Analysis of potential hub genes involved in the pathogenesis of Chinese type 1 diabetic patients
Introduction
Diabetes has assumed a prevailing trend with 463 million adult being affected worldwide (1). A further 1.1 million children and adolescents were diagnosed with type 1 diabetes (T1D), exerting a heavy burden on the affected families and the society (2). Genetic factors play a crucial role in T1D, which is an autoimmune disease resulting from T cell-mediated destruction of islet β cells (3). The human leukocyte antigen (HLA) genes, as well as other non-HLA genes have been found to be strongly associated with the pathogenesis of T1D (2). By far, HLA class I and II clusters serve a pivotal role in T1DM susceptibility, with more than 90% of early-onset T1D patients carrying at least one copy of the DRB*301-DQB*201. Besides, approximately 20% of the T1D patients have a copy of DRB*401-DQA*301-DQB*302 (4). Insulin (INS) is the gene encoding the preproinsulin peptide, and intriguingly, the function of this gene varies. The short (class I) insulin-linked variable number of tandem repeats (INS-VNTR) alleles confer susceptibility to T1D, but the long (class III) alleles are T1D-protective (5). PTPN22 encodes the lymphocyte specialized tyrosine phosphatase (6). LYP negatively regulates T cell kinase signaling and participates in host defense and immune tolerance; its expression is upregulated in the context of T1D (6). CTLA4 is a gene encoding both a T cell-specific transmembrane co-receptor and a T cell activation negative regulator (7). IL2RA is upregulated in stimulated effector T cells and forkhead box P3 (FOXP3)+-regulated CD4+ cells. Moreover, reduced interleukin-2 (IL-2) expression was found to diminish the regulatory T cells in NOD mice (8).
Most of the studies on T1D genetic predisposition were performed in Caucasian people, whose genetic background is distinct from that of Chinese individuals (9). According to a genome-wide association study (GWAS) in the Chinese Han population which recruited 2,596 autoantibody-positive T1D cases and 5,082 control subjects, the effect sizes of some risk loci were stronger for Chinese T1D patients, such as 6p22.2, 10p14, and 12q13.2, while some were weaker such as INS at 11p15.5 than the Caucasians (9). Furthermore, 13 of the 61 reported loci in the Caucasian T1D patients had little effect in the Chinese population, and other 32 T1D risk loci from Caucasian GWASs were not replicable in Chinese patients (9). Notably, risk loci specific to Chinese Han patients with T1D were identified. The novel loci rs4320356 near BTN3A1, rs3802604 in GATA3 and HLA-C position 275 as well as two reported loci, rs1770 in MHC and rs705699 in SUOX, were proven to be relevant to Chinese T1D patients (9). Although the study extended the knowledge on genetic contribution to Chinese patients with T1D, there are still unexplored risk loci for the disease.
To our knowledge, pancreatic islets are the best choice for exploring the pathogenesis of T1D. Several studies have focused on the target tissue and analyzed the gene expression in T1D patients, however, none of them were in the Chinese population. Peripheral blood mononuclear cells (PBMCs) can be an optimal alternative to pancreatic islets for studying diabetes (10). The GSE55100 dataset, accessed from the Gene Expression Omnibus (GEO) database, is the only database recording the messenger RNA (mRNA) expression of PBMCs in Chinese patients with newly-diagnosed T1D and may help us determine the potentially relevant genes and mechanisms of T1D onset. We analyzed differentially expressed genes (DEGs) and biofunctions related to T1D via bioinformatic analysis. Furthermore, enrichment analysis and functional annotation were conducted to demonstrate the potential processes of T1D progression.
Methods
Data resources
The mRNA expression file of GSE55100 was downloaded from GEO database (http://www.ncbi.nlm.nih.gov/geo), a public data source recoding genetic information (11). Transcriptome data were obtained from the GPL570 platform, Affymetrix Human Genome U133 Plus 2.0 Array. The annotation file in the platform matches the probes with the corresponding genes. GSE55100 contains the mRNA information of PBMCs from 12 patients with newly diagnosed T1D and 10 normal controls. All of the participants were Chinese, and the newly-diagnosed T1D was defined as T1D diagnosis less than 12 weeks with insulin medication (12). The data were provided by Ruijin hospital, Shanghai Jiao Tong University (Shanghai, China).
DEG identification
The DEGs between the T1D patients and controls were analyzed with procedures including data preparation (data normalization and summarization) and DEG analysis (DEG selection and annotation). The affy package in R Software was used for data normalization, and limma package was applied for the remaining processes. The progression of DEG selection included model design, linear model fitness, contrast matrix generation, Bayesian model building and gene filtering, all of which were conducted by the functions in the limma package. Genes with the P<0.05 and |log Fc| (fold change) >1 were considered as DEGs.
Enrichment analysis for DEGs
The Kyoto Encyclopedia of Genes and Genomes (KEGG) (http://www.kegg.jp/ or http://www.genome.jp/kegg/) is a data resource for genes and genomes with assigned corresponding functional significances (13). Gene ontology (GO) is a tool for annotating genes from various ontologies (14). The Database for Annotation, Visualization, and Integrated Discovery (DAVID, version 6.8, http://david.ncifcrf.gov) is a resource for interpreting genes originating from genomic studies with bioinformatic information (8). The GO and KEGG analyses of these genes were performed by the DAVID online database with P<0.05 set as statistically significant. The PPI network was constructed and projected with the Search Tool for the Retrieval of Interacting Genes (STRING, version 10.5) (14) online database, and the interaction was considered statistically significant when a combined score >0.4. The interaction network was visualized with Cytoscape (version 3.6.0), which comprehensively combines biomolecular interaction networks with high-throughput expression data and other molecular states (15). The genes and their connections could be regulated in the software, and we represented the genes with ellipses and the connections with gray full lines. The upregulated genes were selected by setting the default filter with a logFc from 0 to 1.833 (the maximum logFc value) and were colored with a yellow background. Molecular Complex Detection (MCODE, version 1.5.1), a plug-in from Cytoscape, was utilized to find the densely connected regions of PPIs for DEGs (16). Significant gene modules were built by setting the selection criteria as follow: (I) MCODE score greater than or equal to 2; (II) degree cut-off equal to 2; (III) node score cut-off equal to 0.2; (VI) max depth equal to 10; (V) k-score equal to 2.
Hub genes
Genes included in the modules built by MCODE were selected as hub genes. Then, their coexpression network was constructed with Coexpedia (http://www.coexpedia.org), a coexpression database annotating co-functional co-expression information (17). The biological process network of these genes was investigated and envisioned with the Biological Networks Gene Ontology tool (BiNGO, version 3.0.3), a plug-in in Cytoscape (18).
Results
DEGs in T1D
After the expression data, phenotype data and annotation file were processed with the affy and limma packages in R Software. After excluding 11 invalid genes, 78 DEGs, including 42 upregulated genes and 42 downregulated genes, were identified between the newly diagnosed Chinese T1D patients and controls (Table S1). The results were displayed in a volcano plot with red dots representing the upregulated genes (n=42), green dots representing the downregulated genes (n=36) and gray dots representing the non-differentially expressed genes (n=54,586) (Figure 1).
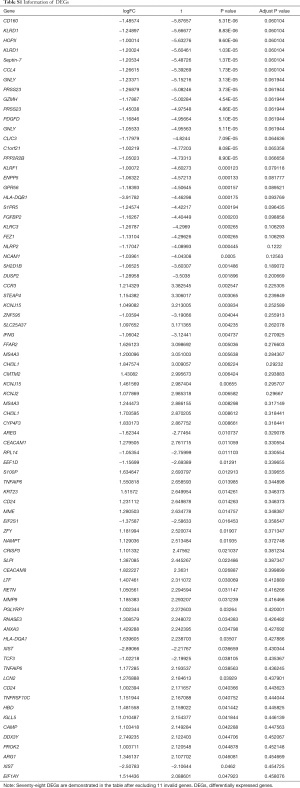
Full table
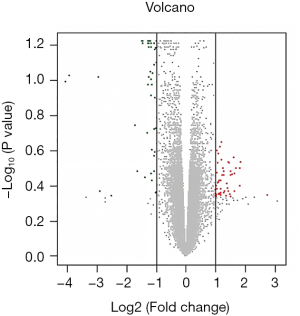
Genes expression data was presented by volcano plots (n=54,664). Red points represented for the up-regulated genes (n=42), green points for the downregulated genes (n=36) and gray points for non-differentially expressed genes (n=54,586).
Enrichment analyses
The enrichment analysis of DEGs performed using DAVID showed that the main DEG biological process changes were collected in immune response, innate immune response, cell adhesion, inflammation response and positive regulation of cell proliferation (Table 1, Figure 2A). Cell component changes were predominantly in the extracellular exosome, extracellular space, extracellular region and integral component of the plasma membrane (Table 1, Figure 2B). The molecular function (MF) changes were largely in cytokine activity, iron ion binding, carbohydrate binding, transmembrane signaling receptor activity and serine-type endopeptidase activity (Table 1, Figure 2C). The KEGG pathway analysis results revealed the potent relationships among DEGs in antigen processing and presentation, influenza A and herpes simplex infection (Table 1, Figure 2D).
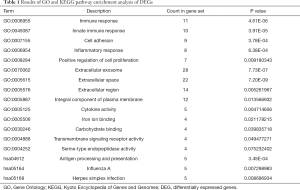
Full table
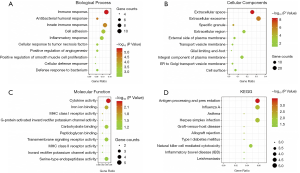
PPI network formation
The DEG PPI network constructed by STRING is shown in Figure 3A, reflecting the outline of the DEG network. Then, the network was analyzed by Cytoscape and was presented in Figure 3B with 36 nodes colored yellow representing the upregulated genes. Two significant modules constructed by MCODE in Cytoscape are presented in Figure 3C and D, including 10 genes (MMP9, ARG1, CAMP, CHI3L1, CRISP3, SLPI, LCN2, PGLYRP1, LTF and RETN) and 3 genes (CEACAM1, CEACAM8 and MS4A3) respectively. The modules were densely connected parts of the PPI network, which might be closely related to the pathogenesis of T1D.
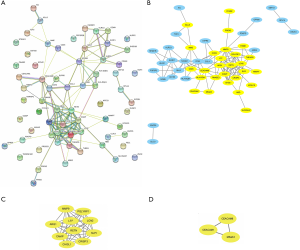
Hub gene selection
Thirteen DEGs with MCODE score ≥2 were selected as hub genes. Genes from the module in Figure 3C had the highest score of 9, whereas those in the other module had a score of 2. Detailed information on hub genes, including gene symbols, full names and gene implications, is shown in Table 2. The coexpression network analyzed by Coexpedia is shown in Figure 4A, presenting the coexpression relationships between hub genes. The biological process network of hub genes explored via BiNGO is shown in Figure 4B, with 31 nodes representing various biological processes and 52 edges representing the connections. In the network, deeper colors represented higher frequencies and 5 nodes with deep yellow backgrounds, i.e., citrulline metabolic process, argininosuccinate metabolic process, arginine biosynthetic process, arginine metabolic process and glutamine family amino acid biosynthesis were with the highest frequencies.
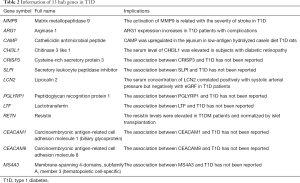
Full table
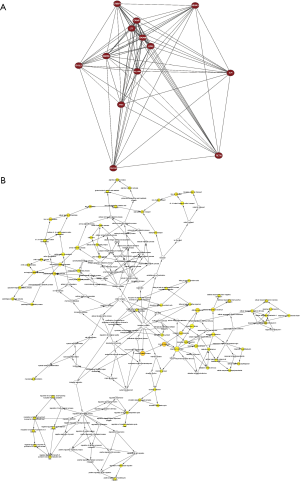
Discussion
Diabetes has become one of the most important chronic disease with a rapidly increasing prevalence during the past decade (19). In contrast to type 2 diabetes (T2D), T1D is an autoimmune-mediated disease resulting from a complex crosstalk between genetic factors and environmental determinants (20,21). MHC genes as well as non-MHC genes have been proven to be involved in the pathogenesis of T1D (22). Understanding the contributory genes is crucial for revealing the underlying mechanisms of T1D and establishing risk prediction models. Researches on etiology-related genes have achieved significant progress; however, obtaining insight into the gene interactions and molecular mechanisms of the disease still has a long way to go.
In this study, we analyzed a microarray dataset based on PBMCs from T1D patients and normal controls, and 89 DEGs were identified. Then, we analyzed the DEGs using GO and KEGG enrichment analyses. The DEGs appeared to be mainly related to the immune response, extracellular exosome, cytokine activity and antigen processing and presentation. T1D is a T lymphocyte-mediated, B lymphocytes-assisted autoimmune disease (2,3); therefore, it is not surprising to find that the DEGs were enriched in immune processes. The results revealed that extracellular exosomes possibly played a role in the etiology of T1D. T1D is an organ-specific autoimmune disease with the target autoantigens on the intracellular membrane; however, the mechanisms of how they initially encounter the immune system is inadequately understood. Cianciaruso et al. (23) found that those intracellular β-cell autoantigens were released in small vesicles, termed exosomes, by pancreatic islets in rats and humans with T1D. When these exosomes anchored GAD65 to exosome-mimetic liposomes, they were taken up and processed by activated dendritic cells (23). Therefore, it could be proposed that stress-induced intracellular autoantigen exosomal and immunostimulatory chaperon release might be related to the autoimmune response initiation in T1D.
Among the DEGs, 13 hub genes were clustered in DEG networks, which may be pivotal to the pathogenesis of T1D. Among these genes, six genes, including CHI3L1, ARG1, MMP9, CAMP, LCN2 and RETN, have been found to be associated with T1D.
CHI3L1, also called YKL-40, encodes the inflammatory biomarker YKL-40 and participates in endothelial dysfunction and atherosclerosis (24). Decreased methylation but increased expression of CHI3L1 have been found in T1D patients with proliferative diabetic retinopathy (25). Furthermore, ARG1 is a gene related to diabetic complications (26), and its expression was upregulated in the muscle homogenates of type 1 diabetic mice (27). Kalani et al. (28) found more severe stroke in the T1D mice than in control mice, which might be related to the intensively activation of MMP9. Moreover, CAMP is a multifunctional antimicrobial molecule, and immunomodulatory peptide colocalize with CD163+ M2 macrophages. Diabetes-prone BioBreeding (BBdp) rats fed a low-antigen hydrolyzed casein (HC) diet had a lower prevalence of T1D than that of the control cereal-fed group. And CAMP expression was upregulated in the jejunum of the experimental group, suggesting that CAMP may be a potentially protective factor for T1D (29). Notably, LCN2, also known as neutrophil gelatinase-associated lipocalin (NGAL), is secreted by multiple types of cells and is regarded as a marker of inflammation as well as ischemic renal injury (30). The serum concentration of LCN2 was found to be positively related to systolic arterial pressure but negatively to eGRF, and the molecule could be considered an early biomarker for kidney dysfunction in T1D individuals without albuminuria (31). Resistin, an adipocyte-secreted hormone expressed by RETN, might be involved in glucose homeostasis in mice and human (32-34). The elevated resistin concentration in T1D subjects could be reversed after islet transplantation, implying that resistin could possibly participate in the pathogenesis of T1D (34).
Relationships between T1D and the hub genes LTF, SLPI, PGLYRP1, CRISP3, CEACAM1, CEACAM8 and MS4A3 have not been reported, however, most of them were found to be involved in the immune reaction and metabolic regulation, thus may be potentially related with diabetic pathogenesis.
LTF is an inherent immune glycoprotein with anti-inflammatory properties (35). Circulating LTF levels have been demonstrated to be negatively linked with factors including fasting triglycerides, glucose concentration, body mass index and waist-to-hip ratio but to be positively associated with HDL cholesterol level (36). Moreover, LTF has been demonstrated to promote the insulin-signaling reaction in mature adipocytes by increasing AKT phosphorylation (37). However, the relationship between LTF and diabetes, particular T1D, has not been fully understood. SLPI, an antiprotease released by neutrophils and mucous membranes, is a powerful inhibitor of the inflammatory cascade in metabolic diseases. López-Bermejo et al. (38) figured out that the concentrations of SLPI were increased in men with metabolic syndrome as well as T2D, but the underlying mechanisms remain to be clarified. Additionally, AMP-activated protein kinase (AMPK) activation was effective for T2D treatment and also led to the downregulation of PGLYRP1, suggesting that PGLYRP1 might participate in the glucose metabolism dysregulation (39). CEACAM1 is a transmembrane glycoprotein that is involved in the maintenance of epithelial cell polarity, insulin sensitivity regulation, liver tolerance and mucosal immunity. Furthermore, CEACAM1-L was found to participate in the regulation of T-cell receptor (TCR)- and IL-12-related signaling pathway. Given that T cells and IL-12 are implicated in T1D pathogenesis, CEACAM1 might be related to T1D onset (40). CEACAM8 (or CD66b) is a surface adhesion molecule of neutrophils. Interestingly, the levels of the CEACAM8 were higher in T2D patients than in normal controls, which has not been duplicated in T1D patients (41). MS4A3 demonstrated decreased methylation in adipose tissues of patients with T2D (42), and it has been reported that gestational diabetes and maternal obesity are related to decreased MS4A3 methylation in children (43). Notwithstanding, the interactions between MS4A3 and T1D remain unrevealed. Moreover, there has been no evidence on the relationships between CRISP3 and diabetes.
The key strength of our study is that we identified potential genes associated with T1D pathogenesis. Given the complexity of T1D pathogenesis, these genes provide novel perspectives for the extensive comprehension of the disease. These genes are relevant to diverse aspects of T1D, including disease onset, development, complications and treatments. Further exploration of these genes might help to figure out the underlying mechanisms of T1D.
Our study had limitations. First, we compared the DEGs with the reported T1D risk genes from the GWASs performed both in Chinese and Caucasian individuals (9), but no overlaps were identified, possibly owning to the limited number of samples in our study. Second, the expression of the identified molecules and related processes in patients with T1D were not validated at the protein levels. Thereby, further experimental and clinical studies are warranted to confirm the results. Finally, the mRNAs we studied were from PBMCs rather than the pancreatic islets, the disease tissue of interest, which may cause inaccuracy. However, given the integrality of the immune system, the information from PBMCs could provide clues to the changes in the pancreatic islets.
Conclusions
In conclusion, we identified 78 DEGs and 13 hub genes potentially associated with T1D via bioinformatic analysis, which might serve as candidate diagnostic molecular and therapeutic recommendation for T1D. However, further studies are required to verify their functions in T1D.
Acknowledgments
Funding: None.
Footnote
Conflicts of Interest: The authors have no conflicts of interest to declare.
Ethical Statement: The authors are accountable for all aspects of the work in ensuring that questions related to the accuracy or integrity of any part of the work are appropriately investigated and resolved.
Open Access Statement: This is an Open Access article distributed in accordance with the Creative Commons Attribution-NonCommercial-NoDerivs 4.0 International License (CC BY-NC-ND 4.0), which permits the non-commercial replication and distribution of the article with the strict proviso that no changes or edits are made and the original work is properly cited (including links to both the formal publication through the relevant DOI and the license). See: https://creativecommons.org/licenses/by-nc-nd/4.0/.
References
- Available online: https://www.idf.org/. Accessed 14th Jan International Diabetes Federation. The IDF Diabetes Atlas 9th Edition, 2020.
- Polychronakos C, Li Q. Understanding type 1 diabetes through genetics: advances and prospects. Nat Rev Genet 2011;12:781-92. [Crossref] [PubMed]
- van Belle TL, Coppieters KT, von Herrath MG. Type 1 diabetes: etiology, immunology, and therapeutic strategies. Physiol Rev 2011;91:79-118. [Crossref] [PubMed]
- Erlich H, Valdes AM, Noble J, et al. HLA DR-DQ haplotypes and genotypes and type 1 diabetes risk: analysis of the type 1 diabetes genetics consortium families. Diabetes 2008;57:1084-92. [Crossref] [PubMed]
- Anjos S, Polychronakos C. Mechanisms of genetic susceptibility to type I diabetes: beyond HLA. Mol Genet Metab 2004;81:187-95. [Crossref] [PubMed]
- Vang T, Congia M, Macis MD, et al. Autoimmune-associated lymphoid tyrosine phosphatase is a gain-of-function variant. Nat Genet 2005;37:1317-9. [Crossref] [PubMed]
- Ukkola O, Santaniemi M. Adiponectin: a link between excess adiposity and associated comorbidities? J Mol Med (Berl) 2002;80:696-702. [Crossref] [PubMed]
- Huang D. W., Sherman B. T., Tan Q., et al. DAVID Bioinformatics Resources: expanded annotation database and novel algorithms to better extract biology from large gene lists. Nucleic Acids Res 2007;35:W169-75. [Crossref] [PubMed]
- Zhu M, Xu K, Chen Y, et al. Identification of Novel T1D Risk Loci and Their Association With Age and Islet Function at Diagnosis in Autoantibody-Positive T1D Individuals: Based on a Two-Stage Genome-Wide Association Study. Diabetes Care 2019;42:1414-21. [Crossref] [PubMed]
- Wang X, Jia S, Geoffrey R, et al. Identification of a molecular signature in human type 1 diabetes mellitus using serum and functional genomics. J Immunol 2008;180:1929-37. [Crossref] [PubMed]
- Zeng M, Liu J, Yang W, et al. Identification of key biomarkers in diabetic nephropathy via bioinformatic analysis. J Cell Biochem 2018. [Epub ahead of print]. [PubMed]
- Yang M, Ye L, Wang B, et al. Decreased miR-146 expression in peripheral blood mononuclear cells is correlated with ongoing islet autoimmunity in type 1 diabetes patients 1miR-146. J Diabetes 2015;7:158-65. [Crossref] [PubMed]
- Ashburner M, Ball CA, Blake JA, et al. Gene ontology: tool for the unification of biology. The Gene Ontology Consortium. Nat Genet 2000;25:25-9. [Crossref] [PubMed]
- Szklarczyk D, Morris JH, Cook H, et al. The STRING database in 2017: quality-controlled protein-protein association networks, made broadly accessible. Nucleic Acids Res 2017;45:D362-D368. [Crossref] [PubMed]
- Shannon P, Markiel A, Ozier O, et al. Cytoscape: a software environment for integrated models of biomolecular interaction networks. Genome Res 2003;13:2498-504. [Crossref] [PubMed]
- Sun C, Yuan Q, Wu D, et al. Identification of core genes and outcome in gastric cancer using bioinformatics analysis. Oncotarget 2017;8:70271-80. [PubMed]
- Yang S, Kim CY, Hwang S, et al. COEXPEDIA: exploring biomedical hypotheses via co-expressions associated with medical subject headings (MeSH). Nucleic Acids Res 2017;45:D389-D396. [Crossref] [PubMed]
- Maere S, Heymans K, Kuiper M. BiNGO: a Cytoscape plugin to assess overrepresentation of gene ontology categories in biological networks. Bioinformatics 2005;21:3448-9. [Crossref] [PubMed]
- Hong J, Zhang Y, Lai S, et al. Effects of metformin versus glipizide on cardiovascular outcomes in patients with type 2 diabetes and coronary artery disease. Diabetes Care 2013;36:1304-11. [Crossref] [PubMed]
- Xia Y, Xie Z, Huang G, et al. Incidence and trend of type 1 diabetes and the underlying environmental determinants. Diabetes Metab Res Rev 2019;35:e3075. [Crossref] [PubMed]
- Atkinson MA, Eisenbarth GS, Michels AW. Type 1 diabetes. Lancet (London, England) 2014;383:69-82. [Crossref] [PubMed]
- Winkler C, Krumsiek J, Buettner F, et al. Feature ranking of type 1 diabetes susceptibility genes improves prediction of type 1 diabetes. Diabetologia 2014;57:2521-9. [Crossref] [PubMed]
- Cianciaruso C, Phelps EA, Pasquier M, et al. Primary Human and Rat β-Cells Release the Intracellular Autoantigens GAD65, IA-2, and Proinsulin in Exosomes Together With Cytokine-Induced Enhancers of Immunity. Diabetes 2017;66:460-73. [Crossref] [PubMed]
- Rathcke CN, Vestergaard H. YKL-40--an emerging biomarker in cardiovascular disease and diabetes. Cardiovasc Diabetol 2009;8:61. [Crossref] [PubMed]
- Agardh E, Lundstig A, Perfilyev A, et al. Genome-wide analysis of DNA methylation in subjects with type 1 diabetes identifies epigenetic modifications associated with proliferative diabetic retinopathy. BMC Med 2015;13:182. [Crossref] [PubMed]
- Forbes JM, Cooper ME. Mechanisms of diabetic complications. Physiol Rev 2013;93:137-88. [Crossref] [PubMed]
- Guimarães JPT, Filgueiras LR, Martins JO, et al. Leukotriene Involvement in the Insulin Receptor Pathway and Macrophage Profiles in Muscles from Type 1 Diabetic Mice. Mediators Inflamm 2019;2019:4596127.
- Kalani A, Kamat PK, Tyagi N. Diabetic Stroke Severity: Epigenetic Remodeling and Neuronal, Glial, and Vascular Dysfunction. Diabetes 2015;64:4260-71. [Crossref] [PubMed]
- Patrick C, Wang GS, Lefebvre DE, et al. Promotion of autoimmune diabetes by cereal diet in the presence or absence of microbes associated with gut immune activation, regulatory imbalance, and altered cathelicidin antimicrobial Peptide. Diabetes 2013;62:2036-47. [Crossref] [PubMed]
- Moschen AR, Adolph TE, Gerner RR, et al. Lipocalin-2: A Master Mediator of Intestinal and Metabolic Inflammation. Trends Endocrinol Metab 2017;28:388-97. [Crossref] [PubMed]
- Papadopoulou-Marketou N, Margeli A, Papassotiriou I, et al. NGAL as an Early Predictive Marker of Diabetic Nephropathy in Children and Young Adults with Type 1 Diabetes Mellitus. J Diabetes Res 2017;2017:7526919.
- McTernan CL, McTernan PG, Harte AL, et al. Resistin, central obesity, and type 2 diabetes. Lancet (London, England) 2002;359:46-7. [Crossref] [PubMed]
- Rajala MW, Obici S, Scherer PE, et al. Adipose-derived resistin and gut-derived resistin-like molecule-beta selectively impair insulin action on glucose production. J Clin Invest 2003;111:225-30. [Crossref] [PubMed]
- Shalev A, Patterson NB, Hirshberg B, et al. Resistin serum levels in type 1 diabetes pre- and post-islet transplantation. Metabolism 2004;53:403-4. [Crossref] [PubMed]
- Ward PP, Paz E, Conneely OM. Multifunctional roles of lactoferrin: a critical overview. Cell Mol Life Sci 2005;62:2540-8. [Crossref] [PubMed]
- Moreno-Navarrete JM, Ortega FJ, Bassols J, et al. Association of circulating lactoferrin concentration and 2 nonsynonymous LTF gene polymorphisms with dyslipidemia in men depends on glucose-tolerance status. Clin Chem. 2008;54:301-9. [Crossref] [PubMed]
- Mayeur S, Spahis S, Pouliot Y, et al. Lactoferrin, a Pleiotropic Protein in Health and Disease. Antioxid Redox Signal 2016;24:813-36. [Crossref] [PubMed]
- López-Bermejo A, Ortega FJ, Castro A, et al. The alarm secretory leukocyte protease inhibitor increases with progressive metabolic dysfunction. Clin Chim Acta 2011;412:1122-6. [Crossref] [PubMed]
- Grempler R, Wolff M, Simon E, et al. Discovery and translation of a target engagement marker for AMP-activated protein kinase (AMPK). PloS One 2018;13:e0197849. [Crossref] [PubMed]
- Horst AK, Najjar SM, Wagener C, et al. CEACAM1 in Liver Injury, Metabolic and Immune Regulation. Int J Mol Sci 2018. [Crossref] [PubMed]
- de Vries MA, Alipour A, Klop B, et al. Glucose-dependent leukocyte activation in patients with type 2 diabetes mellitus, familial combined hyperlipidemia and healthy controls. Metabolism 2015;64:213-7. [Crossref] [PubMed]
- Nilsson E, Jansson PA, Perfilyev A, et al. Altered DNA methylation and differential expression of genes influencing metabolism and inflammation in adipose tissue from subjects with type 2 diabetes. Diabetes 2014;63:2962-76. [Crossref] [PubMed]
- Hjort L, Martino D, Grunnet LG, et al. Gestational diabetes and maternal obesity are associated with epigenome-wide methylation changes in children. JCI Insight 2018. [Crossref] [PubMed]