. Each identified confident protein included at least one unique peptide. For protein quantitation, the Student’s t-test was used for statistical analysis and the relative quantitation of a given protein was reported as the median ratio in Mascot. P<0.05 was considered statistically significant.
Immunohistochemical (IHC) staining for tissue microarray
Tissue microarray sections were immunohistochemically stained for HO-1 to evaluate its expression level. In brief, TMA slides were deparaffinized in xylene and rehydrated in graded alcohols for 5 min, and then immersed in citrate buffer (pH 6.1) at 95 °C for antigen retrieval for 40 min. The slides were blocked for endogenous peroxidase activity in 0.3% hydrogen peroxide for 20 min. After washing and incubation overnight at 4 °C with primary antibodies against HO-1 (1:600 dilution, Abcam, USA), the slides were incubated with rabbit anti-HO-1 at room temperature for 30 min. Following several washing steps, the slides were immersed for 10 minutes using diaminobenzidine as the chromogen and then counterstained with hematoxylin for 5 minutes. The slides were scanned and visualized using the Aperio ScanScope XT (Aperio) to generate high-resolution digital images for evaluating the stained data.
HO-1 expression levels were evaluated semiquantitatively by the staining intensity combined with the percent of positively stained cells. The staining intensity of HO-1 (intensity scores) was scored as: 0 (negative); 1 (weak); 2 (moderate), and 3 (strong). The percentages of positive cells per field (percent positivity scores) were scored as: 1 (<25%), 2 (25% to 50%), 3 (50% to 75%), 4 (>75%). The overall HO-1 expression score was calculated according to the following formula: intensity score × percent positivity score, which ranged from 0 to 12. Therefore, HO-1 expression can be defined as low expression (HO-1 expression score <8) and high expression (HO-1 expression score ≥8).
Two pathologists, blinded to this study, independently evaluated and scored each case. Differences in interpretation were settled by the consensus.
Acknowledgments
Funding: This work was supported by Foundation for Innovative Research Groups of the National Natural Science Foundation of China (No. 81421062), National Natural Science Foundation of China (No. 81470891/81373160), Special Grant from China Postdoctoral Science Foundation (2019T120525), China Postdoctoral Science Foundation (2017M610374), Zhejiang Health Technology Project (2019RC153), and Fundamental Research Funds for the Central Universities (2018FZA7003). The funders had no role in study design, data collection and analysis, decision to publish, or preparation of the manuscript.
Footnote
Conflicts of Interest: The authors have no conflicts of interest to declare.
Ethical Statement: The authors are accountable for all aspects of the work in ensuring that questions related to the accuracy or integrity of any part of the work are appropriately investigated and resolved. The study protocol was approved by the ethics committee review board of the First Affiliated Hospital, Zhejiang University. Informed consent was obtained from all patients for surgical treatment.
Open Access Statement: This is an Open Access article distributed in accordance with the Creative Commons Attribution-NonCommercial-NoDerivs 4.0 International License (CC BY-NC-ND 4.0), which permits the non-commercial replication and distribution of the article with the strict proviso that no changes or edits are made and the original work is properly cited (including links to both the formal publication through the relevant DOI and the license). See: https://creativecommons.org/licenses/by-nc-nd/4.0/.
References
- Adams DH, Sanchez-Fueyo A, Samuel D. From immunosuppression to tolerance. J Hepatol 2015;62:S170-85. [Crossref] [PubMed]
- Sapisochin G, Bruix J. Liver transplantation for hepatocellular carcinoma: outcomes and novel surgical approaches. Nat Rev Gastroenterol Hepatol 2017;14:203-17. [Crossref] [PubMed]
- Emond JC, Griesemer AD. Tolerance in clinical liver transplantation: The long road ahead. Hepatology 2017;65:411-3. [Crossref] [PubMed]
- Demetris AJ, Bellamy C, Hubscher SG, et al. 2016 Comprehensive Update of the Banff Working Group on Liver Allograft Pathology: Introduction of Antibody-Mediated Rejection. Am J Transplant 2016;16:2816-35. [Crossref] [PubMed]
- Xu M, Tan C, Hu J, et al. Expression of hemopexin in acute rejection of rat liver allograft identified by serum proteomic analysis. Shock 2014;42:65-74. [Crossref] [PubMed]
- Wiese S, Reidegeld KA, Meyer HE, et al. Protein labeling by iTRAQ: a new tool for quantitative mass spectrometry in proteome research. Proteomics 2007;7:340-50. [Crossref] [PubMed]
- Qian Y, Yao W, Yang T, et al. aPKC-iota/P-Sp1/Snail signaling induces epithelial-mesenchymal transition and immunosuppression in cholangiocarcinoma. Hepatology 2017;66:1165-82. [Crossref] [PubMed]
- Wong YK, Zhang J, Hua ZC, et al. Recent advances in quantitative and chemical proteomics for autophagy studies. Autophagy 2017;13:1472-86. [Crossref] [PubMed]
- Li J, Liu B, Shi Y, et al. CXCL4 Contributes to the Pathogenesis of Chronic Liver Allograft Dysfunction. J Immunol Res 2016;2016:9276986. [Crossref] [PubMed]
- Haas M, Sis B, Racusen L, et al. Banff 2013 meeting report: Inclusion of c4d-negative antibody-mediated rejection and antibody-associated arterial lesions. Am J Transplant 2014;14:272-83. [Crossref] [PubMed]
- Shen T, Lin BY, Jia JJ, et al. A modified protocol with rituximab and intravenous immunoglobulin in emergent ABO-incompatible liver transplantation for acute liver failure. Hepatobiliary Pancreat Dis Int 2014;13:395-401. [Crossref] [PubMed]
- Consortium GO. Gene ontology consortium: going forward. Nucleic Acids Res 2015;43:D1049-56. [Crossref] [PubMed]
- Mi H, Huang X, Muruganujan A, et al. PANTHER version 11: expanded annotation data from Gene Ontology and Reactome pathways, and data analysis tool enhancements. Nucleic Acids Res 2017;45:D183-9. [Crossref] [PubMed]
- Szklarczyk D, Morris JH, Cook H, et al. The STRING database in 2017: quality-controlled protein–protein association networks, made broadly accessible. Nucleic Acids Res 2017;45:D362-8. [Crossref] [PubMed]
- Bader GD, Hogue CW. An automated method for finding molecular complexes in large protein interaction networks. BMC bioinformatics 2003;4:2. [Crossref] [PubMed]
- Saito R, Smoot ME, Ono K, et al. A travel guide to Cytoscape plugins. Nat Methods 2012;9:1069-76. [Crossref] [PubMed]
- Sarwal MM. Fingerprints of transplant tolerance suggest opportunities for immunosuppression minimization. Clin Biochem 2016;49:404-10. [Crossref] [PubMed]
- Germani G, Rodriguez-Castro K, Russo FP, et al. Markers of acute rejection and graft acceptance in liver transplantation. World J Gastroenterol 2015;21:1061. [Crossref] [PubMed]
- Baroja-Mazo A, Revilla-Nuin B, Parrilla P, et al. Tolerance in liver transplantation: Biomarkers and clinical relevance. World J Gastroenterol 2016;22:7676. [Crossref] [PubMed]
- Brunet M, Shipkova M, van Gelder T, et al. Barcelona consensus on biomarker-based immunosuppressive drugs management in solid organ transplantation. Ther Drug Monit 2016;38:S1-S20. [Crossref] [PubMed]
- Verhelst XP, Troisi RI, Colle I, et al. Biomarkers for the diagnosis of acute cellular rejection in liver transplant recipients: A review. Hepatol Res 2013;43:165-78. [Crossref] [PubMed]
- Okubo K, Wada H, Tanaka A, et al. Identification of Novel and Noninvasive Biomarkers of Acute Cellular Rejection After Liver Transplantation by Protein Microarray. Transplant Direct 2016;2:e118. [Crossref] [PubMed]
- Bonaccorsi-Riani E, Pennycuick A, Londoño MC, et al. Molecular characterization of acute cellular rejection occurring during intentional immunosuppression withdrawal in liver transplantation. Am J Transplant 2016;16:484-96. [Crossref] [PubMed]
- Massoud O, Heimbach J, Viker K, et al. Noninvasive diagnosis of acute cellular rejection in liver transplant recipients: A proteomic signature validated by enzyme-linked immunosorbent assay. Liver Transpl 2011;17:723-32. [Crossref] [PubMed]
- Lozano JJ, Pallier A, Martinez-Llordella M, et al. Comparison of Transcriptional and Blood Cell‐Phenotypic Markers Between Operationally Tolerant Liver and Kidney Recipients. Am J Transplant 2011;11:1916-26. [Crossref] [PubMed]
- Sun B, Karin M. NF-κB signaling, liver disease and hepatoprotective agents. Oncogene 2008;27:6228-44. [Crossref] [PubMed]
- Friedman BH, Wolf JH, Wang L, et al. Serum cytokine profiles associated with early allograft dysfunction in patients undergoing liver transplantation. Liver Transpl 2012;18:166-76. [Crossref] [PubMed]
- Zhang M, Nakamura K, Kageyama S, et al. Myeloid HO-1 modulates macrophage polarization and protects against ischemia-reperfusion injury. JCI Insight 2018. [Crossref] [PubMed]
- Ryter SW, Choi AM. Targeting heme oxygenase-1 and carbon monoxide for therapeutic modulation of inflammation. Transl Res 2016;167:7-34. [Crossref] [PubMed]
- Ozawa E, Abiru S, Nagaoka S, et al. Ferritin/alanine aminotransferase ratio as a possible marker for predicting the prognosis of acute liver injury. J Gastroenterol Hepatol 2011;26:1326-32. [Crossref] [PubMed]
- Zheng H, Ma HP, Wang J, et al. Preoperative HO-1 levels as prognostic factor for adverse cardiac events in elder patients undergoing non-cardiac surgery. PLoS One 2013;8:e58567. [Crossref] [PubMed]
- Froh M, Conzelmann L, Walbrun P, et al. Heme oxygenase-1 overexpression increases liver injury after bile duct ligation in rats. World J Gastroenterol 2007;13:3478. [Crossref] [PubMed]
- Fang X, Wang H. Ferroptosis as a target for protection against cardiomyopathy. Proc Natl Acad Sci U S A 2019;116:2672-80. [Crossref] [PubMed]
- Bonnell MR, Visner GA, Zander DS, et al. Heme-oxygenase-1 expression correlates with severity of acute cellular rejection in lung transplantation. J Am Coll Surg 2004;198:945-52. [Crossref] [PubMed]
- Geuken E, Buis CI, Visser DS, et al. Expression of Heme Oxygenase‐1 in Human Livers Before Transplantation Correlates with Graft Injury and Function After Transplantation. Am J Transplant 2005;5:1875-85. [Crossref] [PubMed]
- Roth JA, Chrobak C, Schädelin S, et al. MELD score as a predictor of mortality, length of hospital stay, and disease burden: A single-center retrospective study in 39,323 inpatients. Medicine 2017;96:e7155. [Crossref] [PubMed]
- Bruns H, Lozanovski VJ, Schultze D, et al. Prediction of postoperative mortality in liver transplantation in the era of MELD-based liver allocation: a multivariate analysis. PLoS One 2014;9:e98782. [Crossref] [PubMed]
- Drummond GS, Baum J, Greenberg M, et al. HO-1 overexpression and underexpression: Clinical implications. Arch Biochem Biophys 2019;673:108073. [Crossref] [PubMed]
- Fahrner R, Dondorf F, Ardelt M, et al. Role of NK, NKT cells and macrophages in liver transplantation. World J Gastroenterol 2016;22:6135. [Crossref] [PubMed]
- Vijayan V, Wagener F, Immenschuh S. The macrophage heme-heme oxygenase-1 system and its role in inflammation. Biochem Pharmacol 2018;153:159-67. [Crossref] [PubMed]
- Nakamura K, Kageyama S, Yue S, et al. Heme oxygenase-1 regulates sirtuin-1-autophagy pathway in liver transplantation: From mouse to human. Am J Transplant 2018;18:1110-21. [Crossref] [PubMed]
- Nakamura K, Zhang M, Kageyama S, et al. Macrophage heme oxygenase-1-SIRT1-p53 axis regulates sterile inflammation in liver ischemia-reperfusion injury. J Hepatol 2017;67:1232-42. [Crossref] [PubMed]
- Kageyama S, Hirao H, Nakamura K, et al. Recipient HO-1 inducibility is essential for posttransplant hepatic HO-1 expression and graft protection: From bench-to-bedside. Am J Transplant 2019;19:356-67. [Crossref] [PubMed]