The influence of tumour mutational burden on renal cancer immune infiltration and survival
In a study of patients with clear cell renal carcinoma from the Cancer Genome Atlas (TCGA) database, Zhang et al. evaluated whether tumour mutational burden (TMB) was associated with reduced patient survival and increased immune infiltrates (1).
The importance of immunity in cancer has been recognised as far back as circa 1,550 BC (2). In the 19th century, Coley produced the first immunological cancer therapy by injecting sarcoma patients with heat killed S. pyogenes and S. marcecsens, and this led to remission in some patients (3). More recent work has elucidated a mechanism for this phenomenon, namely that pathogen-associated molecular patterns can elicit an anti-tumour response through shared pattern recognition receptors (4). It may be possible to treat cancer by promoting pattern recognition receptor signalling, and use checkpoint inhibitors to prevent ‘exhaustion’ of the immune system (5,6). Indeed, a recent systematic review of 25 randomised control trials demonstrated that checkpoint inhibition conferred longest overall survival for patients with metastatic renal cell carcinoma (RCC) (7). These newly developed checkpoint inhibitors are now a first line agent in the treatment of RCC (8), and activation of the immune system plays a key role in the treatment of renal cancer.
Optimal management of renal cancer is important for a number of reasons. It is one of the most common cancers with 400,000 individuals diagnosed globally each year (9). The incidence of RCC appears to be increasing, possibly due to increased incidental detection (10), and 17% of patients have metastatic disease at diagnosis (11). While treatment has improved, 175,000 patients die of renal cancer annually (9).
Clear cell RCC (ccRCC) is the most common subtype of RCC, and one of its distinctive features is immune infiltration (12,13). In other cancers, immune cell infiltration has been linked to prognosis and treatment efficacy (14). Kamal et al. demonstrated that primary metastatic ccRCC had a higher level of immune infiltration when compared to non-metastatic ccRCC (15). These types of analysis are confounded by the differing roles of the wide variety of immune cells present in the micro-environment, be they pro- or anti- tumourigenic. Şenbabaoğlu et al. analysed ccRCC immune infiltrates, transcriptomic and proteomic profiles and found infiltration with Th17 and CD8+ T cells improved survival, whereas infiltration with Th2 and regulatory T cells worsened survival (16). Similarly, McDermott et al. demonstrated that in metastatic RCC effector T cell and myeloid inflammatory gene expression was associated with survival and treatment response (17).
Intuitively, one might expect a strong correlation between high levels of cytotoxic T cells levels and the number of neo-antigens expressed by tumour cells. The easiest measurable surrogate for neo-antigen presentation is TMB. However, neo-antigen presentation not only depends on the number, but also the type of somatic mutations acquired by the tumour. Compared to other solid cancers, ccRCC has a low mutational burden (18), occurring at a rate of 1–2 per Mb, whereas for instance melanoma and non-small cell lung cancer have around 10–400 per Mb (18).
However, ccRCC have relatively high prevalence of indels compared to single nucleotide variants, which may produce a greater array of neo-antigens, possibly explaining the relatively high sensitivity to immune-checkpoint inhibition (19).
TMB has been shown to affect treatment efficacy as well as survival in other cancers (20,21). Samstein et al. demonstrated that TMB was associated with progression free survival after checkpoint inhibitor treatment (20). These findings were not however corroborated by McDermott (17), nor has TMB previously been found to be associated with the level of immune infiltration in RCC (16).
Immune infiltration can also be estimated using a number of histological processes such as immuno-histochemistry. More recently, modern computational histopathology methods have been demonstrated to estimate tumour micro-environment composition from haematoxylin and eosin stained tissue slides without the need for additional tissue processing. Deep transfer learning, where a computer is taught how to do one task and then uses that knowledge to complete a different but related task, was used by Fu et al. to accurately identify different cancer types and normal tissue. The authors were then able to use these learned histopathogical features to predict whole genome duplications, amplifications and deletions, and even driver gene mutations through deep transfer learning (22).
In this journal, Zhang et al. analysed 336 patients with ccRCC from TCGA. They estimated the TMB for each patient and categorised their cohort into lower low-TMB, and high-TMB samples. Using this stratification, they demonstrated that high-TMB was significantly associated with poorer survival, as well as higher tumour stage and grade. Although TMB was not associated with higher T, N, M stage (1).
They determined that nine genes were associated with low- and high-TMB and found that high-TMB was associated with MAPK and Wnt signalling pathways (1).
The authors then used the CIBERSORT algorithm to determine the immune profiles of samples. They demonstrated that lymphoid and myeloid immune infiltrates were lower in the high-TMB group compared to the low-TMB group. Additionally, they identified that low CD8+ T cell and macrophage infiltrates were negatively associated with survival (1).
There are some doubts as to the widespread applicability of the conclusions from this study as we know that tumour mutational burden is both correlated with age (18), and anti-correlated with immune infiltration (23). In clonal tumours without stromal contamination we observe that we can relatively accurately estimate the true tumour mutational burden if the average sequencing coverage is 30× or higher (Figure 1A). However, with higher levels of stromal or immune infiltration the effective coverage drops, and with it the estimated TMB, even if the tumour is sequenced at 60× (Figure 1B). Without accounting for patient age and effective coverage in this data it becomes difficult to infer the biological relationship between TMB and outcome. This relationship may explain why the association between TMB with survival in ccRCC unclear.
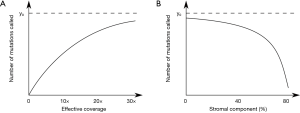
We note that using algorithms such as CIBERSORT to estimate the relative abundance of immune cells based on reference immune datasets could be improved in future studies. Many such cell state estimating algorithms force a fit to the reference data and do not account for a transcriptional profile that is not provided by the reference set. We suggest that in future studies, either a reference of all the cell types expected in RCCs is used (24), or an algorithm that can account for cell types not provided in the reference, to avoid overinflating the presence of some cell types.
Large scale genomic resources such as TCGA provide a vast amount of information for many cancer types. For most cases a wide variety of data is provided. They can be used to provide data on whole exome sequences which can then be used to identify mutations; single nucleotide polymorphisms for copy numbers; and RNA sequencing for gene expression (25). This data can also be used to identify DNA methylation, protein expression, and non-coding mutations. Using this data can help influence precision medicine as genetic signatures associated with survival and response to treatment can inform management choices (25).
It is time that all cancer sequencing studies regardless of sponsorship are made publicly available in repositories such as TCGA, particularly those studies that measure the response of tumours to different therapies. Only by sharing and making such data publically available can we ensure that the research that comes out of them has the highest impact for the patients who have donated their tissue and others that follow them.
Acknowledgments
Funding: TJ Mitchell is supported by Cancer Research UK and the Royal College of Surgeons (C63474/A27176).
Footnote
Conflicts of Interest: The authors have no conflicts of interest to declare.
Ethical Statement: The authors are accountable for all aspects of the work in ensuring that questions related to the accuracy or integrity of any part of the work are appropriately investigated and resolved.
Open Access Statement: This is an Open Access article distributed in accordance with the Creative Commons Attribution-NonCommercial-NoDerivs 4.0 International License (CC BY-NC-ND 4.0), which permits the non-commercial replication and distribution of the article with the strict proviso that no changes or edits are made and the original work is properly cited (including links to both the formal publication through the relevant DOI and the license). See: https://creativecommons.org/licenses/by-nc-nd/4.0/.
References
- Zhang C, Li Z, Qi F, et al. Exploration of the relationships between tumor mutation burden with immune infiltrates in clear cell renal cell carcinoma. Ann Transl Med 2019;7:648. [Crossref] [PubMed]
- Ebbell B. The Papyrus Ebers: the greatest Egyptian medical document. London: Oxford University Press, 1937.
- Decker WK, da Silva RF, Sanabria MH, et al. Cancer immunotherapy: Historical perspective of a clinical revolution and emerging preclinical animal models. Front Immunol 2017;8:829. [Crossref] [PubMed]
- Amarante-Mendes GP, Adjemian S, Branco LM, et al. Pattern recognition receptors and the host cell death molecular machinery. Front Immunol 2018;9:2379. [Crossref] [PubMed]
- Hernandez C, Huebener P, Schwabe RF. Damage-associated molecular patterns in cancer: A double-edged sword. Oncogene 2016;35:5931-41. [Crossref] [PubMed]
- Patel SA, Minn AJ. Combination Cancer Therapy with Immune Checkpoint Blockade: Mechanisms and Strategies. Immunity 2018;48:417-33. [Crossref] [PubMed]
- Wang J, Li X, Wu X, et al. Role of immune checkpoint inhibitor-based therapies for metastatic renal cell carcinoma in the first-line setting: A Bayesian network analysis. EBioMedicine 2019;47:78-88. [Crossref] [PubMed]
- Powles T, Albiges L, Staehler M, et al. Updated European Association of Urology Guidelines Recommendations for the Treatment of First-line Metastatic Clear Cell Renal Cancer. Eur Urol 2018;73:311-5. [Crossref] [PubMed]
- Bray F, Ferlay J, Soerjomataram I, et al. Global cancer statistics 2018: GLOBOCAN estimates of incidence and mortality worldwide for 36 cancers in 185 countries. CA Cancer J Clin 2018;68:394-424. [Crossref] [PubMed]
- Capitanio U, Bensalah K, Bex A, et al. Epidemiology of Renal Cell Carcinoma. Eur Urol 2019;75:74-84. [Crossref] [PubMed]
- Capitanio U, Montorsi F. Renal cancer. Lancet 2016;387:894-906. [Crossref] [PubMed]
- Escudier B, Porta C, Schmidinger M, et al. Renal cell carcinoma: ESMO clinical practice guidelines for diagnosis, treatment and follow-up. Ann Oncol 2014;25:iii49-56. [Crossref] [PubMed]
- Wang QJ, Hanada KI, Robbins PF, et al. Distinctive features of the differentiated phenotype and infiltration of tumor-reactive lymphocytes in clear cell renal cell carcinoma. Cancer Res 2012;72:6119-29. [Crossref] [PubMed]
- Gajewski TF, Schreiber H, Fu YX. Innate and adaptive immune cells in the tumor microenvironment. Nat Immunol 2013;14:1014-22. [Crossref] [PubMed]
- Kamal Y, Cheng C, Frost HR, et al. Predictors of disease aggressiveness influence outcome from immunotherapy treatment in renal clear cell carcinoma. Oncoimmunology 2018;8:e1500106. [Crossref] [PubMed]
- Şenbabaoğlu Y, Gejman RS, Winer AG, et al. Tumor immune microenvironment characterization in clear cell renal cell carcinoma identifies prognostic and immunotherapeutically relevant messenger RNA signatures. Genome Biol 2016;17:231. [Crossref] [PubMed]
- McDermott DF, Huseni MA, Atkins MB, et al. Clinical activity and molecular correlates of response to atezolizumab alone or in combination with bevacizumab versus sunitinib in renal cell carcinoma. Nat Med 2018;24:749-57. [Crossref] [PubMed]
- Alexandrov LB, Jones PH, Wedge DC, et al. Clock-like mutational processes in human somatic cells. Nat Genet 2015;47:1402-7. [Crossref] [PubMed]
- Turajlic S, Litchfield K, Xu H, et al. Insertion-and-deletion-derived tumour-specific neoantigens and the immunogenic phenotype: a pan-cancer analysis. Lancet Oncol 2017;18:1009-21. [Crossref] [PubMed]
- Samstein RM, Lee C-H, Shoushtari AN, et al. Tumor mutational load predicts survival after immunotherapy across multiple cancer types. Nat Genet 2019;51:202-6. [Crossref] [PubMed]
- Snyder A, Makarov V, Merghoub T, et al. Genetic basis for clinical response to CTLA-4 blockade in melanoma. N Engl J Med 2014;371:2189-99. [Crossref] [PubMed]
- Fu Y, Jung AW, Torne RV, et al. Pan-cancer computational histopathology reveals mutations, tumor composition and prognosis. bioRxiv 2019. [Crossref]
- Wang X, Li M. Correlate tumor mutation burden with immune signatures in human cancers. BMC Immunol 2019;20:4. [Crossref] [PubMed]
- Young MD, Mitchell TJ, Vieira Braga FA, et al. Single-cell transcriptomes from human kidneys reveal the cellular identity of renal tumors. Science 2018;361:594-9. [Crossref] [PubMed]
- Creighton CJ. The clinical applications of The Cancer Genome Atlas project for bladder cancer. Expert Rev Anticancer Ther 2018;18:973-80. [Crossref] [PubMed]