Serum and urine metabolomics reveal potential biomarkers of T2DM patients with nephropathy
Introduction
According to the International Diabetes Federation in 2017, 114 million (10.9%) Chinese have been diagnosed with diabetes in the last 20 years, and by 2045, the number is expected to be 119 million (11.6%) (1). The incidence and prevalence of diabetes have increased markedly, especially for type 2 diabetes mellitus (T2DM). A vast number of people with T2DM have progressed toward different complications in recent decades. Among them, the microvascular complications include diabetic nephropathy (DN), diabetic peripheral neuropathy (DPN) and diabetic retinopathy (DR), while the macrovascular complications include cardiovascular diseases (CVDs), stroke and peripheral vascular diseases, such as diabetic foot disease (DFD) (2). Chronic kidney disease (CKD) is a worldwide public health challenge and was first described as a advancing disease that begins with a small amount of albumin in the urine, accompanied with a decrease in kidney function, renal injury, and finally end-stage renal disease (ESRD) (3). CKD is diagnosed by the persistently increased urinary albumin excretion, low estimated glomerular filtration rate (eGFR), or additional characteristics of renal injury (4,5). It has been reported that DN has become the main cause of CKD in China (6). The rapidly elevating prevalence of diabetes global actually assures that the proportion of CKD due to diabetes, will continue to increase, so called diabetic kidney disease (DKD), also known as DN (7). According to World Health Organization data, about 25–40% of T2DM patients develop renal injury and CKD, which significantly worsens the quality of life of patients (8). Once disease progresses, about 20% to 40% of patients irreversibly would develop ESRD (9).
DKD is one of the usual complications of T2DM and is the major cause of ESRD, CVDs and death, but it is difficult to determine which T2DM patients have a higher risk of DKD based solely on glomerular filtration rate and proteinuria. Therefore, there is an urgent need to find biomarkers that can identify high-risk populations of DKD early in diabetes, through which we can predict the disease and prevent the progression of the disease at the early stage.
Metabolomics, a rapidly developing field in systems biology, measures metabolic changes in response to the development and progress of the disease (10,11). It has been widely used to detect metabolic profiles of diabetes-related diseases and to identify potential biomarkers (12). Rossi et al. (13) used liquid chromatography coupled to tandem mass spectrometry (LC-MS/MS) to measure whole blood from streptozotocin-induced DN with tissue inhibitors of metalloproteinase 3-deficient mice, and reported that the abnormal fatty acid metabolism and the related elevations in serum acyl-carnitines (ACs) were associated with the pathogenic process of DN. Li et al. (14) used gas chromatograph coupled with time-of-flight mass spectrometry (GC-TOFMS) to determine the urinary metabolites acquired from DN patients and showed that reduced level of mitochondrial metabolites were closely related to the occurrence of albuminuria. Our former study showed that serum differential metabolites, such as carbohydrates, fatty acids, amino acids, bile acids, lipids, steroids, and tricarboxylic acids, were involved in the formation of T2DM complications according to a 292-patient cohort study using untargeted metabolomics (15). We also recently summarized many different metabolomics studies on diabetic complications (16). As far as we known, no large-scale metabolomics research has characterized serum and urine metabolites systemically, especially in DN.
In this research, we performed serum and urine metabolic profiling between T2DM patients with or without nephropathy using GC-TOFMS. We also analyzed the characteristics of serum and urine metabolites in patients at different stages of DN to investigate the association between serum and urine metabolites and during the development of DN. The purpose of this research was to probe the metabolite changes at different stages of DN, to define the metabolites that are potentially predictive markers for DN.
Methods
Chemicals and reagents
Methoxyamine HCl, fatty acid methyl ester (C7-C30, FAMEs) standards, pyridine and anhydrous sodium sulfate were purchased from Sigma-Aldrich (St. Louis, MO, USA). N-methyl-N-trimethylsilyl trifluoroacetamide (MSTFA) with 1% (vol/vol) trimethylchlorosilane (TMCS), acetonitrile, methanol, dichloromethane, hexane, chloroform and acetone were obtained from Thermo-Fisher Scientific (FairLawn, NJ, USA). Ultrapure water was produced by a Mill-Q Reference system equipped with an LCMS Pak filter (Millipore, Billerica, MA).
Study population
From March 2015 to March 2016, T2DM patients were collected from the endocrinology clinics of Shuguang Hospital, Putuo Hospital and Shanghai Traditional Chinese Medicine Hospital affiliated to Shanghai University of Traditional Chinese Medicine. The 2010 diagnostic criteria from the American Diabetes Association (ADA) were used: (I) glycosylated hemoglobin (HbA1c) ≥6.5%, (II) fasting plasma glucose (FPG) ≥7.0 mmol/L (fasting is defined as at least 8 hours without calorie); (III) 2 hours of blood glucose during oral glucose tolerance analysis ≥11.1 mmol/L, and (IV) in a typical hyperglycemia or hyperglycemia crisis patients, random blood glucose ≥11.1 mmol/L (17). DN was diagnosed as diabetes with proteinuria (the value ratio of urinary albumin to creatinine ≥30 mg/g), damaged glomerular filtration rate (GFR) [<60 mL/min/1.73 m2 evaluated using the chronic kidney disease epidemiology collaboration (CKD-EPI) equation], or both (18). For women with serum creatinine ≤0.7, GFR was calculated as 144× (serum creatinine/0.7)−0.329×(0.993)age; for those who >0.7, GFR was computed as 144× (serum creatinine/0.7)−1.209 ×(0.993)age; for men with serum creatinine ≤0.9, GFR was computed as 141× (serum creatinine/0.9)−0.411×(0.993)age; and for those who >0.9, GFR was computed as 141× (serum creatinine/0.9)−1.209×(0.993)age (19). Renal biopsy is the gold standard for distinguishing DN and nondiabetic nephropathy, but renal biopsy is an invasive test and the patient’s acceptance is low (20,21). The exclusion criteria were as follows: (I) primary kidney disease, this experiment is based on the diagnosis of diabetes, proteinuria, diabetic retinopathy, hematuria, and systolic blood pressure. (II) urinary tract infection, (III) recent acute disease, (IV) malignant tumor, (V) taking drugs affecting bone metabolism in the past six months, and (VI) primary osteoporosis and other endocrine diseases, autoimmune diseases, or similar conditions. Those who were difficult to accurately identify kidney disease was also excluded in this study. Additionally, hypertensive patients were excluded based on their medical histories and measured blood pressure. The information about weight, height, waist-to-hip ratio (WHR) and body mass index (BMI) was collected. All patients who participated in the study offered a signed informed consent form. The ethical approval for the present study was provided by the Ethics Committee of Shanghai University of Traditional Chinese Medicine.
GC-TOFMS analysis
All serum and urine samples were dissolved on ice and centrifuged at 4 °C and 3,000 g for 5 min (Microfuge 20R, Beckman Coulter, Inc., Indianapolis, IN, USA) to separate debris or a lipid layer. Each 50 µL of serum sample aliquot was mixed with 10 µL of the internal standard, to which 175 µL of prechilled methanol/chloroform (v/v =3/1) was added. After mixed at −20 °C for 20 min and centrifuged at 14,000 g and 4 °C for 20 min, the supernatant was transferred to an autosampler vial (Agilent Technologies, Foster City, CA, USA) carefully. In order to remove chloroform, all samples in autosampler vials were evaporated using a CentriVap vacuum concentrator (Labconco, Kansas City, MO, USA). Each urine sample aliquot of 75 µL was mixed with 10 µL of internal standard and was further lyophilized with a FreeZone dryer equipped with a stopping tray dryer (Labconco, Kansas City, MO, USA).
The samples were further analyzed by a GC-TOFMS system (Pegasus HT, Leco Corp., St. Joseph, MO, USA) coupled to an Agilent 7890B gas chromatograph and a Gerstel multipurpose MPS2 sampler with dual heads (Gerstel, Muehlheim, Germany). An Rxi-5 ms capillary column was used for separation (30 m × 250 µm, 0.25-µm film thickness; Restek Corporation, Bellefonte, PA, USA). The carrier gas was set at a constant flow rate of 1.0 mL/min using helium. Injection and transfer interface temperatures were both set to 270 °C, and source temperature was 220 °C. The analysis was made using electron impact ionization (70 eV) in the full scan mode (m/z 50-500).
Statistical analysis
SPSS version 19.0 (SPSS Inc., Chicago, IL, USA) was used for analysis, and P<0.05 was considered statistically significant. Data are presented as the medians (interquartile ranges, IQRs). T-test, Wilcoxon test and Analysis of Variance (ANOVA) test were performed between two groups or among multiple groups. Chi-square tests were used to compare categorical variables. Partial least squares-discriminant analysis (PLS-DA) and orthogonal partial least squares-discriminant analysis (OPLS-DA) were performed using SIMCA-P + 12.0 (Umetrics AB).
Results
Clinical information of T2DM patients with or without nephropathy
The clinical characteristics of the 88 subjects, including 44 DN and 44 T2DM subjects, are summarized in Table 1. According to clinical and pathological processes, the Mogensen staging method divides DN into 5 phases: glomerular hyperfiltration and renal hypertrophy (DN-I), normal albuminuria (DN-II), early diabetic nephropathy (DN-III), clinical diabetic nephropathy (DN-IV), and end-stage renal failure (DN-V) (22). At present, the clinical diagnosis of DN is mainly based on microalbuminuria, while patients with stage I and II DN have no good diagnostic signs, making the condition difficult to diagnose and intervene early. In the present study, according to the Mogensen staging criteria, patients with DN were further divided into three stages: DN-III (n=13), DN-IV (n=17) and DN-V (n=14).
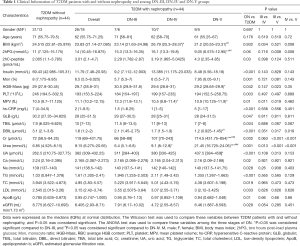
Full table
This study showed that the average T2DM and DN patients gender, age and BMI had no significant differences between the T2DM and DN patients (P>0.05) (Table 1). Compared with the T2DM group, the serum insulin, monocyte ratio, platelet (PLT), total bilirubin (TBIL), direct bilirubin (DBIL), hypersensitive C-reactive protein (hs-CRP), creatinine (Cr), uric acid (UA) and triglyceride (TG) levels of DN patients showed significant differences (P<0.01). Accompanied with the progression of the disease, the serum urea level increased gradually (P<0.05), indicating that the serum urea level may be a potential clinical indicator for the diagnosis of whether T2DM progresses into DN or the severity of DN. The clinical features of other T2DM patients with or without nephropathy are detailed in Table S1.
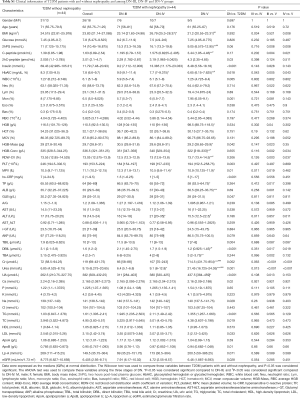
Full table
GC-TOF-MS data analysis of serum and urine in T2DM patients with or without nephropathy
We used GC-TOFMS metabolomics to analyze the serum and urine metabolites of T2DM patients with or without nephropathy. A total of 177 serum metabolites and 159 urine metabolites were determined including amino acids, carbohydrates, fatty acids and organic acids. In order to determine if serum and urine metabolites differed between T2DM patients with or without nephropathy, we constructed OPLS-DA models, which have been broadly used in metabolomics researches (23,24). Typically, R2Y provides the fitting degree of the model to the Y data, while Q2Y is used to estimate the fitting degree of the model to Y. To obtain high predictive ability, Q2Y and R2Y values should be close to 1. As shown in Figure 1A,B, there is a distinct difference in clustering of between DN and T2DM groups. The R2Y and Q2Y were 0.87, 0.822 and 0.797, 0.71 in serum and urine models, respectively, indicating that the models had fine prediction features. The 20-time permutation test showed that the model was steady and reliable (25), with cumulative Q2 at −0.441/−0.265 (Figure S1). As shown in Figure 1C,D, we also obtained differential metabolites by Student's T-test for serum and urine. In Figure 1C, the P values and log 1.5 (fold change, FC) are shown, with cut-off values of 0.05, 0.01 for P value and 1.5 for log.1.5 FC, respectively. The highlighted serum metabolites from the top right corner are positively correlated with subjects on the right side of the OPLS-DA score plot (Figure 1A). The highlighted serum metabolites from the top left corner are negatively correlated with subjects on the right side of the OPLS-DA score plot (Figure 1A), indicating that these metabolites are decreased in these subjects compared to those on the left side of the OPLS-DA score plot. In Figure 1D, the P values and log 1.5 FC are introduced with cut-off values of 0.05 for P value and 1.5 for log.1.5 FC, respectively. The highlighted urinary metabolites from the top right corner are positively correlated with subjects on the right side of the OPLS-DA score plot (Figure 1B). The highlighted urine metabolites from the top left corner are negatively correlated with subjects located on the right side of the OPLS-DA score plot, indicating that these metabolites are decreased in these subjects compared to those on the left side of the OPLS-DA score plot (Figure 1B).
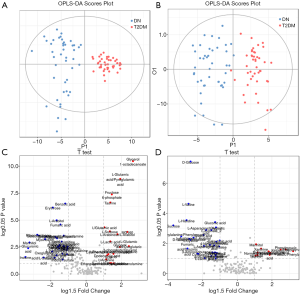
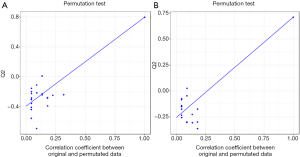
Metabolic features in serum and urine of T2DM patients with or without nephropathy
In order to select potential biomarkers associated with DN, independent sample T-test was performed for the variables between DN and T2DM group, and the differences were statistically significant. Significant differences in metabolites between the two groups (P<0.05) were recognized as potential biomarkers. Then a variable importance plot (VIP) was applied to screen out the important metabolites in the model. In the present research, metabolites with VIP >1 were recognized as candidate biomarkers. According to the above criteria, 61 serum metabolites and 46 urine metabolites were filtered as candidate biomarkers for DN. The summary of the serum and urine biomarker identified in DN is shown in Tables 2 and 3, respectively. More complete information is shown in Supplementary Tables S2 and S3. Among them, the nine most significant changes in serum metabolites are shown in Figure 2A (P<5E-06 and VIP ≥1.5). Compared with T2DM patients, benzoic acid, fumaric acid, erythrose, and L-Arabitol in the DN group were significantly increased. In contrast, glycerol 1-octadecanoate, L-glutamic acid/pyroglutamic acid, fructose 6-phophate, taurine and L-glutamine in DN patients were significantly decreased. The 9 most significant changes in urinary metabolites are shown in Figure 2B (P<5E-04 and VIP ≥1.5). Compared with T2DM patients, urine levels of D-glucose, L-valine, L-histidine, sucrose, gluconic acid, glycine, L-asparagine/L-aspartic acid, L-xylonate-2, and oxalic acid in the DN group were significantly increased.
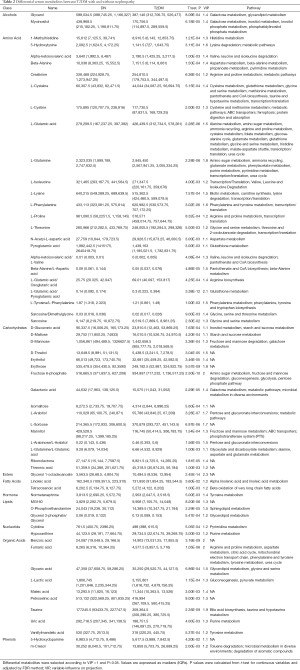
Full table
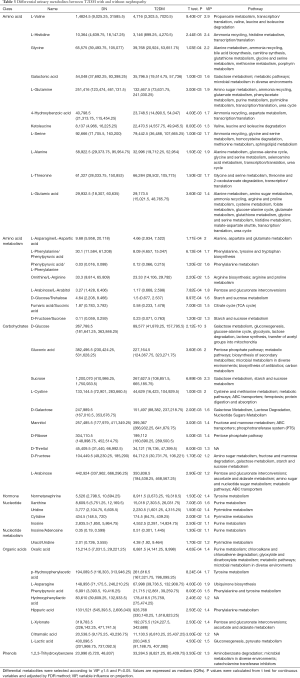
Full table
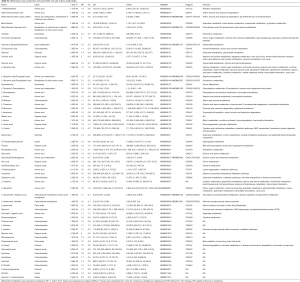
Full table
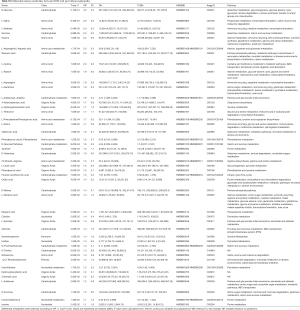
Full table
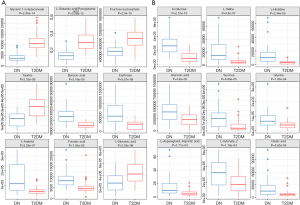
In order to determine which metabolic pathways were major influenced by DN, we summarized pathways analysis based on P values from their impact values and enrichment. As shown in Figure S2, the “metabolome view”, on the based of enrichment analysis (Y-axis) and topology analysis (X-axis), the arrangement shows all metabolic pathways, in which red presents the most significant P values, while yellow and white represent the lowest P values. In order to know the biological significance of the metabolic changes, we conducted a functional enrichment analysis of the experimental data using MetaboAnalyst (26), which provides metabolite set enrichment analysis (MSEA) for metabolites of serum and urine. Then, the metabolic pathways with significant P values <0.05 were screened out (Table 4). Finally, 9 and 12 metabolic pathways were identified with significant differences in serum and urine metabolism, respectively. As the Table 4 shows, 9 metabolic pathways are closely related to the occurrence of serum metabolism of DN, including arginine biosynthesis, galactose metabolism, valine, leucine and isoleucine biosynthesis, starch and sucrose metabolism, alanine, aspartate and glutamate metabolism, glutathione metabolism, D-glutamine and D-glutamate metabolism, glycine, serine and threonine metabolism, and nitrogen metabolism. Additionally, 12 metabolic pathways are closely related to the occurrence of urinary metabolism of DN, including starch and sucrose metabolism, valine, leucine, isoleucine biosynthesis, alanine, aspartate and glutamate metabolism, phenylalanine, tyrosine and tryptophan biosynthesis, glyoxylate and dicarboxylate metabolism, phenylalanine metabolism; glycine, serine and threonine metabolism, D-glutamine and D-glutamate metabolism, arginine biosynthesis, nitrogen metabolism, galactose metabolism, and histidine metabolism. The significant serum and urinary metabolites observed in this study are shown in Figure 3.
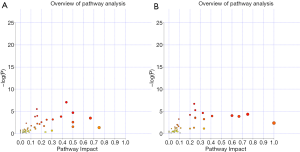
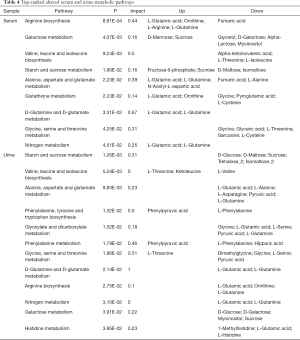
Full table
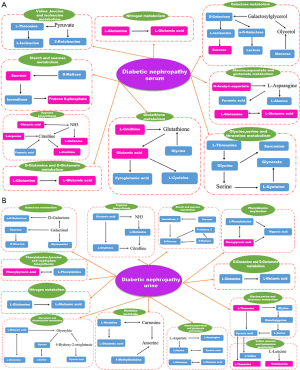
Serum and urinary metabolic profiles in T2DM patients with nephropathy at different stages
We further compared the serum metabolites in three groups of patients with different stages of DN, and the score plots of PLS-DA are shown in Figure 4A. According to the model validation results, the PLS-DA (R2X =0.21; R2Y =0.326; Q2Y =0.29) showed distinct separation among all three groups. A 999-time permutation test was used to validate the reliability of the model, as illustrated in Figure 4B, with R2 =0.132 and Q2 =−0.105. Based on the VIP value of the PLS-DA (VIP >1), combined with ANOVA, 28 potential biomarkers were ultimately identified (P<0.05), including two species of organic acids (vanillylmandelic acid and taurine), ten carbohydrates, six amino acids and other metabolites (Table 5).
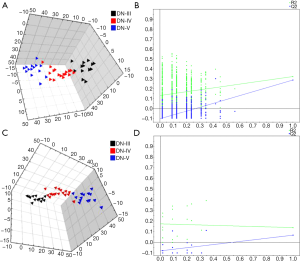
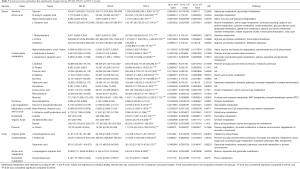
Full table
We also compared the urinary metabolites of the three groups of patients in different DN stages, and the PLS-DA score plot is shown in Figure 4C. PLS-DA model [R2X (cum) =0.09; R2Y (cum) =0.297; Q2Y =0.118] indicated good separation among all three groups. A 999-time permutation test was used to validate the reliability of the model, as illustrated in Figure 4D, with R2 =0.172 and Q2 =−0.079. Based on the VIP value of the PLS-DA (VIP >1), combined with ANOVA, 5 potential biomarkers were ultimately identified (P<0.05), including one organic acid, two types of carbohydrates (galactonic acid and L-arabinose), and their metabolites (Table 5).
Discussion
DN is a serious complication of T2DM. Patients with DN are at a significantly high risk for ESRD, and further the risks for cardiovascular morbidity and mortality increase persistently (3,9). Thus, the early diagnosis of DN can decrease morbidity by allowing for potential therapeutic interventions. However, there is no single diagnostic marker for the detection of DN. Over the last three decades, a large amount of scientific evidence has shown that many inflammatory markers are related to DN, for instance interleukin-1 (IL-1), IL-8, IL-6, tumor necrosis factor-alpha, transforming growth factor beta-1, cytokines, and the neutrophil-lymphocyte ratio in the complete blood count (27,28). Although there have been many studies on the occurrence and development of DN, the overall metabolic changes of T2DM that progress to kidney injury still lack clear characteristics. In this study, we used GC-TOFMS to identify distinct metabolic changes and related pathways during the occurrence and the development of DN in serum and urine samples from patients with DN. Dramatic changes in disease development and progression of DN metabolites could be a potential target for diagnosis or treatment.
Urea is a nitrogen-containing organic end product in protein metabolism, which can remove 80–90% of nitrogen from the human body (29). Recent studies suggest that it is probable that UA and Cr are involved in the pathogenesis of DN (30,31). Compared with T2DM patients, we found that serum UA and Cr levels of DN patients observably increased (P<0.05). Furthermore, when we compared the clinical features of the three stages of DN, we found that serum urea levels increased as the disease progressed (P<0.05). There is a obvious relationship between serum urea levels and disease stage, suggesting that DN progression may be associated with abnormal serum urea. However, we found that three different metabolites among the differential metabolites in serum and urine were involved in the metabolic process of the urea cycle. Those metabolites were fumaric acid, L-glutamine, and L-glutamic acid, which were present at significantly higher levels in the serum of DN patients than in T2DM patients, and L-glutamine, L-alanine and L-glutamate, which were present at significantly lower levels in the urine of DN patients, than in T2DM patients, as shown in Table 2. These changes may be a key warning sign, and if ignored, lead to irreversible kidney damage. However, the detailed mechanisms of serum and urine urea metabolism in T2DM DN need further research.
This research used GC-TOFMS metabolomics approach to identify metabolic changes associated with DN patients (32-34). Our data revealed a variety of organic acid metabolism disorders in DN patients, including lower serum taurine levels and higher serum benzoic acid and fumaric acid levels than in T2DM patients without nephropathy.
Taurine is a lesser-known organic acid in mammalian tissue and has multiple effects, such as bile acid conjugation, osmoregulation, viability and prevention of oxidant-induced tissue injury, and supplements that can stimulate prolactin and insulin release (35,36). Lin et al. (37) detected that taurine administration in diabetic rats conspicuously inhibited any further increase in urinary protein excretion. Winiarska et al. (38) found that taurine had the characteristics of reducing proteinuria and glomerular disease in diabetes. In this study, GC-TOFMS-based metabolomics was used to detect DN-related metabolic changes in serum. Compared with T2DM patients, we observed a significantly decreased serum taurine levels in patients with DN, which suggests that taurine metabolic disorders are associated with diabetic kidney damage. In addition, in order to further assess kidney damage in patients with DN, we also compared the serum taurine levels in different stages of DN. The serum level of taurine decreased stepwise with the aggravation of kidney disease. This result also proves that taurine may be protective against DN (39).
We observed that the serum level of fumaric acid increased significantly in the pathways of arginine biosynthesis and alanine, aspartate, and glutamate metabolism of DN patients compared with the T2DM group. These findings indicate that disordered fumaric acid metabolism may be associated with diabetic renal impairment. Fumaric acid, a dicarboxylic acid, is a precursor to L-malate during the Krebs tricarboxylic acid (TCA) cycle. Zheng et al. (40) showed that the accumulated fumaric acid due to the inactivation of mutations enzyme fumarate hydratase (FH) in the TCA cycle led to oxidative stress. You et al. (41) found that Nox4 inhibited the regulation of fumarate levels, indicating that the TCA cyclic enzyme FH was the downstream target of Nox4. These results indicate that continuous oxidative stress cause diabetic renal injury, while fumaric acid may serve as a possible biomarker for evaluating the progression of DN.
Benzoic acid is a preservative in foods widely and is detoxified by glycine conjugation in human body. After binding to glycine, benzoic acid is excreted as hippuric acid. In this study, serum benzoic acid was significantly elevated in DN patients, probably because of the consumption of serum glycine in DN patients, resulting in a decrease in the binding of benzoic acid to glycine, resulting in the accumulation of benzoic acid and causing renal toxicity (42,43). Whether benzoic acid is a potential biomarker affecting DN progression requires further investigation.
Compared with T2DM patients without nephropathy, the levels of L-glutamic acid and pyroglutamic acid detected in serum samples from DN patients were significantly reduced. L-glutamic acid is a signaling molecule that regulates β-cells and secretes insulin to maintain blood sugar balance in the body (44). Insulin deficiency and increased gluconeogenesis through impaired cellular function can lead to increased L-glutamic acid catabolism, resulting in a significant decrease in L-glutamic acid contents (45). Our research found that serum L-glutamic acid levels differ in T2DM and DN patients, and from DN-III to DN-V, the serum L-glutamic acid content continues to decrease with the severity of kidney disease. Therefore, L-glutamic acid is considered to be a potential biomarker for kidney injury in DN.
We also found changes in carbohydrates in the serum of DN patients, including fructose 6-phosphate, erythrose, and L-Arabitol. The abnormality of these carbohydrates suggested that saccharides plays a vital role in the process of DN. Fructose 6-phosphate, one of the class of organic compounds, is known as hexose phosphates, and is a carbohydrate derivative with a hexose replaced by one or more phosphate groups (46). Elbein (47) reported that glutamine-fructose-6-phosphate aminotransferase is the major rate-limiting enzyme of this pathway, which increases the expression of the glutamine-fructose-6-phosphate transaminase 1 (GFPT1) gene, thereby increasing the susceptibility to diabetes and diabetic nephropathy. We observed a significant decreased serum level of fructose 6-phosphate in DN patients compared with T2DM patients, which suggests that diabetic renal impairment may affect glucose-6-phosphate metabolism. Gluconic acid occurs naturally in fruit. Normally, glucose is provided by glycolysis and cyclic decomposition. In T2DM, there is an obstacle to the catabolism of glucose (48).
Glycerol 1-octadecanoate, a monoacylglyceride or a monoacylglycerol, is a type of glycerol that is covalently bonded to a glycerol molecule by an ester bond through a fatty acid chain (49). Monoacylglycerols are the main end-products in animals during the intestinal digestion of dietary fats by the enzyme pancreatic lipase. They are absorbed directly by intestinal cells and are transformed into triacylglycerols through the monoacylglycerol pathway before being transitted in lymph to the liver (50). Our data show that DN patients had lower serum levels of glycerol 1-octadecanoate than patients with T2DM without nephropathy, which suggests that glycerol 1-octadecanoate metabolism disorder may be related to diabetic kidney damage. Whether glycerol 1-octadecanoate can be a biomarker for the early diagnosis of DN needs further study.
In addition, metabolic disorders in the urine are also traceable. In the present study, T2DM patients with nephropathy excreted daily averages of more urinary l-xylonate-2 and oxalic acid than those without nephropathy. Hyperoxaluria is a metabolic disorder with oxalate crystal deposition in various organs, including the kidney (51). The mechanism leading to hyperoxaluria is an increased load of free fatty acids in the intestine, resulting in reduced binding of calcium to free fatty acids and reduced synthesis of calcium oxalate. Then, an increasing amount of oxalic acid remains free and is absorbed in the intestine, leading to hyperoxaluria (52). Therefore, inhibition of oxalic acid may be a promising treatment strategy for patients with DN.
The levels of L-valine, L-histidine, glycine, L-asparagine, and L-aspartic acid found in urine samples from DN patients were observably elevated, indicating that urine amino acid metabolism associated with the development of DN.
The kidney is an important site of amino acid metabolism. Therefore, abnormal amino acid metabolism may be predicted as kidney damage. L-glycine has been shown the protection roles against several complications of diabetes in recent years (53,54). L-glycine protects the kidneys, but the precise mechanisms by which L-glycine ameliorates DN remain unclear (45). In our population, we observed a significant increase in urine L-glycine concentration in DN patients compared with T2DM patients. Therefore, L-glycine can not only be a drug for the treatment of diabetic complications but also a potential diagnostic biomarker for DN.
The levels of urine glucose, such as D-glucose, sucrose and gluconic acid, found in urine samples of patients with DN were significantly increased. Wei et al. (55) showed that increasing urine levels of TCA cycle intermediates in DN mice, including citrate, cis-aconitate, and fumarate, which might reflect the system pressure caused by hyperglycemia or local influences on kidney impaired tubule transport and mitochondrial function (56). In the ESRD group, the urine contents of galactonic acid and allose were significantly lower than those in the early DN and clinical DN groups. Thus, urine saccharides can be recognized as potential biomarkers for evaluating the progress of DN (57-59).
There are still several limitations in our study. First, the number of patients was relatively small in each group. Second, although we found that serum taurine concentrations were lower in DN patients than in those without nephropathy and decreased along with the progress of nephropathy, direct evidence is lacking to support the association between serum taurine concentration and deterioration of renal function. Third, the study lacks a robust validation in an independent population. In the near future, we plan to select several metabolites that have increased or decreased the most from the GC-TOFMS metabolomics analysis and use targeted metabolomics to quantitatively evaluate the serum or urine levels of those metabolites. In addition, we will combine molecular biology approaches and other methods to further explore factors and the relevant molecular mechanisms that influence the changes of these metabolites and to explain the reasons for the changes of these metabolites.
In addition, we also collected clinical serum and urine samples in other diabetic complications, such as patients with diabetic peripheral neuropathy, and used methods similar to GC-TOFMS-based metabolomics to reveal the metabolic differences between T2DM patients with or without peripheral neuropathy. Some interesting and novel results were found between the two groups, which we will show in the near future.
Conclusions
In the present study, we integrated information regarding serum and urine metabolites using GC-TOFMS-based metabolomics to reveal the differences between T2DM patients with or without nephropathy and metabolic changes in the development of DN. Our results showed that several metabolic disorders occur, including altered organic acid metabolism and disordered glucose metabolism, during the onset and pathogenesis of DN. These results show that GC-TOFMS-based metabolomics is a promising way to reveal potential metabolic pathways to assess the risk of DN complications and to evaluate therapeutic effects.
Acknowledgments
Funding: This work was supported by the National Natural Science Foundation of China (81620108030, 81873076), the Key project of Shanghai 3-year plan (ZY2018-2020-CCCX-2002-01) and Shanghai Talents Development Fund Project (2017090).
Footnote
Conflicts of Interest: The authors have no conflicts of interest to declare.
Ethical Statement: The authors are accountable for all aspects of the work in ensuring that questions related to the accuracy or integrity of any part of the work are appropriately investigated and resolved. All patients who participated in the study offered a signed informed consent form. The ethical approval for the present study was provided by the Ethics Committee of Shanghai University of Traditional Chinese Medicine.
Open Access Statement: This is an Open Access article distributed in accordance with the Creative Commons Attribution-NonCommercial-NoDerivs 4.0 International License (CC BY-NC-ND 4.0), which permits the non-commercial replication and distribution of the article with the strict proviso that no changes or edits are made and the original work is properly cited (including links to both the formal publication through the relevant DOI and the license). See: https://creativecommons.org/licenses/by-nc-nd/4.0/.
References
- International Diabetes Federation. IDF Diabetes Atlas Eighth Edition. 2017.
- Rathmann W, Giani G. Global prevalence of diabetes: estimates for the year 2000 and projections for 2030. Diabetes Care 2004;27:2568-9; author reply 2569. [Crossref] [PubMed]
- Thomas MC, Brownlee M, Susztak K, et al. Diabetic kidney disease. Nat Rev Dis Primers 2015;1:15018. [Crossref] [PubMed]
- American Diabetes A. 10. Microvascular Complications and Foot Care: Standards of Medical Care in Diabetes-2018. Diabetes Care 2018;41:S105-18. [Crossref] [PubMed]
- Bakris GL. Recognition, pathogenesis, and treatment of different stages of nephropathy in patients with type 2 diabetes mellitus. Mayo Clin Proc 2011;86:444-56. [Crossref] [PubMed]
- Zhang L, Wang H, Long J, et al. China Kidney Disease Network(CK-NET) 2014 Annual Data Report. Am J Kidney Dis 2017;69:A4. [Crossref] [PubMed]
- Foundation NK. KDOQI Clinical Practice Guideline for Diabetes and CKD: 2012 update. Am J Kidney Dis 2012;60:850-86. [Crossref] [PubMed]
- Altemtam N, Russell J, El Nahas M. A study of the natural history of diabetic kidney disease(DKD). Nephrol Dial Transplant 2012;27:1847-54. [Crossref] [PubMed]
- Chou CA, Lin CN, Chiu DT, et al. Tryptophan as a surrogate prognostic marker for diabetic nephropathy. J Diabetes Investig 2018;9:366-74. [Crossref] [PubMed]
- Liu J, Wang D, Chen Y, et al. 1H NMR-based metabonomic analysis of serum and urine in a nonhuman primate model of diabetic nephropathy. Mol Biosyst 2013;9:2645-52. [Crossref] [PubMed]
- Fan Y, Li Y, Chen Y, et al. Comprehensive Metabolomic Characterization of Coronary Artery Diseases. J Am Coll Cardiol 2016;68:1281-93. [Crossref] [PubMed]
- Tam ZY, Ng SP, Tan LQ, et al. Metabolite profiling in identifying metabolic biomarkers in older people with late-onset type 2 diabetes mellitus. Sci Rep 2017;7:4392. [Crossref] [PubMed]
- Rossi C, Marzano V, Consalvo A, et al. Proteomic and metabolomic characterization of streptozotocin-induced diabetic nephropathy in TIMP3-deficient mice. Acta Diabetol 2018;55:121-9. [Crossref] [PubMed]
- Li L, Wang C, Yang H, et al. Metabolomics reveal mitochondrial and fatty acid metabolism disorders that contribute to the development of DKD in T2DM patients. Mol Biosyst 2017;13:2392-400. [Crossref] [PubMed]
- Wu T, Xie G, Ni Y, et al. Serum metabolite signatures of type 2 diabetes mellitus complications. J Proteome Res 2015;14:447-56. [Crossref] [PubMed]
- Wu T, Qiao S, Shi C, et al. Metabolomics window into diabetic complications. J Diabetes Investig 2018;9:244-55. [Crossref] [PubMed]
- Xu Y, Wang L, He J, et al. Prevalence and control of diabetes in Chinese adults. JAMA 2013;310:948-59. [Crossref] [PubMed]
- de Boer IH, Rue TC, Hall YN, et al. Temporal Trends in the Prevalence of Diabetic Kidney Disease in the United States. JAMA 2011;305:2532-9. [Crossref] [PubMed]
- Sinn DH, Kang D, Jang HR, et al. Development of chronic kidney disease in patients with non-alcoholic fatty liver disease: A cohort study. J Hepatol 2017;67:1274-80. [Crossref] [PubMed]
- Huang GM, Huang KY, Lee TY, et al. An interpretable rule-based diagnostic classification of diabetic nephropathy among type 2 diabetes patients. BMC Bioinformatics 2015;16 Suppl 1:S5. [Crossref] [PubMed]
- He F, Xia X, Wu XF, et al. Diabetic retinopathy in predicting diabetic nephropathy in patients with type 2 diabetes and renal disease: a meta-analysis. Diabetologia 2013;56:457-66. [Crossref] [PubMed]
- Chawla V, Roshan B. Non-proteinuric diabetic nephropathy. Curr Diab Rep 2014;14:529. [Crossref] [PubMed]
- Wu Q, Zhang H, Dong X, et al. UPLC-Q-TOF/MS based metabolomic profiling of serum and urine of hyperlipidemic rats induced by high fat diet. J Pharm Anal 2014;4:360-7. [Crossref] [PubMed]
- Wang X, Lv H, Zhang A, et al. Metabolite profiling and pathway analysis of acute hepatitis rats by UPLC-ESI MS combined with pattern recognition methods. Liver Int 2014;34:759-70. [Crossref] [PubMed]
- Luan H, Liu LF, Tang Z, et al. Comprehensive urinary metabolomic profiling and identification of potential noninvasive marker for idiopathic Parkinson’s disease. Sci Rep 2015;5:13888. [Crossref] [PubMed]
- Xia J, Wishart DS. Using MetaboAnalyst 3.0 for Comprehensive Metabolomics Data Analysis. Curr Protoc Bioinformatics 2016;55:14.10.1-14.10.91.
- Dhama K, Sachan S, Khandia R, et al. Medicinal and Beneficial Health Applications of Tinospora cordifolia(Guduchi): A Miraculous Herb Countering Various Diseases/Disorders and its Immunomodulatory Effects. Recent Pat Endocr Metab Immune Drug Discov 2017;10:96-111. [Crossref] [PubMed]
- Chen S, Hong SW, Iglesias-de la Cruz MC, et al. The key role of the transforming growth factor-beta system in the pathogenesis of diabetic nephropathy. Ren Fail 2001;23:471-81. [Crossref] [PubMed]
- Pundir CS, Jakhar S, Narwal V. Determination of urea with special emphasis on biosensors: A review. Biosens Bioelectron 2019;123:36-50. [Crossref] [PubMed]
- Razi F, Nasli-Esfahani E, Bandarian F. Association of serum uric acid with nephropathy in Iranian type 2 diabetic patients. J Diabetes Metab Disord 2018;17:71-5. [Crossref] [PubMed]
- Sueud T, Hadi NR, Abdulameer R, et al. Assessing urinary levels of IL-18, NGAL and albumin creatinine ratio in patients with diabetic nephropathy. Diabetes Metab Syndr 2019;13:564-8. [Crossref] [PubMed]
- Cetin E, Civelek S, Andican G, et al. Plasma AGE-peptides and C-peptide in early-stage diabetic nephropathy patients on thiamine and pyridoxine therapy. Minerva Med 2013;104:93-101. [PubMed]
- Hirayama A, Nakashima E, Sugimoto M, et al. Metabolic profiling reveals new serum biomarkers for differentiating diabetic nephropathy. Anal Bioanal Chem 2012;404:3101-9. [Crossref] [PubMed]
- Gong P, Chang X, Chen X, et al. Metabolomics study of cadmium-induced diabetic nephropathy and protective effect of caffeic acid phenethyl ester using UPLC-Q-TOF-MS combined with pattern recognition. Environ Toxicol Pharmacol 2017;54:80-92. [Crossref] [PubMed]
- Koh JH, Lee ES, Hyun M, et al. Taurine alleviates the progression of diabetic nephropathy in type 2 diabetic rat model. Int J Endocrinol 2014;2014:397307. [Crossref] [PubMed]
- Wu G, Yang J, Sun C, et al. Effect of taurine on alcoholic liver disease in rats. Adv Exp Med Biol 2009;643:313-22. [Crossref] [PubMed]
- Lin S, Yang J, Wu G, et al. Preventive effect of taurine on experimental type II diabetic nephropathy. J Biomed Sci 2010;17 Suppl 1:S46. [Crossref] [PubMed]
- Winiarska K, Szymanski K, Gorniak P, et al. Hypoglycaemic, antioxidative and nephroprotective effects of taurine in alloxan diabetic rabbits. Biochimie 2009;91:261-70. [Crossref] [PubMed]
- Han X, Ito T, Azuma J, et al. The quest for an animal model of diabetic nephropathy and the role of taurine deficiency. Adv Exp Med Biol 2015;803:217-26. [Crossref] [PubMed]
- Zheng L, Cardaci S, Jerby L, et al. Fumarate induces redox-dependent senescence by modifying glutathione metabolism. Nat Commun 2015;6:6001. [Crossref] [PubMed]
- You YH, Quach T, Saito R, et al. Metabolomics Reveals a Key Role for Fumarate in Mediating the Effects of NADPH Oxidase 4 in Diabetic Kidney Disease. J Am Soc Nephrol 2016;27:466-81. [Crossref] [PubMed]
- Mitch WE, Brusilow S. Benzoate-induced changes in glycine and urea metabolism in patients with chronic renal failure. J Pharmacol Exp Ther 1982;222:572-5. [PubMed]
- Irwin C, van Reenen M, Mason S, et al. Contribution towards a Metabolite Profile of the Detoxification of Benzoic Acid through Glycine Conjugation: An Intervention Study. PLoS One 2016;11:e0167309. [Crossref] [PubMed]
- Rhee SY, Jung ES, Park HM, et al. Plasma glutamine and glutamic acid are potential biomarkers for predicting diabetic retinopathy. Metabolomics 2018;14:89. [Crossref] [PubMed]
- Du Y, Xu BJ, Deng X, et al. Predictive metabolic signatures for the occurrence and development of diabetic nephropathy and the intervention of Ginkgo biloba leaves extract based on gas or liquid chromatography with mass spectrometry. J Pharm Biomed Anal 2019;166:30-9. [Crossref] [PubMed]
- Tsuruoka M, Hara J, Hirayama A, et al. Capillary electrophoresis-mass spectrometry-based metabolome analysis of serum and saliva from neurodegenerative dementia patients. Electrophoresis 2013;34:2865-72. [PubMed]
- Elbein SC, Zheng H, Jia Y, et al. Molecular screening of the human glutamine-fructose-6-phosphate amidotransferase 1(GFPT1) gene and association studies with diabetes and diabetic nephropathy. Mol Genet Metab 2004;82:321-8. [Crossref] [PubMed]
- Mallipattu SK, Gallagher EJ, LeRoith D, et al. Diabetic nephropathy in a nonobese mouse model of type 2 diabetes mellitus. Am J Physiol Renal Physiol 2014;306:F1008-17. [Crossref] [PubMed]
- Sinha R, Ahn J, Sampson JN, et al. Fecal Microbiota, Fecal Metabolome, and Colorectal Cancer Interrelations. PLoS One 2016;11:e0152126. [Crossref] [PubMed]
- Wang X, Wang J, Rao B, et al. Gut flora profiling and fecal metabolite composition of colorectal cancer patients and healthy individuals. Exp Ther Med 2017;13:2848-54. [Crossref] [PubMed]
- Albersmeyer M, Hilge R, Schrottle A, et al. Acute kidney injury after ingestion of rhubarb: secondary oxalate nephropathy in a patient with type 1 diabetes. BMC Nephrol 2012;13:141. [Crossref] [PubMed]
- Muji A, Moll S, Saudan P. (Oxalate nephropathy: a new entity of acute kidney injury in diabetic patients?). Rev Med Suisse 2015;11:493-4, 6-8.
- Pérez-Torres I, Ibarra B, Soria-Castro E, et al. Effect of glycine on the cyclooxygenase pathway of the kidney arachidonic acid metabolism in a rat model of metabolic syndrome. Can J Physiol Pharmacol 2011;89:899-910. [Crossref] [PubMed]
- Wang Z, Zhang J, Wang L, et al. Glycine mitigates renal oxidative stress by suppressing Nox4 expression in rats with streptozotocin-induced diabetes. J Pharmacol Sci 2018;137:387-94. [Crossref] [PubMed]
- Wei T, Zhao L, Jia J, et al. Metabonomic analysis of potential biomarkers and drug targets involved in diabetic nephropathy mice. Sci Rep 2015;5:11998. [Crossref] [PubMed]
- Salek RM, Maguire ML, Bentley E, et al. A metabolomic comparison of urinary changes in type 2 diabetes in mouse, rat, and human. Physiol Genomics 2007;29:99-108. [Crossref] [PubMed]
- Rainesalo S, Keranen T, Palmio J, et al. Plasma and cerebrospinal fluid amino acids in epileptic patients. Neurochem Res 2004;29:319-24. [Crossref] [PubMed]
- Lim SR, Hyun SH, Lee SG, et al. Potential urinary biomarkers of nephrotoxicity in cyclophosphamide-treated rats investigated by NMR-based metabolic profiling. J Biochem Mol Toxicol 2017. [Crossref] [PubMed]
- Watanabe M, Suliman ME, Qureshi AR, et al. Consequences of low plasma histidine in chronic kidney disease patients: associations with inflammation, oxidative stress, and mortality. Am J Clin Nutr 2008;87:1860-6. [Crossref] [PubMed]